Abstract
Aim:
This study aims to evaluate the accuracy and readability of responses generated by two large language models (LLMs) (ChatGPT-4 and Gemini) to frequently asked questions by lay persons (the general public) about signs and symptoms, risk factors, screening, diagnosis, treatment, prevention, and survival in relation to oral cancer.
Methods:
The accuracy of each response given in the two LLMs was rated by four oral cancer experts, blinded to the source of the responses. The accuracy was rated as 1: complete, 2: correct but insufficient, 3: includes correct and incorrect/outdated information, and 4: completely incorrect. Frequency, mean scores for each question, and overall were calculated. Readability was analyzed using the Flesch Reading Ease and the Flesch-Kincaid Grade Level (FKGL) tests.
Results:
The mean accuracy scores for ChatGPT-4 responses ranged from 1.00 to 2.00, with an overall mean score of 1.50 (SD 0.36), indicating that responses were usually correct but sometimes insufficient. Gemini responses had mean scores ranging from 1.00 to 1.75, with an overall mean score of 1.20 (SD 0.27), suggesting more complete responses. The Mann-Whitney U test revealed a statistically significant difference between the models’ scores (p = 0.02), with Gemini outperforming ChatGPT-4 in terms of completeness and accuracy. ChatGPT generally produces content at a lower grade level (average FKGL: 10.3) compared to Gemini (average FKGL: 12.3) (p = 0.004).
Conclusions:
Gemini provides more complete and accurate responses to questions about oral cancer that lay people may seek answers to compared to ChatGPT-4, although its responses were less readable. Further improvements in model training and evaluation consistency are needed to enhance the reliability and utility of LLMs in healthcare settings.
Keywords
Large language models, ChatGPT, Gemini, oral cancerIntroduction
The potential of artificial intelligence (AI) in dentistry, particularly in oral medicine, is gaining importance [1]. The recently launched ChatGPT, an AI tool developed by OpenAI, is a model trained on large amounts of data capable of understanding and generating human language with high precision and consistency. On March 14, 2023, OpenAI introduced ChatGPT-4, an updated subscription-based model claiming better performance compared to ChatGPT-3.5, including interpreting images, responding to questions about their content, and generating context-specific descriptions (OpenAI, https://cdn.openai.com/papers/GPTV_System_Card.pdf).
As AI large language models (LLMs) like ChatGPT gained popularity, other models were also developed. Bard, the predecessor of Gemini, was introduced by Google in 2023 as a conversational AI chatbot. Despite its capability to generate text, translate languages, and create various types of creative content, Bard’s development focused primarily on providing informative answers. In 2024, Google rebranded Bard as Gemini, updating it with enhanced abilities to comprehend and respond to complex questions, generate different text formats, and translate languages more accurately and naturally. Gemini represents a significant step in the evolution of AI chatbots (https://deepmind.google/technologies/gemini/).
Researchers from different medical specialties have started exploring the utility of LLMs, evaluating especially their accuracy in answering specialty-specific questions [2]. Prior to or during the time of hospital visits, patients attending Oral Medicine clinics may also consult these LLMs to self-assess their conditions, find answers to queries they have, and further understand the treatment plan proposed by the clinician and any recommended procedures, or to familiarize with what other experts say about their condition and treatments. Additionally, they support multilingual communication through language translation. As LLMs expand and become further customized for oral medicine, they could enhance patient care, improve information accessibility, and contribute to advancements in this dental specialty [3]. Their potential applications in oral cancer span a wide spectrum, from enhancing preventive strategies to assisting in understanding treatment complications.
This study aimed to evaluate the accuracy and readability of responses from two LLMs (ChatGPT-4 and Gemini) to frequently asked questions by lay persons (the general public) who are searching for information and answers to questions about oral cancer. These individuals are typically members of the general public, including those who may be personally affected by oral cancer (and being seen in clinics) or who are seeking information out of concern for themselves or loved ones about signs and symptoms, risk factors, screening and diagnosis, treatment, and survival in relation to oral cancer.
Materials and methods
Selection of questions
The summary of the study workflow is shown in Figure 1. To identify possible queries from non-experts in oral cancer, a Google search using the keywords “frequently asked questions about oral cancer” AND “common oral cancer questions” was conducted in March 2024 with the virtual private network (VPN) disabled. The search yielded a number of queries based on patient guidelines developed by institutions, hospitals, and scientific societies like the Mayo Clinic, National Institute of Dental and Craniofacial Research, American Dental Association (ADA), American Head and Neck Society, Moffitt Cancer Center, the American College of Prosthodontists, and British Columbia Cancer Center. Two researchers (MDF, PDD) selected 15 questions covering various topics, including oral cancer detection, signs and symptoms, screening and diagnosis, treatment, treatment complications, prognosis, and survival (Table 1). These questions were entered into ChatGPT-4 (April 23, 2023 version, OpenAI) and Google Gemini AI (Google) using the “New Chat” function for each question.
