Abstract
Aim:
This study aimed to computationally identify and optimize 4-hydroxy isoleucine (4HILe) derivatives from fenugreek as multitarget antidiabetic agents against α-glucosidase, α-amylase, and aldose reductase [PDB (protein data bank) IDs: 5NN8, 4GQR, 4QX4].
Methods:
A multi-step computational workflow was employed to identify and optimize 4HILe derivatives as antidiabetic agents. Molecular docking using the Schrödinger Suite screened 23 ligands against three enzyme targets to evaluate binding affinities and interactions. Molecular dynamic (MD) simulations conducted with GROMACS (Groningen machine chemical simulations) over 100 ns assessed conformational stability through RMSD (root mean square deviation) and RMSF (root mean square fluctuation) analysis. Binding free energy calculations [MM-GBSA (molecular mechanics-generalized Born surface area)] and free energy landscape (FEL) studies are performed to validate the thermodynamics of protein-ligand interactions. Additionally, generative AI modeling using LigDream generated 100 novel compounds derived from 4HILe, subsequently validated through docking studies to identify promising inhibitors.
Results:
The study identified 4HILe-4, 2R-3S-4R-4HILe, and 4HILe-Amide-2 as potent derivatives with superior binding affinities [ΔG (Gibbs free energy): −49.3 to −42.3 kcal/mol] compared to co-crystal ligands (−45.3 kcal/mol), as determined by docking and MM-GBSA calculations. MD revealed stable protein-ligand complexes, evidenced by low RMSD values (0.2–0.4 nm) and minimal residue fluctuations (RMSF), confirming their structural integrity. The generative AI approach using LigDream also generated 100 novel 4HILe derivatives, with top candidates exhibiting strong docking scores and key molecular interactions against α-glucosidase, α-amylase, and aldose reductase. Notably, compound 10 (−9.424 kcal/mol), compound 4 (−8.167 kcal/mol), and compound 28 (−13.760 kcal/mol) emerged as promising inhibitors for further investigation.
Conclusions:
The study highlights 4HILe derivatives as promising inhibitors for diabetes-associated enzymes, demonstrating robust binding and dynamic stability. Integrating molecular dynamics, free energy calculations, and AI-driven generative modeling provides a strong framework for accelerating antidiabetic drug discovery. These findings pave the way for experimental validation and the development of next-generation therapeutics targeting insulin resistance and hyperglycemia.
Keywords
Diabetes, α-glucosidase, α-amylase, aldose reductase, inhibitors, docking, molecular dynamics, binding free energyIntroduction
Diabetes mellitus (DM) is a chronic metabolic disease marked by elevated blood glucose levels, leading to severe complications affecting the eyes, heart, and renal system [1–4]. There are four distinct types of diabetes, with type 1 (insulin-dependent) and type 2 (non-insulin-dependent) being the most common [5, 6]. Type 1 DM (T1DM) is characterized by a complete absence of insulin secretion, necessitating external insulin administration to regulate blood glucose levels [7]. On the other hand, type 2 DM (T2DM) arises from insulin response dysfunction due to impaired insulin secretion or reduced sensitivity of insulin receptors [8].
T2DM rapidly increases worldwide, accounting for approximately 90% of diabetes cases [9]. Factors such as obesity, physical activity, genetic predisposition, and chronic stress significantly contribute to insulin resistance and disrupted glucose metabolism [10]. The latest projections estimate that by 2030, approximately 643 million people aged 20 to 79 will have diabetes, a sharp increase from the current 537 million cases [11]. Over the past 15 years, nearly $966 billion has been spent on diabetes-related expenditures [12]. Despite numerous therapeutic options, many patients struggle to maintain optimal blood glucose levels [13].
Efforts to manage T2DM have focused on pharmacological and non-pharmacological interventions [14]. Pharmacological treatments include oral agents such as sulfonylureas, biguanides, meglitinides, thiazolidinediones, and α-glucosidase inhibitors, which are crucial in controlling blood glucose levels [15]. Non-pharmacological interventions, such as dietary modifications, regular physical activity, and weight management, contribute to improving glucose regulation without medication [16]. These strategies help to prevent diabetes-related complications like retinopathy, neuropathy, and nephropathy [17]. Additionally, enzyme inhibition approaches targeting α-glucosidase, α-amylase, and aldose reductase have been explored for their potential in controlling hypoglycaemia and diabetes complications [18].
Although synthetic drugs have been extensively studied, many have been excluded from clinical trials due to poor pharmacokinetics and severe side effects, such as weight gain, chronic tissue damage, and limited efficacy [19]. This has led to a paradigm shift toward exploring medicinal plants as a source of antidiabetic agents. Natural products and their derivatives have demonstrated therapeutic potential due to their diverse structural properties [20].
One such natural compound, 4-hydroxy isoleucine (4HILe), has gained significant attention for its insulinotropic, anti-dyslipidemic, and hyperglycemic properties [21, 22]. First identified in fenugreek seeds, 4HILe has shown promising effects in glucose metabolism and diabetes management [23, 24]. The pathophysiology of DM has been linked to key enzymes, including α-glucosidase, α-amylase, and aldose reductase [25, 26]. α-glucosidase is a carbohydrate hydrolyzing enzyme in the small intestine that catalyzes the final step of carbohydrate digestion by breaking down disaccharides into glucose [27, 28]. Excessive α-glucosidase activity contributes to postprandial hyperglycemia, a hallmark of T2DM [29]. Inhibition of α-glucosidase slows carbohydrate digestion, reducing glucose absorption and lowering postprandial blood sugar levels [30].
Similarly, α-amylase is a calcium-dependent metalloenzyme responsible for breaking down polysaccharides like starch into oligosaccharides and maltose, which are further hydrolyzed into glucose [31]. Hyperactivity of α-amylase can exacerbate postprandial glucose spikes. Inhibiting α-amylase slows starch digestion, thereby controlling postprandial hyperglycemia [32]. Aldose reductase is a key enzyme in the polyol pathway, converting excess glucose into sorbitol using NADPH as a cofactor. Under hyperglycemic conditions, this pathway becomes overactivated, leading to sorbitol accumulation, increased osmotic stress, and oxidative damage, contributing to complications like retinopathy, nephropathy, and neuropathy [33, 34]. Inhibiting aldose reductase prevents the harmful buildup of sorbitol and reactive oxygen species, reducing diabetes-associated microvascular complications [35].
While α-glucosidase and α-amylase inhibitors primarily control postprandial glucose levels by reducing carbohydrate digestion and absorption, aldose reductase inhibitors mitigate oxidative stress and tissue damage, addressing long-term diabetic complications. Studies have shown that 4HILe and its derivatives encourage glucose absorption by skeletal muscle cells in a dose-dependent manner [24]. Regarding enzyme inhibition, 4HILe has demonstrated non-insulin-dependent antidiabetic effects by inhibiting α-glucosidase and α-amylase [36]. Additionally, it has shown aldose reductase inhibitory activity, further supporting its potential as an antidiabetic agent [37, 38].
The current study aims to identify potent and high-affinity inhibitors for DM through molecular docking investigations. Furthermore, molecular dynamics (MD) simulations, molecular mechanics-generalized Born surface area (MM-GBSA), principal component analysis (PCA), and FEL (free energy landscape) studies have been employed to gain deeper insights into protein-ligand interactions. This computational approach enables the examination of molecular mechanisms at an atomic level, allowing for precise calculations of conformational changes and dynamic characteristics. Ultimately, this research seeks to discover novel and effective inhibitors for diabetes treatment.
