Abstract
Table olives are one of the most widespread fermented foods in the Mediterranean area, presenting an exponential increase in global consumption in the latest years. As a fermented product, its microbiota consists of a complex ecosystem, the composition of which depends on a multitude of factors and affects the quality attributes of the final product. The swiftly developing and constantly evolving field of omics technologies is being applied to unravel the profile of the microbial ecosystem and enable a deeper understanding of the fermentation process. In particular, the use of amplicon metagenomics facilitates the thorough analysis of the microbiota involved as it encompasses both culturable and unculturable microorganisms. Volatilomics aims at the identification and quantification of the volatile metabolites formed during fermentation with a direct involvement in the safety and quality evaluation of the food product. The integration of metagenomic and volatilomic data, through the application of bioinformatics can enhance the understanding of the interplay between the microbial profile and volatilome, resulting in a more comprehensive view of the system. This review summarized the overall amplicon metagenomics and volatilomics analytical approaches, along with the currently available bioinformatics tools for the data analysis in the field of table olives. Emphasis is given to the integration of amplicon metagenomic and volatilomic data employed to characterize the diversity of microbial populations and reveal the relationships between them and the volatile compounds. The latter may provide an extensive view of the microbial community dynamics, which is key in table olive fermentation and the microbiota’s functional properties. The potentiality to evaluate their effect in shaping the quality and unique features of the final product is highlighted.
Keywords
Metagenomics, amplicon metagenomics, volatilomics, table olives, fermentation, food analysis, bioinformatics, omics data integrationIntroduction
Table olives (Olea europaea L.) are considered one of the most important plant-based fermented foods in the Mediterranean area, occupying a prominent position in the field of agriculture and the processing sector of these countries [1–3]. According to data published by the International Olive Council (IOC) for the years 2022/2023 regarding the production of table olives in Europe, Spain holds the first place (50.2% of total production), followed by Greece (39.4%) and Italy (7.6%) [4]. A constant increase is being reported over the years concerning the worldwide consumption of table olives [3]. This has been attributed to their high nutritional value and functional attributes [2], deriving from bioactive and health promoting compounds [5], such as monounsaturated fats, polar phenolic compounds, alpha-tocopherol [6] and the role of table olives as carriers of potential probiotics [5]. An approximate guide of raw olive flesh composition (% w/w) is 60–80% moisture, 10–30% fat, 1–1.5% protein, sugars (2–5%) and polar phenolic compounds (1–3%). The primary polar phenolic compound is oleuropein, which is responsible for the bitter taste of the drupe, making the direct consumption of the olives impossible [3, 7–9]. Therefore, the main goal of the processing is to turn the olive fruit into an edible final product with desirable organoleptic properties that is also safe for consumption [5, 9, 10].
According to the Trade Standard Applying to Table Olives (COI/OT/NC. Nº 1/2004) [11], there are three types of olives: green, turning color and black which can be classified according to the trade preparations applied (alkali treated, natural, dehydrated, darkened by oxidation) and the way they are presented to the consumer (styles), e.g., whole, stoned, stuffed etc., as depicted in Figure 1. The most popular commercial types of table olives are the Spanish-style green olives, the Greek-style natural olives in brine and the Californian-style black ripe olives. During the Spanish-style preparation, green olives are treated in alkali, followed by brining and fermentation. The Greek-style is a milder processing method where the olives are directly brined and undergo fermentation naturally. It involves primarily black olives. For the Californian processing method, the olives are darkened by iron-salt treatment and air-oxidation in an alkaline medium and then are heat treated before packaging [9, 11, 12].
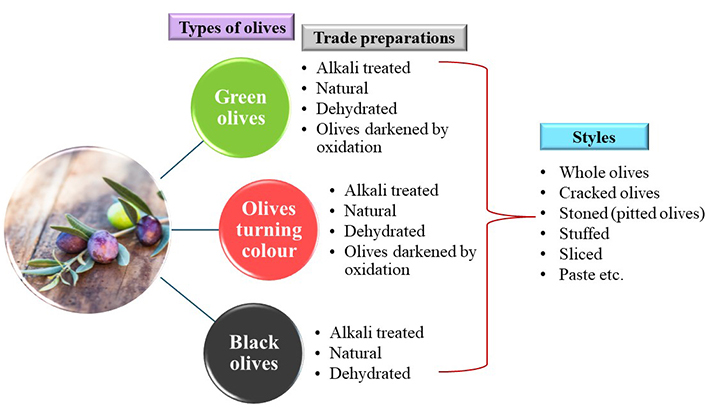
Classification of table olives according to type, trade preparations and styles, as reported by the COI/OT/NC. Nº 1/2004 standard [11]
Table olive fermentation primarily occurs spontaneously and is the outcome of the competitive activities of the indigenous microbial composition along with a variety of contaminating microorganisms that derive from the fermentation vessels, pipelines, pumps, and any other device that may come into contact with the olives and brine. The indigenous microbiota in return is influenced by numerous features of the raw olive fruit (cultivar, geographical origin, integrity, harvesting conditions, and postharvest treatment) that impact greatly the quality attributes of table olives [13]. The key microbiota that drives the fermentation of table olives and determines the safety and quality of the final product are lactic acid bacteria (LAB) and yeasts, which dominate the entire fermentation process forming a microbial consortium. At the beginning of the fermentation other non-dominant members can be detected which include the Enterobacteriaceae family, the genera Clostridium, Pseudomonas, Vibrio, Staphylococcus [2, 3, 14] and occasionally molds, mainly in natural black olives [3]. Representative genera of the LAB microbiota detected during table olive fermentation are Lactiplantibacillus (former Lactobacillus), Lacticaseibacillus (former Lactobacillus), Leuconostoc, Pediococcus, Enterococcus and Streptococcus [3, 15, 16]. However, independently of the olive cultivar and the processing method, the dominant and most frequently detected species are Lactiplantibacillus plantarum and Lpb. pentosus [5, 7, 17]. LAB play a crucial role during fermentation. They convert the fermentable sugars mainly into lactic acid and other compounds, leading to the acidification of the brine (final products pH approximately 4) [15]. This property, combined with the production of antimicrobial compounds inhibits the growth of spoilage microorganisms and food-borne pathogens enhancing the microbiological stability and extends the shelf life of the final product [5, 16, 18]. Their role also expands to the synthesis of compounds that improve the organoleptic properties (e.g., flavor), as well as to the natural debittering process, through enzyme production (β-glucosidase and esterase), which catalyze oleuropein degradation to non-bitter end products, hydroxytyrosol and elenolic acid [5, 17, 18]. Concerning the yeast profile, the most significant and frequently detected genera form different table olive processings are Candida, Debaryomyces, Pichia, Rhodotorula, Saccharomyces and Wyckerhamomyces [2, 19, 20]. During table olive processing, yeasts produce various compounds such as alcohols, ethyl acetate, acetaldehyde and organic acids contributing to the preservation and flavor of the product. However, yeasts can lead to gas pocket formation due to carbon dioxide production, softening of the olive tissue, clouding of the brines and off-flavor production [5, 12, 16, 19].