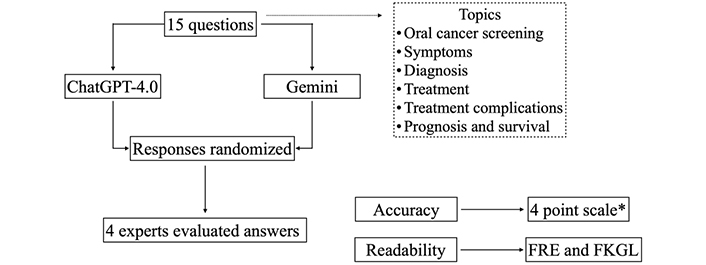
Study workflow. FKGL: Flesch-Kincaid Grade Level; FRE: Flesch Reading Ease; *: 1: complete, 2: correct but insufficient, 3: includes correct and incorrect/outdated information, 4: completely incorrect
Frequently asked questions about oral cancer
Questions about oral cancer |
---|
1. What is oral cancer screening?2. How often should I have an oral cancer screen?3. What are the benefits of oral cancer screening?4. How long does oral cancer screening take?5. How common is oral cancer?6. What are the early signs and symptoms of oral cancer?7. What are the early signs of lip cancer?8. How is oral cancer diagnosed?9. What are the risk factors for developing oral cancer?10. How could one prevent oral cancer?11. What treatment options are available for oral cancer?12. What are the side effects of oral cancer treatments?13. What is the prognosis and survival rate for oral cancer?14. How can lifestyle changes impact the progression or recurrence of oral cancer?15. What follow-up care is necessary after oral cancer treatment? |
Evaluation of responses
The accuracy of each response was rated using the following scores: 1: complete, 2: correct but insufficient, 3: includes correct and incorrect/outdated information, 4: completely incorrect [4]. The evaluation was performed by four experts (PDD, RMLP, ARS, and SW) who have experience in managing oral cancer patients. These experts were selected based on their specific postgraduate training in oral medicine, their authorship of numerous research articles on oral cancer, and their more than 20 years of clinical experience in the field. They were unaware of the source of the responses. The average score of all four experts to evaluate the accuracy of each response (and then the overall score) was calculated. Additionally, the concordance among the evaluators was calculated as a percentage.
Readability was assessed using the Flesch Reading Ease (FRE) and the Flesch-Kincaid Grade Level (FKGL) indices, which evaluate readability by incorporating average sentence length and syllables per word. FRE scores range from 0 to 100, with scores above 80 indicating conversational English. FKGL scores indicate the approximate USA education level needed to understand the text. The analysis used an online free-access readability scoring tool (https://readabilityformulas.com/). Ethical approval was not necessary as no human or animal subjects were involved in the study.
Statistical analysis
Each of the 4 experts rated the 15 responses provided by each LLM resulting in a total of 60 evaluations per LLM. Frequency, mean scores, and standard deviation (SD) for each question and overall were calculated. The Shapiro-Wilk test was used to determine the normality of the distribution of the continuous variables. The non-parametric Mann-Whitney U test was used to evaluate any differences between the mean scores of ChatGPT-4 and Gemini. Additionally, the Student’s t-test for independent samples was used to analyze possible differences in readability scores between ChatGPT-4 and Gemini responses. Statistical significance was set at p ≤ 0.05.
Results
ChatGPT-4 answers
The distribution of response accuracy scores for ChatGPT-4 is shown in Figure 2. Agreement rate on the scores among researchers was 71.6%. Most responses (65%) received the highest score (Score 1), while Scores 2 and 3 were given to 20% and 15% of responses, respectively. No response was rated as completely incorrect (Score 4). These results indicate predominantly positive evaluations of the responses by this LLM, suggesting that although generally accurate and relevant, there is still room for improvement in detail and information currency.
