Abstract
Ischemic heart disease (IHD) is a major global health issue, frequently resulting in myocardial infarction and ischemic cardiomyopathy. Prompt and precise diagnosis is essential to avert complications such as heart failure and sudden cardiac death. Although invasive coronary angiography remains the gold standard for high-risk patients, noninvasive multimodality imaging is becoming more prevalent for those at low-to-intermediate risk. This review evaluated the current state of multimodality imaging in IHD, including echocardiography, nuclear cardiology, cardiac magnetic resonance imaging (MRI), computed tomography (CT) angiography, and invasive coronary angiography. Each modality has distinct strengths and limitations, and their complementary use provides a comprehensive assessment of cardiac health. Integrating artificial intelligence (AI) into imaging workflows holds promise for enhancing diagnostic accuracy and efficiency. AI algorithms can optimize image acquisition, processing, and interpretation of complex imaging data. Emerging technologies like 4D flow MRI, molecular imaging, and hybrid systems [e.g., positron emission tomography (PET)/MRI, PET/CT] integrate anatomical, functional, and molecular data, providing comprehensive insights into cardiac pathology and potentially revolutionizing the management of IHD. This review also explored the clinical applications and impact of multimodality imaging on patient outcomes, emphasizing its role in improving diagnostic precision and guiding therapeutic decisions. Future directions include AI-driven decision support systems and personalized medicine approaches. Addressing regulatory and ethical challenges, such as data privacy and algorithm transparency, is crucial for the broader adoption of these advanced technologies. This review highlighted the transformative potential of AI-enhanced multimodality imaging in improving the diagnosis and management of IHD.
Keywords
Artificial intelligence, ischemic heart disease, multimodality imagingIntroduction
Ischemic heart disease (IHD), also referred to as coronary artery disease (CAD), is a primary global health concern, frequently resulting in myocardial infarction and ischemic cardiomyopathy [1]. It affects approximately 126 million individuals worldwide, with a higher prevalence in men than women, and its occurrence increases with age. Despite a decline in age-standardized rates in many regions, IHD remains the primary cause of death, disability, and suffering worldwide [2]. Precise diagnosis is crucial for effective treatment and prevention of complications associated with IHD. Early and accurate diagnosis is essential to prevent morbidity and mortality, requiring identification of underlying causes, such as CAD, and assessment of heart muscle damage [3]. Timely management is also critical to prevent further damage and reduce the risk of complications like heart failure, arrhythmias, and sudden cardiac death [4].
Over the past few decades, significant developments have been made in managing CAD angiography by invasive methods, which remains the preferred method of diagnosing and treating patients at high risk for CAD [5]. However, for patients at low to moderate risk, this approach is often not initially recommended due to its time-consuming nature and potential procedural risks such as bleeding and contrast-induced nephrotoxicity [6]. Multimodality imaging is a comprehensive approach for these patients, utilizing multiple imaging techniques to provide a more precise and detailed assessment of IHD. The aim is to enhance the early detection of significant asymptomatic CAD and simplify the diagnostic process by reducing the necessity for invasive coronary angiography [7].
The increasing availability of large datasets and powerful computers makes integrating artificial intelligence (AI) into the current multimodality imaging workflow for CAD diagnosis promising [8]. AI can help radiologists and cardiologists meet the growing demand for cardiovascular imaging examinations, potentially improving diagnostic accuracy and efficiency. This review examined the current applications of AI in multimodality imaging for CAD management and highlighted its substantial potential to revolutionize CAD diagnosis and treatment.
Current state of multimodality imaging in IHD
Currently, a multimodality approach in IHD is appropriate for correctly assessing and managing these patients, considering that each technique can have an important role and limitations (Figure 1).
