Abstract
Aim:
To determine temporal changes in the frequency of asthma and mental illness in California emergency department (ED) visits and examine predictors of both asthma diagnosis and non-routine discharge from asthma visits.
Methods:
Public-use, all-payer ED data from non-federal, acute-care hospitals (2005–2014) were obtained for cross-sectional analysis. Due to substantial missing data, we used fully conditional specification multiple imputation with discriminant functions for age group, sex, race, and ethnicity. Multivariable logistic regression was used to examine asthma diagnosis (yes/no) among all ED visits and non-routine discharge (sent home vs. all else) among visits with asthma diagnosis. Primary independent variables were mental illness and the 3-digit zipcode of the patient’s residence. Covariates included demographics, payer type, and hospital characteristics.
Results:
During 2005–2014 there were 96,180,176 visits at 349 hospitals, and asthma diagnosis increased from 3.3% of ED visits in 2005 to 5.9% in 2014. However, asthma as a primary diagnosis decreased from 1.7% to 1.4% of ED visits. Among visits with asthma diagnosis (n = 4,419,629), co-occurring mood disorders increased from 2.1% in 2005 to 9.2% in 2014. Predictors of asthma diagnosis included attention deficit/conduct disorders [adjusted odds ratio (AOR) 1.41, 95% confidence interval (1.40–1.42)] and mood disorders [AOR 1.37, (1.36–1.37)]. Compared to Los Angeles, cities/areas most associated with asthma diagnosis were Richmond [zipcode 948, AOR 1.22 (1.20–1.24)], Bakersfield [933, AOR 1.21 (1.19–1.24)], and San Bernardino [924, AOR 1.20 (1.19–1.22)]. Ninety-six percent of ED visits with asthma resulted in routine discharge. Predictors of non-routine discharge included suicide/self-harm [AOR 4.74 (4.67–4.81)], schizophrenia [1.97 (1.94–1.99)], and mood disorders [1.35 (1.34–1.36)]. Areas associated with non-routine discharge included the Bakersfield vicinity [932, 1.29 (1.17–1.41)] and Ventura [930, 1.23 (1.10–1.38)].
Conclusions:
Increased co-occurring mental illness among asthma-related ED visits suggests a need to improve care among those having co-occurrence. Understanding regional differences in asthma-related ED visits and hospitalization may improve interventions.
Keywords
Asthma, emergency service, hospital, schizophrenia, mood disorders, anxiety disorders, citiesIntroduction
Asthma prevalence varies by multiple factors, particularly socio-demographics [1]. Surveillance data from the 2021 National Health Interview Survey revealed an overall prevalence of 7.7% of current asthma in the United States, with a rate of 6.5% among children/youth and 8.0% among adults 18 years of age and greater [2]. Females had a higher rate of asthma than males, 8.9% vs. 6.5%, respectively, as did those below the federal poverty level (FPL: 10.4%) versus 6.8% among those at greater than 450% of FPL [2]. In addition, the overall prevalence of current asthma ranged from 4.1% among Asians to 10.9% among Blacks and 12.3% among American Indian/Alaska Natives [2–4].
There are complex interactions of socio-demographics and geography regarding asthma prevalence. A study of U.S. counties found temperature to be negatively associated with asthma and poverty to be positively associated [5]. Higher rates of smoking and depression were also associated with higher rates of asthma [5]. Research in Spain regarding lifestyle, socioeconomic, and climate indicators found that lifestyle was most closely tied to the prevalence of asthma in most of the study area, though in some areas urban characteristics and local climate had a stronger impact [6]. As one example of human/environment interaction, research of three U.S. cities, including Los Angeles, found that extreme heat and ozone had the strongest asthma impact on elderly adults [7].
National Hospital Ambulatory Medical Care Survey data from 2010 to 2015 indicated 1.77 million asthma-related emergency department (ED) visits per year, with 1.32% of all U.S. ED visits being asthma-related [3]. Multiple factors contribute to asthma treatment. For example, age and sex have a significant role in when an individual will seek medical attention for asthma [8], such as women in their forties being more likely to seek medical care compared to men [9]. African Americans have a higher rate of ED visits [10], while those who are non-white [11] and lower income are more likely to be hospitalized [12]. Depression is associated with reduced adherence to treatment and increased risk of hospitalization [13]. Among patients with asthma, those reporting psychological distress were more likely to have ED visits than those having no psychological distress [14]. A national study of those with asthma found that after an ED visit, whites and females were most likely to be hospitalized; there were seasonal trends, children with Medicaid were less likely to be hospitalized while adults with Medicaid were more likely, and the likelihood was highest among those living in lower-income zipcodes [15]. Furthermore, limited access to medical care, medications, and monitoring technology, as well as other barriers to treatment adherence all contribute to unnecessary acute care [4]. Some of the barriers to asthma medication adherence include binge drinking, smoking, and being overweight [16].
The current study focused on ED visits in one large state—California. Objectives were to: 1) determine the occurrence of asthma diagnoses and explore the association of hospital and patient characteristics, particularly mental illness and patient residence on the presence of asthma; 2) explore the association of patient and hospital characteristics on the discharge status of asthma ED visits; 3) explore changes over time in asthma and mental illness diagnoses.
Materials and methods
Data & subjects
Public-use ED data on CD-ROMs were obtained from the California Office of Statewide Health Planning and Development (OSHPD) for general acute-care hospitals, excluding federal hospitals. Though public-use CDs are no longer available, researchers may obtain hospital-related data through the Department of Health Care Access and Information (HCAI).
The utilization data included 97,639,808 ED visits between January 1, 2005, and December 31, 2014. Annual hospital-level summary measures [17] were merged with utilization data using a hospital identification code and year of visit. Of ED visits, 1.49% were not matched to hospital data and thus dropped, with the final analysis being done using 96,180,176 visits at 349 unique hospitals.
Measures
Analysis was guided by Andersen’s Behavioral Model of Health Services Utilization [18]. The conceptual model considers the effects of contextual factors, such as time, environment, and healthcare system as well as individual characteristics (predisposing, enabling, and need) on access to healthcare and outcomes. With this theoretical model, it is appropriate to use reasonable measures for each element of the model, even if a specific variable is not significantly associated with the outcome. Equitable access occurs when the clinical need for services is the biggest predictor of utilization and inequitable to the extent that individual predisposing factors such as race and sex, enabling measures such as income and insurance, and contextual measures including the healthcare “system” dominate observed utilization [18]. The two outcome measures were any asthma diagnosis (yes/no) among all ED visits and non-routine disposition (yes/no) among asthma-related visits. This mostly included hospitalization and transfer to another facility but also included leaving against medical advice and mortality [19].
