Abstract
Artificial intelligence (AI) is revolutionizing plant sciences by enabling precise plant species identification, early disease diagnosis, crop yield prediction, and precision agriculture optimization. AI uses machine learning and image recognition to aid ecological research and biodiversity conservation. It plays a crucial role in plant breeding by accelerating the development of resilient, high-yielding crops with desirable traits. AI models using climate and soil data contribute to sustainable agriculture and food security. In plant phenotyping, AI automates the measurement and analysis of plant characteristics, enhancing our understanding of plant growth. Ongoing research aims to improve AI models’ robustness and interpretability while addressing data privacy and algorithmic biases. Interdisciplinary collaboration is essential to fully harness AI’s potential in plant sciences for a sustainable, food-secure future.
Keywords
Artificial intelligence, plant sciences, precision agriculture, machine learning, sustainable agricultureIntroduction
Artificial intelligence (AI) has emerged as a transformative force across various domains, and its integration into the field of plant sciences is poised to revolutionize the way we understand, cultivate, and sustainably manage plant life [1]. This paper provides a concise overview of the pivotal role of AI in advancing plant sciences, emphasizing its multifaceted applications, benefits, and potential future developments.
The utilization of AI in plant sciences including plant identification, disease diagnosis, yield prediction, phenotyping, and precision agriculture [2, 3]. Machine learning algorithms, coupled with image recognition techniques, have enabled rapid and accurate plant species identification, advancing ecological research as well as biodiversity conservation. AI-driven diagnostic tools empower plant pathologists and agronomists to early detection of diseases and pests, facilitating timely interventions that minimize crop losses [4]. Contribution of AI in the field of plant breeding is particularly noteworthy, as it aids in the development of resilient and high-yielding crop varieties. AI models accelerate the selection of superior genetic traits by analyzing vast datasets which accelerates the breeding process [5]. Furthermore, AI-based prediction models are leveraging climate and soil data that offer valuable insights into optimizing crop management practices and mitigating environmental impact [6]. This, in turn, promotes sustainable agriculture and food security in a world struggling with climate change and growing population pressures [7].
In the realm of plant phenotyping, AI-driven technologies automate the measurement and analysis of plant characteristics which provides a deeper understanding of plant growth and adaptation mechanisms for scientists [8]. The real-time monitoring of plant health and growth opens new avenues for innovative research and improved crop management practices [9].
Although the influence of AI on plant sciences is significant, ongoing research efforts are focused on enhancing AI models for robust and interpretable results [10, 11]. There is also increasing attention to ethical considerations regarding data privacy, algorithm biases, and the responsible use of AI in agriculture [12]. In addition to these interdisciplinary collaboration among plant scientists, data scientists, and engineers is essential for optimizing the potential of AI in the agricultural field [13].
This article provides an overview of the integration of AI into plant sciences represents a paradigm shift and offers unprecedented capabilities for plant species identification, disease management, breeding, phenotyping, and sustainable agriculture. As this technology continues to evolve, it is essential that stakeholders work together to harness its full potential while addressing the ethical and social implications of its adoption. This abstract highlights the transformative role of AI in advancing our understanding of plant life and optimizing agricultural practices for a more sustainable and food-secure future.
Big data analytics
In recent years, the field of plant science has undergone a major transformation with the advent of big data analytics and AI technologies [2]. The integration of these two fields has opened up new opportunities to understand and improve various aspects of plant biology, agriculture, and crop production [14]. Big data analytics in plant science is the use of advanced computational techniques to analyze large and complex datasets generated from various sources including genomics, phenomics, transcriptomics, proteomics, metabolomics, and environmental sensors [15]. This comprehensive analysis provides researchers to gain valuable insights into plant growth, development, stress responses, disease resistance, and yield optimization as shown below in Figure 1.