It becomes evident that this complex and dynamic ecosystem is closely related to the safety and the development of desirable table olives attributes, as well as to their cultivar and geographical origin discrimination. Therefore, the characterization of the microbiota of table olives could provide valuable insights into the mechanisms of the fermentation, allow the standardization of the process and lead to the development of starter cultures, to produce a consistent and high-quality product with desirable features, extended shelf-life and even health-promoting properties [5, 17]. For a long time, the only tool available to investigate this complex ecosystem were culture-dependent techniques [2]. The use of culture-dependent techniques, besides being labor intensive and time consuming [21], exhibits significant limitations as they capture only a fraction of this complex ecosystem [3]. They rely on the cultivation and isolation of the microorganisms prior to their phenotypic and genotypic characterization [2]. Therefore, the ones belonging to the viable but not culturable category are omitted and the interactions among the microbial communities are overlooked [21]. More than 90% of microorganisms from natural environments are estimated to be unable to grow in vitro, and about 25–50% of the ones found in fermented foods remain uncultured when conventional culture techniques are used [21, 22]. On the contrary, culture-independent techniques (e.g., metagenomics, metatranscriptomics, etc.) offer a deeper understanding of these complex and dynamic microbial communities and their effect on the fermentation process and the characteristics of the final products [3, 21].
The omics field comprises of various high-throughput methodologies, such as genomics, transcriptomics, proteomics, metabolomics as well as their respective meta-omics derivatives, such as metaproteomics sequencing-based meta-omics (metagenomics and metatranscriptomics), etc. [2, 3, 21]. Single-omics technologies address only a single biological process and do not enable thorough investigation of the interactions between the community members during fermentation as they provide single results about the nucleic acids, proteins, or metabolite levels. What is more, single-omics such as metagenomics fail to differentiate living from dead cells and therefore the active microbiota contributing to the fermentation and its interactions with other metabolites [23]. On the other hand, the combination of various omics technologies, a multi-omics approach in the field of fermented foods is a strategy that offers a holistic view of this complex ecosystem covering different aspects in parallel (e.g., metagenome and metabolome) [24]. Multi-omics could facilitate a more accurate understanding of the changes and interactions taking place among the microbial community during the different stages of fermentation as well as their connection to the production of compounds influencing the quality attributes and safety of the final product. What is more, this approach allows the comprehension of the mechanisms driving the fermentation and the selection of optimal starter cultures. This in return could lead to the improvement of the fermentation conditions and increase the value of fermented foods that meet consumers’ demands for healthier, flavorful, and functional foods [23]. Crucial is also the impact of the application of multi-omics in food safety minimizing the risk of foodborne pathogens and improving control over spoilage process [24]. Such advanced research in table olive fermentation is still in need of application [25]. In this scope, several studies have utilized the integration of volatilomic and metagenomic data as a promising approach to unravel the relationships between the microbiota and the microbial volatile profile and evaluate their impact on the quality attributes of table olives [2, 25].
Terminology concerning the classification of the metagenomic analysis varies [26–29]. The term “metagenomics” has both been used to characterize the untargeted sequencing of the metagenome of a sample as well as a generic term [27] that includes amplicon sequencing, also referred to as metabarcoding or metataxonomics [21]. In this review, the terminology used follows the suggestion of Nam et al. [29] and, hence, the two primary approaches to examine the genomic data that can be distinguished are “amplicon metagenomics”, which investigates the microbial diversity and “shotgun metagenomics”, that allows the sequencing of the metagenome and also investigates the functional properties of the microbial community [30]. Overall, omics technologies along with bioinformatics tools are suitable for the investigation of an elaborate biological system, such as table olive fermentation, whose composition, activity, and functions significantly affect the quality of the final product [2]. Standardized bioinformatics pipelines are essential for data sharing and interpretation in a comparable and reliable way [21].
This review summarizes the overall metagenomics and volatilomics analytical processes, along with the currently available bioinformatics tools for the data analysis in the field of table olives. Following the screening of the scientific literature, limited results were generated regarding the application of shotgun metagenomics and metatranscriptomics in table olive fermentation system in contrast to the notable abundance regarding amplicon metagenomics. Therefore, this technology is a focal point of this review. In particular, special focus is given on the integration of amplicon metagenomic and volatilomic data, employed to characterize the diversity of microbial populations and reveal the relationships between them and the volatile compounds. The latter may provide an extensive view of the microbial community dynamics, which is key in table olive fermentation and their functional properties. Recent research papers that employ this strategy are discussed and the potentiality to evaluate their effect in shaping the quality and unique features of the final product is highlighted.