Materials and methods
System configuration
The computational studies presented in this study were conducted using the Glide module of Schrödinger Maestro 2022 and GROMACS (Groningen machine chemical simulations) 2022.3 suite, a Linux-based workstation, both installed on a Dell Linux Enterprise version 8.0 system equipped with an i7 processor and 64 GB of RAM (random access memory) [39–41].
Protein and ligand preparation
The 3D structure of the human form α-glucosidase, aldose reductase, and α-amylase was retrieved from the protein data bank (PDB) (https://www.rcsb.org; accessed on 26th June 2023) with the PDB ID of 5NN8, 4QX4, 4GQR (Figure 1) and with a resolution of 2.45, 1.26, 1.20 Å respectively. The protein preparation was carried out with the help of a Protein Preparation Wizard (Maestro software 2023-1, Schrӧdinger, Inc., LLC, New York, USA) in the Schrӧdinger suite (www.schrodinger.com). The missing side chains and loops in the protein structure were filled with the help of Prime (v12.3, Schrödinger, Inc., LLC, New York, USA). The crystallographic water molecules and other interfering ligands were deleted, hydrogen molecules were included, and formal charges and bond orders were assigned during the protein preparation step. All other unwanted residues were removed. The protein structure was energy minimized and then was used in the various calculations. Ligand preparation was performed using the 23 molecules through the Ligprep module (Maestro software 2023-1, Schrӧdinger, Inc., LLC, New York, USA). The purpose of this process was to enhance optimization, turn 2D structures into 3D ring conformers, and establish ionization and tautomer states while being influenced by the OPLS (optimized potentials for liquid simulations)-2005 force field at a pH range of 7 ± 2 [42].
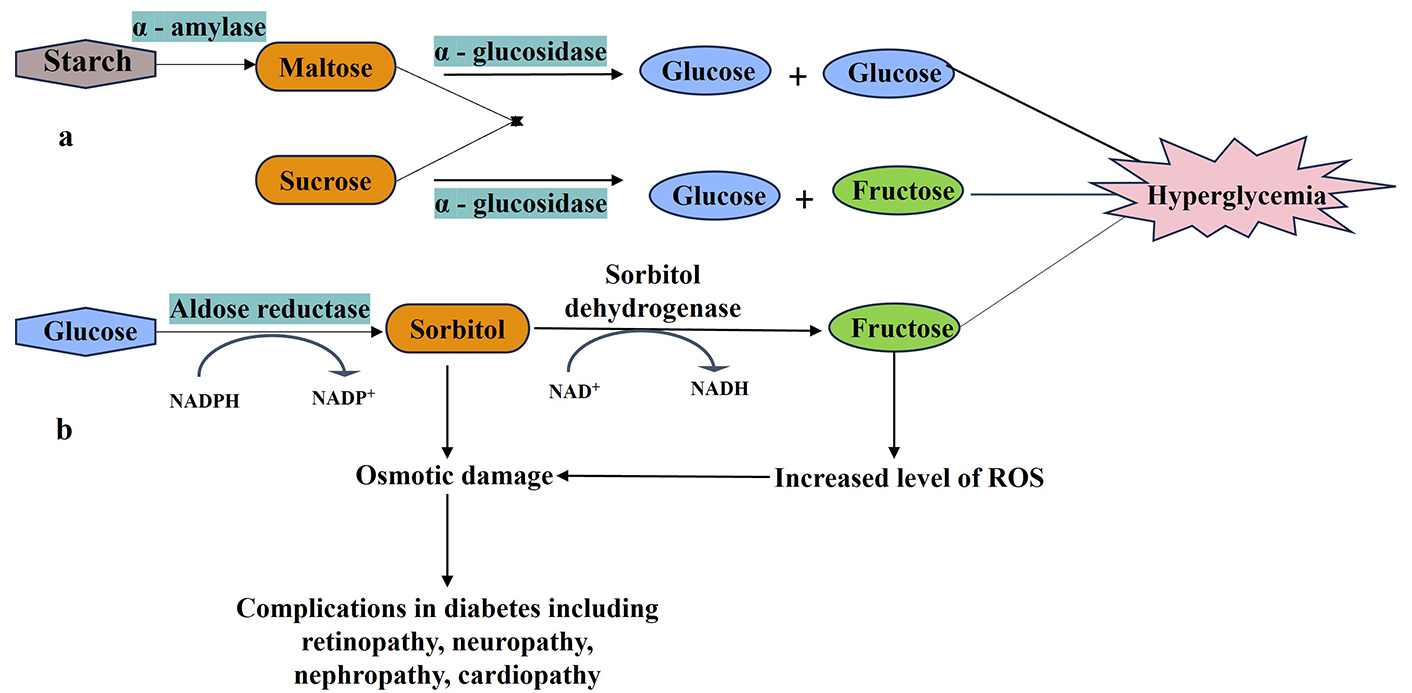
Roles of α-amylase, α-glucosidase, and aldose reductase in diabetic complications. a: α-amylase and α-glucosidase digest dietary starch into glucose, triggering postprandial hyperglycemia; b: aldose reductase initiates the polyol pathway, converting glucose to sorbitol (later metabolized to fructose by sorbitol dehydrogenase), leading to sorbitol accumulation, NADPH depletion, and oxidative stress—key drivers of diabetic complications
Receptor grid generation and docking
We selected the centroid XYZ coordinates of the co-crystal ligand within the receptor to create the receptor grid using the Receptor Grid Generation Glide module (Maestro software 2023-1, Schrӧdinger, Inc., LLC, New York, USA). The default settings of the OPLS-2005 force field were applied, along with a van der Waals radius scaling factor of 1 Å and a partial charge cutoff within the range of 0.25 Å. Subsequently, the ligands were docked into the generated grid to validate the protein and ligand interactions. This step was performed using Glide XP (Maestro software 2023-1, Schrӧdinger, Inc., LLC, New York, USA).
Binding characterization
A 2D interaction diagram is often used to visualize and analyze the intermolecular interactions between a protein and ligand or small molecule compounds in studying binding mechanisms. The selected compounds or ligands are also represented in a simplified 2D manner, usually as molecular structures or formulas. This representation helps identify the chemical properties and features of the ligands that are important for binding. Hydrogen bonds help identify molecular recognition and binding. In the interaction diagram, hydrogen bond interactions are represented by dashed lines between the donor and acceptor atoms involved in the hydrogen bond formation. Hydrophobic interactions are nonpolar regions or clusters within the protein and ligand structures. We can identify the critical amino acid residues in the protein that form hydrogen bonding with the ligands or observe hydrophobic regions where nonpolar ligand groups may interact favorably.
Validation of a docking protocol
It is a crucial step to ensure its reliability and accuracy in predicting protein-ligand interactions. Metrics such as root mean square deviation (RMSD) and root mean square fluctuation (RMSF) can be determined to compare the similarity of predicted and experimental complexes [43]. It allows for determining the docking protocol’s ability to discriminate between ligands and correctly rank their binding affinities.
MD simulation study
MD simulation was performed to get insights into binding stability properties, protein compactness, and interactions; the top molecular complexes were subjected to simulation for 100 ns using the GROMACS 2022.3 suite (http://www.gromacs.org), a Linux-based workstation [44]. CHARMM27 (Chemistry at Harvard Macromolecular Mechanics 27) all-atom force field was applied with TIP3P (transferable intermolecular potential three point) water to the complex and generated the topology of the complex. Under periodic boundary conditions, a cubic solvation box was employed, with a width of 10 Å distance between the box’s edge and protein surface. The right amount of Na+ and Cl− ions were added to neutralize the complexes. Energy minimization was done as the initial step, ensuring that our starting structure had an appropriate geometry and solvent orientation. Before starting the actual dynamics, we must balance the solvent and ions around the protein. Two steps are commonly taken during equilibration. An NVT ensemble (fixed number of particles, volume, and temperature) performs the initial stage. Here, the system was equilibrated for 100 ps at 100 K. It is obvious that the temperature of the system quickly arrives at the desired value (300 K) and remains steady throughout the equilibration [43].