ED data included the year treated, demographics, expected source of payment, primary diagnosis, up to 24 secondary diagnoses, and disposition of discharge [20]. Primary diagnosis is generally the main reason for the visit and the main billable code. Asthma was measured by the presence of an International Classification of Diseases, Ninth Edition (ICD-9: 493) diagnosis of asthma. Mental illness was considered a personal predisposing—vulnerable domain measure [21]. It was measured using the Clinical Classification Software (CCS) schema for ICD-9 [22] developed by the Agency for Healthcare Research and Quality. CCS categorizes thousands of individual ICD-9 codes into 270 clinically meaningful categories. Five groups of mental illness are shown in Table 1.
Descriptive statistics for ED visits, by asthma diagnosis
Measures | No Asthma (%)n = 91,760,547Percent 95.40% | Asthma (%)n =4,419,629Percent 4.60% | p-value* |
---|---|---|---|
Context | |||
Year of visit | < 0.0001 | ||
2005 | 8.81 | 6.15 | |
2006 | 8.74 | 6.54 | |
2007 | 9.01 | 7.13 | |
2008 | 9.28 | 7.72 | |
2009 | 10.22 | 9.50 | |
2010 | 10.01 | 10.32 | |
2011 | 10.31 | 11.37 | |
2012 | 10.80 | 12.63 | |
2013 | 11.12 | 13.55 | |
2014 | 11.70 | 15.10 | |
Hospital control | < 0.0001 | ||
Government | 17.20 | 13.80 | |
For-Profit | 15.29 | 13.38 | |
Non-Profit | 67.51 | 72.82 | |
Hospital teaching/location | < 0.0001 | ||
Urban, non-teaching | 77.33 | 78.96 | |
Rural, non-teaching | 8.46 | 7.53 | |
Urban, teaching | 14.21 | 13.51 | |
Hospital licensed bed size | < 0.0001 | ||
1 to 49 beds | 3.32 | 2.41 | |
50 to 99 beds | 4.86 | 4.76 | |
100 to 149 beds | 9.96 | 9.50 | |
150 to 199 beds | 12.43 | 13.26 | |
200 to 299 beds | 20.01 | 22.21 | |
300 to 499 beds | 35.24 | 35.30 | |
500+ beds | 14.20 | 12.56 | |
Hospital system status | < 0.0001 | ||
In system of 3+ hospitals | 57.06 | 61.52 | |
Not in a system | 42.94 | 38.48 | |
DSH status | < 0.0001 | ||
DSH hospital | 35.71 | 34.07 | |
Non-DSH hospital | 64.29 | 65.93 | |
Hospital Kaiser Permanente status | < 0.0001 | ||
Non-Kaiser | 86.86 | 80.93 | |
Kaiser | 13.14 | 19.07 | |
Pre-disposing+ | |||
Age group | < 0.0001 | ||
0 to 17 years | 2.46 | 1.06 | |
18 to 64 years | 53.11 | 50.74 | |
65 years and greater | 7.90 | 4.84 | |
Unknown | 36.53 | 43.36 | |
Sex | < 0.0001 | ||
Male | 27.43 | 23.07 | |
Female | 32.65 | 31.12 | |
Unknown | 39.92 | 45.81 | |
Race | < 0.0001 | ||
White | 35.33 | 29.38 | |
Non-White | 18.91 | 20.28 | |
Unknown | 45.76 | 50.34 | |
Ethnicity | < 0.0001 | ||
Hispanic | 19.17 | 16.19 | |
Non-Hispanic | 32.71 | 31.74 | |
Unknown | 48.11 | 52.07 | |
Age group | < 0.0001 | ||
0 to 17 years | 4.07 | 2.87 | |
18 to 64 years | 83.39 | 86.74 | |
65 years and greater | 12.54 | 10.39 | |
Sex+ | < 0.0001 | ||
Male | 43.24 | 41.32 | |
Female | 56.76 | 58.68 | |
Race | < 0.0001 | ||
White | 63.65 | 60.56 | |
Non-white | 36.35 | 39.44 | |
Ethnicity | < 0.0001 | ||
Hispanic | 37.96 | 36.39 | |
Non-Hispanic | 62.04 | 63.61 | |
Predisposing-vulnerable domain | |||
CCS 651 anxiety disorders | < 0.0001 | ||
Yes | 3.10 | 5.03 | |
No | 96.90 | 94.97 | |
CCS 652 attention-deficit, conduct, and disruptive behavior disorders | < 0.0001 | ||
Yes | 0.24 | 0.70 | |
No | 99.76 | 99.30 | |
CCS 657 mood disorders | < 0.0001 | ||
Yes | 2.85 | 6.16 | |
No | 97.15 | 93.84 | |
CCS 659 schizophrenia and other psychotic disorders | < 0.0001 | ||
Yes | 1.02 | 1.12 | |
No | 98.98 | 98.88 | |
CCS 662 suicide and intentional self-inflicted injury | < 0.0001 | ||
Yes | 0.69 | 0.55 | |
No | 99.31 | 99.45 | |
Enabling | |||
Payer source | < 0.0001 | ||
Self-pay | 16.38 | 12.66 | |
Medicare | 10.35 | 8.60 | |
Medicaid | 27.95 | 34.87 | |
Private insurance | 38.55 | 38.59 | |
Other government | 3.48 | 2.27 | |
Other | 3.30 | 3.01 | |
Need | |||
Asthma as a primary diagnosis | < 0.0001 | ||
Yes | 0.00 | 33.93 | |
No | 100.00 | 66.07 | |
Outcome | |||
Discharge status | < 0.0001 | ||
Routine/home | 93.95 | 96.00 | |
Admitted to inpatient | 2.06 | 1.66 | |
Left against medical advice | 2.23 | 1.09 | |
Died | 0.17 | 0.06 | |
Court/law enforcement | 0.18 | 0.14 | |
Other, such as long-term care | 1.40 | 1.05 |
CCS: Clinical Classification Software; ED: emergency department; DSH: Disproportionate Share Hospital; *: comparisons are for groups, not individual levels; +: imputed demographics based on averages of 961 million records
The three-digit zip code for patient residence served as the primary context/environment measure, with all zips outside of California being recoded to one value and another for homeless/missing data. Summary hospital data served as a group of context measures and included OSHPD-defined categories for licensed bed size, academic affiliation/rural status, ownership type, any receipt of Disproportionate Share funds (for serving many low-income patients), and whether the hospital was part of Kaiser Permanente (a large integrated health maintenance organization). In addition, OSHPD provided a system name was provided for hospitals being part of a system having three or more hospitals, which we re-coded to yes/no.
Between 2005 and 2011 the public-use data included patient age (individual years, 5-year groupings, and 3 groups (youth/adult/senior), sex, race, and ethnicity. No demographic data was released in public-use files between 2012 and 2015. There have been other analyses despite such missing data, though with a different imputation strategy [23]. A minority of missing/unknown demographic data between 2005 and 2011 could be considered missing at random (MAR). Most of the missing demographic data during that time was missing not at random (MNAR) since patient-level masking was done by OSHPD according to an algorithm based on the number of visits to a specific hospital. Between 2012 and 2014 one might argue MAR since missingness applied equally to everyone. Prior to running multiple imputations, we searched ICD-9 codes to update unknown demographics for those most likely to be female, i.e. pregnancy-related diagnoses (1,796,149 visits), male reproductive-related (463,679 visits), or youth, i.e. newborn/infant-related (93,564 visits).