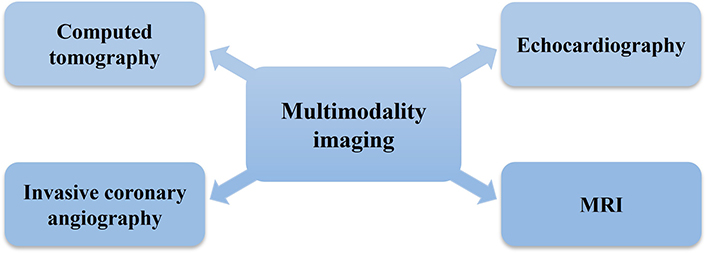
Current state of multimodality imaging in IHD. MRI: magnetic resonance imaging; IHD: ischemic heart disease
Echocardiography
This imaging technique, known as echocardiography, uses sound waves to create detailed images of the heart’s structure and function. It is widely accessible, cost-effective, and provides real-time information about cardiac anatomy, function, and hemodynamics [9]. However, it has limitations as it is unable to effectively visualize the coronary arteries. Additionally, patients with conditions like obesity or chronic obstructive pulmonary disease (COPD) may have poor acoustic windows, leading to lower image quality. This makes echocardiography less useful for detecting coronary artery stenosis in these cases [10]. Recent advances in three-dimensional echocardiography are enhancing the evaluation of complex cardiac structures and function, particularly in the context of valve diseases and IHD.
Nuclear cardiology
Techniques like single-photon emission computed tomography (SPECT) and positron emission tomography (PET) can evaluate myocardial perfusion and viability. They are effective in detecting myocardial ischemia and infarction but are limited by their lower spatial resolution and radiation exposure [11]. Recent developments include new radiotracers aimed at improving the specificity and sensitivity of myocardial perfusion imaging (MPI).
Cardiac magnetic resonance imaging
This imaging technique provides excellent soft tissue contrast and detailed information about the structure, function, and characteristics of the heart. It’s useful for assessing heart muscle viability, the size of a heart attack, and scar tissue [12]. However, its availability and cost can be limiting factors. Research is being conducted to assess how effective stress perfusion magnetic resonance imaging (MRI) is at detecting reduced blood flow to the heart, offering a radiation-free alternative to nuclear imaging.
Computed tomography angiography
Computed tomography angiography (CTA) provides high-resolution images of the coronary arteries, allowing for the detection of stenosis and plaque burden. It is less invasive than conventional angiography but is associated with radiation exposure and contrast-related risk [13]. However, there are specific limitations associated with it. Similar to nuclear imaging, CTA exposes patients to ionizing radiation, which can be a concern, especially with repeated use. Computed tomography (CT) images can be affected by artifacts caused by patient movement, calcium deposits, or metal implants, potentially complicating the interpretation [14]. Studies are underway to validate the noninvasive assessment of CAD using comput-ational models to derive fractional flow reserve (FFR) from standard CT angiograms.
Invasive coronary angiography
Although not an imaging modality in the traditional sense, invasive coronary angiography remains the gold standard for evaluating coronary anatomy and identifying obstructive lesions [15]. It allows simultaneous intervention if necessary but carries risks associated with invasive procedures. The procedure is invasive, requiring arterial access, which carries risks such as bleeding, infection, and vascular complications [16]. The synergy between these imaging techniques is essential for a thorough assessment of IHD. For instance, stress echocardiography can be used in combination with nuclear imaging to improve diagnostic precision. Additionally, cardiac MRI can offer supplementary insights into myocardial function and viability when a nuclear imaging study yields inconclusive results. Current research is integrating optical coherence tomography (OCT) and intravascular ultrasound (IVUS) with invasive angiography to deliver compre-hensive imaging of vessel walls and plaque morphology.
Artificial method
It is not easy to pinpoint the exact year AI was born. An English mathematician, Alan Turing was sometimes called the “father of AI”. Turing was employed by the British government and was credited with developing. The Bombe, a famous code-cracking tool that helped the German army decipher enigma during World War II [17]. One may argue that using tools is an “extension” of the body’s natural function. Similarly, one could consider computers “extensions” of the human brain.
AI may have surpassed or is close to surpassing human performance in several areas, according to the quick development of computer power, the collection of enormous volumes of data, and developments in the theory of computation [18]. Intelligence has always been the most important resource for humanity to survive on earth. There are many reasons to think that the continued advancements in AI technology will bring a new era and possibly transform people’s productivity. AI is a term with many different meanings from different perspectives. Among the widely acknowledged goals are the following: AI is a branch of computer science that enables machines to do tasks that humans typically perform. An alternative viewpoint suggests that AI is a system that can monitor its surroundings and make decisions to increase its chances of success in a particular task [19]. AI is set to be incorporated into several medical domains, such as drug discovery, clinical care, risk prediction, treatment plans, and medical diagnosis [20].