The second phase is completed in an NPT ensemble (number of particles, pressure, and temperature) that remains constant. The system was also equilibrated for 100 ps at 100 K, with an average pressure of 12.62 ± 117.2 bar; it was evident that the system’s pressure quickly arrived at the target value of 1 bar and remained steady throughout the equilibration. A density study was also done after pressure progression using energy. The typical value over 100 ps is 1,015 ± 2.3 kg m−3, not far from the experimental weight of 1,000 kg m−3.
Trajectory analysis
A system stability assessment was carried out after conducting MD simulations, and it involved different methods and criteria, which involved analyzing trajectories throughout the simulation period. The protein backbone’s RMSD was monitored during this analysis. The RMSD values acceptable for stability in MD simulations can differ depending on the system. Still, the threshold is generally around 2−3 Å, and the evaluation included assessing the RMSF of distinct residues. Furthermore, the assessment included the number of hydrogen bonds within inter-chain residues of the complex and the occupancy of hydrogen bonds.
MM-GBSA-binding free energy calculation
Docking studies aren’t always regarded as the most reliable or effective procedure. As a result, a more precise computational technique is necessary for lead hit detection. The MM-GBSA approach was used to estimate free binding energies for the best hit-docked complexes utilizing MM force fields and implicit solvation. Prime MM-GBSA is a combination of three terms: MME (molecular mechanics energy), SGB (solvation model for polar solvation), and GNP (nonpolar solvation energy) term composed of nonpolar solvent accessible surface area and van der Waals interactions. The general formula for calculating total binding free energy is expressed as [45]:
Where G = MME (molecular mechanics energy) + GSGB (generalized born solvation model for polar solvation) + GNP (nonpolar solvation energy).
Using frames recovered from MD simulation trajectories, the Prime MM-GBSA method was utilized to calculate each receptor-ligand complex’s free energy of binding. In the MD simulation step, 1,000 frames were calculated for each complex. One frame was extracted from each simulation trajectory at 1 ns intervals (i.e., 100 frames for each simulation) to compute the MM-GBSA binding free energy using in-house Schrödinger Prime scripts and the VSGB 2.0 solvation model.
FEL & PCA analysis
To understand the importance of analyzing dynamic properties and molecular system changes, it is crucial to effectively employ FEL and PCA. Our studies used PCA to identify key motion patterns and FEL analysis to visualize energy landscapes. Using both covariance matrix analysis and Gibbs FELs provides a complete framework to explore the compound’s dynamic patterns and structural energies, providing a clearer understanding of crucial molecular interactions and conformational changes that are of utmost importance in drug discovery and MD. The approach employed in the study involves extracting coordinated movements from all trajectories to construct a covariance matrix. This analysis was done using the g-cover and g-analog modules, which were used to analyze selected, carefully screened compounds and reference inhibitors. This process generated a set of eigenvectors by diagonalizing the covariance matrix. By utilizing these eigenvectors, we can acquire valuable insights into the energetic influence of each component on the molecular motions observed. We performed Gibbs FEL calculations to gain a deeper understanding of the dynamic behavior of the studied system. By utilizing the sham function in GROMACS, the predictions of the top two principal components were employed. This analysis provided a visualization and understanding of the energy landscapes that control conformational changes and interactions within the molecular systems under study [46].
AI-based generative model
In this work, we have developed a novel method for generating new molecules from 3D representations using shape-based generative modelling. The SMILES (simplified molecular input line entry system) string of 4HILe was uploaded to the server using an artificial neural network-driven platform called the LigDream module of the PlayMolecule server (https://github.com/Bibyutatsu/ligdream/tree/master), which was then used to generate 100 new SMILE strings for different compounds. LigDream incorporates autoencoders and captioning networks to variationally design multiple molecules starting from a single volumetric representation, leveraging deep learning techniques to explore molecular space. The platform performs featurization by encoding the 3D structure of the seed molecule into a latent space, which is then used to generate novel compounds while retaining the seed molecule’s structural features.
The training of the model was performed on existing molecular datasets, enabling the model to learn a diverse range of chemical patterns and molecular geometries. The ability to generate structurally diverse molecules is a key feature, as the method does not require retraining on focused libraries. This makes it highly efficient in generating compounds that exhibit tunable similarity to the seed molecule while maintaining structural diversity. Following the generation of new compounds, the molecules were subjected to docking studies against three diabetic targets: α-glucosidase, α-amylase, and aldose reductase. This demonstrated the potential of the platform to design novel compounds for drug discovery. The significance of this method lies in its ability to efficiently generate structurally similar yet diverse compounds from a single seed, offering a powerful tool for tackling common challenges in drug discovery while minimizing resource consumption [47, 48].
Results
Docking validation
Docking studies provide valuable insights into the mechanism of interactions between drugs and proteins. To confirm the accuracy, we repeated the docking of the co-crystallized inhibitor within its initial structure. Remarkably, this redocking process resulted in a low RMSD value of 0.2−0.4 Å (Figure 2). The docking outcomes were thoroughly examined, focusing on the binding conformation and interaction analysis detailed in Tables S1, S2, and S3.
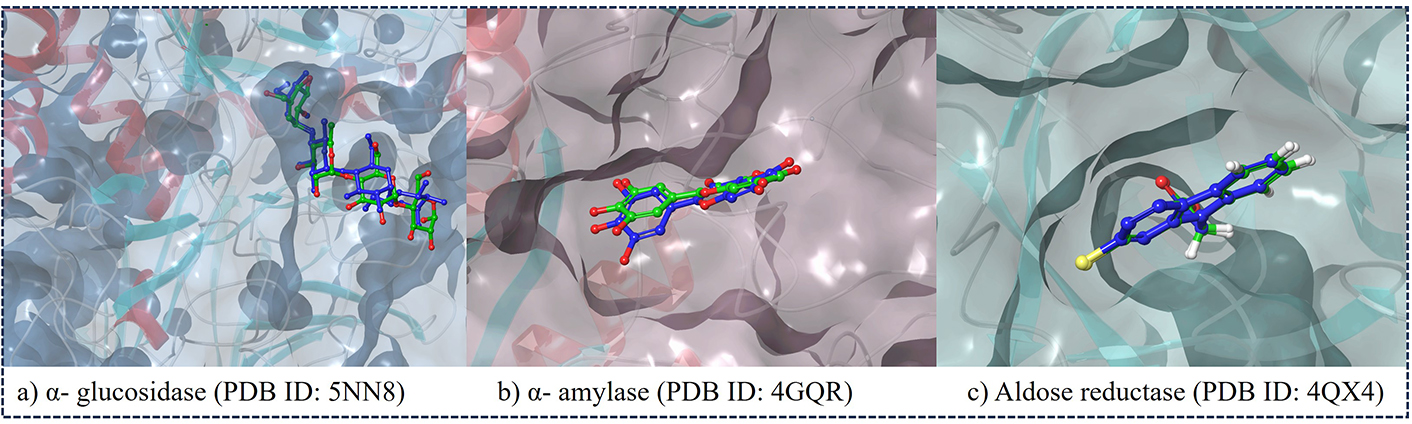
The redocked poses of the co-crystal ligands within the binding pockets of their respective target proteins. The ligands are shown in the active sites of (a) α-glucosidase (PDB ID: 5NN8), (b) α-amylase (PDB ID: 4GQR), and (c) aldose reductase (PDB ID: 4QX4). PDB: protein data bank
Docking studies
The docking studies involved diverse conformers of 4HILe and its mimetics in assessing their binding affinity with key diabetes targets. These investigations identified the top 2 ligands for each target out of a total of 23 ligands based on their docking score and interactions resembling those of the target co-crystal ligands. The chosen compounds demonstrated substantial interactions with the key active site residues; analyzing these detailed binding interactions and evaluating the therapeutic potential of the top-selected compounds can help guide further exploration and development of these promising drug candidates (Figure 3). This in-depth understanding of the compound-target interactions is crucial for assessing the viability of these compounds for continued drug development efforts.
