Abstract
Aim:
AI research, development, and implementation are expanding at an exponential pace across healthcare. This paradigm shift in healthcare research has led to increased demands for clinical outcomes, all at the expense of a significant gap in AI literacy within the healthcare field. This has further translated to a lack of tools in creating a framework for literature in the AI in medicine domain. We propose HUMANE (Harmonious Understanding of Machine Learning Analytics Network), a checklist for establishing an international consensus for authors and reviewers involved in research focused on artificial intelligence (AI) or machine learning (ML) in medicine.
Methods:
This study was conducted using the Delphi method by devising a survey using the Google Forms platform. The survey was developed as a checklist containing 8 sections and 56 questions with a 5-point Likert scale.
Results:
A total of 33 survey respondents were part of the initial Delphi process with the majority (45%) in the 36–45 years age group. The respondents were located across the USA (61%), UK (24%), and Australia (9%) as the top 3 countries, with a pre-dominant healthcare background (42%) as early-career professionals (3–10 years’ experience) (42%). Feedback showed an overall agreeable consensus (mean ranges 4.1–4.8, out of 5) as cumulative scores throughout all sections. The majority of the consensus was agreeable with the Discussion (Other) section of the checklist (median 4.8 (interquartile range (IQR) 4.8-4.8)), whereas the least agreed section was the Ground Truth (Expert(s) review) section (median 4.1 (IQR 3.9–4.2)) and the Methods (Outcomes) section (median 4.1 (IQR 4.1–4.1)) of the checklist. The final checklist after consensus and revision included a total of 8 sections and 50 questions.
Conclusions:
The HUMANE international consensus has reflected on further research on the potential of this checklist as an established consensus in improving the reliability and quality of research in this field.
Keywords
Artificial intelligence, checklist, consensus, machine learning, medicineIntroduction
Artificial intelligence (AI) is a heavily expanding facet of the healthcare landscape. This mutual overlap between medicine and computer science has led to a new discipline, the so-called “artificial intelligence in medicine”, or AIM in short [1]. The rise in interdisciplinary collaborations and investment in health technologies has led to preliminary studies exploring different aspects of AI in healthcare [2, 3]. This is seen in fields such as ophthalmology where an AI diagnosis system can recommend treatments for more than 50 eye diseases with 94% accuracy [4]. AI has been proven to be effective in analyzing radiological data for improved accuracy and diagnosis using medical imaging [5]. According to Accenture [6], hospitals will invest up to $6.6 billion annually in AI-enabled technologies by 2021. Safavi and Kalis [7] predict that AI innovations could bring up to $150 billion in annual savings for U.S. healthcare by 2026.
The early impact of AI during the Coronavirus Disease 2019 (COVID-19) pandemic has been observed in 1) early warning system and predictive modeling, 2) contact tracing, 3) diagnostics, 4) drug discovery and development, and 5) social control [8]. This paradigm shift in research has inadvertently prioritized the demands for fast-paced clinical outcomes at the expense of a significant gap in knowledge in the field of AI [7]. Furthermore, there is a lack of data-based collaboration and real-world application, which has led to the creation of AI models that are unfit for clinical use in the detection and treatment of COVID-19 [9].
Studies that involve machine learning (ML) are applied to various disciplines of medicine with a lack of standardization in the AI domain [10]. Due to the selective nature of assessing outcomes in all clinical specialties, there is no relevant research model as a reference for validation of this literature. This challenge in AI in medicine research has led to the creation of guidelines, checklists, and consensus focused on different specialties of medicine [11–13]. A solution of this nature would help set the framework for future studies and help guide the foreseeable quality of AI-related innovation. It can further tackle the challenge of ethics regarding the explainability and transparency of AI as a so-called “black box” in research [14]. Due to the lack of validity of these existing guidelines, the majority of them have not been reproducible in contemporary research. Additionally, there is existing literature for prediction model development and validation, such as the TRIPOD (Transparent Reporting of a multivariable prediction model for Individual Prognosis Or Diagnosis) checklist [15, 16], but an AI or ML-specific robust checklist is missing.
We propose a HUMANE (Harmonious Understanding of Machine Learning Analytics Network) Delphi-validated checklist for establishing an international consensus for authors and reviewers involved in research focused on AI or ML in Medicine. The goal of this project was to develop a Delphi-validated checklist for establishing an international consensus for authors and reviewers involved in research focused on AI or ML in medicine. This checklist will further pave the way to investigate the validity of the research framework in the form of a large-scale systematic review for future studies exploring the intersection of AI in healthcare.