Following the diagnosis update, 51.23% of cases had valid data in all four demographics, 8.73% had valid data for two or three demographic measures, and 34.55% had no demographic data. In addition, 3.27% had only age group, 2.20% had only sex, 0.02% had only race, and 0.1% had only ethnicity. Missingness for specific variables is shown in Table 1. We used a fully conditional specification multiple implementation methods [24], with a discriminant function for age group (0–17 years, 18–64, 65 plus), sex (male/female), race (white/non-white), and ethnicity (Hispanic/non-Hispanic). In addition to these four demographic measures, the imputation approach included continuous measures for year, patient zip code, and actual number of hospital beds. We generated ten iterations. It would have been preferable to have more iterations, but we faced computational limitations.
Analysis
Cross-tabulations of utilization data were used to create line graphs by year showing a number of visits and percentages based on asthma and mental illness diagnoses. Cross-tabulations and Chi-square testing were conducted to present and compare patient and hospital characteristics, grouped by asthma status (yes/no). Most of this was done using the original data file. Demographic comparisons were done using averages from the 10× multiple imputation file.
The combined influence of need, enabling, predisposing, and contextual characteristics on both asthma diagnosis (yes/no) and discharge status (routine/non-routine) were assessed with multivariable logistic regression using the multiple imputation dataset.
The multiple imputation analysis consisted of running separate multivariable regressions for each iteration (in our case 10 iterations) and then summarizing the parameter estimates. The logistic regression for any asthma diagnosis was based on a ten-iteration imputed file (n = 961,801,760). The multiple imputation analyze function had a relative efficiency of 0.949985 for youth and 0.98032 for Hispanic, with the relative efficiency for all other variables exceeding 0.999. Logistic regression for non-routine disposition among asthma cases was based on a smaller subset of imputed data (n = 44,196,290).
Finally, in order to examine the potential interaction of mental illness and time, we ran logistic regression models on the original data using all variables except for demographics, since the MI analyze procedure could not present combined interaction terms. The mental illness and year interaction terms were graphed using Microsoft Excel.
Data processing and analyses were conducted using SAS 9.4 software (SAS Institute, Gary, North Carolina). Statistical significance was set at p < 0.05. This research was determined to not need human subjects review by the Institutional Review Board review at Loma Linda University (IRB# 5240531).
Results
Figure 1A shows that the number of ED visits increased by 34.6%, from 8,355,151 in 2005 to 11,399,148 in 2014. The percentage of ED visits having any asthma diagnosis increased by 44.4%, from 3.25% of all visits in 2005 to 5.85% in 2014. On the other hand, the percentage of ED visits with asthma as the primary diagnosis decreased by 17.6%, dropping from 1.69% in 2005 to 1.43% in 2014.
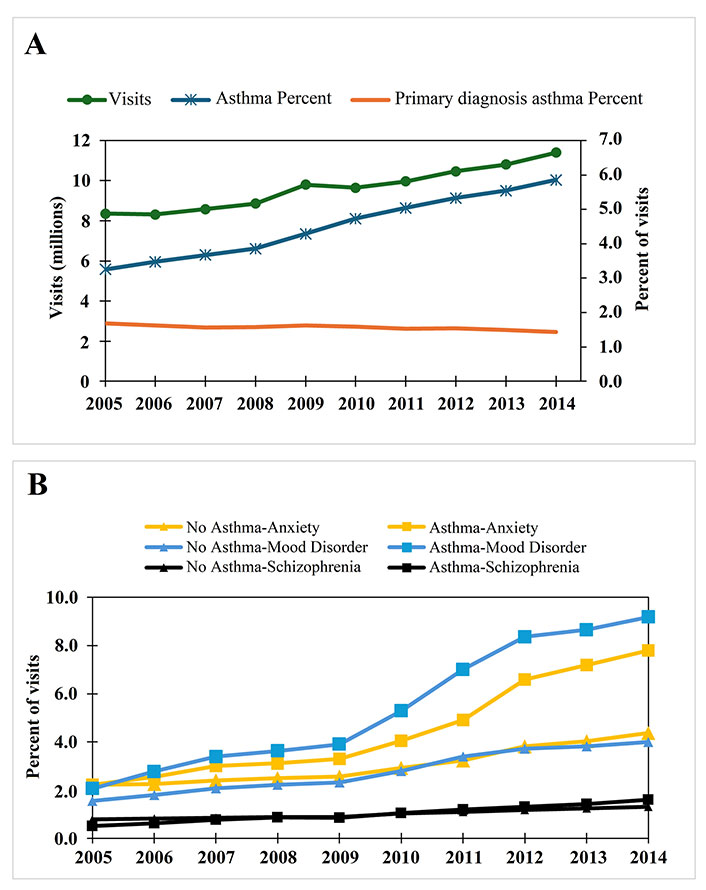
Diagnosis-related trends in California emergency departments (ED). A. California emergency department visits and asthma diagnoses, 2005–2014 (n = 96,180,176); B. percentages of selected mental diagnoses based on asthma status among California ED visits
As shown in Figure 1B, the percentage of visits with a mental illness diagnosis tripled among asthma visits, while at most doubling among non-asthma visits. The biggest increase was for mood disorders, which increased from 2.06% among asthma visits in 2005 to 9.18% in 2014. Next was anxiety disorders, which increased from 2.22% among asthma visits in 2005 to 7.79% of visits in 2014. Finally, schizophrenia increased from 0.50 in 2005 to 1.60% in 2014.
Table 1 and Table 2 present characteristics in hospital and patient characteristics between visits based on the presence of an asthma diagnosis. Due to the large sample size, all comparisons were significant at p < 0.001. Asthma was documented in 4.60% of all ED visits. Among context measures, the relative number of visits with asthma increased each year compared to non-asthma cases and were more common at non-profit hospitals and those in a system of three or more hospitals, particularly Kaiser hospitals. Under pre-disposing measures, both the raw demographics with missing data are shown, as well as the multiple implementation values. The largest demographic difference is that non-whites accounted for a larger percentage of asthma visits (39.44% vs. 36.35%). Mood disorders accounted for 6.16% of asthma-related visits and anxiety disorders for 5.03%. Those with Medicaid as a payer source accounted for 34.87% of asthma visits and 27.95% of non-asthma visits. Most ED visits resulted in patients being sent home, 96.00% of asthma visits and 93.95% of non-asthma visits. One third of ED visits with any asthma diagnosis had asthma as the primary diagnosis.
