Abstract
Healthcare strives to ensure overall physical, mental, and emotional well-being for individuals while managing limited resources efficiently. Digital technologies can offer cost reduction, improved user experience, and expanded capacity. In addition, modern automation technologies, which were implemented in industrial control systems or industrial automation control systems, are essential for ensuring the availability of societies’ critical cyber-physical systems (CPSs) and the services they provide, such as healthcare. This narrative literature review produces information that can be applied when planning and implementing an interdisciplinary biomedical and health informatics (BMHI) master’s education focused on the challenges of digitalization in the health sector. The review results that virtual human twins (VHTs) are revolutionizing healthcare by addressing people’s complex medical problems with real-time monitoring and precision care while digital twin (DT) technology can make the hospital’s operational processes resilient and efficient. Thus, future BMHI education must address these technologies with a multidisciplinary approach, including computer science, information science, engineering, basic sciences, health sciences, socio-behavioral sciences, and ethical, legal, and policy aspects. Collected and cumulative data is essential for cognitive DTs. A prerequisite for this data is information sharing between different CPSs. Better information sharing and the development of scalable cognitive DTs and VHTs, the provision of critical services, quality, and cost-effectiveness, as well as health, safety, and resilience, will improve. Similarities between peoples’ health information exchange and information needed for ensuring the resilience of CPSs exist. Since humans are in many ways more complex than CPSs, security engineers have a lot to learn from VHTs in maintaining the resilience of CPSs. Cross-sectoral research and cooperation with different disciplines are essential for the progress of both human health and the resilience of CPSs. Along with interdisciplinary research cooperation, educational cooperation should also be intensified.
Keywords
Biomedical and health informatics, digital twins, virtual human twins, cognitive digital twins, people’s health, system resilience, health interventionsIntroduction
The health sector has been under immense pressure to respond to the protection, safety, and increasing well-being needs of aging societies such as Europe [1]. The current pandemic situation has given greater centrality and urgency to the health sector and related service providers [2]. The public expects more capacity and faster response from health systems. Digital technologies offer significant opportunities to reduce costs, improve user experience, and increase the capacity of health systems and services, giving new horizons to scientific work and organizational models that address it [3]. On the other hand, modern automation technologies, deployed in modern industrial control systems or industrial automation control systems, have become pervasive, playing a crucial part in ensuring the availability of essential and critical services, such as smart grid, water distribution, digital healthcare, etc. [4].
Digital technologies contribute to both knowledge and operating models/systems. The massive processing of clinical, administrative, or system status data, in the context of preventive or clinical medicine and of service management, the sensing of biological responses in monitoring therapies or in diagnosis, the integration between equipment and data, the robotization of organizational procedures, the virtualization of specific contexts of interaction and the adoption of remote care models are examples of the contribution of digital technologies to health sector transformation [5]. According to Khan et al. [6], digital twin (DT) technology is one of the fastest-growing digital health technologies.
A DT is a virtual representation of a physical entity that was first introduced in 2002 as a concept in the manufacturing industry for product life-cycle management [7]. With this method, the real physical world could be described in a cyber world [8] utilizing various data sources such as sensors, Internet of Things (IoT) devices, and other digital sources [9]. A DT mirrors its physical counterpart in real-time or near-real-time, allowing its behavior and performance to be monitored, analyzed, and simulated [10]. A DT can provide a faithful environment for security analysis or evaluation of potential mitigation strategies to be deployed in the face of specific situations [4].
The objective of this narrative review [11] is to contribute to the promotion of excellence and innovation in postgraduate higher education in the healthcare sector. A narrative review methodology has been applied because it is useful when exploring topics that are under-researched as well as for new insights or ways of thinking regarding well-developed, robustly researched fields [12]. The goal is to produce information that can be used in the ManagiDiTH project [13] when planning and implementing an interdisciplinary master’s level education focused on the challenges of digitalization in the field of biomedical and health informatics (BMHI) where information and communication technologies intersect with health, healthcare, life sciences, and biomedicine. The research question is: How should DT technology be considered in the BMHI education offered by higher education institutions? When searching for literature from databases (Google Scholar, ACM Digital Library, ProQuest Central, ScienceDirect, Web of Science), the research question was iteratively divided into pieces, such as what the current landscape of digital health and healthcare system is, and what the state of the art in DT technology in higher education is. The inclusion criteria were that the study in question added value to the original research and that it had undergone peer review. In the absence of research literature, online materials from trusted sources have been used in topics critical to the research.