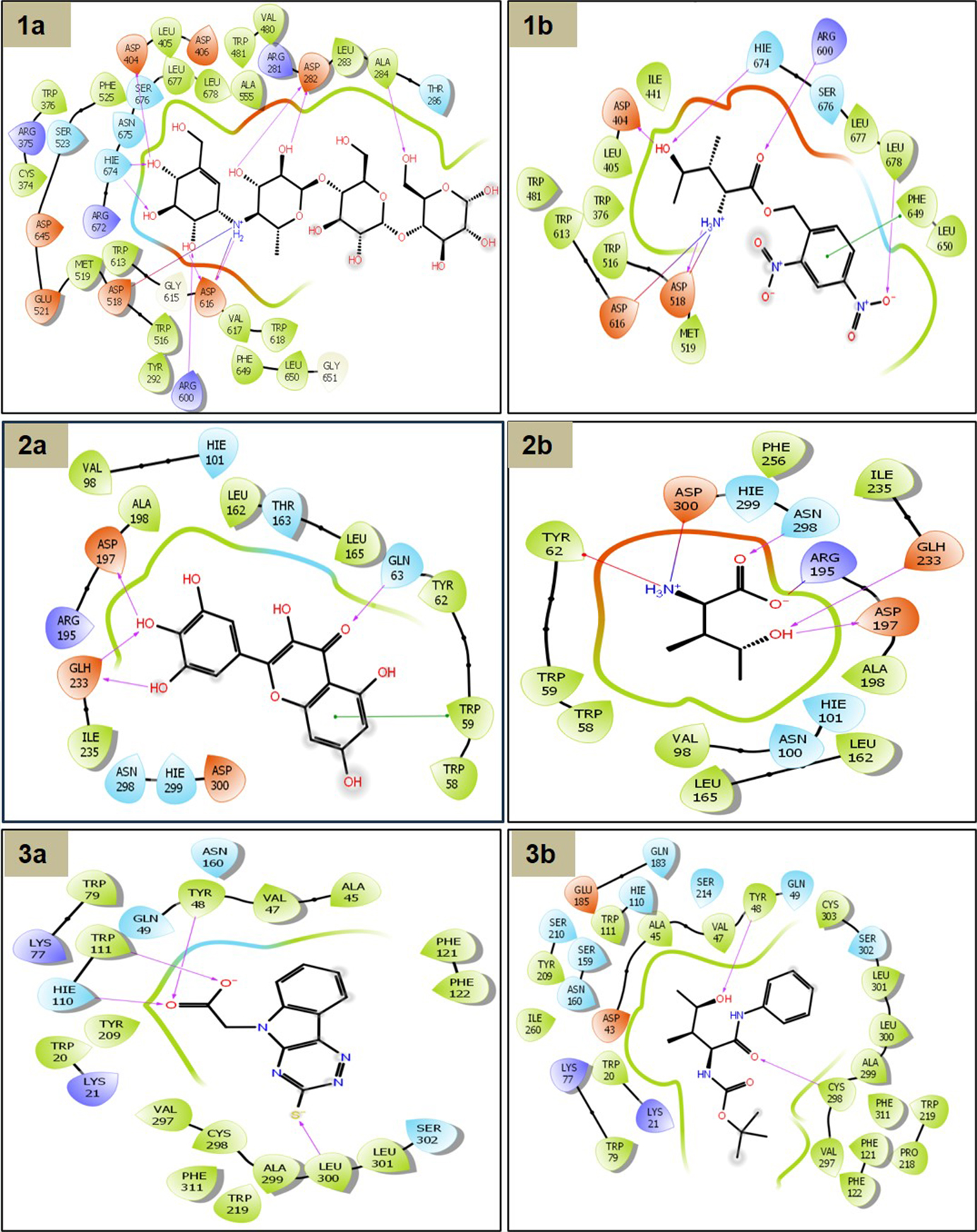
2D interaction schemes of binding poses were predicted. (1a) α-glucosidase with co-crystal ligand; (1b) 4HILe-4; (2a) α-amylase with co-crystal; (2b) 2R-3S-4R-4HILe; (3a) aldose reductase with co-crystal; (3b) 4HILe-Amide-2. The solid line with an arrow in the 2D diagram is denoted by magenta indicating the hydrogen bond, green represents pi-pi stacking, red represents the aromatic hydrogen bond. 4HILe: 4-hydroxy isoleucine
Upon docking studies, it was revealed that out of the 23 ligands used in the study (Table S2), ligand 4HILe-4 has the best docking score of −8.3 kcal/mol. As depicted in Figure 3(1b) ligand, 4HILe-4 formed multiple stabilizing interactions within the active site of α-glucosidase, including hydrogen bonds, salt bridges, and pi-pi stacking interactions as compared to wild type [Figure 3(1a)]. The pi-pi stacking is at the distance of 5.13 Å with the residue PHE649, while hydrogen bonding interactions with the residues ASP404, ASP518, ARG600, HIE674, and LEU678 within the space of 1.94, 1.65, 2.12, 2.22, and 2.54 Å, respectively. The amine group of the ligand shows pi-pi interaction at 5.13 Å with the aromatic ring of PHE649—two salt bridge formations with ASP518 and ASP616 at distances of 3.07 and 4.73 Å, respectively. By inhibiting α-glucosidase, 4HILe-4 slows the conversion of complex carbohydrates into glucose, leading to a gradual release of glucose into the bloodstream, effectively reducing postprandial hyperglycemia. These findings suggest that 4HILe-4 is a promising α-glucosidase inhibitor, demonstrating potent binding interactions that may contribute to its antidiabetic effects.
The co-crystalized ligand of the target α-amylase, myricetin as shown in Figure 3(2a) exhibits hydrogen bonding and pi-pi stacking interactions with the protein, including pi-pi stacking at distance of 3.85 Å with TRP59; hydrogen bonding interactions with GLN63, ASP197, and GLH233 within the space of 2.1, 1.5, 2.1, and 2.6 Å, respectively. 2R-3S-4R-4HILe shows additional pi-cation and salt bridge interactions compared with the co-crystal ligand. The amine group of the ligand shows pi-cation interaction at 6.3 Å with the aromatic ring of TYR62. Two salt bridge formations with ASP300 at 3.5 and 3.6 Å [Figure 3(2b)]. The amino and carboxy groups of VAL-L-4HILe form salt bridges with negative and positively charged residues ASP197 and ARG195 of 2.68 and 4.76 Å. These results highlight the potential of inhibitors like myricetin and 2R-3S-4R-4HILe to effectively suppress the hydrolysis polysaccharides like starch into maltose and oligosaccharides by breaking α-(1→4) glycosidic bonds, facilitating carbohydrate digestion and glucose absorption by regulating blood sugar levels in diabetic patients.