Frequencies of total emergency department visits and percent with an asthma diagnosis by three-digit zipcode in California, 2005–2014
Patient 3-digit zip code | Frequency | Percent with asthma |
---|---|---|
900 Los Angeles | 6,076,455 | 4.61 |
902 Inglewood vicinity | 2,789,523 | 4.11 |
903 Inglewood | 390,618 | 5.95 |
904 Santa Monica | 198,352 | 3.30 |
905 Torrance | 327,874 | 3.36 |
906 Whittier | 1,768,856 | 4.28 |
907 Long Beach vicinity | 1,603,982 | 3.91 |
908 Long Beach | 1,318,318 | 4.64 |
910 Pasadena vicinity | 504,214 | 3.72 |
911 Pasadena | 322,061 | 4.39 |
912 Glendale | 404,414 | 3.16 |
913 Van Nuys vicinity | 3,049,688 | 3.97 |
914 Van Nuys | 819,786 | 4.16 |
915 Burbank | 220,722 | 3.46 |
916 Hollywood | 505,943 | 3.86 |
917 Alhambra vicinity | 4,097,578 | 3.80 |
918 Alhambra | 141,356 | 3.37 |
919 San Diego South | 1,242,587 | 5.65 |
920 San Diego North | 2,475,059 | 4.29 |
921 San Diego | 2,684,575 | 4.80 |
922 Indio | 2,505,410 | 3.64 |
923 San Bernardino vicinity | 3,404,321 | 5.37 |
924 San Bernardino | 1,027,121 | 4.98 |
925 Riverside | 3,492,626 | 5.19 |
926 Santa Ana vicinity | 2,126,497 | 3.14 |
927 Santa Ana | 1,062,106 | 3.52 |
928 Anaheim | 2,716,389 | 4.17 |
930 Ventura | 1,657,137 | 3.46 |
931 Santa Barbara | 349,038 | 3.07 |
932 Bakersfield vicinity | 2,311,558 | 4.01 |
933 Bakersfield | 1,563,058 | 5.22 |
934 San Luis Obispo | 1,379,139 | 3.27 |
935 Mojave | 1,662,707 | 3.44 |
936 Fresno vicinity | 1,811,091 | 4.88 |
937 Fresno | 1,658,948 | 6.13 |
939 Salinas | 1,138,052 | 3.56 |
940 San Francisco South | 1,636,328 | 4.28 |
941 San Francisco | 1,772,992 | 4.24 |
943 Palo Alto | 189,168 | 4.43 |
944 San Mateo | 318,536 | 3.27 |
945 Oakland vicinity | 5,977,433 | 6.55 |
946 Oakland | 1,455,333 | 6.85 |
947 Berkeley | 260,083 | 5.84 |
948 Richmond | 619,956 | 6.72 |
949 San Rafael | 889,523 | 4.63 |
950 San Jose vicinity | 1,653,386 | 3.89 |
951 San Jose | 2,127,741 | 4.06 |
952 Stockton North | 1,419,857 | 5.51 |
953 Stockton South | 3,554,136 | 4.01 |
954 Santa Rosa | 1,645,513 | 4.83 |
955 Eureka | 647,029 | 5.65 |
956 Sacramento vicinity 1 | 2,765,891 | 5.63 |
957 Sacramento east/vicinity 2 | 557,628 | 5.83 |
958 Sacramento | 2,297,537 | 6.20 |
959 Marysville | 1,587,958 | 4.21 |
960 Redding | 1,223,827 | 4.96 |
961 Reno (NV) | 324,867 | 3.08 |
Outside of California | 1,411,432 | 3.50 |
Homeless/unknown | 1,036,863 | 3.21 |
A contextual measure for geography is presented in Table 2, where data are shown for 57 spatial regions in California based on patient residence. The number of visits ranged from 6.08 million ED visits in the city of Los Angeles (three-digit zip code of 900) down to 141,356 visits in Alhambra (918). The percentage of ED visits with asthma ranged from 3.07% in Santa Barbara (931) to 6.85% in Oakland (946).
Table 3 explores multivariate associations of patient and hospital characteristics with presence of an asthma diagnosis. One can see that the likelihood of asthma started increasing in 2010. Among the ten zip codes with the highest adjusted odds ratio (AORs) compared to Los Angeles: five were in the Bay Area (Richmond, Oakland, Palo Alto, Oakland vicinity, and Sacramento); two were in the agricultural Central Valley (Bakersfield and Fresno), one in the Inland Empire (San Bernardino), and two just over the mountains from Bakersfield: Ventura to the West—along the coast and Mojave to the East in the desert. Asthma was most likely among hospitals having 150 to 199 beds (AOR 1.27 compared to 500+ beds), urban teaching hospitals (AOR 1.21 compared to urban non-teaching), and hospitals in a system (AOR 1.15 compared to non-system hospitals). Hispanics and males were less likely to have asthma, with the strongest reduction being among children (AOR 0.80 compared to adults). Mental illnesses most strongly associated with an asthma diagnosis were attention-deficit/conduct disorders (AOR 1.41), mood disorders (AOR 1.37), and suicide/self-harm (AOR 0.72). The most notable payer source was Medicaid (AOR 1.31 compared to private insurance).
Multivariate odds of a California ED visit having an asthma diagnosis (regression using 10× imputed dataset, i.e. n = 961,801,760)
Variable | AOR | 95% CI | p-value |
---|---|---|---|
Context | |||
Year of visit | |||
2005 (ref.) | |||
2006 | 0.85 | (0.85, 0.86) | < 0.0001 |
2007 | 0.86 | (0.86, 0.86) | < 0.0001 |
2008 | 0.89 | (0.89, 0.89) | < 0.0001 |
2009 | 0.97 | (0.96, 0.97) | < 0.0001 |
2010 | 1.06 | (1.06, 1.06) | < 0.0001 |
2011 | 1.12 | (1.12, 1.12) | < 0.0001 |
2012 | 1.16 | (1.15,1.16) | < 0.0001 |
2013 | 1.19 | (1.19, 1.19) | < 0.0001 |
2014 | 1.22 | (1.21, 1.22) | < 0.0001 |
Patient three-digit zip code | |||
Los Angeles (ref.) | |||
Richmond | 1.22 | (1.20, 1.24) | < 0.0001 |
Bakersfield | 1.21 | (1.19, 1.24) | < 0.0001 |
San Bernardino | 1.20 | (1.19, 1.22) | < 0.0001 |
Oakland | 1.17 | (1.16, 1.19) | < 0.0001 |
Palo Alto | 1.14 | (1.11, 1.17) | < 0.0001 |
Oakland vicinity | 1.11 | (1.09, 1.12) | < 0.0001 |
Ventura | 1.08 | (1.06, 1.11) | < 0.0001 |
Mojave | 1.08 | (1.06, 1.10) | < 0.0001 |
Sacramento | 1.07 | (1.05, 1.08) | < 0.0001 |
Fresno | 1.07 | (1.05, 1.09) | < 0.0001 |
Bakersfield vicinity | 1.06 | (1.04, 1.07) | < 0.0001 |
Berkeley | 1.05 | (1.03, 1.07) | < 0.0001 |
San Francisco | 1.05 | (1.03, 1.06) | < 0.0001 |
San Jose | 1.04 | (1.03, 1.06) | < 0.0001 |
San Bernardino vicinity | 1.04 | (1.03, 1.05) | < 0.0001 |
Stockton North | 1.04 | (1.02, 1.06) | < 0.0001 |
San Diego | 1.04 | (1.03, 1.05) | < 0.0001 |
San Francisco South | 1.03 | (1.02, 1.05) | < 0.0001 |
Long Beach | 1.03 | (1.01, 1.04) | < 0.0001 |
Inglewood | 1.02 | (1.01, 1.04) | 0.0041 |
Eureka | 1.02 | (0.99, 1.06) | 0.195 |
Van Nuys | 1.02 | (1.00, 1.03) | 0.0526 |
