Artificial intelligence (AI) has taken the daily news with increasing impact. The crescent growth of computational power and the rapid development of algorithms to harness this computational capacity delineate the perfect scenario for this avalanche of information about AI. Drug science is not immune to this influence, and many drug discovery projects employ AI. A search on PubMed using as strings “artificial intelligence” and “drug discovery” returned 1,149 publications up to 2022 (January 23, 2023). The histogram is shown Figure 1. The plot indicates a rapid increase in publications after 2018.
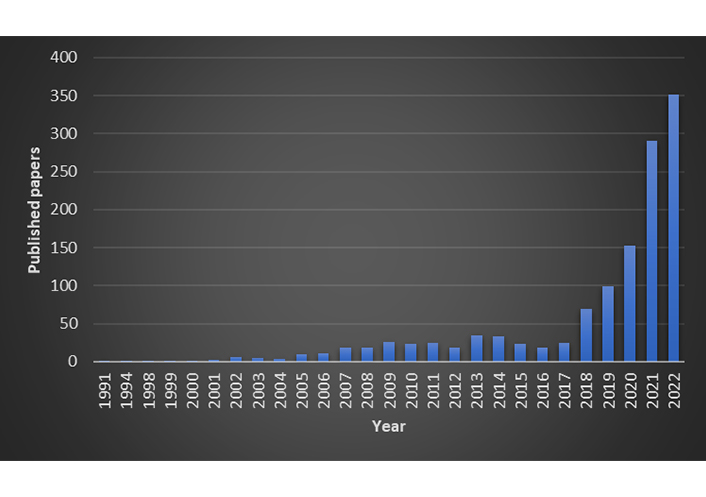
Publications related to applications of AI to drug discovery found in PubMed from 1991 to 2022
One recent application of AI to drug science is a study of rapamycin using Chat Generative Pre-trained Transformer (ChatGPT) [1]. This study used Pascal’s wager argument to speculate on the potential uses of rapamycin [1] to prolong life. ChatGPT (an AI program developed by Open AI) took the preclinical results and identified the effects of rapamycin on the extent of life. This special issue centers on machine learning (ML), a subfield of AI. ML focuses on automatically learning from data without being without explicit programming. ML techniques benefit from the explosion of biological and drug data to generate models to predict drug efficiency.
Computer-aided drug design (CADD) is the application of computational techniques in drug development [2]. CADD comprises two main computational approaches to address the design of a new drug: ligand-based drug design (LBDD) and structure-based drug design (SBDD) [3]. LBDD is used when the structure of a target is not available. LBDD employs experimental data available for ligands and seeks to derive a model based on descriptors to predict the efficiency of a molecule. SBDD relies on three-dimensional (3D) information about the targets. It is possible to have the structural information derived from experimental techniques [e.g., X-ray diffraction (XRD) crystallography] or computational modeling. The most striking application of the generation of 3D using computational approaches was the development of deep learning (DL) techniques to model structures of proteins [4, 5]. DL methods rely on multiple layers of neural networks to generate models of protein structures based on sequence information. In the taxonomy of AI, the DL techniques are included in a subfield of ML methods.
LBDD and SBDD benefit from ML approaches. LBDD may use ML to generate polynomial equations to predict affinity based on ligand structures [6]. For SBDD, it is possible to employ ML to explore the scoring function (SF) space (SFS) concept [7]. SFS is a mathematical space composed of SFs. These SFs predict binding affinity based on the atomic coordinates of a protein-ligand complex. It is common to use experimental structures or complexes obtained through protein-ligand docking simulations. ML techniques may explore SFS to find an adequate computational model to predict binding affinity.
SFS brings together ML techniques and systems biology thinking. The concept of SFS set up a systems-level approach to address the creation of computational models to calculate affinity based on the atomic coordinates. SFS abstraction is illustrated in Figure 2. Consider an element of the protein space [e.g., cyclin-dependent kinase 2 (CDK2)] complexed with a ligand of the chemical space (CDK2 inhibitor). ML techniques can generate an SF to estimate the affinity based on the atomic coordinates. It is possible to employ docking to create CDK2-inhibitor complexes for which binding affinity data is available. Then the dataset of CDK2-inhibitor complexes is split into two subsets, named training and test sets. ML techniques employ the training set to generate a new model to predict the binding affinity. The predictive performance is determined using metrics such as root-mean-squared error (RMSE), mean absolute error (MAE), and coefficient of determination (R2). A recent study recommended this set of metrics (RMSE, MAE, and R2) to validate supervised ML (SML) models in biology [8]. SML techniques englobe several regression methods (e.g., random forest, ensemble methods, and DL) with different predictive performances dependent on the characteristics of the dataset.