The content of the rest of the article is divided as follows: The second section explores how digitization can enhance health outcomes while highlighting the increased demands it places on healthcare system resilience. The third section outlines recommendations from the International Medical Informatics Association (IMIA) regarding the teaching of BMHI in higher education institutions. The fourth section delves into technologies enabling DTs and associated terminology. The fifth section discusses the application of DT technology in higher education. The sixth section provides insights into DT research within the healthcare ecosystem. The last section offers concluding remarks for this review.
Current landscape of digital health and healthcare system
A healthy person generally refers to an individual who possesses overall physical, mental, and emotional well-being. Healthcare aims to pursue improved benefits for patients, the population, and society, producing as much benefit as possible and causing as little harm as possible to patients taking equity into account [14]. Since healthcare resources are always limited, cost considerations are necessary and new cost-effective technologies and services need to be developed in public healthcare providers, technology companies, and higher education institutions [15].
The utilization of digital technologies corresponds to the growing demand for designing patient-centered healthcare systems, emphasizing responsiveness to individual needs [16]. This transition towards patient-centered health services underscores the human and social dimensions of technological advancement. However, promoting this shift necessitates the cultivation of new qualifications and skills that integrate sector-specific knowledge, understanding of service users, and proficiency in supporting technologies. In essence, the era of digital transformation underscores the importance of interdisciplinary collaboration, drawing connections between diverse areas of expertise and resources [17].
Electronic health records (EHR) facilitate the storage, retrieval, and sharing of health information among healthcare professionals [18]. The introduction of EHR has paved the way for health information exchange (HIE) and data interoperability, playing crucial roles in the modern healthcare system [19]. The real-time accessibility of comprehensive patient health data allows healthcare professionals to make informed decisions promptly, ensuring the delivery of high-quality and safe care and promoting the safety of patients [20]. Moreover, these technologies help minimize redundant tests and administrative burdens, ultimately leading to cost savings for healthcare organizations and reduced paperwork for patients. The significance of HIE and data interoperability became exceptionally evident during the COVID-19 pandemic when the need for rapid and widespread health data exchange was akin to assembling a complex puzzle [21]. Recent artificial intelligence (AI) models based on HIE data demonstrated commendable predictive ability in clinical evaluations, highlighting the transformational potential of combining AI and HIE [22]. As the healthcare path becomes intertwined with AI, a diverse and informed approach is essential to ensure the delivery of reliable and effective AI-enhanced healthcare solutions [22]. As AI spreads, concerns about risk, trust, and security will emerge as reliance on AI-based healthcare systems increases [23]. The quality of EHR data is also variable. Although there are consistent assessment models, there is still no standard method for assessing the quality of EHR data [24]. According to Lewis et al. [24], scalable and flexible guidelines are needed for EHR data quality assessment to improve the efficiency, transparency, comparability, and interoperability of data quality assessment.
The healthcare system is one part of our society’s critical infrastructure which is a complex network of cyber-physical systems (CPSs) interconnecting various sectors, such as energy, telecommunications, and financial services [25]. The healthcare system exhibits strong interrelationships, especially with information and communication technology and energy. Disruptions in the electrical grid or telecommunications can significantly impact healthcare operations, efficiency, and costs [26].
Resilience refers to a system’s ability to withstand, adapt to, and recover from disturbances, shocks, or changes while maintaining its core functions and integrity [27]. Unfortunately, critical infrastructure lacks inherent resilience, typically losing essential functionality following adverse events [28]. Therefore, resilience must be systematically built as a feature of all critical systems [29]. In the engineering field, a resilient system can refer to structures, networks, or processes designed to tolerate and recover from failures, disruptions, or extreme conditions. This could include buildings designed to withstand earthquakes, power grids with backup systems, or computer networks with redundancy to ensure continued operation in the face of cyberattacks [28].
Cognitive situational awareness and common operational picture (COP) represent crucial elements when diverse stakeholders in emergency response must collaborate to manage a significant crisis involving multiple events that pose potential threats to critical infrastructure or life [30]. The COP is collaboratively developed by involved parties, taking into account available information, and encompasses a depiction of the ongoing situation, and future predictions, as well as considerations of resources and actions [31]. The cognitive nature of situational awareness is imperative for the success of emergency management efforts. The COP plays a pivotal role in aiding decision-makers to make informed decisions while coordinating operational units through various event stages [30]. However, creating COP and promoting cognitive situational awareness in an emergency is usually late. Therefore, their continuous maintenance is a necessary condition for building the resilience of a complex network of CPSs such as the healthcare system.