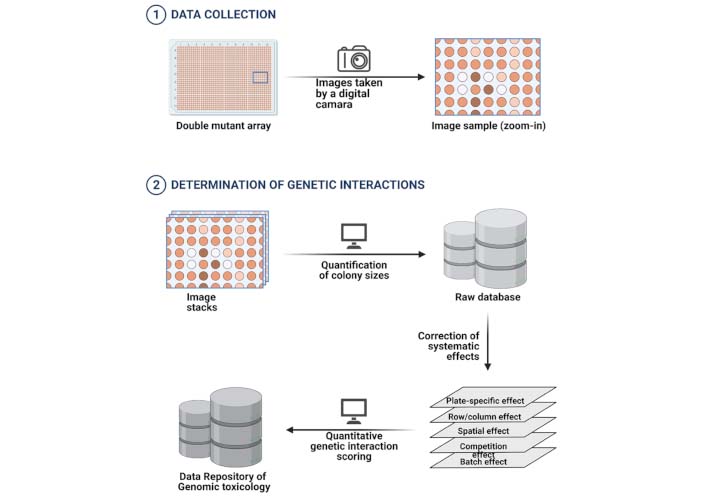
Blockchain in genomic toxicology. Digital gene interactions by synthetic gene array and storage of quantitative genetic interaction score in a blockchain data repository. The query genes are extracted from toxin-led mutants to determine genetic interaction with the ones from the original genome. The images of an array are taken by a digital camera and these image stacks are quantified and a raw database is created. The correction in this raw data with respect to different effects leads to genetic interaction scores which are stored in blockchain data repositories of genomic toxicology [16]
Note. Reprinted from “Digital Transformation in Toxicology: Improving Communication and Efficiency in Risk Assessment” by Singh AV, Bansod G, Mahajan M, Dietrich P, Singh SP, Rav K, et al. ACS Omega. 2023;8:21377–90 (https://doi.org/10.1021/acsomega.3c00596). CC BY.
Big data analytics techniques play an important role in extracting meaningful information from large-scale plant science datasets [17]. These techniques involve the application of statistical modeling, machine learning algorithms, and AI-based approaches to identify patterns, correlations, and predictive models [18]. Statistical methods such as regression analysis, Principal Component Analysis (PCA), and clustering algorithms help to identify relationships between different variables and group similar samples together [19].
Machine learning algorithms such as random Forests, Support Vector Machines (SVM), deep learning neural networks (DLNNs), and Bayesian networks are employed for tasks such as classification, regression, feature selection, and anomaly detection [20]. The applications of big data analytics in plant science provide numerous applications that contribute to the understanding and improvement of plant biology, agriculture, and crop production. Some of the key applications include:
(a) Genomics and breeding: the employment of big data to enhance the accuracy of complex trait prediction during hybrid breeding of crop plants [21]. Big data analytics enables the identification of genetic variations associated with desirable traits in plants [21].
(b) Phenomics and crop improvement: phenomics data obtained from high-throughput phenotyping platforms can be analyzed using big data analytics techniques to understand the complex relationships between genotype and phenotype [22]. This analysis helps to identify key traits that contribute to crop performance under different environmental conditions [22]. By integrating phenomics and genomics data, researchers can develop predictive models for crop performance and optimize breeding [23].
(c) Crop monitoring and precision agriculture: big data analytics with AI technologies enable real-time monitoring of crops using remote sensing, satellite imagery, and sensor networks [24, 25]. This allows farmers to make informed decisions regarding irrigation, fertilization, pest control, and harvesting based on accurate and updated information about crop health, growth stage, and yield potential [26, 27].
(d) Plant disease diagnosis and management: big data analytics can help in the early detection and diagnosis of plant diseases by analyzing large-scale datasets containing information about disease symptoms, environmental factors, and pathogen genomics [28].
(e) Climate change adaptation: big data analytics can help in understanding the impact of climate change on plant growth, development, and distribution [29–32]. By analyzing historical climate data and plant performance data, researchers can identify regions or specific crops that are most sensitive to climate change [33–35].
Blockchain technology
Blockchain technology is a decentralized and distributed ledger system that enables secure and transparent transactions as shown below in Figure 2 [36]. It has attracted significant attention in recent years due to its potential applications in plant science [37]. Blockchain technology along with AI can revolutionize the way plant science research is conducted, data is managed, and collaborations are established [38].