Additionally, the application of AI in medicine is rapidly progressing. Machine learning (ML), cognitive computing, and big data analytics are the three primary subcategories of AI technologies. There are two main ML approaches, each with many variants. In the first approach, known as “supervised ML”, a dataset with each case labeled with a class is provided to the model. The computer model then searches for commonalities. Between each case belonging to the same class, it “learns” to identify any new unlabeled case belonging to that class [21]. The second approach to “unsupervised ML” involves feeding all the data to the computer model without telling which cases go in which class. It is then left to make its connections, which are used to categorize the subjects into groups according to the bonds they developed during the learning process. Unsupervised ML has the potential to be incredibly helpful in gaining new insights into complex systems with many variables; it can make clustering decisions and feature associations on its own [22] (Figure 2).
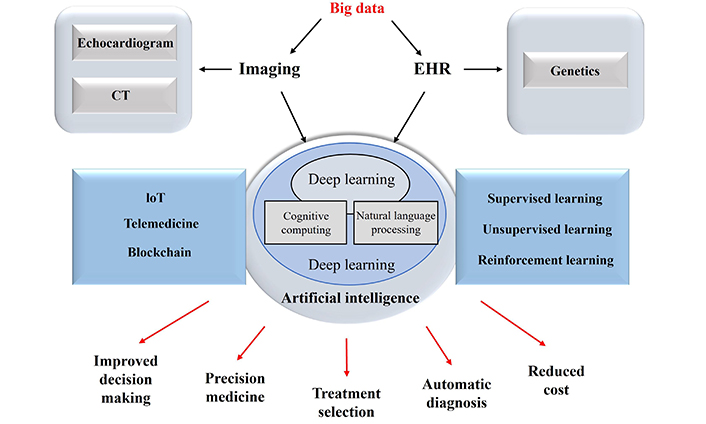
Overview of AI technology. EHR: electronic health record; CT: computed tomography; IoT: internet of things; AI: artificial intelligence
Application of AI in medicine
Recent studies have shown that AI is used in medicine to manage digital medical records and conduct cutting-edge, technologically enabled patient examinations. It provides answers, especially in the form of customized treatment plans, carefully blended drugs, and focused therapies [23]. AI is a state-of-the-art tool that helps surgeons diagnose, treat, and operate. The main application of this technology is to enhance complex case decision-making [24]. It can also help with hospital infection tracking, detection, inves-tigation, and management. Online systems for scheduling patient appointments are created and improved by this system. In all medical domains, it will help humanity in the future. Numerous IT companies and research institutions are advancing AI technology in various healthcare domains, including clinical medicine, public health, health systems management, and medical research. Notable strides have been made in developing AI technologies in clinical medicine, including computer vision and image analysis. The classification and detection of medical images have significantly improved due to integrating these technologies with AI, which is especially advantageous for medical imaging applications. This development is especially noticeable in cardiology, where these technologies provide better imaging diagnostics [25]. In the future, clinicians will receive assistance in making accurate decisions and predicting patient outcomes from cognitive computers trained in ML.
Role of AI in multimodality imaging
AI includes various technologies enabling machines to perform tasks requiring human-like intelligence. In medical imaging, ML, a subset of AI, plays a crucial role. ML involves training algorithms on large datasets to recognize patterns and make predictions. Deep learning is a more advanced form of ML that uses neural networks with multiple layers to analyze complex data, such as medical images. These algorithms can be trained to detect subtle abnormalities, differentiate between tissue types, and identify disease markers with high accuracy [26]. AI’s application in multimodality imaging for IHD includes enhancing image acquisition, processing, and analysis. In image acquisition, AI algorithms can optimize scanning parameters in real-time, reducing noise and artifacts while improving resolution. This leads to more explicit images, enabling more accurate assessments of cardiac structures and functions [27]. AI algorithms can reconstruct images from raw data more effectively than traditional methods in image processing. For instance, in CT and MRI, AI can produce high-quality images from lower doses of radiation or shorter scanning times, minimizing patient exposure and discomfort. AI-enhanced image reconstruction techniques can also highlight specific features, such as coronary artery plaques, with greater detail [28].