Materials and methods
Creation of the HUMANE group
Through word of mouth and referrals from other experts, we reached out to individuals involved in AI medicine research. We utilized purposeful sampling of subject matter experts (SMEs) in the healthcare and computer science fields. We sent out an email explaining the aim of the project and the current design. We recruited eight AI experts through this process. These individuals were part of a two-round consensus process.
Creation of the checklist
We performed a PubMed search of AI manuscripts and current guidelines between January 2015 to February 2020 (Figure 1). Each member of the HUMANE group proposed a set of AI papers to consider for the development of the guidelines. Additionally, each expert was invited to include items that they thought were pertinent to the checklist. A bullet point list of recommendations was created and organized into a Google Forms survey. The checklist was given to AI experts to score each checklist item. After finalization of the checklist using our two-round process, this data was transferred to REDCap for an easy user interface (Table 1).
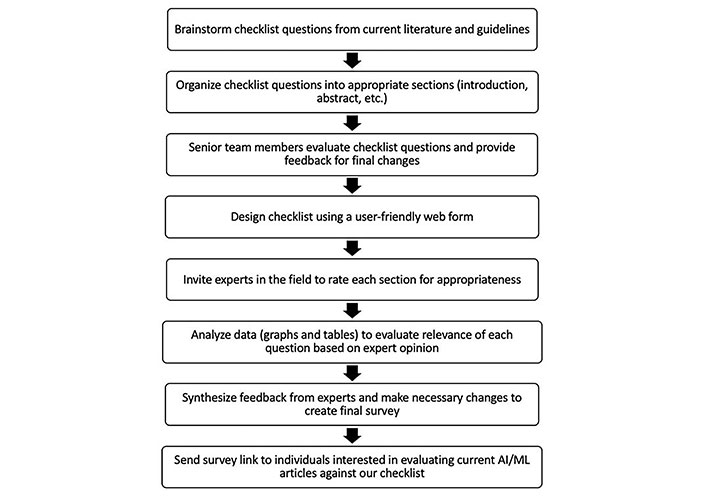
Flowchart of the process involved in deriving checklist questions. AI: artificial intelligence; ML: machine learning
Major changes in the HUMANE checklist
Section | Google Forms v1.08 sections, 56 questions | REDCap v2.08 sections, 50 questions | |||
---|---|---|---|---|---|
No. of questions | Content | Answer format | No. of questions | Changes made | |
Title | 2 | The basic structure of the title | 5-point Likert scale (strongly disagree to strongly agree) | 2 | The answer format changed from a numerical scale to Yes/No |
Abstract | 1 | Essential components of abstract | 5-point Likert scale (strongly disagree to strongly agree) | 1 | The answer format changed from a numerical scale to Agree/Partially agree/Disagree |
Introduction | 5 | Various aspects of background and introduction with a focus on rationale, objectives, knowledge gap, and potential impact | - | 6 | The first question expanded to elaborate on the rationale of the introduction with Yes/No answers |
Methods 4a | 8 | Various aspects of data: data source, study design, timeline, data pre-curation steps and data categorization | 5-point Likert scale (strongly disagree to strongly agree) | 8 | The answer format of the question on the difference between training and validation datasets changed to Temporally/Geographically/Both/None. Answer format of other questions changed to Yes/No/Unclear/NA as appropriate |
Methods 4b | 2 | Informed consent and inclusion-exclusion criteria as a part of the methods section | 5-point Likert scale (strongly disagree to strongly agree) | 2 | Answer format changed to Yes/No/NA |
Methods 4c | 5 | AI model outcomes | 5-point Likert scale (strongly disagree to strongly agree) | 5 | The answer format changed to Yes/No/NA. Question on models using triage or diagnostic pathways adjusted to include options for “intended role” and “diagnostic elements”, respectively. Redundant questions on the knowledge gap and overfitting of AI models were removed |
Methods 4d | 3 | Statistical analysis methods | 5-point Likert scale (strongly disagree to strongly agree) | 4 | The answer format changed to Yes/No/NA. Question on overfitting protocol rephrased |
Section 5a (Ground Truth) | 5 | Ground truths applied in AI model development | 5-point Likert scale (strongly disagree to strongly agree) | 6 | The answer format changed to Yes/No/NA. Answer options for “prospective” and “retrospective” were added where appropriate. Branching logic of Yes/No question on ground truth supervised learning model was added |
Section 5b (Expert(s) Review) | 5 | Experts’ role in reviewing ground truth labels | Numerical scale | 1 | Answer options changed to reflect the nature of expert roles with Unclear/NA options added where appropriate. One representative question is kept in the final checklist |
Results | 14 | Comprehensive cover of reporting of results | - | 9 | Added free text boxes to elaborate if selected “other” option for calibration and performance metrics. Some questions changed to allow more than one response. Question addressing algorithmic bias added. Removed 6 questions due to not being relevant to the results section, including comparison with past literature, whether the validation dataset was distinct, evaluating model fairness, providing differential diagnoses and confidence estimates, reporting values of the measured variable, and reiterating the purpose of AI technology (removed mode of fairness, diagnostic cues, diagnostic distinct, differential diagnosis, values of the measured variable) |