Fundamentals and classification of sequencing-based meta-omic approaches in the analysis of microbial communities in table olive fermentation system
In this section a more detailed description of the sequencing-based meta-omic approaches, amplicon metagenomics, shotgun metagenomics and metatranscriptomics will be provided.
Amplicon metagenomics is of targeted nature and is based on the amplification of specific genes of interest (marker genes) or genomic regions. Afterwards, sequencing of the produced amplicons follows [31] and then alignment against a reference database to unravel the bacterial taxonomy [32]. This targeted approach of metagenomics is also referred to as metagenetics, or metataxonomics, or metabarcoding and even as 16S metagenomics or 16S ribosomal RNA (rRNA) gene sequencing [21]. For the identification of bacteria, the gene that is primarily targeted is the 16S rRNA gene which is universally found in bacteria. It consists of nine highly conserved regions, where the primers bind during the polymerase chain reaction (PCR) amplification and nine hypervariable regions (V1-V9) which are utilized for the investigation of the bacterial diversity [21, 28]. Amplicon metagenomics allows the investigation of less explored microbial communities that were unable to be investigated through the conventional culture-dependent techniques, consequently revealing a more complex consortium of yeasts and LAB in table olives [33]. Although, it is a cost-effective technology to achieve identification of a wide range of bacteria and eukaryotes, can be applied to large-scale research and the analysis of the generated data is easy and quick, it focusses only on the target gene content, omits the identification of viruses and facilitates identification only up to the species level [26, 34, 35]. However, amplicon metagenomics has become the most extensively employed approach to investigate the microbial ecosystem [28, 36] and is widely implemented in table olive research. Besides the ability to assess the microbial dynamics at different stages of the fermentation process, it could lead to the enrichment of the knowledge of the fermentation process and the identification of biomarkers (e.g., specific volatile compounds related to specific microbiota) for the evaluation of the quality and therefore improve the production of the final product [36].
Two recent reviews, by Vaccalluzzo et al. [2] and Tsoungos et al. [3] relating to the application of omics and meta-omics technologies in table olive research include an extensive recording of studies employing amplicon metagenomics in table olives to unveil the microbial dynamics taking place during table olive fermentation. Table 1 summarizes the information concerning different regions and/or primers used for amplicon metagenomics in table olive microbiome research, that has been extracted from the studies included in the above-mentioned reviews, to create an evidence table highlighting these differences. It becomes noticeable that the majority of the papers employ the V3-V4 region and that sequencing is performed on the Illumina platform. However, indiscriminately of the region selection (i.e., V1-V3, V3-V4, V2-4-8, V3-7-9), the common denominator in almost all cases is the V3 region. As reported in Table 1, both Kazou et al. [37] and Michailidou et al. [38] investigated the microbiota of Greek-style Kalamata olives, employing different regions for the sequencing of the 16S rRNA gene (V1-V3 and V3-V4 respectively). Noticeable differences are reported from the bacterial genera detected. It would be unwise though to speculate that this result derives solely from the selection of the sequencing region as it could be attributed to various factors. These include the processing period, the geographical origin of the samples and the environment of the processing unit. Furthermore, although both Randazzo et al. [39] and Vacalluzzo et al. [40] employed the same region (V3) to investigate the bacterial diversity of natural green Nocellara Etnea olives, it also resulted in the detection of different genera with the (former) Lactobacillus genus being common in both cases. Once again, these findings could be due to the same factors mentioned above and even due to the different percentage of NaCl in brines (8% and 8% and 5% salt respectively). In both cases marine salt was used to prepare the brine explaining the detection of halophilic bacteria. Evident is also the fact that, in some cases although the same hypervariable region of the 16S rRNA gene is selected, different primers are used. Sequencing platforms like Roche 454 pyrosequencing frequently targets the V1-V3 region, while the Illumina platform focusses primarily on the V3 and V4 region [28, 35]. The target microbial group, the nature and size of the samples, the cost of the analysis and available bioinformatic tools for data analysis are among the factors that influence the selection of the sequencing platform [41]. Less variations are observed concerning the regions for yeast/fungi identification, where the selection of ITS1 and ITS2 dominates.