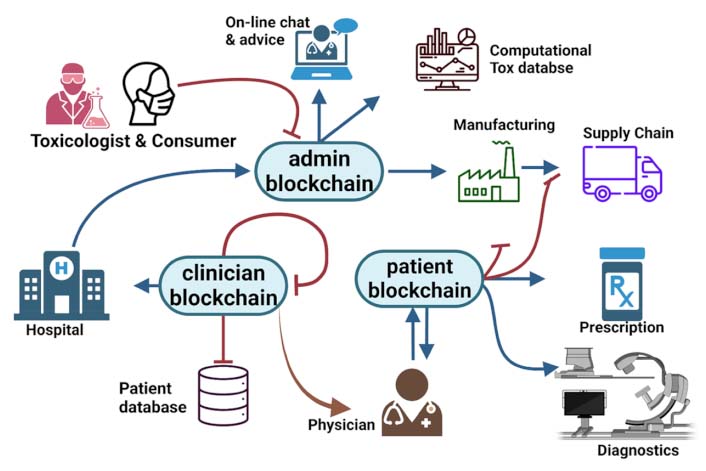
Blockchain technology in toxicology can aid in decentralized data keeping and evaluate data that and potential risks to consumers with exposure risks through available chemical databases. Material Safety Datasheet (MSDS) contains information about potential hazard-causing agents and how to work with them. Blockchain also involves traceability in the distribution network and the use of product barcodes in manufacturing. Collection, analysis, and evaluation of the data in lab testing and pharmacies are possible due to blockchain [36]
Note. Reprinted from “Digital Transformation in Toxicology: Improving Communication and Efficiency in Risk Assessment” by Singh AV, Bansod G, Mahajan M, Dietrich P, Singh SP, Rav K, et al. ACS Omega. 2023;8:21377–90 (https://doi.org/10.1021/acsomega.3c00596). CC-BY.
One of the biggest challenges in plant science research is the lack of transparency and trust in data sharing and collaboration [39]. Researchers often face difficulties in accessing and verifying data, which hindering progress and slows scientific discoveries. Blockchain technology can address these challenges by providing a secure and immutable platform for storing, sharing, and verifying data [40, 41]. Moreover, blockchain technology can help combat counterfeit seeds or plants by providing a tamper-proof record of their origin and authenticity. This is important in plant breeding programs where maintaining the integrity of genetic resources is essential for development of new varieties [42].
3-D printing
3-D printing also known as additive manufacturing, is a revolutionary technology that has attracted significant attention in various fields, including plant science [43]. When it comes to the intersection of 3-D printing and plant science, AI plays a key role in enhancing the capabilities and applications of this technology [43].
Tissue engineering and organogenesis: one of the key applications of 3-D printing in plant science is tissue engineering and organogenesis [44]. Using AI algorithms, scientists can design and create complex structures that mimic the architecture of plant tissues and organs [45] (Figure 3).
Precision agriculture: another area where 3-D printing intersects with AI in plant science is precision agriculture [47]. Precision agriculture’s aim is to optimize crop production by monitoring and managing agricultural practices using data-driven approaches [47, 48]. By integrating AI algorithms with 3-D printing technology, farmers can create tools and equipment customized to the specific requirements of their crops [49].
Plant micropropagation: plant micropropagation, also known as tissue culture, is a technique for rapidly propagate plants in a controlled environment. The combination of 3-D printing and AI could revolutionize this process by enabling the production of customized growing media and plant culture containers [44].
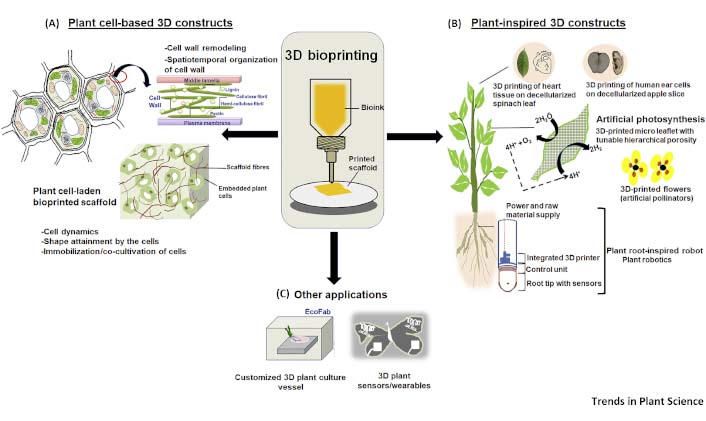
Exploring the growth and possibilities of 3-D bioprinting in advancing plant science research [46]
Note. Reprinted with permission from “3D Bioprinting in Plant Science: An Interdisciplinary Approach” by Mehrotra S, Kumar S, Srivastava V, Mishra T, Mishra BN. Trends Plant Sci. 2020;25:9–13 (https://doi.org/10.1016/j.tplants.2019.10.014). © 2019 Elsevier Ltd.