Discussion | 5 | Study summary, strengths and weaknesses of study, conclusion | 5-point Likert scale (strongly disagree to strongly agree) | 5 | Rephrased for conciseness. Answer format changed to Yes/No/NA |
Other and conflict of interest | 1 | Free text boxes for feedback | - | 1 | Only one free text box retained |
Collaborative author details | - | Details of survey respondents | - | - | - |
-: No data. AI: artificial intelligence
Checklist items
All items that were suggested by experts, along with guideline considerations from current AI papers in medicine, were incorporated into one checklist. We created eight sections in our checklist according to the sections in any standard publication: Title, Abstract, Introduction, Methods, Results, Discussion, and Other (e.g., conflict of interest). Subsequently, a section on ground truth was also included in this survey based on expert feedback for gold standard comparison. The final checklist contained 8 sections and 50 questions following a “5-point Likert scale”.
Consensus process
As a part of the Delphi process, the first version of the Google Form survey was sent to the eight AI experts originally recruited to be part of this project. These individuals provided feedback and additional suggestions that were incorporated into this checklist. In the next round of Delphi, a final version was sent to all members for further review and approval. We then asked each individual in the HUMANE group to send out this checklist to 3–5 key opinion leaders (KOLs) or SMEs to complete in the third round. These SMEs and KOLs were invited from diverse geographical backgrounds and expertise in clinical medicine, research informatics, and ML. There were a total of 33 KOLs/SMEs recruited. Everyone evaluated the necessity of the checklist item on a 5-point Likert scale with 1 being “strongly disagree” and 5 “strongly agree”. An “additional comments” textbox was available at the end of each section. This concluded the Delphi process.
Data collection & analysis
Data analysis was carried out using Excel 10.15.5. Mean, median, and interquartile range (IQR) were calculated for each individual question. These results were then used to calculate the means and medians of each section. The country of origin for each expert was mapped using MapChart (https://mapchart.net/).
Results
A total of 33 SMEs/KOLs and collaborators participated in this Delphi process (Table 2). The majority of respondents were aged 36–45 years (15, 45.4%) and male (28, 84.8%). Most participants were from the USA (20, 61%), UK (8, 24.2%), and Australia (3, 9.1%) (Figure 2 and Table S1). The most common professions of these individuals were in healthcare (14, 42.4%) or physicians (11, 33.3%). Fourteen (42.4%) individuals were early in their career, 10 (30.3%) were in the middle of their career, and 9 (27.3%) had considered themselves to be late in their career.
Demographic characteristics of 33 key opinion leaders and subject matter experts
Characteristic | (n = 33) |
---|---|
Age | |
26–35 | 7 (21.2%) |
36–45 | 15 (45.4%) |
46–55 | 9 (27.3%) |
> 55 | 2 (6.1%) |
Sex | |
Male | 28 (84.8%) |
Female | 5 (15.2%) |
Location | |
USA | 20 (60.6%) |
UK | 8 (24.2%) |
Australia | 3 (9.1%) |
Other | 2 (6.1%) |
Profession | |
Healthcare | 14 (42.4%) |
Physician | 11 (33.3%) |
Information technology | 4 (12.1%) |
Engineering | 2 (6.1%) |
Other | 2 (6.1%) |
Professional level | |
Early career (3–10 years) | 14 (42.4%) |
Mid-career (11–20 years) | 10 (30.3%) |
Later career (> 20 years) | 9 (27.3%) |
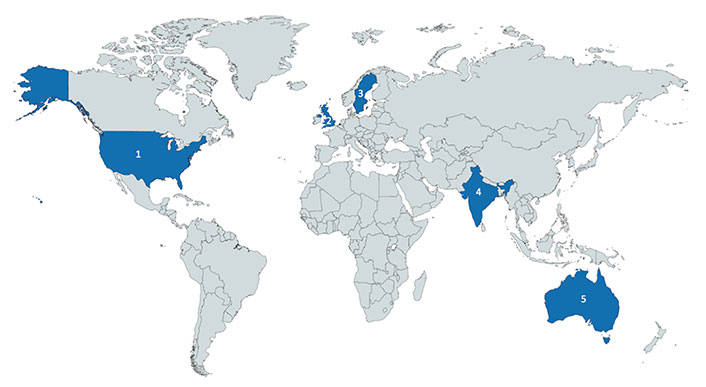
Country of origin of experts who participated the in derivation of the checklist. From left to right: 1. USA (n = 20); 2. UK (n = 8); 3. Sweden (n = 1); 4. India (n = 1); 5. Australia (n = 3). Generated by the tool provided by https://mapchart.net/ on 12/19/2020, licensed under CC BY-SA 4.0
In the initial survey, there were 8 sections and 56 questions. This was created by organizing sections based on feedback from stakeholders who recommended categories and through a literature review of AI in medicine. The first stage of the process involved moving different questions around into their relevant sections and additional questions suggested by AI experts (Table 1). Questions that were redundant were also removed. Questions related to reproducibility and generalizability of the dataset were included in the Results section. Two further sections were added: ground truth and declaration of conflicts of interest.