For interpretation, AI systems assist radiologists by automating the detection and quantification of IHD indicators, such as coronary artery calcification, myocardial perfusion defects, and wall motion abnormalities. These systems can quickly analyze vast amounts of imaging data, providing preliminary findings and highlighting areas of interest for further review by radiologists. AI can significantly speed up the diagnostic process and reduce the chances of human error, particularly in imaging for IHD. AI has the potential to improve diagnostic accuracy in IHD imaging dramatically. ML algorithms can be trained on annotated datasets, including images of healthy and diseased hearts. Through this training, the algorithms learn to differentiate between normal and pathological features with high sensitivity and specificity [29]. Studies have shown that AI can match or surpass human experts in identifying certain cardiac conditions [30]. Moreover, AI can integrate data from multiple imaging modalities, such as echocardiography, CT, MRI, and PET, to comprehensively assess the heart. By combining structural, functional, and metabolic information, AI systems offer a more holistic view of cardiac health, leading to more accurate diagnoses and tailored treatment plans. Efficiency is another key benefit of AI in medical imaging. Automated analysis reduces the time radiologists spend on routine tasks, allowing them to focus on complex cases and clinical decision-making [31] (Figure 3).
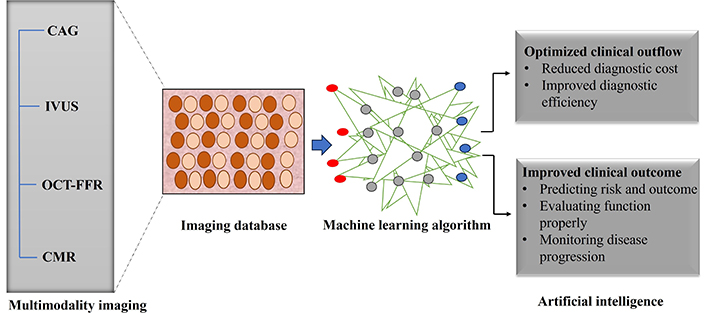
Role of AI in multimodality imaging. CAG: computer-assisted geometry; IVUS: intravascular ultrasound; OCT-FFR: optical coherence tomography-fractional flow reserve; CMR: cardiac magnetic resonance
Emerging technologies in multimodality imaging for IHD
Multimodality imaging is witnessing rapid advancements, with novel imaging techniques and technologies pushing the boundaries of traditional approaches. These innovations are up-and-coming in assessing and managing IHD, offering more precise and comprehensive evaluations of cardiac health.
Overview of novel imaging techniques
4D flow MRI
One of the cutting-edge advancements in cardiac imaging is 4D flow MRI, which extends traditional three-dimensional imaging by incorporating the dimension of time. This technique enables the visualization and quantification of blood flow dynamics throughout the cardiac cycle. In the context of IHD, 4D flow MRI provides detailed insights into abnormal blood flow patterns, such as turbulent flow in stenotic coronary arteries, and helps assess these abnormalities’ impact on myocardial perfusion and function. This comprehensive flow assessment can improve the detection of hemodynamically significant lesions and guide therapeutic decisions [32].
Molecular imaging
Molecular imaging is another transformative technology beyond anatomical visualization that provides functional and molecular information about the heart. Techniques such as PET and SPECT can detect metabolic changes, receptor expression, and cellular activity in myocardial tissue [33]. For IHD, molecular imaging can identify areas of myocardial ischemia and viability, assess inflammation, and detect early signs of atherosclerosis. These capabilities are crucial for personalized medicine, allowing for tailored treatment strategies based on the underlying molecular processes [34].
Integration of multiple imaging modalities
The integration of multiple imaging modalities offers a comprehensive assessment of IHD by combining the strengths of each technique. For instance, hybrid imaging systems that combine PET and CT (PET/CT) or PET and MRI (PET/MRI) provide both anatomical and functional information in a single scan [35]. This integration allows for the precise localization of metabolic abnormalities within the anatomical context of the coronary arteries and myocardium. Another example is the use of echocardiography combined with cardiac MRI. Echocardiography offers real-time functional assessment of cardiac motion and valve function, while MRI provides detailed anatomical and tissue characterization [36]. Together, these modalities can offer a more complete picture of cardiac health, particularly in complex cases where a single modality may not provide sufficient information.
Innovative technologies in IHD imaging
AI-enhanced imaging
AI, including ML algorithms, is increasingly integrated into imaging systems to enhance their capabilities. AI can assist in image reconstruction, noise reduction, and automated analysis, improving the efficiency and accuracy of IHD analysis [37]. For example, AI algorithms can automatically quantify coronary artery calcification, detect myocardial perfusion defects, and analyze complex flow patterns in 4D flow MRI.