Biomedical and health informatics education
The IMIA has drawn up recommendations for teaching BMHI [32] and health information technology competencies [33]. These include, among other things, understanding the principles of new technologies related to the field, such as blockchain, cloud, edge computing, IoT and DTs. The purpose of the IMIA recommendations is fourfold [34]:
to aid educators in crafting BMHI curricula across various educational tiers,
to pinpoint vital skills and competencies for certifying healthcare professionals and BMHI practitioners,
to furnish evaluators of academic BMHI programs with a benchmark for assessing program quality and accreditation, and
to encourage universities, organizations, and health authorities to acknowledge the necessity of establishing and enhancing BMHI educational initiatives.
A trend brought by digitization in curriculum planning is the integration of disciplines. The core competencies related to BMHI are shown in Figure 1, where the white circle in the middle represents the core competencies specific to BMHI. The eight outermost sections describe disciplines that share knowledge, methods, and tools with BMHI. The area surrounded by a dashed line represents overlaps of disciplines and their impact on the BMHI [34]. Many disciplines in Figure 1 also overlap. For example, data science is an interdisciplinary field that combines knowledge and skills from computer science, statistics, and application domain [35]. Basic sciences refer to studies that support the versatility of professional education in different knowledge areas [36]. Nursing, in particular, is such a comprehensive knowledge area that it cannot do without basic sciences [36].
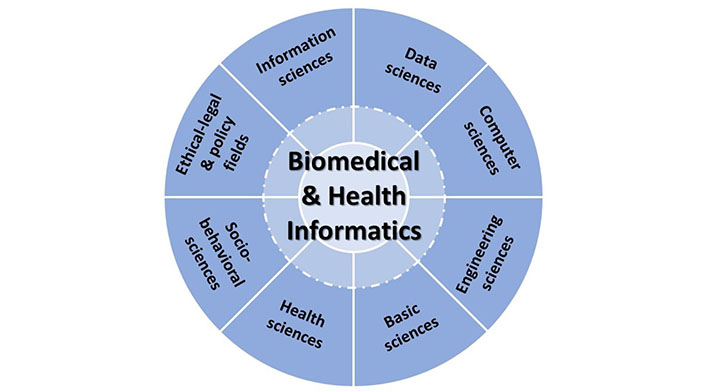
Biomedical and health informatics and related disciplines [34]
Note. Adapted with permission from “Recommendations of the International Medical Informatics Association (IMIA) on Education in Biomedical and Health Informatics: Second Revision” by Bichel-Findlay J, Koch S, Mantas J, Abdul SS, Al-Shorbaji N, Ammenwerth E, et al. Int J Med Inform. 2023;170:104908. (https://www.sciencedirect.com/science/article/abs/pii/S1386505622002222). © 2022 Elsevier B.V.
New multidisciplinary programs developed based on the IMIA recommendations are to give students the skills to effectively navigate the healthcare industry and utilize digital technologies to innovate practices and improve service offerings. Studies have shown that many healthcare professionals have low levels of digital literacy [37]. The extensive use of information technology in the health sector has led to the need for all healthcare workers to have some basic knowledge of BMHI, the amount of which depends on the employee’s position, healthcare role, amount of responsibility, education level, and career development. The reform of the recommendations now proposes three BMHI roles—user, generalist, and specialist [34]. However, these roles described in Table 1 should not be considered general job and education categories rather than job descriptions.