The second stage of the process involved experts scoring each question and providing comments on all current sections. Reviewers suggested adding in if ethics approval was necessary, and if not, stating why. Some questions needed to be separated into individual Yes/No options. For example, a question on the presence of a differential diagnosis and confidence intervals were separated. After the second stage of the process, there were 8 sections and 50 questions in the checklist. The key changes are summarized in Table 1.
Each individual question with Likert scale responses had an associated mean and median based on reviewer responses, which can be found in Table S2. Overall, the final version of the checklist ranged from a score of 4.2 to 4.8 on the Likert scale (Table 3 and Table S3). The most agreed upon section was the Discussion (Other) with a mean of 4.8 (± 0). The lowest scoring section was Section 5b: Ground Truth (Expert(s) Review) with a mean of 4.0 (± 0.20).
Checklist sections and their cumulative score
Checklist sections | Mean (± SD) | Median (25-75% IQR) |
---|---|---|
Section 1: Title | 4.4 (± 0.21) | 4.4 (4.3–4.4) |
Section 2: Abstract | 4.5* | 4.5 (4.5–4.5) |
Section 3: Introduction | 4.4 (± 0.21) | 4.3 (4.3–4.5) |
Section 4a: Methods (Data Source) | 4.4 (± 0.17) | 4.3 (4.2–4.4) |
Section 4b: Methods (Participants) | 4.4 (± 0.13) | 4.4 (4.4–4.5) |
Section 4c: Methods (Outcomes) | 4.2 (± 0.16) | 4.1 (4.1–4.1) |
Section 4d: Methods (Statistical Analysis) | 4.3 (± 0.16) | 4.3 (4.2–4.4) |
Section 5a: Ground Truth (Labels) | 4.2 (± 0.12) | 4.2 (4.2–4.2) |
Section 5b: Ground Truth (Expert(s) Review) | 4.0 (± 0.20) | 4.1 (3.9–4.2) |
Section 6: Results | 4.2 (± 0.21) | 4.2 (4.0–4.3) |
Section 7: Discussion | 4.2 (± 0.35) | 4.3 (4.1–4.4) |
7: Discussion (Other) | 4.8* | 4.8 (4.8–4.8) |
* Standard deviation unable to be calculated due to only having a single question in the section. IQR: interquartile range
This article is based on our previous research which was presented as a poster and published in the Beyond Sciences Initiative as a conference abstract (20220225 (2022) Beyond Sciences). Available at: https://www.beyondsciences.org/hotdoc2022/20220225/ (Accessed: 18 April 2024).
Discussion
Given that AI and ML in medicine a relatively novel concepts, creating a tool to help define AI in medicine research can be valuable to ensure consistency amongst new research findings. The HUMANE checklist incorporates valuable items that are relevant to AI with the help of current AI experts. Feedback from questions in the checklist was collected using a Likert scale that showcased an overall agreeable consensus (mean ranges 4.1–4.8, out of 5) as cumulative scores throughout all sections. The majority of the consensus was agreeable with the Discussion (Other) section of the checklist (median 4.8 (IQR 4.8–4.8)), whereas the least agreed section was the Ground Truth (Expert(s) review) section (median 4.1 (IQR 3.9–4.2)) and the Methods (Outcomes) section (median 4.1 (IQR 4.1–4.1)) of the checklist. Comments on modifying, removing, and accepting various aspects of the checklist were also implemented based on feedback provided. The final checklist after consensus and revision included a total of 8 sections and 50 questions. Through a two-round consensus method, we incorporated feedback from evidence-based recommendations. Questions were organized into appropriate sections according to a standard scientific article, such as the introduction, methods, and discussion. Overall, cumulative scores suggested a good consensus across all sections, however, minor adjustments were made to allow more clarity for the future readers.