High-resolution CT imaging
Advances in CT technology, such as dual-energy and photon-counting CT, are pushing the limits of spatial resolution and contrast differentiation. These technologies allow for more accurate characterization of coronary plaques, identification of high-risk plaque features, and assessment of myocardial perfusion [38]. High-resolution CT imaging is particularly valuable for noninvasive coronary angiography, offering a safer alternative to invasive procedures.
Nanotechnology-based imaging
Nanotechnology is opening new frontiers in molecular imaging. Nanoparticles can target specific molecular markers of IHD, such as atherosclerotic plaques or inflammatory cells [39]. These nanoparticles can be labeled with imaging agents detectable by MRI, CT, or PET, providing high-resolution images of molecular processes involved in IHD.
Clinical applications and impact of multimodality imaging in IHD
Multimodality imaging, which combines various imaging techniques, transforms the diagnosis, prognosis, and treatment planning for IHD. This approach leverages the strengths of different modalities to provide a comprehensive understanding of cardiac health. The clinical utility of multimodality imaging is increasingly supported by case studies and clinical trials, demonstrating its significant impact on patient outcomes and healthcare costs. A study published in “The Lancet” demonstrated that integrating coronary CTA with MPI improved the accuracy of IHD diagnosis. Patients with inconclusive stress tests underwent CTA followed by MPI, which allowed for precise identification of ischemic regions and led to appropriate interventions. The combined use of these modalities reduced the need for invasive coronary angiography by 25% [40]. The SPINS [Stress CMR (cardiac magnetic resonance) Perfusion Imaging in the United States] study emphasized the importance of combining stress CMR imaging with late gadolinium enhancement (LGE) for predictive purposes. The study evaluated patients with suspected IHD using stress CMR to assess perfusion defects and LGE to identify myocardial scarring. This comprehensive approach effectively stratified the risk, identifying patients at higher risk for adverse cardiac events. Over a 5-year follow-up period, patients with both perfusion defects and myocardial scarring experienced a significantly higher incidence of major adverse cardiac events (MACE) [41]. In the International Study of Comparative Health Effectiveness with Medical and Invasive Approaches (ISCHEMIA) trial, multimodality imaging, including CTA and functional testing, was instrumental in guiding management strategies for patients with stable IHD. The trial demonstrated that patients with moderate to severe ischemia identified through multimodality imaging experienced more significant benefits from an invasive strategy (revascularization) than medical therapy alone [42].
Impact on patient outcomes
Multimodality imaging enhances diagnostic accuracy and facilitates better-tailored treatment strategies for IHD. By combining anatomical and functional data, clinicians can make more informed decisions, resulting in improved management of IHD. For instance, identifying the exact location and extent of ischemia and myocardial viability helps select appropriate revascularization procedures, reduce the risk of recurrent events, and improve survival rates [43]. Although multimodality imaging may incur higher initial costs, it can result in substantial long-term savings by reducing unnecessary procedures and hospitalizations. Accurate diagnosis and appropriate treatment reduce the incidence of complications and repeated interventions. For example, multimodality imaging can help avoid unnecessary invasive coronary angiography and interventions in patients with non-obstructive IHD.
Challenges in understanding AI outputs in biological contexts
The application of AI in biological and medical contexts, particularly in multimodality imaging for IHD, presents unique challenges.
Human vs. AI understanding
In well-defined domains like chess, AI has demonstrated exceptional capabilities. The game of international chess, originating in India, involves fixed rules and clear objectives, making it an ideal candidate for AI application. Former world champion chess players have acknowledged AI’s prowess in the game, where human and AI understanding can align closely due to the structured nature of the domain. Contrastingly, biological systems are inherently complex and less predictable. AI algorithms in medical imaging often produce outputs that are difficult for human intelligence to interpret. Unlike chess, where the moves and strategies are transparent, AI’s “black box” nature in biology means that the reasoning behind AI decisions is not always clear [44]. This lack of transparency poses a significant challenge in clinical settings, where understanding the rationale behind a diagnosis or prediction is crucial for patient care.
Handling artifacts
One significant issue in applying AI to biological contexts is the incorporation of artifacts into analyses. In medical imaging, artifacts can arise from various sources, including equipment limitations, patient movement, and environmental factors. AI algorithms may not always distinguish between true signals and artifacts, leading to potential misinterpretations [45]. For example, an AI system might incorrectly identify an artifact as a pathological feature, resulting in false-positive results.