Biomedical and health informatics (BMHI) roles [34]
BMHI roles | Description |
---|---|
BMHI user | Learners from non-BMHI disciplines in healthcare who utilize information systems for data management. Their education covers fundamental BMHI topics and should be integrated into various healthcare curricula. They need familiarity with computer systems, software, and information and communications technology. This can be achieved through mandatory BMHI subjects or by integrating BMHI aspects into existing courses. |
BMHI generalist | Including learners from non-BMHI healthcare disciplines, focusing on intermediate-level education. They bridge information technology and healthcare, mastering skills such as eliciting clinical user needs, advising on system requirements, developing and implementing clinical information systems, and evaluating their effectiveness. They also gain knowledge of emerging technologies’ application in health services. This requires a mix of computer science, healthcare services, and BMHI subjects. |
BMHI specialist | Postgraduate learners with advanced expertise in their chosen BMHI discipline. They develop new information processing methods, deliver training, and conduct research. Specialists often earn master’s or doctoral degrees in BMHI or complete dedicated postgraduate programs. They typically work in academia and pursue research careers. |
Enabling technologies and digital twin terminology
DTs have matured thanks to advances in AI, machine learning (ML), big data, and the IoT. Recent advances in these technologies have greatly improved DT technology, developing DTs’ real-time capabilities and forecasting accuracy. DTs have spread to different application areas as a way to virtualize different physical entities. DT data is often obtained via IoT-connected devices, enabling the gathering of high-level information that can subsequently be merged into the virtual model [38]. Table 2 presents different types of IoT-related systems that can be utilized to generate DT data.
IoT systems
IoT type | Description |
---|---|
Internet of Things (IoT) | An information network is composed of numerous interconnected things including sensors, actuators, controllers, human operators, etc. [39]. |
Industrial Internet of Things (IIoT) | The use of conventional IoT concepts in industrial applications [39]. |
Internet of Medica Things (IoMT) | Medical things with the facility to transfer data over a network without demanding human-to-human or human-to-computer interaction [40]. |
Internet of Vehicles (IoV) | A decentralized network of vehicles allowing the utilization of data generated by linked automobiles and Vehicular Ad Hoc Networks (VANETs) [38]. IoV is outfitted with sensors, software, and technologies linking and exchanging data over the Internet following agreed-upon standards [41]. |
Internet of Drones (IoD) | An infrastructure aiming to offer control and access to drones and users over the Internet. Drones are quickly becoming commonplace commodities, allowing each user to fly many missions in regulated airspace [38]. |
Social IoT (SIoT) | Modeling the internet-worked devices based on relationships among humans and common tasks [39]. |
Internet of Services (IoS) | Uses the internet as a medium for offering and selling services [39]. |
Internet of People (IoP) | Considers humans as part of the network structure rather than just data sources. People act as a part of the network management services [39]. |
Internet of All (IoA) | Includes not only traditional IoT but also humans as a fundamental element [39]. |
Internet of Everything (IoE) | Connects humans, data, processes, and entities to enhance business communication and facilitate employment, well-being, education, and healthcare among various communities of people [39]. |
DTs offer various capabilities by integrating modeling, real-time data collection, and data analytics. These capabilities are increasingly used to predict system health and resilience, detect anomalies, perform proactive maintenance, and explore “what if” scenarios without disrupting physical operations. DTs are mainly used in engineering fields such as aerospace, civil, energy, manufacturing, and mechanical systems. They also offer healthcare systems opportunities to meet the challenges of patient care, physician well-being, and facility operations. However, the implementation of a DT in healthcare is more complex due to socio-technical factors such as biological processes, human decision-makers, and cooperation between service providers and technology. These complexities make it challenging to monitor healthcare processes in real-time, create accurate virtual replicas, and predict behavior effectively [42].
The discrepancy in definitions of DTs stems from their varied application areas and intended use cases. For example, NASA’s definition of DT encapsulates purposes across the lifecycle of space missions [43], while in manufacturing, real-time monitoring and control of processes are prioritized [44]. Confusion arises from inaccurate labeling of DT research in different fields, often due to unclear distinctions between terms like digital mode (DM), digital shadow (DS), and digital twin (DT) [45].
As shown in Figure 2, the terms DM, DS, and DT differ in the richness of data integration between physical and virtual objects, with DTs requiring bidirectional data flow. It’s crucial to recognize these distinctions to avoid mislabeling in the research literature. Modeling methods create digital replicas from physical artifacts while twinning methods link the physical and virtual worlds. Understanding these methods is essential for addressing challenges and developing new approaches in various fields, including healthcare systems [42].