Different regions sequenced and primers used in amplicon metagenomics in table olives
Processing method | Olive cultivar | Gene | Region/loci | Primers | Sequencing platform | Target microorganisms | Genera | Reference |
---|---|---|---|---|---|---|---|---|
Natural green | Nocellara Etnea | 16S rRNA | V3 | Probio_Uni & Probio_Rev | Ion Torrent | Bacteria | Halomonas, Achromobacter, Marinobacter, Serratia, Bradyrhizobium, (former) Lactobacillus, Pediococcus, Hafnia, Enterococcus | Randazzo et al. [39] |
Natural green | Aloreña de Málaga | 16S rRNA | V2-V3 | M13F-27F & M13R-357R | Roche 454 | Bacteria | Celerinatantimonas, Pseudomonas, Propionibacterium, Modestobacter, Rhodovibrio, Salinibacter | Medina et al. [42] |
Natural green | Nocellara Etnea | 16S rRNA | V3 | Probio_Uni & Probio_Rev | Illumina | Bacteria | (Former) Lactobacillus, Weissella, Enterobacter, Bacteroides, Faecalibacterium, Klebsiella, Raoultella | Vacalluzzo et al. [40] |
Spanish-style & natural green | Manzanilla | 16S rRNA | V3-V4 | PCR_1 F & PCR_1 R | Illumina | Bacteria | (Former) Lactobacillus, Alkalibacterium, Enterococcus, Serratia, Allidiomarina, Halomonas, Marinobacter, Pseudomonas | Ruiz-Barba et al. [43] |
ITS1-ITS2 | ITS1_KYO2_F & ITS2_KYO2_R | Yeast/Fungi | Saccharomyces, Pichia, Nakazawaea, Candida | |||||
Spanish-style | Hojiblanca | 16S rRNA | V3-V4 | Pro341F & Pro805R | Illumina | Bacteria | Vibrio, Marinilactibacillus, Lactiplantibacillus, Enterococcus, Secundilactobacillus, Loigolactobacillus, Amphibacillus, Pediococcus, Halolactibacillus, Weissella, Lentilactobacillus | Correa-Galeote et al. [44] |
Spanish-style & Greek-style | Halkidiki & Konservolia | 16S rRNA | V2-4-8 and V3-7-9 | Data not available | Ion Torrent | Bacteria | (Former) Lactobacillus, Pediococcus | Argyri et al. [45] |
ITS1-ITS2 | ITS1F & ITS2R | Yeast/Fungi | Pichia, Wickerhamomyces, Brettanomyces, Aureobasidium, Schwanniomyces | |||||
Greek-style | Bella di Cerignola | 16S rRNA | V1-V3 | 28F & 519R | Roche 454 | Bacteria | (Former) Lactobacillus, Hafnia, Methylobacterium, Propionibacterium, Clostridium | De Angelis et al. [46] |
Greek-style | Kalamata | 16S rRNA | V1-V3 | 27F & 519 R | Illumina | Bacteria | (Former) Lactobacillus, Leuconostoc, Celerinatantimonas, Propionibacterium, Pseudomonas, Staphylococcus, Enterobacter | Kazou et al. [37] |
ITS1-ITS2 | ITS1F & ITS2R | Yeast/Fungi | Pichia, Saccharomyces, Ogataea, Penicillium, Millerozyma, Engyodontium, Wickeramomyces | |||||
Greek-style | Nyons | 16S rRNA | V3-V4 | S-DBact-0341-b-S-17 & S-D-Bact-0785-a-A-21 | Illumina | Bacteria | Celerinatantimonas, Photobacterium, Marinobacterium, Halomonas, Iodomarina, Bacillus, Lactococcus, Leuconostoc, Lacticaseibacillus | Penland et al. [10] |
ITS2 | ITS3f & ITS4_Kyo1 | Yeast/Fungi | Citeromyces, Wickerhamomyces, Zygotorulaspora, Candida, Pichia | |||||
Greek-style | Kalamata | 16S rRNA | V3-V4 | S-D-Bact-0341- b-S-17 & S-D-Bact-0785-a-A-21 | llumina | Bacteria | (Former) Lactobacillus, Pediococcus, Curvibacter, Sphinghomonas, Pannonibacter, Phenylobacterium | Michailidou et al. [38] |
18S rRNA | V7-V8 | FR1 & FF390 | Yeast/Fungi | Pichia, Brettanomyces, Issatchenkia, Cladosporium, Saccharomyces, Candida | ||||
Greek-style & natural green | Taggiasca | 26S rRNA | D1 | NL4R & LS2-MF | Illumina | Yeast/Fungi | Aureobasidium, Cyteromyces, Wickeramomyces, Debaromyces, Pichia, Alternaria, Cystobasidium, Yamadazyma | Traina et al. [47] |
Greek-style &natural green | Cypriot, Kalamata, Picual | 16S rRNA | V3-V4 | V3 &V4 | Illumina | Bacteria | (Former) Lactobacillus, Lactiplantibacillus, Streptococcus, Lactococcus, Pediococcus, Salinivibrio, Weissella, Latilactobacillus, Staphylococcus | Kamilari et al. [48] |
ITS1 | BITS & B58S3 | Yeast/Fungi | Aspergillus, Botryosphaeria, Meyerozyma, Zygosaccharomyces, Saccharomyces, Geosmithia, Wickeromyces, Candida, Penicillium, Hanseniospora |
rRNA: ribosomal RNA
To the best of our knowledge, there have been no reports comparing the various regions for sequencing in the field of table olives microbiota research, unlike other scientific domains (e.g., gut microbiota [49], environmental water [50], pathogen detection [51], etc.). Kim et al. [52] evaluated partial sequences of the different regions of the 16S rRNA gene for the phylogenetic analysis of microbiomes and reported that the V1-V3 and the V4-V7 regions are more suitable for the analysis of archaea, whereas bacterial analysis should target the V1-V3 and the V1-V4 regions. After studying the suitability of the V1-V8 regions to distinguish pathogenic bacteria, Chakravorty et al. [51], concluded that certain hypervariable regions were more appropriate for the identification of specific genera. To name a few of their findings, although the V2 and V3 regions allowed the differentiation of the bacteria analyzed to genus level, V2 was more suitable for the differentiation among the Mycobacterium species, while V3 was more appropriate for the Haemophilus species. The investigation of the bacterial diversity of Lake Baikal’s water column and bottom sediment by Bukin et al. [50] indicated that the V2 and V3 regions allowed higher resolution for lower-rank genera and species in comparison to the V3 and V4 regions. However, both V2-V3 and V3-V4 hypervariable regions of the 16S rRNA gene allowed the assessment of the bacterial diversity during metataxonomic analysis. In a similar context, Chen et al. [53] compared the regions V1-V2, V3-V4 and V4 to select the most suitable for gut microbiota analysis. They reported that the V1-V2 region did not successfully detect the majority of the Bifidobacterium members, hence deeming it less suitable for this purpose. On the contrary, Kameoka et al. [49] employed an updated version of the primers used by Chen et al. [53], which improved the detection of the Bifidobacterium genus. In their study, although sequencing of the regions V1-V2 and V3-V4 allowed the successful detection of Bifidobacterium and Akkermansia genera, interestingly the selection of the V3-V4 region led to an overestimation of the actual abundance of these genera as revealed by quantitative real-time PCR. The primer set corresponding to the V1-V2 region was deemed the most suitable for this purpose. These findings indicate that besides the region selection, the specificity of the primers for the same region is also highly influential on the outcome of the analysis. Overall, it is reported that the hypervariable regions of the 16S rRNA gene have specific properties, hence the region of the gene to be sequenced is selected according to the aim of the experimental study [29]. Thus, the design and standardization of amplicon metagenomics workflows for the analysis of table olives microbial diversity seems to be critical to ensure the quality and interoperability of the generated datasets.