Machine learning
Machine learning in plant science is a rapidly growing field that uses AI techniques to analyze and interpret complex biological data related to plants [50]. By applying machine learning algorithms to large datasets, researchers can uncover patterns that make predictions and gain insights into various aspects of plant biology including plant growth, development, disease resistance, and crop yield optimization [5]. This integration of machine learning and plant science has the potential to revolutionize agriculture and contribute to sustainable food production [45].
One of the primary applications of machine learning in plant science is in plant phenotyping [5, 51]. Phenotyping involves the measurement and analysis of observable traits and characteristics of plants, such as leaf area, height, biomass, and photosynthetic efficiency [52]. Traditionally, phenotyping has been a labor-intensive and time-consuming process [23]. However, with the advent of machine learning techniques, it is now possible to automate and simplified this process [53].
Machine learning algorithms can be trained on large datasets of plant images or sensor data collected from various sources such as drones, satellites, and field sensors [54]. From these data sources, these algorithms have the capability to recognize patterns and extract valuable information [28]. For example, convolutional neural networks (CNNs) a type of deep learning algorithm, have been successfully used to classify different plant species based on images of leaves. By analyzing thousands of leaf images with known species labels, CNNs can learn to identify key features that distinguish one species from another [55].
Machine learning can help in the optimization of crop management practices. Algorithms can identify optimal planting dates, irrigation schedules, and fertilizer application rates by analyzing large data sets that include information on soil properties, weather conditions, and crop performance [56].
Machine learning techniques are also being applied to plant genomics and transcriptomics. Genomic data provide valuable insight into the genetic basis of plant traits and responses to environmental stimuli [5]. Machine learning algorithms can analyze genomic data to identify genes associated with specific traits and predict gene functions [57]. Similarly, machine learning can analyze transcriptomic data that captures pattern of gene expression under diverse circumstances to understand how plants respond to various stresses and treatments [58, 59] (Figure 4).
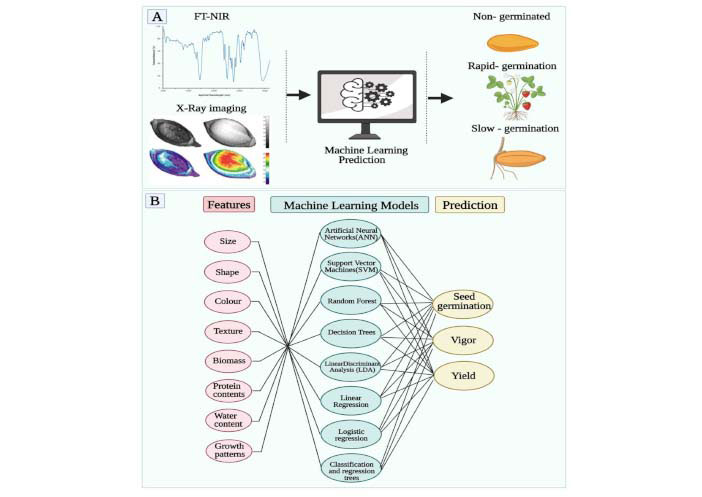
Artificial intelligence (AI) and Machine learning (ML) may assist with Infrared spectroscopy and X-ray image data mining and curation. AI & ML may further complement via optimizing seed priming technology based on chemi-analytic profiling for heat and moisture content assisting in breaking seed dormancy. Post nano-priming, AI feedback tools help in managing irrigation, water usage, and soil management thereby leading to efficient seed production for the future [60]
Note. Reprinted from “Sustainable Agriculture through Multidisciplinary Seed Nanopriming: Prospects of Opportunities and Challenges” by Shelar A, Singh AV, Maharjan RS, Laux P, Luch A, Gemmati D, et al. Cells. 2021;10:2428 (https://doi.org/10.3390/cells10092428). CC BY.