The co-crystal ligand of aldose reductase (4QX4) forms an essential hydrogen bonding interaction with the polar and hydrophobic residues in Figure 3(3a), including HIE110, TRP111, TYR48, LEU300, and aromatic hydrogen bond with the VAL47. The 4HILe-Amide-2 showed a better binding affinity of −7.7 kcal/mol than the co-crystal ligand, (3-thioxo-2,3-dihydro-5H-[1,2,4]triazino[5,6-b]indol-5-yl) acetic acid. The hydroxyl (-OH) group of the ligand forms hydrogen bonds with TYR48 [Figure 3(3b)]. Additionally, salt bridges are observed, particularly involving GLU185 (negatively charged) and LYS21, LYS77 (positively charged), contributing to electrostatic stability. The presence of hydrophobic residues (PHE122, PRO218, PHE311, TRP219, LEU300, VAL297, CYS298) suggests strong van der Waals interactions, further reinforcing ligand binding. The polar residues (GLN183, SER302, TYR209, HIE110) contribute additional stabilizing effects. The overall interaction pattern indicates a well-balanced binding pocket, where hydrophilic, hydrophobic, and charged residues work collectively to enhance ligand affinity and stability. These inhibitors bind to the enzyme’s active site, blocking glucose access and disrupting NADPH utilization through hydrogen bonding, hydrophobic interactions, and pi-pi stacking preventing substrate access to the active site.
Molecular dynamic analysis
The stability of interactions and conformations within physiological environments can be assessed using molecular dynamic simulations. To evaluate the structural and flexibility properties of the top protein-ligand complexes and the reference compound for each antidiabetic target, we underwent 100 ns MD simulation. The conformational and interaction stability of receptor-ligand complexes were assessed using RMSD and RMSF. Notably, complexes involving 4HILe-4 and 4HILe of target α-glucosidase (5NN8), 2R-3S-4R-4HILe and 4HILe of target α-amylase (4GQR), 4HILe-Amide-2 and 4HILe of target aldose reductase (4QX4) exhibited remarkable stability RMSD when compared to known compounds. RMSD values of all the above-mentioned complexes fell between 0.2 and 0.4 nm. RMSF analysis demonstrated minimal residue variation across all complexes (Figure 4), highlighting their constant stability.
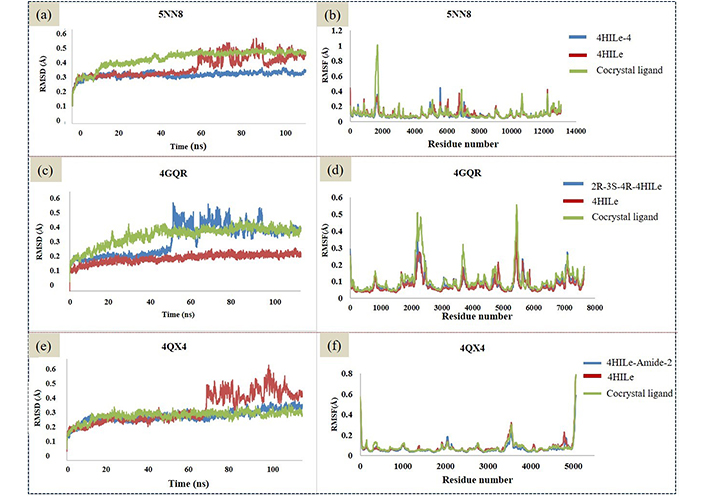
RMSD and RMSF analysis of the ligands binding to the target α-glucosidase (5NN8), α-amylase (4GQR), and aldose reductase (4QX4). (a, c, e) RMSD trajectories (in Å) for 5NN8 (a), 4GQR (c), and 4QX4 (e) over 100 ns, highlighting stable conformational equilibration; (b, d, f) corresponding structural dynamics analyses (RMSF) for 5NN8 (b), 4GQR (d), and 4QX4 (f). 4HILe: 4-hydroxy isoleucine; RMSD: root mean square deviation; RMSF: root mean square fluctuation
Hydrogen bonding analysis
The stabilization of the protein-ligand complex is greatly aided by hydrogen bonding. Figure 5 shows the number of hydrogen bonds formed in complexes during the last 100 ns trajectory. Their polar interactions greatly influence the binding affinity between the protein and ligand. The occupancy percentages for hydrogen bonds indicate how many trajectories were involved in the forming of hydrogen bonds during a simulation. In the case of the α-glucosidase ligand, 4HILe-4 shows many hydrogen bond interactions, with the specific residues ASP404, ASP518, ARG600, HIE674, and LEU678 within the space of 1.94, 1.65, 2.12, 2.22, and 2.54 Å, respectively. Hydrogen bonding interaction with the co-crystal ligand characteristic residues ASP404, ASP518, ARG600, and HIE674 within a range of 2.5 Å. The co-crystallized form of α-glucosidase contained 23 hydrogen bonds, in which the ligand shows the occupancy rate with ASP616 and ASP282 at 76.13% and 76.95%. Meanwhile, the 4HILe-4 exhibited a higher occupancy rate than the co-crystal ligand.
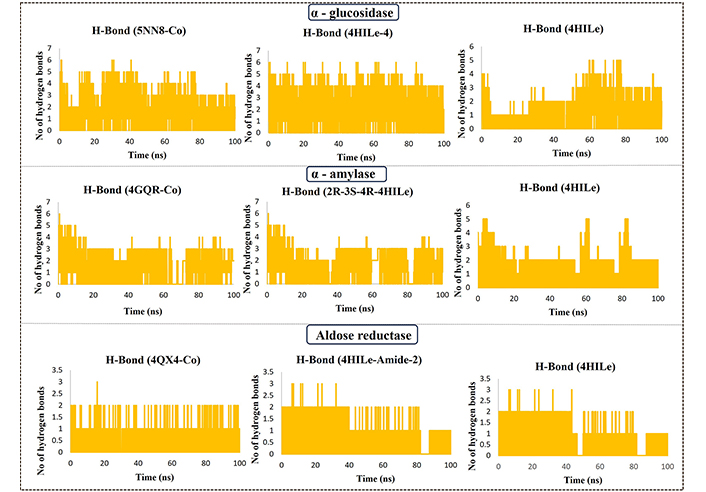
H-bond occupancy result of protein-ligand complex of the target α-glucosidase (5NN8) with co-crystal, 4HILe-4, 4HILe; α-amylase (4GQR) with co-crystal, 2R-3S-4R-4HILe, 4HILe; aldose reductase (4QX4) with co-crystal ligand, 4HILe-Amide-2, 4HILe. 4HILe: 4-hydroxy isoleucine; H-bond: hydrogen bond; No: number
The co-crystalized ligand of the target α-amylase shows hydrogen bonding interactions with the residues GLN63, ASP197, and GLH233 within the space of 2.1, 1.5, 2.1, and 2.6 Å, respectively. 2R-3S-4R-4HILe shows hydrogen bonding interaction with characteristic residues ASP197, ARG195, GLH233, and ASN298 within a range of 2 Å. VAL-L-4HILe forms hydrogen bonding interaction at 1.7, 1.7, 1.8, 1.9, 1.7 Å with characteristic residues such as ASP197, ARG195, GLH233, HIE299, and ASP300. The co-crystallized form of α-amylase contained 32 hydrogen bonds, in which the ligand shows the highest occupancy rate with the residue ASP300 showed 26.94%. At the same time, the 2R-3S-4R-4HILe was found to show a higher occupancy rate with the ASP300 with 30.77% compared with the co-crystal ligand. The co-crystal ligand of aldose reductase forms an essential hydrogen bonding interaction with the polar and hydrophobic residues HIE110, TRP111, TYR48, LEU300, and aromatic hydrogen bonds with the VAL47. A significant number of hydrogen bonds with various target protein-ligand complexes indicates that these are suitable inhibitors. It is possible that these residues could have a substantial impact on the interaction between the protein-ligand complex. Specific residues involved in molecular interactions can be identified by analyzing hydrogen bond interactions.