Shotgun metagenomics, commonly referred to as metagenomics and Whole Genome Shotgun metagenomics, is of untargeted nature lying on the sequencing of the entire metagenome, the complete set of a sample’s genetic material [21, 54]. Therefore, it enables the analysis of DNA and identification across all domains of microorganisms, such as bacteria, viruses, archaea, and eukaryotes [26, 29, 30]. The produced data give insights into the taxonomic composition and the functional properties of the genes of the microbial consortium of the sample [28]. As opposed to the targeted approach mentioned above, shotgun metagenomics is PCR independent, thus, exceeding the amplification bias amplicon metagenomics entail due to primer binding [21]. It requires, however, reference genomes for classification [26]. Furthermore, it is characterized by greater sensitivity, which facilitates taxonomic classification up to the strain level and allows the detection of unknown microorganisms in a sample manner in contrast to targeted sequencing [21, 28].
Another sequencing-based meta-omics approach is metatranscriptomics. This technology enables the untargeted sequencing of total messenger RNA (mRNA) found in a sample, creating a profile of the transcriptionally active microorganisms. Therefore, it identifies the viable and most active members of the microbial consortium, deepening the knowledge of the interactions taking place within the microbial community and providing information about their gene expression under a complex food ecosystem [2, 21]. Metatranscriptomics can also detect the RNA-based virome and hence foodborne RNA viruses, like norovirus [21]. However, mRNA is unstable and therefore additional technical precautions are needed [36].
Despite the fact that shotgun metagenomics and metatranscriptomics offer bigger amounts of information concerning the microbial diversity and the interactions among the microbial communities compared to amplicon metagenomics, the high cost, the challenges in library preparation and conduction of bioinformatic analysis prevents their application for the routine analysis of fermented foods [36]. Search conducted in the databases Scopus, Web of Science and PubMed using the keywords: “shotgun metagenomics”, “metagenomics”, “table olives”, “table olive fermentation” and “olive microbiota” (accessed on July 16 2024), without restrictions in the year of publication resulted in only one article employing shotgun metagenomics to study the microbiota of table olives. Soto-Giron et al. [55] revealed a great microbial diversity as well as previously uncultured genera from various vegetables, including brined green olives, using shotgun metagenomics with an aim to highlight the potential health benefits of plant microbes to the gut microbiota. Therefore, it can be concluded that the application of shotgun metagenomics in the field of table olives is still underexploited. In comparison to table olives, shotgun metagenomics appears to be constantly gaining ground on the study of other fermented foods, such as dairy [56, 57], vegetables [58, 59], fish [60], meat products [61], beverages like Pu-erh tea [62] and other food categories, such as miso [63]. Regarding metatranscriptomics, it has been applied to cheese, sourdough and wine focusing on the interactions within the microbial ecology [2]. The recent review by Tsoungos et al. [3] and our literature search in the same databases as mentioned above using “metatranscriptomics”, “transcriptomics”, “table olives”, “table olive fermentation” and “olive microbiota” (accessed on July 16 2024), pointed out that the employment of metatranscriptomics in table olives is still in its infancy. In particular, three related papers have been found regarding transcriptomics but not metatranscriptomics analysis on bacterial strains isolated from table olive fermentation. The studies of Alonso García et al. [64, 65] investigated the transcriptomic profile and potential probiotic properties of Lpb. pentosus strains, isolated from Aloreña green table olives. Additionally, López-García et al. [66] conducted transcriptomic analysis on the Lpb. pentosus LPG1 strain to investigate its differential gene expression during Spanish-style green table olive fermentations, emphasizing on its ability to adhere to the olive surface and form a non-pathogenic biofilm. Despite not involving the above keywords in their study, De Angelis et al. [46] extracted the total RNA along with the total DNA directly from olive and brine samples. This approach indicated that at the end of the fermentation of Bella di Ceringola olives Lpb. plantarum and Lpb. pentosus were the dominant and metabolically active microbial communities. Although the majority of phyla detected from DNA and RNA sequencing were common in both cases, differences were marked in their abundance.
Following the screening of the scientific literature limited results were produced concerning shotgun metagenomics and metatranscriptomics in comparison to the substantial abundance found for amplicon metagenomics analysis in table olive fermentation system. Based on these findings, this review will focus on amplicon metagenomics and its combined application with volatilomics to reveal relationships between the microbial community and the volatile compounds. On the following chapters emphasis will be given on the workflow of amplicon metagenomics to evaluate the microbial diversity in table olive fermentation system along with its bioinformatics workflow to analyze and interpret the amplicon metagenomic data of table olives microbiome. Both shotgun metagenomics [29] and metatranscriptomics [67], share common steps with the typical workflow of amplicon metagenomics and therefore appropriate comments will be made to address this and provide additional information when necessary.