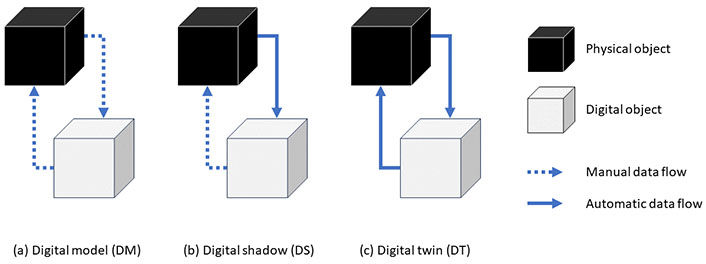
A visualization of the terms digital model, digital shadow, and digital twin [42]
Note. Adapted from “A systematic literature review of digital twin research for healthcare systems: research trends, gaps, and realization challenges” by Xames MD, Topcu TG. IEEE Access. 2024;12:4099–126 (https://ieeexplore.ieee.org/abstract/document/10379674). CC BY.
The DT acts as a digital representation of a physical system, encapsulating parameters such as temperature, velocity, pressure, and frequency from their physical equivalent into digital form [46]. In addition to mirroring the data and structure of the physical twin, the digital component contains algorithms to replicate the physical constraints and functions [47]. When the DT is connected to a physical dual unit, users can access and manipulate low-level functions. This feature facilitates manual or AI-based analysis and optimization of physical systems and processes. The DT Consortium provides a glossary of terms related to DTs, including the following definitions [48]:
DT is a virtual presentation of real-world entities and processes synchronized at specified frequency and fidelity.
Physical twin is a set of real-world entities and processes that correspond to a DT.
DT platform is a set of integrated services, applications, and other DT subsystems that are designed to be used to implement DT systems.
Digital twin technology in higher education
Education, training, and collaboration are integral parts of modern society, fostering intelligence, problem-solving, and innovation beyond the capabilities of individuals. The training focuses on increasing knowledge and the goal of the training is to develop skills. Traditional methods included on-site events such as lectures and group work, but digitization has given rise to a generation accustomed to learning through digital platforms. Teachers now face the challenge of adapting to this digital native generation and utilizing digital tools for education and collaboration, called Education 4.0 [49]. Similarly, Industry 4.0 reflects the digital evolution of the industry, utilizing technologies such as AI, IIoT, virtual engineering, and extended reality (XR) to improve efficiency [50]. Virtual environments (VEs) provide natural learning and collaboration environments for digital natives, facilitating immersive training and risk-free simulations [46]. The integration of DT enables interaction with real hardware, bridging the virtual and physical worlds. Two-way communication enables physical robots to be operated remotely from the VE, which ensures safe training environments. As industries move into the metaverse, DTs play a key role in bridging the virtual and real worlds, enabling multi-user VEs for different purposes [46].
DT technology spans multiple disciplines, including engineering, computer science, data analytics, and domain-specific fields such as healthcare and smart cities [51]. In higher education, DT technology is mostly utilized in engineering, computer science, and related fields, where it increases understanding of complex systems, provides realistic scenarios, and promotes immersive learning experiences [52]. Courses covering topics such as simulation modeling, data analytics, and IoT technologies provide students with the foundational knowledge and skills needed to understand and implement DT systems. Educators can assess student performance and provide feedback using DT platforms that provide flexibility for model development [52].
In healthcare, a DT can be, for example, a predictive modeling of a person and their health status based on health history and is an effective way to provide more accurate and faster services for, for example, elderly healthcare [53]. With the help of ML, the correspondence of the DT to the physical space can be improved, and this concept has great potential to change the current healthcare system by making it more individualized in two ways [54]. When focusing on the biological aspect, the meaning of personalization is like accuracy, where the general goal of personal healthcare is to fine-tune healthcare with health-related information and provide treatments that are likely to be most effective and cause the least harmful side effects for the patient [54]. Achieving personalization in this sense does not require active participation from the patient, because the biological perspective of the DT can be taught, for example, with the help of health sensors [55]. In contrast, when focusing on the non-biological aspect, implementing personalization means respecting the personal commitments and values of individual patients. In this sense, personal healthcare is the ideal of healthcare, the goal of which is to restore the patient’s freedom of will and help the patient to independently choose a course of treatments that best reflects his values or meets his special needs [54]. Thus, improving the patient’s physical health is not a primary consideration, but it opens opportunities for a person’s individual, situational emphasis on ethical values, and how he or she should act in various situations requiring ethical decision-making.