MM-GBSA
The post-molecular docking analysis, which is essential for determining the stability of receptor-ligand complexes, was computed using the Prime MM-GBSA approach. Recent studies have shown that docking score findings can be consistently verified using the computation of binding free energy. The calculations for the energy required to bind are expressed as ΔG (Gibbs free energy). In mathematical terms, a stable protein-ligand complex is characterized by negative ΔG values. The MM-GBSA graph (Figure 6) presents the binding free energy values of various compounds against three enzymes: α-glucosidase (5NN8), α-amylase (4GQR), and aldose reductase (4QX4). Lower binding free energy values indicate stronger binding and better inhibition potential of the compound. From the stereoisomeric forms, the 2R-3R-4R-4HILe variant exhibits the most favourable binding energy (−46.2 kcal/mol, −45.6 kcal/mol, −48.50 kcal/mol), suggesting it has the highest binding energy among all stereoisomers. Other forms, such as 2R-3S-4S-4HILe and 2R-3S-4R-4HILe, also show strong interactions but are slightly less effective than the 2R-3R-4R form. In contrast, 2S-3S-4R-4HILe, 2S-3S-4S-4HILe, and 2S-3R-4R-4HILe display higher binding energy values (less negative), indicating weaker binding energy. Other promising compounds include 4HILe-Amide-2 and 4HILe-4, which showed the strongest binding affinities of −42.3 kcal/mol and −49.3 kcal/mol for α-glucosidase, −43.8 kcal/mol and −46.6 kcal/mol for α-amylase, and −47.2 kcal/mol and −46.9 kcal/mol for aldose reductase, respectively. These values indicate that these compounds have comparable or superior inhibitory potential relative to the co-crystal (Figure 6). Overall, 2R-3R-4R-4HILe, 4HILe-4, and 4HILe-Amide-2 emerge as the most potent inhibitors across all three enzymes, consistently outperforming the co-crystal and other compounds.
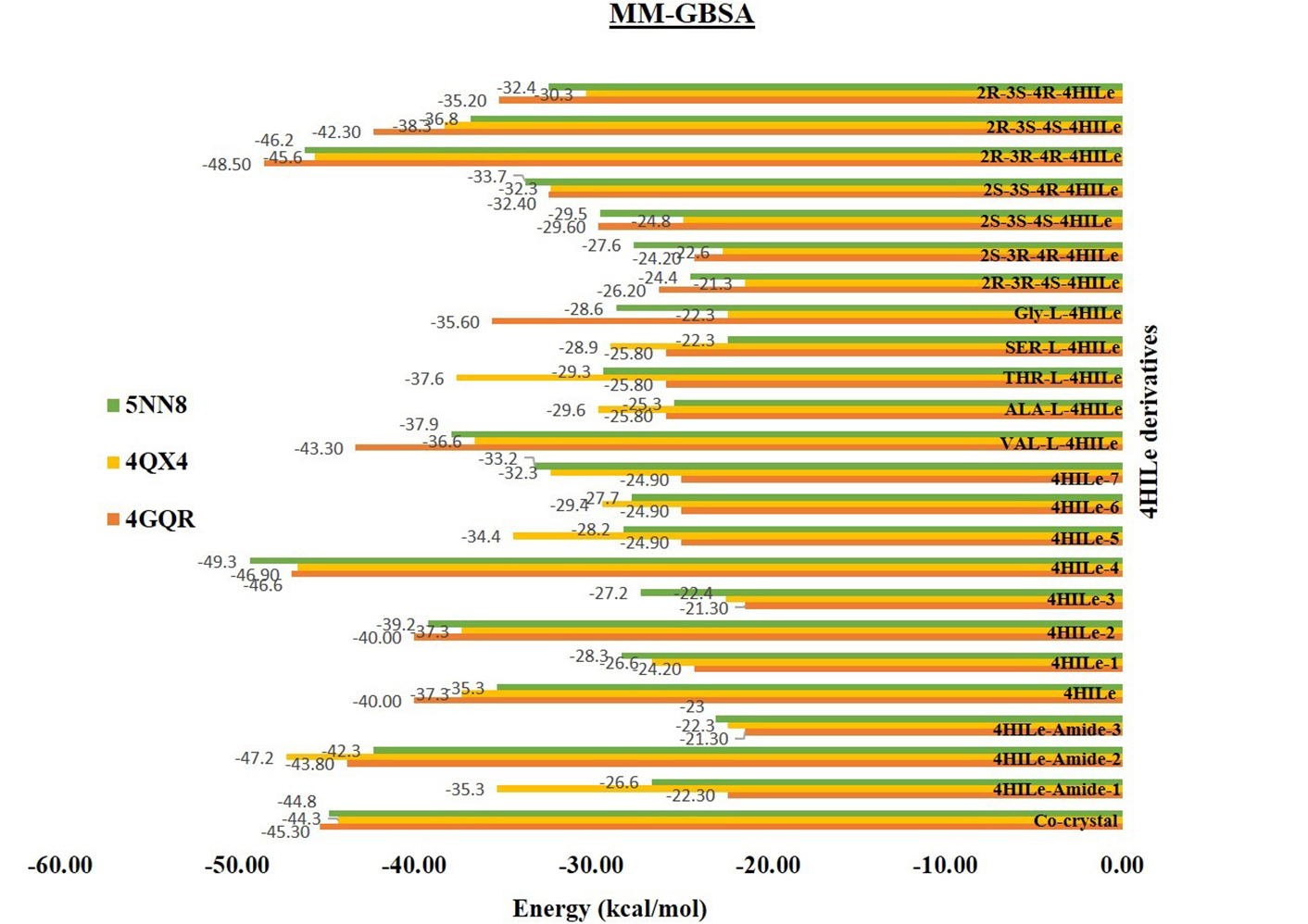
Binding free energy calculation with the derivatives of the 4HILe against three targets α-glucosidase (5NN8), α-amylase (4GQR), and aldose reductase (4QX4). 4HILe: 4-hydroxy isoleucine; MM-GBSA: molecular mechanics-generalized Born surface area
FEL
Gmx Covar, Gmx Anaeig, and Gmx Sham were utilized to calculate the Gibbs FEL, using projections from their own first (PC1) and second (PC2) eigenvectors. In Figure 7, the Gibbs FEL is shown in a color-coded manner. Trajectories are used to analyze the direction of fluctuation for all C atoms in complex structures investigated by the Gibbs FEL through trajectories. A deeper blue indicates lower energy, which corresponds to the free energy contour map. After binding these compounds, the primary free energy in the global free energy region was found to have undergone a complete change. These free energies strongly indicate these molecules’ stable conformational states. Different global minima of various targets are observed during the 100 ns of MD simulations due to the binding of ligands to the respective targets. A small energy barrier separates the metastable conformational states from several distinguishable minima on the energy landscape. The most metastable conformational states were seen in the binding in the case of (α-glucosidase) 5NN8 with 4HILe and 5NN8 with 4HILe-4, (α-amylase) 4GQR with 4HILe and 4GQR with 2R-3S-4R-4HILe, (aldose reductase) 4QX4 with 4HILe and 4QX4 with 4HILe-Amide-2 in which local minima were distributed to about three to four regions within the energy landscape. The complexes mentioned above formed two to three metastable conformations during the whole trajectories.