Workflow of amplicon metagenomics for assessing microbial diversity in table olive fermentation system
An amplicon metagenomics experiment consists of several elemental steps which are presented in Figure 2. A typical workflow consists of sample collection (step 1), DNA extraction (step 2), Next Generation Sequencing (NGS) library preparation (step 3), followed by sequencing (step 4) and finally metagenomic data analysis (step 5), with the use of bioinformatics tools [28, 68]. Integration of the metagenomic data with other omics data can potentially follow (step 6).
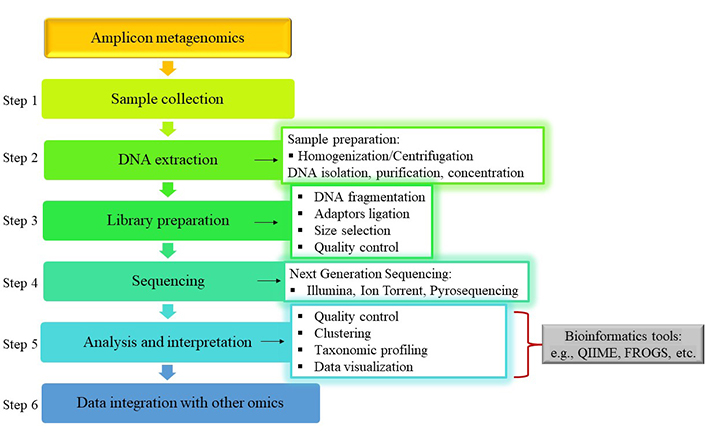
Typical workflow of amplicon metagenomic analysis for the evaluation of table olive microbial diversity. QIIME: Quantitative Insights Into Microbial Ecology; FROGS: Find Rapidly OTUs with Galaxy Solution. The olive and/or brine samples are collected and then prepared for the extraction of the DNA through homogenization and/or centrifugation. Library preparation is part of the pre-sequencing preparation encompassing four basic steps, DNA fragmentation, ligation of adaptors, size selection of ligated sequences and their quality control. Various sequencing platforms can be utilized for the execution of NGS, while bioinformatics tools are applied for the analysis of the metagenomic data. Afterwards, these data can be integrated with data from other omics technologies
To assist the extraction of high-quality DNA to be used for sequencing, the appropriate preparation of olive/brine samples is required. In particular, brine samples are centrifuged and the cell pellet is washed and then resuspended before DNA extraction. Similarly, olive samples are homogenized first and then centrifuged like the brine samples [10, 37, 39, 42, 45, 48]. Most studies employ commercially available kits for the DNA isolation and purification, which could also involve directions regarding the sample preparation. Various kits have been applied in the last few years for the DNA extraction from table olives and/or brine samples as presented by recent examples in Table 2. Throughout the entire process attention should be paid to the prevention of contamination from other DNA sources as well as to the elimination of inhibitors (e.g., phenolic compounds) for the DNA extraction [29]. Once the DNA is isolated and purified, its quality is evaluated. This involves evaluation for integrity through agarose gel electrophoresis and purification control with the use of a spectrophotometer by measuring the absorbance ratio A260/A280 and A260/A230. Quantification of the DNA (i.e., measurement of DNA concentration) can take place with the use of a photometer or fluorometer [37, 48]. In case of low concentration (e.g., < 5 ng/μL) or if contaminants are detected with the A260/A230 absorbance ratio, the resuspended pellet is treated with β-mercaptoethanol before the extraction of the DNA [48]. β-Mercaptoethanol (HOCH2CH2SH) is a strong reducing agent used to extract high quality DNA from plant-based samples such as table olives by removing phenolic compounds [69].
Presentation of various commercially available kits for DNA extraction, employed in table olives and brine microbial diversity research
Commercially available kit | Company-headquarters | References |
---|---|---|
DNeasy®PowerFood®Microbial Kit | Qiagen; Hilden, Germany | Kamilari et al. [48], Ruiz-Barba et al. [43] |
DNeasy®mericon® Food Kit | Qiagen; Hilden, Germany | Randazzo et al. [39], Vacalluzzo et al. [40] |
DNeasy®PowerSoil® Kit | Qiagen; Hilden, Germany | Kazou et al. [37] |
NucleoSpin® Soil Kit | Macherey-Nagel; Dueren, Germany | Penland et al. [10] |
NucleoSpin® Food Kit | Macherey-Nagel; Dueren, Germany | Argyri et al. [45] |
ZymoBIOMICS™ DNA Miniprep Kit | Zymo Research; Irvine, USA | Michailidou et al. [38] |
FastDNA™ Pro Soil-Direct Kit | MP Biomedicals; Illkrich, France | De Angelis et al. [46] |
The aim of the DNA library preparation is to process the DNA (or RNA) and ensure its suitability for the following sequencing step (step 3). In amplicon metagenomics the sequence of the target region is known (e.g., V3-V4 region of the 16S rRNA gene) and can be amplified through PCR with the use of specific primers. This produces amplicons of a specific size range. For the library preparation in shotgun metagenomics, purified DNA is cut into short fragments with the use of enzymes or mechanically, such as ultrasonication shearing and nebulization [28, 70]. In the case of metatranscriptomics, the RNA is first enriched, fragmented, converted to complementary DNA (cDNA) with the means of reverse transcriptase and then cDNA of desired size is produced through library preparation [67].