In training healthcare professionals, DT technology provides a dynamic platform for simulating clinical scenarios, allowing students to practice decision-making, patient management, and surgical procedures in a risk-free environment. By interacting with realistic patient models, students can enhance their diagnostic skills, treatment planning abilities, and communication with patients. The European virtual human twins (VHTs) initiative is an EU framework that supports the emergence and adoption of the next generation of VHT solutions in healthcare [56]. Its key opportunities relate to targeted prevention, tailored clinical pathways, and supporting healthcare professionals in VE. The VHT initiative helps to develop, run, and better simulate the models in ways previously not possible, speeding up the development of VHTs through enabling large AI foundational model training specific to human health and diseases [56]. According to Viceconti et al. [57], each DT in healthcare is developed with a specific clinical use in mind, but the VHT is an infrastructure that facilitates the development and validation of DTs, as well as enabling the reuse of existing models to develop new, more complex models.
Perspectives of digital twin research for healthcare ecosystem
A DT is both a DS reflecting the status/operation of its physical twin (e.g., hospital operations or patients) and a digital thread recording the evolution of the physical twin over time [58]. A DT relies on both long-term collected data and real-time sensor data to enable predictions, anticipate failures, optimize systems, design new features, accelerate decision-making, and increase productivity [58]. The collected and cumulated data serve as basic data for simulations and ML algorithms. DTs have already evolved beyond mere models of physical assets. Increasingly, they develop autonomously through simulation and AI-driven algorithms, allowing them to understand, learn, reason, and react to hypothetical scenarios [59]. However, the virtualization of physical assets has so far only been implemented from the point of view of a closed system. Ricci et al. [59] introduce the Web of Digital Twins approach in which the DT paradigm is meant to be pervasively applied to virtualize large-scale, dynamic, possibly cross-domain physical realities of an organization and across different organizations, resulting in an open distributed ecosystem of connected DTs.
In the healthcare ecosystem, DT technology can change public health by revolutionizing planning, enabling real-time monitoring and analysis, facilitating predictive analytics, personalizing healthcare, enhancing training, and promoting collaboration [6] and this development should also be taken into account in education and research. According to Khan et al. [6], DT technology can lead to better health outcomes, optimized resource allocation, and improve the well-being of individuals and communities through, for example, the following applications: (1) improved and faster mass immunization planning, (2) remote and personalized health management, (3) smart health cities, (4) medical facility and operations design, (5) clinical research, (6) drug and vaccine development, (7) health equity planning, and (8) treatment optimization.
Above mentioned examples illustrate the potential of DT technology to revolutionize healthcare across various domains, driving innovation, improving outcomes, and addressing critical healthcare challenges. On the other hand, while DT technology has seen significant adoption in various industry sectors, such as manufacturing, construction, automobile, aerospace, smart manufacturing, and electricity [10], its integration into public health interventions remains limited. In utilizing DT technology to its full potential in addressing public health challenges, there appear to be several gaps that should be explored in future research programs. Khan et al. [6] identify the following as the key limitations and challenges related to implementing DTs in digital public health: (1) data privacy and security, (2) data interoperability and standardization, (3) ethical considerations, and (4) skill and knowledge gaps. These limitations and challenges also apply to many other sectors.
The DT concept has also been developed for the infrastructure domain to improve the resilience of critical systems [60], such as the public healthcare system. In the development of security monitoring and resilience management mechanisms for critical healthcare infrastructures, DT technology is becoming an integral part of cognitive situational awareness [61]. This development is leading to the concept of cognitive DTs (CDTs), which autonomously perform intelligent tasks about physical assets, such as intelligent management, maintenance, and performance optimization, or at least guide a human operator in making such decisions [62].
Conclusions
The paper focuses on BMHI education, and its purpose is to produce basic information that our university needs when developing BMHI education. When developing DT technology and planning curricula and research in health universities, a clear trend is the integration of disciplines that are closely related to BMHI’s core area. As shown in Figure 1, DT and VHT development of healthcare ecosystems requires collaboration and integration between the following disciplines, each providing unique expertise and perspectives to create comprehensive and efficient models of physical systems:
Information sciences provide methodologies for managing, organizing, and using information related to physical systems. These include techniques for data integration, information retrieval, knowledge representation, and database management. They ensure DTs have access to relevant and reliable information for modeling and analysis.
Data sciences provide techniques for processing, analyzing, and interpreting large volumes of data produced by the physical system. Statistical analyses, ML, and data visualization improve DT models’ accuracy and efficiency.