In the context of plant science, machine learning algorithms are employed in plant sciences to analyze plant-related data, such as genomic information, phenotypic traits, environmental factors, and agronomic practices to gain insights about plant biology, improve crop yield and quality, and optimize agricultural practices [50].
Supervised learning algorithms
Supervised learning algorithms are widely used in plant science to build predictive models based on labeled training data [61]. These algorithms learn from input-output pairs and can be used for tasks such as plant disease diagnosis, yield prediction, and crop classification. Supervised learning algorithms commonly used in plant science include:
SVM: SVM is a powerful algorithm that can be used for both classification and regression tasks [62].
Random Forests: Random Forest is an ensemble learning method that combines multiple decision trees to make predictions. Each tree is trained with a random subset of the training data and features to reduce overfitting and improve generalization [63].
Gradient Boosting: Gradient Boosting is another ensemble learning technique that combines multiple weak learners (usually decision trees) to build a powerful predictive model. It has been successfully used in plant science for tasks such as predicting crop yields, diagnosing diseases, and predicting traits [64].
Unsupervised learning algorithms
Unsupervised learning algorithms are used to discover patterns and structures in unlabeled data without using predefined outputs [65]. These algorithms are particularly useful in plant science for tasks such as clustering similar plants, identifying hidden patterns in gene expression data, and discovering new plant traits. Some common unsupervised learning algorithms used in plant science include:
K-means Clustering: K-means clustering is a common algorithm used to partition data into a specified number of clusters [66]. The aim is to minimize the distance between data points within each cluster while maximizing the distance between different clusters. K-means clustering is used in plant science for tasks such as plant phenotyping, genotypic clustering, and trait recognition [50].
PCA: PCA is a dimensionality reduction technique that transforms high-dimensional data into a low-dimensional space while preserving the most important information [67]. PCA has been widely used in plant science for tasks such as gene expression analysis, trait visualization, and trait selection [68, 69].
Self-Organizing Maps (SOM): SOM are a type of artificial neural network (ANN) that learns to map high-dimensional input data onto a low-dimensional network grid of nodes or neurons [70, 71]. SOMs are used in plant science for tasks such as gene expression analysis, phenotypic mapping, and genotype-phenotype association studies [72].
Expert systems and fuzzy logic
Expert systems and fuzzy logic are two important concepts in the field of AI and have been applied in various domains, including plant science [73, 74]. These techniques are used to improve decision-making processes, improve plant growth and productivity, and optimize resource management in agricultural practices [75].
An expert system is a computer-based system that mimics the decision-making abilities of a human expert in a certain field [76]. It consists of a database that stores domain-specific information and an inference engine, which uses logical rules to reason and make decisions based on the available information [77]. In the context of plant science, expert systems can be developed to assist in tasks such as diagnosing plant diseases, recommending suitable fertilizers and pesticides, and providing guidance on crop management practices [78].
Fuzzy Logic, on the other hand, is a mathematical framework that deals with the uncertainty and imprecision of decision-making processes [79]. In contrast to traditional binary logic, which deals with definite values (true or false), fuzzy logic allows for degrees of truth between 0 and 1. This flexibility allows fuzzy logic to handle complex and ambiguous situations more effectively [80]. In plant science, fuzzy logic has been applied in various fields such as crop forecasting, yield prediction irrigation scheduling, and pest management. Integrating expert systems and fuzzy logic into plant science can lead to more intelligent and efficient agricultural practices [81].
ANNs and genetic algorithms
ANN is a computational model inspired by the structure and functions of biological neural networks in the human brain [82]. They consist of interconnected nodes called artificial neurons or units that process and transmit information through weighted connections [83]. On the other hand, genetic algorithms (GAs) are search algorithms based on the principles of natural selection and genetics [84]. They imitate evolutionary processes to find optimal solutions to complex problems [84].