San Diego South | 1.02 | (1.00, 1.03) | 0.0529 |
Santa Barbara | 1.01 | (0.98, 1.05) | 0.4907 |
San Mateo | 1.01 | (0.98, 1.03) | 0.5338 |
San Rafael | 1.01 | (0.99, 1.03) | 0.4707 |
Sacramento vicinity 1 | 1.01 | (1.00, 1.02) | 0.2251 |
Van Nuys vicinity | 1.00 | (0.99, 1.01) | 0.7977 |
Sacramento east/vicinity 2 | 1.00 | (0.98, 1.02) | 0.9604 |
Riverside | 1.00 | (0.99, 1.01) | 0.7456 |
Santa Monica | 1.00 | (0.97, 1.02) | 0.8119 |
Pasadena | 0.99 | (0.97, 1.01) | 0.521 |
Alhambra vicinity | 0.99 | (0.98, 1.00) | 0.0147 |
Stockton South | 0.99 | (0.97, 1.00) | 0.0955 |
Hollywood | 0.98 | (0.96, 1.00) | 0.0292 |
San Jose vicinity | 0.98 | (0.97, 1.00) | 0.014 |
Pasadena vicinity | 0.98 | (0.96, 1.00) | 0.0219 |
Indio | 0.97 | (0.96, 0.99) | 0.0014 |
Whittier | 0.97 | (0.96, 0.98) | < 0.0001 |
Burbank | 0.97 | (0.94, 0.99) | 0.0078 |
Long Beach vicinity | 0.96 | (0.95, 0.98) | < 0.0001 |
Redding | 0.96 | (0.94, 0.99) | 0.0118 |
San Diego North | 0.96 | (0.95, 0.98) | < 0.0001 |
Alhambra | 0.96 | (0.93, 0.99) | 0.0062 |
Santa Rosa | 0.95 | (0.93, 0.97) | < 0.0001 |
Marysville | 0.95 | (0.93, 0.97) | < 0.0001 |
Inglewood vicinity | 0.95 | (0.94, 0.96) | < 0.0001 |
Reno (NV) | 0.95 | (0.91, 0.98) | 0.0041 |
Fresno vicinity | 0.95 | (0.93, 0.96) | < 0.0001 |
Salinas | 0.92 | (0.89, 0.95) | < 0.0001 |
San Luis Obispo | 0.92 | (0.89, 0.95) | < 0.0001 |
Outside of California | 0.92 | (0.91, 0.93) | < 0.0001 |
Torrance | 0.90 | (0.88, 0.92) | < 0.0001 |
Anaheim | 0.89 | (0.88, 0.90) | < 0.0001 |
Santa Ana vicinity | 0.86 | (0.85, 0.87) | < 0.0001 |
Glendale | 0.85 | (0.84, 0.87) | < 0.0001 |
Santa Ana | 0.83 | (0.82, 0.84) | < 0.0001 |
Homeless/unknown | 0.79 | (0.78, 0.79) | < 0.0001 |
Hospital control | |||
Government | 1.08 | (1.07, 1.10) | < 0.0001 |
For-Profit | 0.94 | (0.93, 0.94) | < 0.0001 |
Non-Profit (ref.) | |||
Hospital teaching/location | |||
Urban, non-teaching (ref.) | |||
Rural, non-teaching | 0.88 | (0.87, 0.89) | < 0.0001 |
Urban, teaching | 1.21 | (1.20, 1.22) | < 0.0001 |
Hospital licensed bed size | |||
1 to 49 beds | 0.65 | (0.63, 0.66) | < 0.0001 |
50 to 99 beds | 0.98 | (0.97, 0.99) | < 0.0001 |
100 to 149 beds | 1.14 | (1.13, 1.15) | < 0.0001 |
150 to 199 beds | 1.27 | (1.26, 1.28) | < 0.0001 |
200 to 299 beds | 1.11 | (1.10, 1.12) | < 0.0001 |
300 to 499 beds | 0.95 | (0.94, 0.96) | < 0.0001 |
500+ beds (ref.) | |||
Hospital system status | |||
In system of 3+ hospitals | 1.15 | (1.15, 1.16) | < 0.0001 |
Not in a system (ref.) | |||
DSH status | |||
DSH hospital | 1.01 | (1.00, 1.01) | 0.0001 |
Non-DSH hospital (ref.) | |||
Hospital Kaiser Permanente status | |||
Non-Kaiser (ref.) | |||
Kaiser | 1.14 | (1.11, 1.17) | < 0.0001 |
Pre-disposing | |||
Sex | |||
Male | 0.98 | (0.98, 0.99) | < 0.0001 |
Female (ref.) | |||
Age group | |||
0 to 17 years | 0.80 | (0.80, 0.81) | < 0.0001 |
18 to 64 years (ref.) | |||
65 years and greater | 1.00 | (1.00, 1.00) | 0.7542 |
Race | |||
White (ref.) | |||
Non-White | 1.04 | (1.04, 1.05) | < 0.0001 |
Ethnicity | |||
Hispanic | 0.95 | (0.95, 0.95) | < 0.0001 |
Non-Hispanic (ref.) | |||
Predisposing-vulnerable domain (any versus no diagnosis) | |||
Anxiety disorders | 1.14 | (1.14, 1.15) | < 0.0001 |
Attention-deficit, conduct, and disruptive behavior disorders | 1.41 | (1.40, 1.42) | < 0.0001 |
Mood disorders | 1.37 | (1.36, 1.37) | < 0.0001 |
Schizophrenia and other psychotic disorders | 0.96 | (0.96, 0.97) | < 0.0001 |
Suicide and intentional self-inflicted injury | 0.72 | (0.71, 0.72) | < 0.0001 |
Enabling | |||
Payer source | |||
Self-pay | 0.87 | (0.86, 0.87) | < 0.0001 |
Medicare | 0.98 | (0.98, 0.99) | < 0.0001 |
Medicaid | 1.31 | (1.31, 1.31) | < 0.0001 |
Private insurance (ref.) | |||
Other government | 0.76 | (0.75, 0.76) | < 0.0001 |
Other | 1.15 | (1.14, 1.15) | < 0.0001 |
Regression using 10× imputed dataset, i.e. n = 961,801,760. The model also includes dummy variables to represent 349 unique hospitals. AOR: adjusted odds ratio; DSH: Disproportionate Share Hospital; CI: confidence interval
Table 4 explores associations of patient and hospital characteristics with non-routine visit disposition, i.e. not sent home, among ED visits with an asthma diagnosis. There was a mixed pattern over time, with a higher likelihood of non-routine discharge in 2013 and 2014. The ten zip codes with the highest odds ratios were different from Table 3, except for Ventura and Mojave. Though the main city of Bakersfield did not make the top ten, the area outside the city did. The highest rate (AOR 1.80) was for patients who were homeless or had an unknown zip code. Other high zip codes included coastal Santa Barbara (AOR 1.22) and higher-income cities within Los Angeles County (Pasadena, Glendale, Burbank, and Santa Monica), with the tenth being San Francisco. Hospital factors associated with non-routine disposition included hospital bed size of 1 to 49 beds (AOR 1.79 compared to 500 plus), government control (AOR 1.38 compared to non-profits), rural non-teaching (AOR 1.22 compared to urban non-teaching) and being a Kaiser hospital (AOR 0.59). Adults 65 years of age and greater were more likely to have non-routine discharge (AOR 1.23 compared to adults 18–64 years of age as were males (OR 1.06). On the other hand, Hispanics were less likely (OR 0.98). All mental diagnoses were associated with an increased likelihood of nonroutine discharge, particularly suicide/self-harm (AOR 4.74), schizophrenia (AOR 1.97), and mood disorders (AOR 1.35). Those with Medicare were more likely to have a non-routine discharge (AOR 1.15 compared to private), while those with Medicaid were less likely (AOR 0.77). Asthma as a primary diagnosis was associated with a reduced risk of non-routine discharge (AOR 0.87 compared to those with a secondary diagnosis).