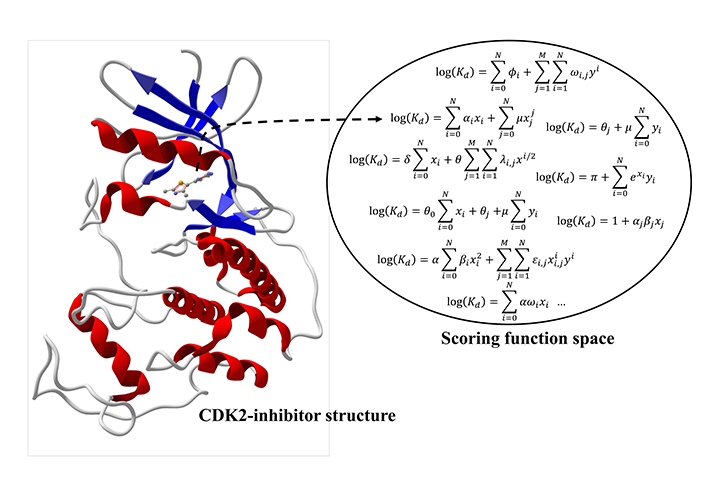
Schematic view of the SFS. Equations on the SFS are generic polynomials only to indicate the concept of an infinite number of equations. ┄→: the best scoring function for this protein
One key aspect of the progress of ML techniques is the available libraries for computational tools employed to generate ML models (e.g., scikit-learn). Scikit-learn was used to develop Statistical Analysis of Docking Results and SF (SAnDReS) [9, 10]. This program relies on energy terms calculated using docking programs to generate a targeted SF. This state-of-the-art interpretation of CADD adds plasticity to the procedure ignoring the concept of one-size-fits-all to create SFs [7]. Taking this abstraction, the focus is on discovering an adequate model from the SFS for one target. With this perception, targeted SF is employed to rank protein-ligand complexes in virtual screening simulations.
Due to the crescent number of complexes with affinity and structural data, unexplored parts of the protein and chemical spaces are now reachable. Particularly for the protein structures, additional protein space is reachable thanks to DL techniques used to model proteins. These 3D models are available at the protein data bank (PDB) (https://www.rcsb.org/) and Uniprot (https://www.uniprot.org/). All this advancement brings new opportunities to create models for one protein target. Also, as software progress continues, the number of ML models to calculate binding affinity will quickly increase, making it possible to create SF databases (SFDBs). These SFDBs will keep developed SFs that could be downloaded and employed for docking simulations or to calculate the affinity for 3D structures solved using XRD crystallography, nuclear magnetic resonance (NMR) spectroscopy, or cryogenic electron microscopy (cryo-EM). The SFS concept is a new paradigm for CADD, letting us generate more consistent ML models to predict protein-drug affinity.
In this special issue, it is explored that the SFS concept and other emerging ML techniques used in the study of drug science. This volume has a team of authors with experience in this interdisciplinary field who contributed to this issue.
Abbreviations
3D: |
three-dimensional |
AI: |
artificial intelligence |
CADD: |
computer-aided drug design |
CDK2: |
cyclin-dependent kinase 2 |
DL: |
deep learning |
LBDD: |
ligand-based drug design |
ML: |
machine learning |
SBDD: |
structure-based drug design |
SF: |
scoring function |
SFS: |
scoring function space |
Declarations
Author contributions
WFdAJ: Writing—original draft.
Conflicts of interest
The author declares that he has no conflicts of interest.
Ethical approval
Not applicable.
Consent to participate
Not applicable.
Consent to publication
Not applicable.
Availability of data and materials
Not applicable.
Funding
WFdAJ’s research is funded by CNPq (Brazil) [309029/2018-0; 306298/2022-8]. The funder had no role in study design, data collection and analysis, decision to publish, or preparation of the manuscript.
Copyright
© The Author(s) 2023.