Nonlinear functions and predictive value
AI models often use complex, nonlinear functions to make predictions. While these models can achieve high accuracy metrics such as the area under the curve (AUC), their practical utility can be limited. A pertinent example is the use of a traditional 12-lead electrocardiogram (ECG) to predict left ventricular ejection fraction < 35%, as demonstrated by Attia et al. [46]. The AI model achieved a high AUC of 0.93. Yet, the positive predictive value was only 32%. This discrepancy highlights the challenge of relying solely on AI outputs without understanding the underlying decision-making process.
Validation issues and overfitting
AI applications in medicine must undergo rigorous validation to ensure reliability. Overfitting, where an AI model performs well on training data but poorly on new, unseen data, is a common problem. The fat attenuation index (FAI) developed using AI in CT imaging of coronary arteries is an example of a promising tool still under validation [47]. Ensuring that AI models generalize well across diverse patient populations and imaging conditions is crucial for their clinical adoption.
Recent developments and future directions in multimodality imaging for IHD
In innovative health care, Cardiovascular Business has shed light on the applications of AI in medicine. Cardiovascular applications rank as the second most common, with 122 FDA-cleared AI tools [48]. These tools range from diagnostic aids to predictive analytics, demonstrating the potential of AI to revolutionize cardiovascular care. However, despite these advancements, challenges such as overfitting, data quality issues, and the “black box” nature of AI remain. Addressing these limitations is crucial as AI and ML continue to revolutionize multimodality imaging for IHD. Advanced algorithms will enhance image acquisition, reconstruction, and interpretation, leading to more accurate and efficient diagnoses. AI-driven decision support systems will assist clinicians in developing personalized treatment plans based on comprehensive imaging data. The future of IHD management lies in personalized medicine, where treatment strategies are tailored to the individual patient’s genetic, molecular, and imaging profiles. Integrating multimodality imaging with genomic and proteomic data will provide a more holistic view of the disease, enabling precise and effective interventions [49]. The development of hybrid imaging systems, such as PET/MRI and PET/CT, will continue to advance. These systems combine anatomical, functional, and molecular information, offering a comprehensive assessment of IHD [50]. Future innovations may include multi-parametric imaging, where multiple imaging parameters are analyzed simultaneously to provide deeper insights into cardiac health. Advances in wearable technology and remote monitoring devices will enable continuous assessment of cardiac function and early detection of ischemic events. Integrating these devices with AI algorithms can provide real-time alerts and actionable insights, improving patient management and outcomes. The future of multimodality imaging for IHD will benefit from interdisciplinary collaboration among cardiologists, radiologists, data scientists, and regulatory experts [51]. Such partnerships will foster the development of innovative imaging techniques and ensure their safe and effective implementation in clinical practice.
Conclusions
Multimodality imaging represents a significant advancement in the diagnosis and management of IHD. By leveraging the strengths of various imaging techniques, healthcare providers can achieve a comprehensive assessment of cardiac health, aiding in the early detection and treatment of IHD. The integration of AI into these imaging modalities further enhances diagnostic accuracy and efficiency, paving the way for personalized medicine approaches. Emerging technologies, such as 4D flow MRI, molecular imaging, and hybrid imaging systems, hold promise for providing detailed anatomical, functional, and molecular insights into cardiac pathology. While the potential of these technologies is vast, addressing regulatory and ethical challenges, including data privacy and algorithm transparency, is crucial for their broader adoption. The transformative potential of AI-enhanced multimodality imaging is poised to revolutionize IHD management, ultimately improving patient outcomes and reducing healthcare costs.
Abbreviations
AI: | artificial intelligence |
AUC: | area under the curve |
CAD: | coronary artery disease |
CMR: | cardiac magnetic resonance |
CT: | computed tomography |
CTA: | computed tomography angiography |
IHD: | ischemic heart disease |
LGE: | late gadolinium enhancement |
ML: | machine learning |
MPI: | myocardial perfusion imaging |
MRI: | magnetic resonance imaging |
PET: | positron emission tomography |
SPECT: | single-photon emission computed tomography |
Declarations
Author contributions
PKCS: Conceptualization, Data curation, Resources, Software, Writing—original draft, Writing—review & editing. RV: Investigation, Supervision, Validation.
Conflicts of interest
Both authors declare that they have no conflicts of interest.
Ethical approval
Not applicable.
Consent to participate
Not applicable.
Consent to publication
Not applicable.
Availability of data and materials
Not applicable.
Funding
Not applicable.
Copyright
© The Author(s) 2024.