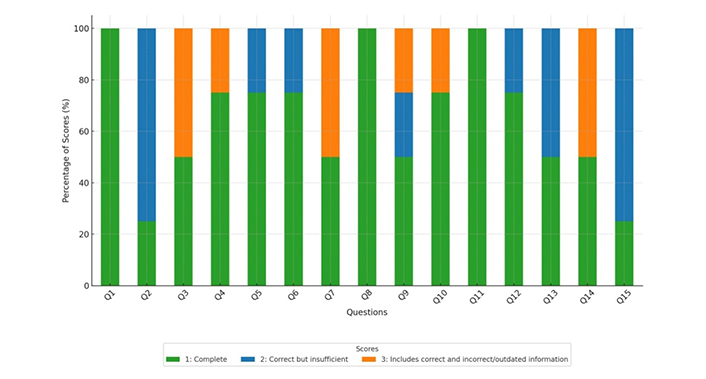
Distribution of scores assigned by the four evaluators for each response provided by ChatGPT-4
The mean score of responses obtained via ChatGPT-4 was 1.50 (SD 0.36), indicating that on average, ChatGPT-4 responses are correct but, on several counts, insufficient (Figure 3). Most responses (65%) received a score of 1, 20% received a score of 2, and 15% received a score of 3, with none receiving a score of 4. The scores suggest that while some responses are complete, there are areas where the provided information could be improved in accuracy and comprehensiveness.
Gemini answers
The distribution of response accuracy scores for Gemini is shown in Figure 4. The mean score of responses obtained via Gemini was 1.20 (SD 0.27). Agreement rate among evaluators was 83.3%. Most responses (80%) received the highest score (Score 1), while Score 2 was assigned to the remaining 20% of responses. No Scores 3 or 4 were assigned. These results indicate a high level of satisfaction with Gemini’s responses, with no reports of incorrect information.
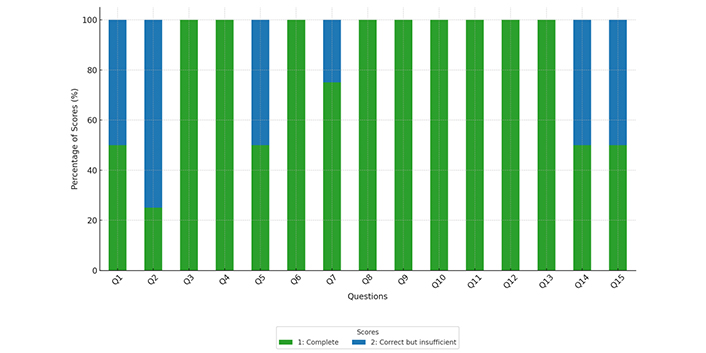
Distribution of scores assigned by the four evaluators for each response provided by Gemini
The overall mean score of 1.2 (SD 0.27), indicates that Gemini responses are generally more complete than insufficient (Figure 5). Specifically, 11 of the 15 questions received a mean score of 1.00.
Gemini appears to provide more complete and accurate responses compared to ChatGPT-4 (p = 0.02).
Readability scores
Although FRE scores differed with ChatGPT averaging 46.8 and Gemini 42.2, this analysis confirms that ChatGPT responses were generally easier to understand than those provided by Gemini, but this difference was not statistically significant (p = 0.200). Statistical analysis showed significant differences in FKGL scores between the two LLMs (p = 0.004), indicating that ChatGPT generally produces content at a lower grade level (average FKGL: 10.3) compared to Gemini (average FKGL: 12.3). This suggests that ChatGPT content is more accessible to a broader audience.
Discussion
The internet has transformed how patients access medical information, reconfiguring the dynamics of patient empowerment and communication with physicians. However, while free access to medical knowledge can be helpful in certain circumstances, not all online sources are reliable, and the general public and patients may encounter incorrect or misleading information leading to a state of confusion or self-misdiagnosis [5].
Cancer patients face numerous challenges throughout their cancer journey, ranging from emotional stress, functional disability, and treatment-related side effects, and would engage in assimilating complex medical information [6]. Patient education and support are crucial components of comprehensive oral cancer care, establishing a solid foundation for patients to actively participate in their treatment plans and face the associated challenges [7].
Many patients report that the information provided by healthcare professionals is not always clear and that at times they feel uncertain about asking questions from their healthcare provider. As a result, they often turn to the Internet to search for health-related information [8].
One of the most promising applications of AI in medicine is the development of conversational agents (chatbots) that provide information and support to the general public and patients to manage their health conditions [9]. Johnson et al. (2023) [10] found that ChatGPT provides accurate information about common cancer myths and misconceptions similar to that provided on the National Cancer Institute (NCI) webpage.