Odds ratios for non-routine disposition after asthma-related ED visit (regression using 10× imputed dataset, n = 44,196,290)
Variable | AOR | 95% CI | p-value |
---|---|---|---|
Context | |||
Year of visit | |||
2005 (ref.) | |||
2006 | 1.00 | (0.98, 1.02) | 0.9225 |
2007 | 1.04 | (1.03, 1.06) | < 0.0001 |
2008 | 0.85 | (0.84, 0.87) | < 0.0001 |
2009 | 0.90 | (0.88, 0.91) | < 0.0001 |
2010 | 1.00 | (0.98, 1.01) | 0.539 |
2011 | 1.01 | (1.00, 1.03) | 0.1272 |
2012 | 1.01 | (1.00, 1.03) | 0.096 |
2013 | 1.05 | (1.03, 1.06) | < 0.0001 |
2014 | 1.10 | (1.09, 1.12) | < 0.0001 |
Patient three-digit zip code | |||
Los Angeles (ref.) | |||
Homeless/unknown | 1.80 | (1.73, 1.88) | < 0.0001 |
Bakersfield vicinity | 1.29 | (1.17, 1.41) | < 0.0001 |
Ventura | 1.23 | (1.10, 1.38) | 0.0002 |
Santa Barbara | 1.22 | (0.98, 1.52) | 0.0807 |
Mojave | 1.19 | (1.09, 1.31) | 0.0002 |
Pasadena | 1.16 | (1.05, 1.28) | 0.004 |
Glendale | 1.15 | (1.04, 1.26) | 0.0045 |
Burbank | 1.13 | (0.99, 1.29) | 0.0602 |
Santa Monica | 1.12 | (0.98, 1.29) | 0.0996 |
San Francisco | 1.11 | (1.03, 1.21) | 0.0097 |
Torrance | 1.07 | (0.97, 1.18) | 0.1782 |
San Luis Obispo | 1.07 | (0.89, 1.28) | 0.458 |
Alhambra | 1.06 | (0.92, 1.22) | 0.3961 |
San Bernardino | 1.06 | (0.98, 1.14) | 0.1374 |
San Rafael | 1.06 | (0.94, 1.18) | 0.3311 |
Santa Ana vicinity | 1.05 | (0.98, 1.13) | 0.1456 |
San Mateo | 1.05 | (0.94, 1.17) | 0.4201 |
Sacramento vicinity 1 | 1.05 | (0.98, 1.11) | 0.1515 |
San Bernardino vicinity | 1.04 | (0.98, 1.10) | 0.1969 |
Eureka | 1.03 | (0.84, 1.27) | 0.7503 |
Long Beach vicinity | 1.03 | (0.97, 1.09) | 0.2878 |
Santa Rosa | 1.03 | (0.92, 1.14) | 0.6431 |
Fresno | 1.01 | (0.91, 1.12) | 0.8441 |
Long Beach | 1.01 | (0.94, 1.07) | 0.8557 |
Inglewood vicinity | 1.00 | (0.96, 1.05) | 0.8904 |
Inglewood | 1.00 | (0.92, 1.07) | 0.9305 |
Anaheim | 0.99 | (0.94, 1.05) | 0.7281 |
San Diego North | 0.99 | (0.92, 1.06) | 0.7574 |
Van Nuys vicinity | 0.98 | (0.92, 1.05) | 0.6024 |
Redding | 0.98 | (0.84, 1.16) | 0.8328 |
San Diego South | 0.98 | (0.91, 1.06) | 0.6116 |
Richmond | 0.98 | (0.90, 1.06) | 0.5837 |
Pasadena vicinity | 0.98 | (0.89, 1.07) | 0.5829 |
Indio | 0.98 | (0.89, 1.07) | 0.6124 |
Sacramento | 0.97 | (0.90, 1.03) | 0.3128 |
San Diego | 0.96 | (0.90, 1.04) | 0.3123 |
San Francisco South | 0.96 | (0.89, 1.03) | 0.2601 |
Whittier | 0.96 | (0.91, 1.01) | 0.1053 |
Hollywood | 0.96 | (0.87, 1.05) | 0.353 |
Riverside | 0.95 | (0.90, 1.02) | 0.1512 |
Fresno vicinity | 0.94 | (0.86, 1.03) | 0.2095 |
Santa Ana | 0.93 | (0.86, 1.01) | 0.0793 |
San Jose vicinity | 0.93 | (0.86, 1.01) | 0.1001 |
Reno (NV) | 0.92 | (0.75, 1.12) | 0.3821 |
Alhambra vicinity | 0.92 | (0.87, 0.96) | 0.0005 |
San Jose | 0.91 | (0.84, 0.98) | 0.0182 |
Van Nuys | 0.91 | (0.83, 0.99) | 0.0235 |
Stockton North | 0.89 | (0.82, 0.97) | 0.0103 |
Sacramento east/vicinity 2 | 0.89 | (0.82, 0.97) | 0.01 |
Stockton South | 0.89 | (0.82, 0.96) | 0.0028 |
Bakersfield | 0.88 | (0.79, 0.98) | 0.0214 |
Palo Alto | 0.87 | (0.78, 0.98) | 0.0166 |
Oakland | 0.86 | (0.81, 0.92) | < 0.0001 |
Oakland vicinity | 0.86 | (0.82, 0.91) | < 0.0001 |
Marysville | 0.85 | (0.76, 0.96) | 0.0078 |
Outside of California | 0.84 | (0.80, 0.89) | < 0.0001 |
Salinas | 0.84 | (0.68, 1.03) | 0.1011 |
Berkeley | 0.75 | (0.67, 0.83) | < 0.0001 |
Hospital control | |||
Government | 1.38 | (1.30, 1.47) | < 0.0001 |
For-Profit | 0.86 | (0.82, 0.90) | < 0.0001 |
Non-Profit (ref.) | |||
Hospital teaching/location | |||
Urban, non-teaching (ref.) | |||
Rural, non-teaching | 1.22 | (1.14, 1.32) | < 0.0001 |