ANNs have been successfully employed in various plant science tasks. For example, in plant classification, ANNs can be trained on large plant image datasets to accurately identify different species or cultivars [85]. By learning from a diverse set of features such as leaf shape, color, texture, and venation patterns, ANNs can classify plants with high accuracy [55, 86, 87]. It has implications for biodiversity conservation, ecological research, and agriculture [88]. In disease diagnosis, ANNs have shown promising results in identifying plant diseases based on symptoms and visual signals [89]. This allows early detection and rapid intervention, preventing the spread of disease and minimizing crop losses [90].
GAs have also been applied in plant science, especially in optimization problems [91]. For example, GA has been used to optimize irrigation schedules, with the goal of determining optimal watering rates and timing to maximize crop yield and minimizing water consumption [91]. By representing different irrigation strategies as candidate solutions and evaluating their suitability based on predefined criteria (e.g., yield, water use efficiency, etc.), GAs can search for near-optimal solutions in a large solution space [92, 93]. Similarly, GA has also been used to optimize crop management, including determining optimal plant density, fertilizer application rates, and pest control strategies [94].
Predictive analytics
Predictive analytics uses statistical models, machine learning algorithms, and data mining techniques to extract meaningful patterns and relationships from large data sets [95]. In the context of plant science, these techniques can be applied to various types of data, including genomic data, phenotypic data, environmental data, and historical crop yield data [96]. By analyzing these datasets, predictive analytics can help researchers and farmers make informed decisions about crop management, disease prevention, crop yield optimization, and resource allocation [96].
One of the most important applications of predictive analytics in plant science is crop yield prediction. By analyzing past crop yield data along with environmental factors such as weather conditions, soil quality, and nutrient availability, AI algorithms can learn patterns and relationships that can be used to predict future crop yields [97].
Another important application of predictive analytics in plant science is disease detection and prevention. Plant diseases can seriously affect crop yield and overall agricultural productivity [97].
In addition to these applications, predictive analytics in plant science can also be used for genomic selection, crop breeding, trait prediction, climate change impact assessment, etc. [98, 99].
Agents and robotics
In recent years, the integration of AI technologies such as agents and robotics has significantly impacted the field of plant science. An intelligent agent is a computer program that can perceive the environment, draw conclusions, and take action to achieve a specific goal [100]. In the context of plant science, intelligent agents can be used to automate tasks such as data collection, analysis, and decision-making. For example, autonomous robots equipped with sensors and cameras can be deployed in agricultural fields to collect data on plant growth, soil conditions, and pest infestation [101]. These agents can use AI algorithms to analyze the collected data and provide real-time insights to farmers and agronomists.
Robotics refers to the design, development, and application of use of robots. A robot is a mechanical device that is programmed to perform tasks autonomously or semi-autonomously [102].
In the context of plant science, robots are designed to interact with plants and their environment to collect data, conduct experiments, and performs specific tasks [103].
These robots can be equipped with a variety of sensors, actuators, and imaging systems to gather information about plant growth, health, and environmental conditions [104].
By combining robotics with AI techniques such as machine learning and computer vision, researchers can create intelligent systems that can automate phenotyping, optimize resource in precision agriculture, accelerate plant breeding, and improve crop protection strategies [105]. These advances can improve crop yields, enhance sustainability, and respond to the challenges of climate change in agriculture.
Internet of Things sensors
In recent years, the integration of Internet of Things (IoT) sensors and AI has revolutionized various fields including plant science [106]. IoT sensors are devices that collect and transmit data from the physical environment and send it to a central system, where AI algorithms analyze this data to gain valuable insights and make informed decisions [107].
In the context of plant science, IoT sensors play an important role in monitoring and optimizing various aspects of plant growth, health, and productivity. This comprehensive integration has led to advances in precision agriculture, smart farming, and sustainable crop management practices [108]. One of the key applications of IoT sensors in plant science is environmental monitoring. These sensors can measure parameters such as temperature, humidity, light intensity, soil moisture and nutrient content in real-time [106]. Another important application of IoT sensors in plant science is in pest and disease management [109]. Early detection and prevention of pests and diseases are crucial to minimize crop losses and reduce the need for chemical interventions [110].