Following the step of fragmentation, adapter sequences (short, double stranded known sequences) are ligated to the fragments at the 3΄ and 5΄ ends. They function as binding sites for the primers to be used at amplification and interact with the sequencing platform by anchoring the DNA [28], for example they immobilize the DNA on the planar solid surface of a flow cell in the case of the Illumina sequencing platform, on ionized spheres in the case of Ion Torrent sequencing, or on micron-scale beads in the case of pyrosequencing [70]. The size of the DNA fragments that are ligated with the adapter sequences is selected through agarose gel electrophoresis or with the use of columns or magnetic beads [28, 70]. The final step of the library preparation is the Quantifying and quality control of the library with the use of a Bioanalyzer system or Quantitative real-time PCR, to evaluate the quality and length size of the DNA fragments as well as the concentration of the library [28].
The microbiome of fermented products, such as table olives microbiota is a remarkably complex system that requires modern technology to comprehensibly investigate its biodiversity in a representative way, as there are species found in different abundances within the sample. This can be achieved with the use of second and third generation sequencing technologies (step 4), comprising the NGS. Regarding the second generation, sequencing platforms such as Roche/454 pyrosequencing, MiSeq/HiSeq-Illumina platform and Ion Torrent sequencing technology seem to be the most widely used and yield millions of short reads (150–400 bp). Their function is based on the use of specifically labeled nucleotides or the detection of light or hydrogen [71, 72]. The third-generation sequencing includes the PacBio technology and Oxford Nanopore Technology that may lead to longer reads (6–20 kb), though with fewer reads per run [71], but does not require PCR amplification [72]. In the field of table olives research, Illumina appears to be the preferred sequencing technology, especially the MiSeq Illumina sequencing platform [37, 38, 40, 43, 45, 48, 73], followed by Ion Torrent [39, 45] and Roche 454 pyrosequencing [42, 46, 74].
Workflow of bioinformatics for analyzing and interpreting amplicon metagenomic data of table olives microbiome
The completion of the sequencing step generates a massive amount of data (sequence data reads) even in a single study, which need to be analyzed with the use of suitable bioinformatics tools, to transform the generated data into substantial information [28, 54]. Bioinformatics is an interdisciplinary science field that involves the application of computational tools in order to analyze and interpret biological data and is an essential tool for the management of these data [75]. A typical bioinformatics pipeline for the processing of amplicon metagenomic data after sequencing consists of the following basic steps, quality control of the reads, clustering, taxonomic profiling, and visualization of the data [29] (step 5 in Figure 2).
Firstly, quality control takes place to remove the low-quality reads from the generated data. In amplicon metagenomics, sequences (reads) are selected based on similarity and clustered into Operational Taxonomic Units (OTUs). The next step is the assignment of the OTUs to a taxonomic identity with the use of reference databases and the creation of phylogenetic tree [34, 76]. OTUs with 97% similarity are approximately classified at species level [10, 54]. Taxonomic profiling provides answers to the question “Who is there?” [54], offering insights into the taxonomic composition (microbial biodiversity) of the sample. Apart from identification, it also allows the estimation of the relative abundances of the microorganisms in the specific ecosystem [28]. In the case of shotgun metagenomics, quality control is followed by assembly and binning [29]. During assembling, contiguous sequences are constructed from the fragmented sequenced data, while binning clusters raw reads or contigs (after assembly) of similar species, assigning them to a group which represents a biological taxon [71]. Afterwards, the data can be used for gene prediction (identification of genes and hence putative proteins), as well as for gene annotation, which encompasses prediction of domains, functions and metabolic pathways involving the products of genes (functional profiling) through comparison to different databases [29, 71]. For metatranscriptomics analysis, after the reads are filtered, they can be aligned to reference sequences. In case of their unavailability, de novo assembly takes place. The steps that follow are annotation and statistical analysis [67]. The final step in metagenomic/metatranscriptomic data analysis is visualization, which enables the understanding of the microbial composition. Various bioinformatics tools have been developed for the facilitation of each step of the data analysis [29].
One of the most widely used software for analyzing and visualizing of amplicon metagenomic data, to unravel the microbial biodiversity in table olives is Quantitative Insights Into Microbial Ecology (QIIME, QIIME2) [38–40, 42, 46, 48, 77] with the employment of various third party tools. The QIIME pipeline includes processing of the sequenced data by performing quality control, denoising, clustering, taxonomy assignment and visualization of the amplicon metagenomic data, through generation of Unweighted Pair Group Method with Arithmetic mean (UPGMA) and principal coordinates analysis (PCoA) plots that allow the visual discrimination between the samples [76]. Another popular bioinformatics pipeline in the table olives field is Find Rapidly OTUs with Galaxy Solution (FROGS) [10, 78], which can analyze large amounts of amplicon sequences, performs quality control, generates tables of OTUs and performs taxonomic assignment to the species level (taxonomic affiliation). It includes the Swarm algorithm as clustering tool and VSEARCH as Chimera removal tool [79]. Other widely used tools in amplicon metagenomics for the sequence filtering, clustering, and quantification of the data in table olive research include the Mothur software [44], VSEARCH [38, 78], DADA2 [40], the Swarm algorithm [10], USEARCH [46, 74], Uparse [10] and Deblur [29]. For the statistical and phylogenetic analysis, as well as visualization of sequencing data already clustered into OTUs, the software tool, R phyloseq package within the R environment [80] has also been applied in the investigation of microbial diversity in table olives [10, 38, 81].
Open access databases that have been developed for amplicon metagenomic analysis have been used for the investigation of the microbial community of table olives, through taxonomic assignment of OTUs, comprise of the SILVA database [38, 42, 77], Greengenes [48], GenBank [46], National Center for Biotechnology Information (NCBI) [37, 44, 74], Recombination Detection Program (RDP) [73] for the 16S rRNA sequences and the UNITE fungal classification database for the ITS datasets [10, 40, 48, 73, 78]. One of the most frequently used tools for the taxonomic assignment of the OTUs against the reference databases is the Nucleotide Basic Local Alignment Search Tool (BLAST) algorithm [10, 37, 46, 77].