Many core principles of AI algorithms should be included in any paper and therefore included in the HUMANE checklist. The title should be specific to the aim of the paper and should include relevant terms such as “ML” or “deep learning” [17]. With any introduction, it is imperative that the authors state the clinical goal, objectives, and the prediction problem being addressed [18]. Describing the clinical setting of the prediction model can highlight the goals of the prediction model, as well as their clinical suitability [17, 19]. However, translating these AI systems into the real-world setting is still a challenge and requires collaboration between AI researchers and clinicians [19].
Building a prediction model involves statistical analysis and modeling techniques which would need to be discussed in any AI paper [19]. The importance of data splitting into training, validation, and testing cohorts is important for evaluating AI technology and should be included in any paper [17]. However, one of the current challenges in data splitting is access to large datasets that are suitable, and so this should be kept in mind when creating any program [20].
With any AI program, there is a risk of biases due to the nature of the program or how the software is designed [20]. Generally, clinical AI systems aim to produce results with high sensitivity and specificity. However, it may discriminate against certain groups of patients, especially if they were not included in the data used in the development of the algorithm [20, 21]. Therefore, it is crucial that researchers use validation datasets that are representative of the target population or address the presence of overrepresented subgroups if there are any [22]. These questions were put into consideration for our checklist and are included in the Results section due to their significance.
The strengths of the HUMANE checklist include the ability to be utilized by various medical specialties as it takes into consideration the general format of AI research. This checklist was evaluated by 33 stakeholders in AI and their feedback was incorporated every step of the way, thus creating a checklist that is comprehensive in nature. It will allow individuals to communicate their AI study in a clear and concise format. Additionally, we were able to create this checklist without the necessity of external funding, rather, we integrated information from current AI online resources and recent literature.
Limitations to this study exist. Although our studies recruited 33 experts who were familiar with AI research in medicine, we have limited representation from all continents. Areas such as Africa and South Asia were not represented in this study and so we may be missing vital add-ons or changes to the survey. Furthermore, as this is a generalized checklist for AI in medicine, it may leave out valuable items that may differ from specialty to specialty. Future studies should appropriately implement the checklist with this in mind. One should also consider that the demographics of the stakeholders may not represent the demographics of the target population who use this survey. Finally, this study was completed in 2020, which may raise concerns; however, this Delphi process did not include any duration-sensitive literature so it would not influence the results.
In conclusion, the HUMANE checklist can act as a guide for researchers in writing and evaluating papers on AI in medicine. A standardized checklist can provide value for future researchers in AI within the realm of medicine. Through a two-round consensus method, we utilized the knowledge from experts and current literature to create a robust checklist. The checklist is being validated in specific medical fields such as hypertension research [23, 24], followed by a broader review of medical AI research in critical care medicine (sepsis), endocrinology (diabetes), and dermatology (skin disease labeling).
Abbreviations
AI: | artificial intelligence |
HUMANE: | Harmonious Understanding of Machine Learning Analytics Network |
IQR: | interquartile range |
KOLs: | key opinion leaders |
ML: | machine learning |
SMEs: | subject matter experts |
Supplementary materials
The supplementary tables for this article are available at: https://www.explorationpub.com/uploads/Article/file/101118_sup_1.pdf.
Declarations
Author contributions
ND and FAN equally contributed to: Conceptualization, Investigation, Writing—original draft, Writing—review & editing. CT and TT: Investigation, Formal analysis, Writing—review & editing. CM, PM, SR, SV, TP, KM, and SP: Validation, Writing—review & editing, Supervision. RK: Conceptualization, Investigation, Validation, Writing—review & editing, Supervision. All authors read and approved the submitted version.
Conflicts of interest
The authors declare that they have no conflicts of interest.
Ethical approval
Not applicable.
Consent to participate
All participants in this study agree to participate.
Consent to publication
All participants in this study agree to publish their personal information.
Availability of data and materials
The raw data supporting the conclusions of this manuscript will be made available by the authors, without undue reservation, to any qualified researcher.
Funding
Not applicable.
Copyright
© The Author(s) 2024.
Publisher’s note
Open Exploration maintains a neutral stance regarding jurisdictional claims in published maps and institutional affiliations.