Object image capture and analysis
Object image capture refers to the process of obtaining high-quality images of plants or plant parts for further analysis [111, 112]. In the context of plant science, this usually involves capturing images of leaves, flowers, fruits, or whole plants using various imaging techniques such as digital cameras, hyperspectral imaging, or 3-D scanners [113]. The goal is to obtain detailed visual information that can be used to extract relevant and meaningful features and characteristics of plants [112].
For example, computer vision algorithms can automatically identify, recognize, and track plants based on images, enabling the development of autonomous robotic systems that can capture images of plants in large-scale field trials. These systems can be equipped with cameras mounted on drones or ground-based platforms to capture images from different angles and perspectives [112, 114].
Image analysis
Once the images are captured, AI techniques are used to analyze them and extract valuable information from them. Image analysis in plant science includes various tasks such as segmentation, feature extraction, classification, and quantification [115, 116].
Applications in plant science
Some notable applications include:
Plant phenotyping: AI-based image analysis enables efficient phenotyping by automatically extracting and quantifying various plant traits from large-scale image data sets [117, 118].
Disease detection: early detection of plant diseases is crucial for effective disease management in agriculture [119, 120].
Yield prediction and crop management: by analyzing images of plants throughout their growth stages, AI models can estimate crop yields based on factors such as plant density, canopy cover, and fruit count [121].
Application of ANN in plant science
ANNs have found numerous applications in plant science and have revolutionized the field by providing powerful tools for data analysis, modeling, and prediction [122]. Plant phenotyping is an important field where ANNs have made significant contributions [123]. The prediction of crop yield is another important application of ANNs in plant science. Accurate yield prediction is essential to optimize agricultural practices and ensure food security [124].
Diagnosis of plant diseases is a challenging task, but significant benefits can be obtained from the use of ANNs. Plant diseases can cause significant economic losses by reducing crop yields or even complete crop failure [125]. ANNs can be trained on large datasets containing information about disease symptoms, environmental conditions, and the occurrence of pathogens to develop accurate diagnostic models [123, 124].
Gene expression analysis is another area where ANNs have been successfully used in plant science. ANNs can analyze large-scale gene expression datasets to identify patterns and relationships between genes and their functions [126].
ANNs use experimental data to identify the relationships between environmental factors (such as light, temperature, and nutrient availability) and plant growth parameters (such as leaf area, biomass accumulation, and root development) [127].
Conclusion
In conclusion, the integration of AI into plant sciences has ushered in a transformative era. It has opened up new avenues for understanding and managing plant life, which is useful for ecological research, biodiversity conservation, disease detection, crop breeding, and sustainable agriculture. The potential of AI in this field is immense, but it comes with the responsibility of refining AI models for robustness and addressing ethical considerations. As we navigate this exciting journey, interdisciplinary collaboration remains the cornerstone that guides us toward a future that is not only more sustainable but also more food-secure. AI in plant sciences represents a powerful tool in our quest to feed a growing global population while preserving our environment.
Abbreviations
AI: | artificial intelligence |
ANN: | artificial neural network |
GAs: | genetic algorithms |
IoT: | Internet of Things |
PCA: | Principal Component Analysis |
SOM: | Self-Organizing Maps |
Declarations
Acknowledgments
During the preparation of this work, the authors used the QuillBot and Perplexity AI to improve the language and readability of the paper. After using the tool/service, the authors reviewed and edited the content as needed and took full responsibility for the content of the publication.
Author contributions
DKG: Conceptualization, Methodology, Data curation, Writing—original draft. AP: Investigation, Formal analysis, Visualization, Writing—review & editing. PZ: Supervision, Project administration, Funding acquisition, Writing—review & editing. AKS: Software, Validation, Writing—review & editing. Each author has contributed significantly to the research project, and the order of authors reflects their respective roles in the study. All authors read and approved the submitted version.
Conflicts of interest
Not applicable.
Ethical approval
Not applicable.
Consent to participate
Not applicable.
Consent to publication
Not applicable.
Availability of data and materials
Not applicable.
Funding
Not applicable.
Copyright
© The Author(s) 2024.