Urban, teaching | 0.80 | (0.76, 0.84) | < 0.0001 |
Hospital licensed bed size | |||
0 to 49 beds | 1.79 | (1.52, 2.10) | < 0.0001 |
50 to 99 beds | 0.94 | (0.89, 0.99) | 0.0262 |
100 to 149 beds | 0.75 | (0.72, 0.79) | < 0.0001 |
150 to 199 beds | 0.91 | (0.87, 0.96) | 0.0001 |
200 to 299 beds | 0.86 | (0.82, 0.91) | < 0.0001 |
300 to 499 beds | 1.15 | (1.07, 1.23) | < 0.0001 |
500+ beds (ref.) | |||
Hospital system status | |||
In system of 3+ hospitals | 0.99 | (0.97, 1.01) | 0.2128 |
Not in a system (ref.) | |||
DSH status | |||
DSH hospital | 1.00 | (0.99, 1.02) | 0.7694 |
Non-DSH hospital (ref.) | |||
Hospital Kaiser Permanente status | |||
Non-Kaiser (ref.) | |||
Kaiser | 0.59 | (0.53, 0.65) | < 0.0001 |
Pre-disposing | |||
Sex | |||
Male | 1.06 | (1.05, 1.06) | < 0.0001 |
Female (ref.) | |||
Age group | |||
0 to 17 years | 0.98 | (0.95, 1.00) | 0.0716 |
18 to 64 years (ref.) | |||
65 years and greater | 1.23 | (1.21, 1.26) | < 0.0001 |
Race | |||
Non-White | 1.00 | (0.99, 1.01) | 0.9535 |
White (ref.) | |||
Ethnicity | |||
Hispanic | 0.96 | (0.95, 0.97) | < 0.0001 |
Non-Hispanic (ref.) | |||
Predisposing-vulnerable domain (any versus no diagnosis) | |||
Anxiety disorders | 1.04 | (1.03, 1.05) | < 0.0001 |
Attention-deficit, conduct, and disruptive behavior disorders | 1.16 | (1.13, 1.19) | < 0.0001 |
Mood disorders | 1.35 | (1.34, 1.36) | < 0.0001 |
Schizophrenia and other psychotic disorders | 1.97 | (1.94, 1.99) | < 0.0001 |
Suicide and intentional self-inflicted injury | 4.74 | (4.67, 4.81) | < 0.0001 |
Enabling | |||
Payer source | |||
Self-pay | 0.87 | (0.86, 0.88) | < 0.0001 |
Medicare | 1.15 | (1.13, 1.17) | < 0.0001 |
Medicaid | 0.77 | (0.76, 0.78) | < 0.0001 |
Private insurance (ref.) | |||
Other government | 0.80 | (0.78, 0.83) | < 0.0001 |
Other | 1.68 | (1.64, 1.71) | < 0.0001 |
Need | |||
Asthma | |||
Secondary diagnosis (ref.) | |||
Primary diagnosis | 0.87 | (0.86, 0.87) | < 0.0001 |
Model also includes dummy variables to represent 349 unique hospitals. AOR: adjusted odds ratio; DSH: Disproportionate Share Hospital; CI: confidence interval
Figures 2A and 2B present interaction terms for mental illness and time. Figure 2A shows that as time progressed, the presence of a mental diagnosis generally became more positively associated with the presence of an asthma diagnosis. However, Figure 2B shows that among ED visits having an asthma diagnosis, over time the presence of a mental diagnosis generally became negatively associated with a non-routine discharge.
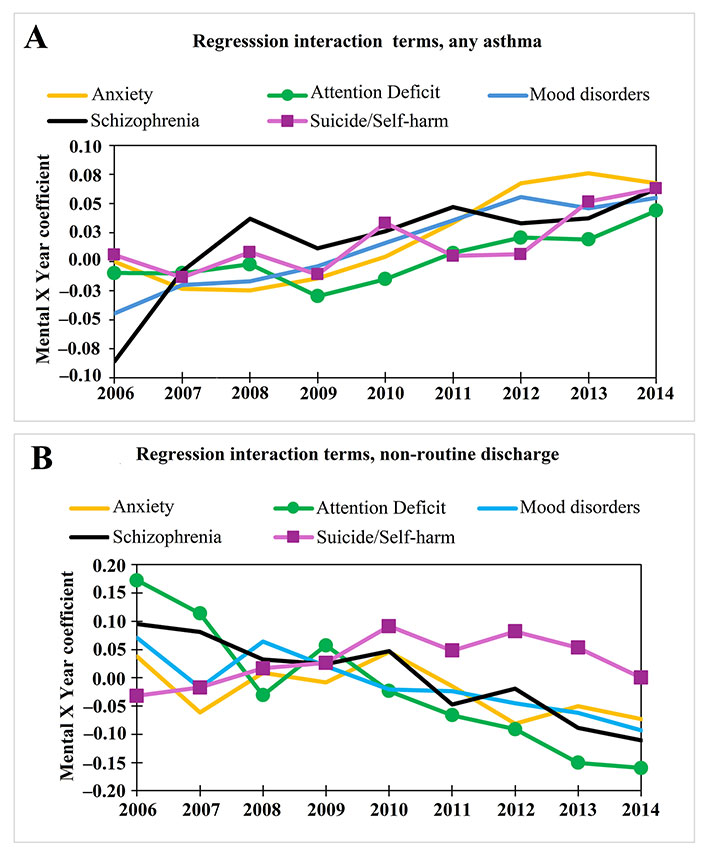
Regression interactions of discharge year with mental diagnosis. A. Interaction coefficients of mental illness and year in a logistic regression model predicting any asthma diagnosis. The interaction model used all terms as the model shown in Table 3, but without imputed demographics (n = 96,180,176); B. Interaction coefficients of mental illness and year in a logistic regression model predicting non-routine discharge status following ED visits having an asthma diagnosis. The interaction model used all terms as the model shown in Table 4, except for imputed demographics (n = 4,419,629)
Discussion
Analysis of public-use data between 2005 and 2014 revealed that California EDs saw an increasing percentage of visits having an asthma diagnosis, even as the percentage of visits with a primary diagnosis of asthma decreased slightly. Furthermore, the percentage of visits with a mood disorder/depression or anxiety diagnosis increased much more dramatically among asthma visits than among non-asthma visits.