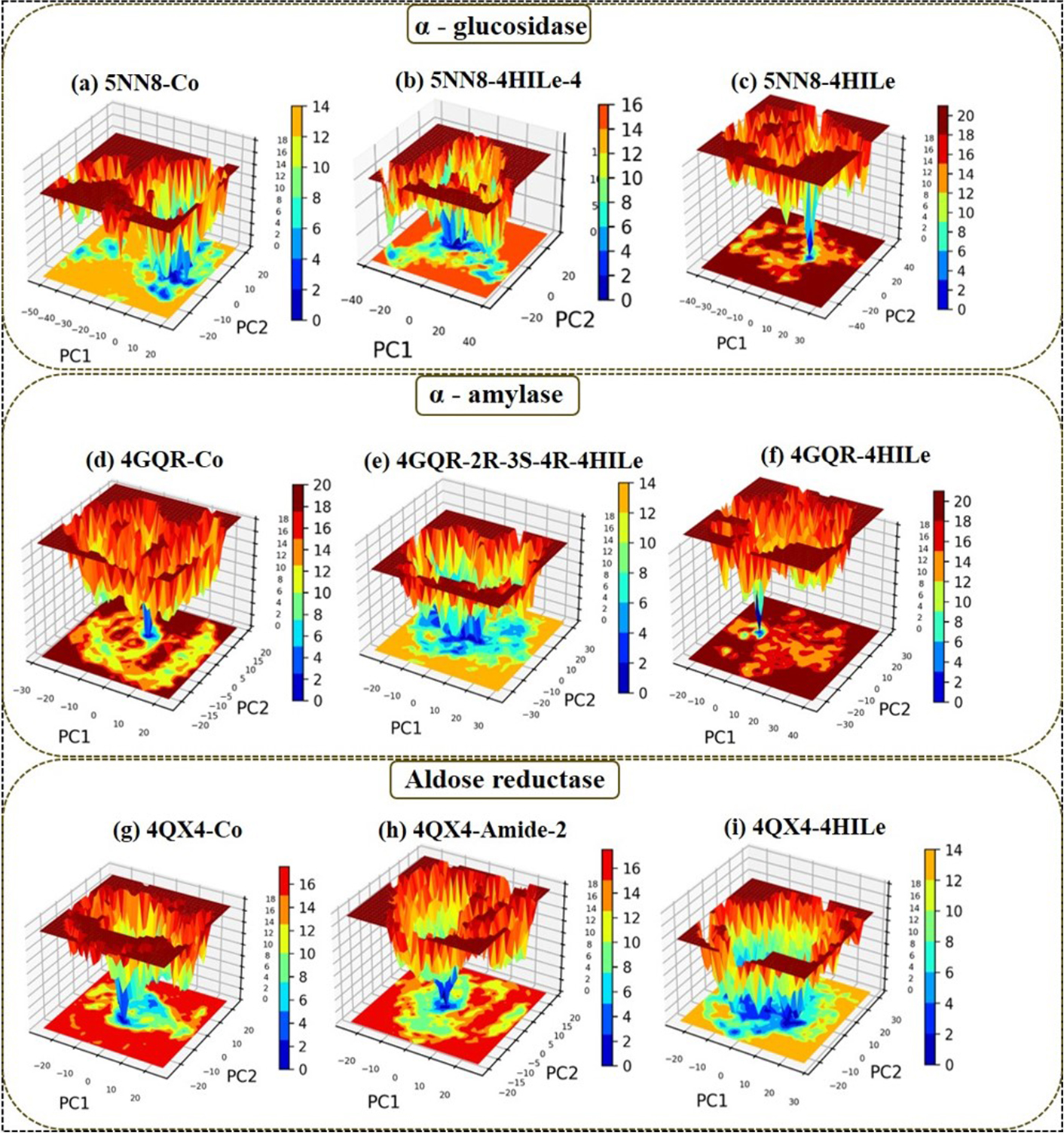
The Gibbs free energy landscape with the derivatives of the 4HILe against three targets. α-glucosidase (5NN8): (a) co-crystal, (b) 4HILe-4, (c) 4HILe; α-amylase (4GQR): (d) co-crystal, (e) 2R-3S-4R-4HILe, (f) 4HILe; and aldose reductase (4QX4): (g) co-crystal, (h) 4HILe-Amide-2, (i) 4HILe. 4HILe: 4-hydroxy isoleucine; PC1: principal component 1; PC2: principal component 2
Shape-based generative modeling and docking for diabetic enzyme inhibition
The computational workflow successfully generated 100 novel compounds from the SMILES string of 4HILe using the LigDream module on the PlayMolecule server. After checking for structural similarity and removing duplicates (Table S4), the final set of compounds designed to retain similarity to the seed molecule was obtained and subjected to docking studies against three diabetic targets: α-glucosidase, α-amylase, and aldose reductase. The top four compounds for each target were identified based on docking scores and interaction profiles (Figure 8). For α-glucosidase, compound 10 achieved the best docking score of −9.424 kcal/mol, forming hydrogen bonds and a salt bridge with ASP616, along with hydrophobic interactions involving PHE649, TRP516, MET519, LEU677, and LEU678. Compound 47, with a score of −8.870 kcal/mol, formed hydrogen bonds with ARG600, ASP518, ASP404, and HIE674 and a salt bridge with ASP518. Compound 11 (score: −7.262 kcal/mol) interacted via hydrogen bonds with ASP404, LEU677, and LEU678 and hydrophobic interactions with SER523 and PHE525, among others. Compound 13 (score: −7.070 kcal/mol) displayed hydrogen bonds with ASP282, ASP518, and ARG600 and a salt bridge with ASP518 and ASP616.
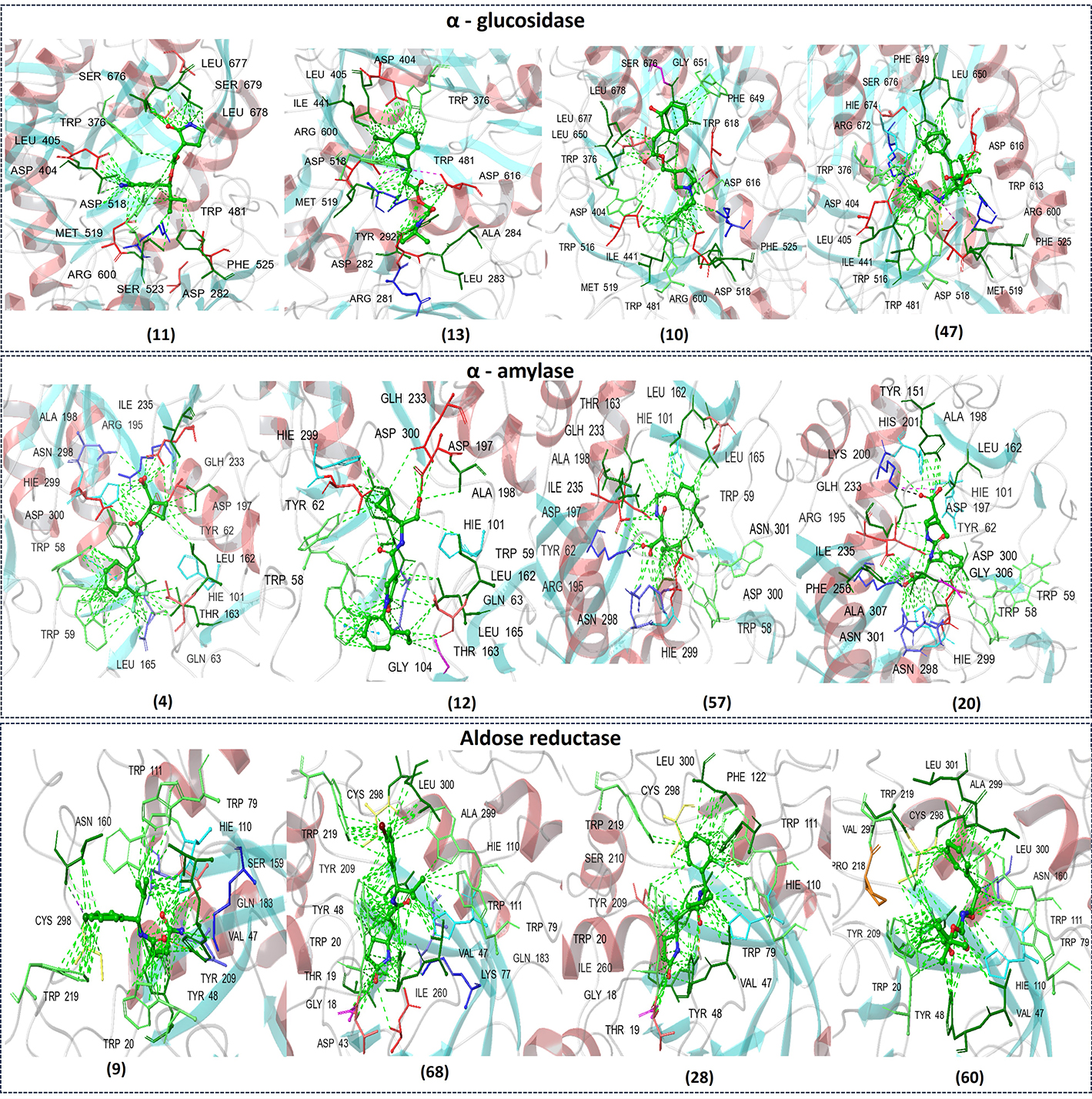
The figure represents the chemical structures of selected compounds and their corresponding binding interactions with the active site residues of α-glucosidase (top), α-amylase (middle), and aldose reductase (bottom)
For α-amylase, compound 4 exhibited the best docking score of −8.167 kcal/mol, forming hydrogen bonds with GLH233 and ASP197, pi-pi stacking with TRP58, and hydrophobic interactions with residues such as HIE299, TRP59, and LEU165. Compound 12 (score: −7.947 kcal/mol) showed hydrogen bonds with GLN63 and ALA198, pi-pi stacking with TRP58, and hydrophobic interactions with residues like ASP300 and HIE101. Compound 57 (score: −7.885 kcal/mol) demonstrated pi-pi stacking with TRP58, hydrogen bonds with ASP197 and GLH233, and hydrophobic interactions with TRP58 and ASN298. Compound 20, with a score of −7.613 kcal/mol, interacted through hydrogen bonds with ASN298, ARG195, and GLH233, along with hydrophobic interactions involving TYR62, and HIE299. For aldose reductase, compound 28 achieved the best docking score of −13.760 kcal/mol, forming hydrogen bonds with TRP20, TYR48, and HIE110 and hydrophobic interactions with TRP79, LEU300, and CYS298. Compound 68 (score: −13.595 kcal/mol) showed hydrogen bonding with TYR209 and hydrophobic interactions with residues like TRP79, TYR48, and ALA299. Compound 9 (score: −11.671 kcal/mol) formed hydrogen bonds with GLN183, SER159, TYR48, and hydrophobic interactions with TRP111. Compound 60 (score: −10.995 kcal/mol) interacted via hydrogen bonds with TYR48 and HIE110 and hydrophobic interactions with TRP20 and LEU300.
Discussion
The computational study demonstrated effective management of diabetes with 4HILe derivatives, showing no significant deterioration of glycemic control over the study period. The binding affinity of these compounds was evident through molecular docking analyses, which revealed strong interactions with key enzymes associated with carbohydrate metabolism, including α-glucosidase, α-amylase, and aldose reductase. Although the changes were not statistically significant, molecular docking analyses suggested the potential therapeutic benefits of 4HILe derivatives in managing hyperglycemia and preventing disease progression. Additionally, MD simulations confirmed the stability of 4HILe derivatives in managing hyperglycemia and preventing disease progression. Additionally, MD simulations confirmed the stability of 4HILe derivatives in binding to these targets, further reinforcing their inhibitory potential.