Considering the complex nature of oral cancer, patient education and awareness are paramount. However, online information about oral cancer was shown to be of poor quality [11] and difficult to read even before the advent of ChatGPT [12]. Alcaide-Raya et al. (2010) [13] found that information on oral premalignant disorders could at times be confusing to lay persons as many web pages appeared to be designed primarily for the healthcare professional rather than for the general public. Hassona et al. (2024) [14] evaluated ChatGPT’s effectiveness in providing information on the early detection of oral cancer using 108 questions from expert sources. ChatGPT responses were rated for quality, reliability, readability, and usefulness, with most responses considered very useful and scoring high in quality and reliability. However, there were concerns about readability and actionable information, indicating that while ChatGPT is a promising resource for informing patients, it still needs improvements to be fully resourceful in this area [14]. Providing comprehensive education before, during, and after cancer treatment has been demonstrated to enhance quality of life, decrease the frequency of emergency department visits, and minimize hospital admissions [15].
In a recent review of generative AI applications in healthcare, Moulaei et al. (2024) [2] found that ChatGPT and Google Bard (Gemini) were the most used LLMs. The study identified 24 different applications of generative AI in healthcare, with the most common being providing information about health conditions through question responses and disease diagnosis and prediction [2].
Giannakopoulos et al. (2023) [16] compared responses from four LLMs (Bard Google LLC, ChatGPT-3.5, ChatGPT-4 OpenAI, and Bing Chat Microsoft Corp) to clinically relevant questions in dentistry. While ChatGPT-4 statistically outperformed ChatGPT-3.5, Bing Chat, and Bard, all models occasionally exhibited inaccuracies, generalities, outdated content, and a lack of source references. Evaluators noted instances where LLMs provided irrelevant information, vague responses, or information that was not entirely accurate [16].
In evaluating the accuracy of LLMs in answering questions about oral cancer, it is crucial to recognize the differences in their design and training focus. ChatGPT excels in generating coherent and contextually relevant responses based on vast textual data, making it effective in providing information on well-established medical knowledge [17]. In contrast, Gemini (previously Google Bard) might synthesize information across modalities, such as integrating textual and visual data, which could offer more nuanced or interdisciplinary insights; moreover, Gemini benefits from accessing the most current data, offering users more timely relevant information [18]. However, both models’ accuracy in the domain of oral cancer is fundamentally dependent on the quality and scope of the data they were trained on, and ongoing validation against clinical guidelines and expert consensus remains essential for reliable application in healthcare contexts.
The challenge in the readability of chatbot responses may hinder their accessibility and ease of use by the general public. Although the readability scores suggested that ChatGPT’s responses were generally easier to understand than those provided by Gemini, on the FRE scale, ChatGPT’s content falls into the “fairly difficult” range, typically suited for readers at a 10th to 12th-grade level, while Gemini’s content, being slightly more difficult, aligns with a similar range but closer to a more challenging level. On the other hand, FKGL scores revealed a significant difference between the two models. ChatGPT generally produces content at a lower grade level, making it more accessible to high school students, while Gemini’s content is written at a higher grade level, more appropriate for early college students. This indicates that ChatGPT’s content is more accessible to a broader audience, whereas Gemini’s content requires a higher level of reading proficiency.
To date, there is a limited body of research comparing the readability of responses generated by both models within the field of dentistry. De Souza et al. (2024) [19] assessed the performance of several LLMs including ChatGPT-3.5, ChatGPT-4, and Google Bard (Gemini) in answering 10 questions covering a wide range of topics relevant to head and neck cancers, sourced from the NCI. The results showed that Google Bard (Gemini) aligned more closely with the NCI’s readability standards, suggesting its effectiveness in delivering detailed yet accessible information [19].
On the other hand, Dursun and Bilici Geçer (2024) [20] evaluated the readability of responses generated by ChatGPT-3.5, ChatGPT-4, Gemini, and Copilot concerning orthodontic clear aligners. Their results indicate that Gemini’s responses are notably more readable compared to those of the other chatbots [20]. This discrepancy highlights that the readability of AI-generated content may vary depending on the specific context and subject matter, suggesting that further research is needed to fully understand the factors influencing these differences in readability across different applications.
In the context of patient-centered care, which emphasizes the significance of a well-informed patient actively engaging in decision-making, the provision of effective information is crucial. An important factor to consider is to ensure that it is comprehensible to the intended population [21]. This is particularly relevant in the context of oral cancer, once social determinants of health, such as low socioeconomic status, including low educational attainment, are strongly associated with a higher risk and diagnostic delay [22–25]. García-Valencia et al. (2024) [26] highlight the substantial potential of advanced AI models, such as ChatGPT-3.5 and 4, in bridging language gaps within the healthcare sector. By delivering high-quality translations that ensure both accuracy and cultural sensitivity, these tools can significantly improve the accessibility of medical information, particularly for underserved, non-English-speaking communities [26].