We observed a large increase over time in asthma as a secondary diagnosis. This is consistent with national data for 2010 to 2021 which indicates the increasing overall prevalence of asthma among adults and decreasing prevalence among kids; with decreasing rates of asthma-related ED visits and hospitalizations, of course with socio-demographic variations [25]. One possible explanation for more asthma diagnoses is the Affordable Care Act. The emphasis on quality/value payments motivated hospitals to be more thorough in documenting the severity of patients, with some changes in coding and practice occurring before implementation in 2010 [26].
The vast majority of asthma-related ED visits did not result in hospitalization, suggesting potentially inadequate primary care, including follow-up treatment for those who had already visited the ED [27]. Indeed, many cases of uncontrolled asthma are associated with inadequate asthma management training and inhaled corticosteroid ICS use [28]. The reduced likelihood of hospitalization/non-routine discharge for patients having a primary diagnosis of asthma may reflect improved treatments for acute care, such as not requiring intubation [29].
Our Medicaid findings are mostly consistent with a Massachusetts study which found that, compared to children covered by private insurance, those on Medicaid had a higher prevalence of asthma, a higher likelihood of acute care, and also a lower likelihood of routine care [30]. Similarly, others have found that the risk of hospitalization after an asthma-related ED visit was lowest at urban teaching hospitals [15]. However, we found that the likelihood of admission was generally lower among those with Medicaid, not higher [15].
The general rise over time in mental illness diagnoses is consistent with an analysis of California Health Interview Survey (CHIS) data, which found increasing levels of population-based psychological distress between 2011 and 2013 [31]. There may also have been increasing interest in mental health due to the California Mental Health Services Act (Proposition 63) which went into effect in 2005, and interest in both mental health and asthma with the implementation of the Affordable Care Act in 2010. The observation that over time co-occurring mental illness had a slight decreasing impact on non-routine discharge may indicate improved treatment. Additionally, though the CHIS study found modest regional differences in poor mental health; the authors argued it would be more effective to focus on sex (female) and race (African American) [31].
Many factors, such as socio-demographics and mental illness influence the type of care and outcomes among those who have asthma. In our study, risk factors for increased likelihood of asthma during ED visits were female sex and non-white race. Blacks and Hispanics in particular have been shown to have higher rates of ED visits and hospitalization [10, 11]. Reasons include less reliance on routine care for asthma, plus being given less information about asthma management [32]. On the other hand, those less than 18 years of age and Hispanics were less likely to have an asthma diagnosis. Though national studies have shown worse asthma outcomes among Hispanics, others have found that Mexicans specifically are less affected [33]. Furthermore, hospitalization use by Hispanics in California may be reduced due to immigration-related issues [34].
Our findings both of increasing prevalence of mental illness among asthma ED visits and positive association of mental illness with hospitalization are consistent with others who have also found that comorbid mental illness increases the likelihood of ED visits and hospitalization among those with asthma [19, 35, 36]. Our findings are also consistent with a Swiss cohort study which found a positive relationship between asthma and having a major depressive episode [37].
Though we did not formally analyze hospital outcomes, the presence of mental illness may increase the length of stay and total costs of asthma hospitalizations [14]. There are multiple pathways through which mental illness may influence asthma health care and outcomes. For example, those with poor mental health are likely to have worse diets [38] and may be less interested in exercise. Furthermore, medical staff tend to treat the physical illnesses of people with mental illness less thoroughly and less effectively [39].
There are limitations to our study. In using secondary data reported from hospitals, we did not have access to primary care data, socio-demographic measures such as marital status and education, self-reported data such as quality of life and medication adherence, or outcomes following the ending of ED visits. With public-use data, i.e. no patient identifiers or patient ID codes, it was not possible to distinguish unique patients versus visits. Thus, we were not able to identify those individuals having multiple ED visits. Repeat visits are an important issue for asthma management, with socio-demographic differences between those with only one ED visit or multiple visits [40]. Analysis with public use data can illuminate health services patterns but is not as powerful for quality improvement/clinical interventions.
Missing data added uncertainty regarding demographics, though we used standard multiple imputation techniques to obtain reasonable estimates. However, given the multiple imputations, we decided not to stratify the analysis based on age, even though much asthma research is stratified as pediatric or adult. There may also have been variations in diagnostic coding practices across hospitals, particularly for secondary diagnoses. In addition, there was likely a change in coding practices over time as a result of the Affordable Care Act. Results from one state may not generalize to other states or the entire nation. However, we have ten years of data for all ED visits from practically all general acute, non-federal hospitals within one populous state. Results are descriptive for the entire state of California, there was no need to use statistical sampling techniques. Furthermore, the large number of cases provides stability for statistical analysis. These findings are generally consistent with other studies and suggest interesting associations of asthma and mental diagnoses with acute care, which may be generalizable nationally and further studied with confidential data.
Finally, geography was a minor aim. It is well-documented that zip codes developed for mail delivery are not ideal for public health research [41]. Rather, county, Census tracts, or zip code tabulation areas (ZCTAs) would be more ideal. That would allow for more direct consideration of environmental socio-demographics and infrastructure. One can use Geographic Information System techniques with enough five-digit zip codes in attempts to merge zip codes and census data [42]. Even with three-digit zips, if there was also more detailed time information one could estimate environmental exposures such as temperature or wildfire smoke. However, the available zip code information can parse the tens of millions of Californians into roughly 60 population centers. Findings can inform future research.
In conclusion, within California, there has been both a steady increase in the rate of ED visits having an asthma diagnosis and an increase in the percentage of asthma-related ED visits also having a mental diagnosis. Though some of the increase may be due to changes in coding practices, population-based surveys suggest an increased prevalence of both mental illness and asthma. This suggests a need for improved care among those having co-occurring asthma and mental illness, especially patients who are Hispanic or Black.
Abbreviations
AOR: | adjusted odds ratio |
CCS: | Clinical Classification Software |
CHIS: | California Health Interview Survey |
ED: | emergency department |
ICD-9: | International Classification of Diseases, Ninth Edition |
MAR: | missing at random |
OSHPD: | Office of Statewide Health Planning and Development |
Declarations
Author contributions
JEB: Conceptualization, Formal analysis, Writing—original draft. ICE: Writing—review & editing. CG: Writing—review & editing. MW: Writing—review & editing. JMB: Writing—review & editing.
Conflicts of interest
The authors declare that they have no conflicts of interest.
Ethical approval
Ethical approval for this study was obtained by the Loma Linda University Health Institutional Review Board which determined that this was not human subjects research (IRB# 5240531).
Consent to participate
The informed consent to participate in this study was exempted by Loma Linda University Health Institutional Review Board due to analyzing public-use data.
Consent to publication
Not applicable.
Availability of data and materials
Public-use data is no longer available. Confidential emergency department data for California may be obtained at: https://hcai.ca.gov/data-and-reports/research-data-request-information/#patient-discharge-data-pdd
Funding
Not applicable.
Copyright
© The Author(s) 2024.