It is worth noting that while some derivatives demonstrated stronger binding interactions, their metabolic stability and bioavailability remain crucial factors requiring further investigation. Among the tested derivatives, 4HILe-4, 2R-3S-4R-4HILe, and 4HILe-Amide-2 exhibited enhanced stability and superior binding energetics compared to reference inhibitors, suggesting their potential for further drug development.
The AI-driven generative modeling using LigDream facilitated the design of novel inhibitors, with compound 10 emerging as a promising candidate. This compound displayed strong intermolecular interactions, structural stability, and optimal dynamic properties, positioning it as a potential next-generation antidiabetic agent. FEL and PCA validated the conformational stability of the selected inhibitors, confirming their suitability for therapeutic applications.
This study had several limitations. The structural variations in 4HILe derivatives may lead to different pharmacokinetic profiles, which were not addressed in the current computational framework. Additionally, the study did not incorporate experimental validation of the computational findings, highlighting the need for further in vitro and in vivo studies. Future research should focus on optimizing these inhibitors for improved metabolic stability and efficacy in biological systems.
In conclusion, this study successfully identified and optimised 4HILe derivatives as potential multi-target antidiabetic agents using an integrated computational approach. Molecular docking, MM-GBSA binding free energy calculations, and MD simulations confirmed the stability and strong binding affinities of selected 4HILe derivatives against key antidiabetic targets like α-glucosidase, α-amylase, and aldose reductase. Notably, 4HILe-4, 2R-3S-4R-4HILe, and 4HILe-Amide-2 demonstrated superior binding energetics and dynamic stability compared to reference ligands. Furthermore, AI-driven generative modeling using LigDream enabled the design of novel inhibitors, with compound 10 emerging as a promising candidate due to its strong binding interactions and structural stability. The integration of FEL and PCA further reinforced the selected inhibitors’ dynamic stability and conformational preferences. While the computational findings are promising, further experimental validation is required to confirm their efficacy. This study lays the foundation for developing next-generation therapeutics targeting diabetes and its complications.
Abbreviations
ΔG: | Gibbs free energy |
4HILe: | 4-hydroxy isoleucine |
DM: | diabetes mellitus |
FEL: | free energy landscape |
GNP: | nonpolar solvation energy |
GROMACS: | Groningen machine chemical simulations |
MD: | molecular dynamics |
MME: | molecular mechanics energy |
MM-GBSA: | molecular mechanics-generalized Born surface area |
PCA: | principal component analysis |
RMSD: | root mean square deviation |
RMSF: | root mean square fluctuation |
SGB: | solvation model for polar solvation |
SMILES: | simplified molecular input line entry system |
T2DM: | type 2 diabetes mellitus |
Supplementary materials
The supplementary Tables for this article are available at: https://www.explorationpub.com/uploads/Article/file/1008104_sup_1.pdf.
Declarations
Acknowledgments
The authors thank the National Institute of Pharmaceutical Education and Research (NIPER), S.A.S Nagar, and Department of Pharmaceuticals, Ministry of Chemicals and Fertilizers, New Delhi, Government of India for providing the facility.
Author contributions
LMK: Writing—original draft, Data curation, Software, Formal analysis, Visualization, Methodology. MSG and MES: Investigation, Writing—review & editing, Conceptualization, Resources, Methodology, Supervision. All authors approved the final version of the manuscript.
Conflicts of interest
The authors declare that they have no conflicts of interest.
Ethical approval
Not applicable.
Consent to participate
Not applicable.
Consent for publication
Not applicable.
Availability of data and materials
The data generated for this study are available from the corresponding author upon reasonable request.
Funding
Not applicable.
Copyright
© The Author(s) 2025.
Publisher’s note
Open Exploration maintains a neutral stance on jurisdictional claims in published institutional affiliations and maps. All opinions expressed in this article are the personal views of the author(s) and do not represent the stance of the editorial team or the publisher.