Differences in the content responses and readability between the two models may be attributable to the distinct algorithms employed by different LLM chatbots [27]. However, comprehensive information regarding the dataset and software methods utilized for both models has not been disclosed in detail, owing to trade secrets and confidentiality concerns. Consequently, there is insufficient data to clarify the differences between these two AI models. To enhance transparency and foster a better understanding of AI, it is crucial for leading developers to share more information on technical aspects [28].
In recent years, the potential applications of LLMs in medicine, and more specifically in dentistry, have generated significant interest within the scientific community. However, their implementation in real-world settings remains limited [29]. LLM models could help patients understand their oral cancer diagnoses, treatment options, and self-care by providing easy-to-understand information, leading to more informed interactions with healthcare providers [30, 31].
One of the limitations of ChatGPT-4 and Gemini is their reliance on internet-based data, which could potentially lead to inaccuracies if the information sources are outdated or biased. To mitigate this issue, it is essential that these models undergo continuous updates using validated medical sources, with regular oversight from healthcare professionals to ensure the accuracy and reliability of the information provided. The LLMs provide general, but not personalized, medical guidance. They should be considered complementary tools and should not replace the information given to patients by medical professionals [32].
On the other hand, these models can assist healthcare providers to identify early signs of oral cancer and to make decisions in primary care settings, especially when professionals with expertise in oral medicine are not available. Indeed, LLM models can be used for triage by assessing symptom severity, helping prioritize urgent cases, and recommending appropriate actions, which is particularly useful for optimizing healthcare resources [33, 34].
From the perspective of healthcare professionals, AI systems can serve as a valuable complement and support, alleviating the workload on healthcare staff [35]. By leveraging these advanced technologies, healthcare providers can enhance the quality of patient care while simultaneously minimizing the need for unnecessary in-person interactions [36].
Limitations
A limitation of this study is that only questions and answers provided in English language were evaluated. Considering that oral cancer is more prevalent in Asian-Pacific countries the responses may not suit these non-English-speaking countries [37, 38]. Additionally, the study was based on a relatively small set of 15 oral cancer-related questions, which may not fully capture the range of possible responses and scenarios that these models could encounter. While this was sufficient for a preliminary evaluation, we acknowledge that a larger set of questions would provide a more comprehensive assessment. Future studies should consider expanding the number of questions and including multiple languages to enhance the robustness and generalizability of the findings. As this was a cross-sectional study that evaluated specific versions of two LLMs our analysis of the reliability and validity of the results was limited.
A strength of the study is that, unlike previous studies, it compared responses from two of the most used LLMs in scientific literature, with responses evaluated by four different experts in oral cancer and the evaluation of medical data on the internet. It should be noted that no universally accepted reference standards or quality criteria exist for this purpose.
Conclusions
Although Gemini showed slightly superior performance in answering questions related to oral cancer, its responses were less readable. This suggests that its texts are less accessible to lay persons, which is crucial as content accessibility can significantly influence the understanding and usefulness of the information provided. LLMs are a new resource with undeniable potential that will eventually be implemented in clinical practice. This opportunity may require professionals to reconsider the doctor-patient relationship, the quality and accuracy of advice provided, and even the decision-making process.
Abbreviations
AI: | artificial intelligence |
FKGL: | Flesch-Kincaid Grade Level |
FRE: | Flesch Reading Ease |
LLMs: | large language models |
NCI: | National Cancer Institute |
SD: | standard deviation |
Declarations
Acknowledgments
Declaration of generative AI and AI-assisted technologies in the writing process: During the preparation of this work the author(s) used ChatGPT in order to improve readability and language. After using this tool/service, the authors reviewed and edited the content as needed and took full responsibility for the content of the publication.
Author contributions
MDF: Conceptualization, Investigation, Formal analysis, Writing—original draft, Writing—review & editing. RMLP, ARSS, and SW: Investigation, Writing—review & editing. PDD: Conceptualization, Investigation, Formal analysis, Writing—review & editing. All authors read and approved the submitted version.
Conflicts of interest
Saman Warnakulasuriya who is the Guest Editor of Exploration of Digital Health Technologies had no involvement in the decision-making or the review process of this manuscript.
Ethical approval
Not applicable.
Consent to participate
Not applicable.
Consent to publication
Not applicable.
Availability of data and materials
The data are available upon request to the corresponding author.
Funding
Not applicable.
Copyright
© The Author(s) 2024.