Computer sciences provide the algorithms and computational infrastructure needed to develop and implement DTs. These include software engineering, computer graphics, visualization tools, simulation models, real-time monitoring systems, and algorithms for data fusion, optimization, and control.
Engineering sciences provide expertise in mathematical modeling, simulation, and control of physical systems. These allow DTs to simulate different scenarios, analyze performance, and optimize operations in real-time.
Basic sciences provide the foundational principles and theories that underpin the understanding of physical phenomena and systems. Physics, chemistry, biology, and mathematics describe the behavior of the physical world and are necessary for creating accurate and reliable models in DT environments.
Health sciences contribute to the development of VHTs by providing insights into human physiology, anatomy, and disease mechanisms. Medical professionals and researchers in collaboration with engineers and data scientists can develop VHTs that simulate biological processes, predict disease progression, and optimize treatment strategies.
Socio-behavioral sciences deal with the social, cultural, and organizational dynamics, behavioral aspects, and human factors that affect the adoption and use of DT technologies. Social-behavioral research provides information on the development of user interfaces, training programs and implementation strategies to promote the acceptance and implementation of DTs.
Ethical, legal, and policy considerations are critical in the development and deployment of DTs in the healthcare domain. Data privacy, security, ownership, and liability issues ensure that DT technologies comply with ethical standards and regulatory requirements.
By leveraging insights from all above mentioned BMHI-related disciplines, DTs can drive innovation, improve decision-making, and address complex challenges in various domains. DT teaching in health higher education institutions should be planned separately for all three BMHI roles: BMHI user, BMHI generalist, and BMHI specialist.
DTs and VHT will play a key role in people’s health and healthcare services in the future offering considerable opportunities for solving complex medical problems, such as real-time monitoring, dynamic analysis, and precise treatment. DT technologies change operating methods, and organizational boundaries and require the cooperation of public, private, and third-sector actors in creating new services. Strengthening digital expertise, functioning services, and updating processes are key to the successful introduction of digital services. With the principles of the platform economy, it is possible to make even better use of the many possibilities offered by digital technology, personalized data, and AI.
Collected and cumulative data are essential for cognitive situational awareness, a COP, and CDTs. A prerequisite for this is a functional exchange of information between different CPSs in the data, technology, and social domains. Better information sharing and the development of scalable CDTs and VHTs, the provision of critical services, quality, and cost-effectiveness, as well as health, safety, and sustainability, will improve. There are similarities between the HIE of people and the HIE of CPSs (cognitive situational awareness and COP). Humans are in many ways more complex than CPSs, so security engineers have a lot to learn from healthcare professionals about their DTs in maintaining the resilience (“health”) of CPSs. That’s why research that crosses scientific boundaries should be done even more in the future, and the results of VHT research must be utilized in CDT development and vice versa. Along with interdisciplinary research cooperation, educational cooperation should also be intensified.
Abbreviations
AI: | artificial intelligence |
BMHI: | biomedical and health informatics |
CDTs: | cognitive digital twins |
COP: | common operational picture |
CPSs: | cyber-physical systems |
DS: | digital shadow |
DT: | digital twin |
EHR: | electronic health records |
HIE: | health information exchange |
IMIA: | International Medical Informatics Association |
IoT: | Internet of Things |
ML: | machine learning |
VEs: | virtual environments |
VHTs: | virtual human twins |
Declarations
Acknowledgments
Disclaimer: Views and opinions expressed are those of the author(s) only and do not necessarily reflect those of the European Union or European Commission. Neither the European Union nor the granting authority can be held responsible for them.
Author contributions
JR: Conceptualization, Data curation, Formal analysis, Writing—original draft, Writing—review & editing. The author read and approved the submitted version.
Conflicts of interest
The author declares that there are no conflicts of interest.
Ethical approval
Not applicable.
Consent to participate
Not applicable.
Consent to publication
Not applicable.
Availability of data and materials
Not applicable.
Funding
The work was supported by the Master of Managing Digital Transformation in the Health Sector (ManagiDiTH) Project funded by the European Health and Digital Executive Agency (HADEA) EU executive agency, under the powers delegated by the European Commission. The project number is [101083896]. The funders had no role in study design, data collection and analysis, decision to publish, or preparation of the manuscript.
Copyright
© The Author(s) 2024.