Microbial volatilome of fermented foods
Metabolomics can be defined as the comprehensive analysis that leads to the global profiling of metabolites in a biological sample [82]. Its application lies in the determination and quantification of intracellular low molecular-mass compounds (< 1,500 Da) that are produced through the metabolism of living organisms such as microorganisms [83]. The omics approach that focuses on the qualitative and quantitative characterization of the volatile metabolites is defined as volatilomics [84, 85]. The complete set of volatile compounds found in an ecosystem or food matrix, including those produced during the microbial metabolism comprises the volatilome [85]. Microorganisms produce various metabolites, a great fraction of which are the volatile organic and compounds (VOCs) [84]. Main characteristics of VOCs are low solubility in water and high vapor pressure ≥ 10 Pa. The microbial volatilome is influenced by various factors among which are the growth stage of the microorganism, environmental conditions (temperature, pressure, humidity), interactions with other organisms in their ecosystem [86]. According to the opinion paper by Meredith and Tfaily [84], current microbial research on metabolomics primarily investigates the nonvolatile organic metabolites profile, dismissing the VOCs, characterizing them as a missing part of the metabolome. Therefore, the comprehensive understanding of a microbial system’s metabolic profile remains only partially explored.
Applications of volatilomics can be found in the domain of food safety, food quality and food authenticity where volatilome analysis can provide insights into the sensory, chemical, and even nutritional quality of a variety of food products (meat, fruits, vegetables, spices, fermented foods) [85]. The complex formulation of flavor (aroma and taste) consists of a wide range of VOCs, such as acids, aldehydes, ketones, alcohols, esters, and hydrocarbons [87]. Analyzing the flavor compounds allows the objectification of food flavor quality and provides the means for its improvement [88]. Moreover, the growing region, the biological origin and storage conditions of the food matrix, could render the volatile profile discriminative for each foodstuff. Therefore, the volatilomics approach could be a useful tool in the identification of specific compounds, acting as biomarkers on the food quality and authenticity evaluation [85]. In the field of table olives, volatilomic analysis can not only be applied to identify compounds related to the flavor of the product, but also to investigate how the volatilome is affected by chemical and enzymatic conversions that take place during fermentation [3]. Table olives, as all fermented products, encompass a variety of VOCs, mainly products of the microbial metabolism, whose composition directly influences the aroma and consumer’s acceptance of the final product [85, 89]. Furthermore, odor formation is considered to establish the shelf life [85] and is employed as a quality index for table olives [90].
Workflow of microbial volatilome analysis in table olive fermentation system
Volatilomics technology has been widely implemented in the field of table olives research. However, its combination with other omics techniques is less frequent. In the following part of this review, emphasis will be given on the studies integrating volatilomic and amplicon metagenomic data (multi-omic approach). The success of the volatilome analysis lies heavily on the appropriate preparation of the olive and brine samples. Common processes applied are freeze drying, treatment with liquid nitrogen, grinding, homogenization and filtering [10, 46, 73, 89]. The addition of NaCl (30 % w/v) in the brine samples and in the homogenized olive samples increases the extraction rate of volatile compounds [39, 90–92]. Attention should also be paid to the extraction of the volatile compounds so that they are compatible with the analytical platform used. The extraction techniques and techniques for compound detection described below are focusing on the volatilomics evaluation of table olives microbiota and the complete typical workflow is depicted in Figure 3.
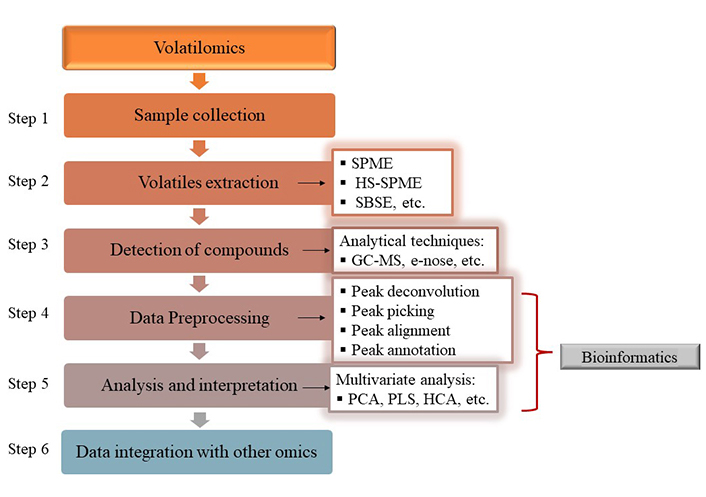
Typical workflow of microbial volatilome analysis in table olive fermentation system. SPME: solid-phase microextraction; HS-SPME: headspace-SPME; SBSE: stir-bar sorptive extraction; GC-MS: gas chromatography-mass spectrometry; PCA: principal component analysis; PLS: partial least squares; HCA: hierarchical cluster analysis. The main purpose of the preparation of the sample is the extraction of the volatile compounds, which are most frequently detected with the headspace solid-phase microextraction (HS-SPME), followed by GC-MS technique in table olive research. The data are then transformed in suitable formats for further data analysis with the use of appropriate bioinformatics tools and finally, integration with data from other omics technologies, such as metagenomics can take place
For the extraction of volatile compounds (step 2), techniques that isolate the compounds from the vapor phase above the food sample (headspace techniques) are usually applied such as static headspace and dynamic headspace, as well as solid-phase microextraction (SPME) [93, 94]. Headspace (HS) techniques are fast, allow volatilomics analysis from various matrices and the clean-up step can be omitted. One of the most commonly used techniques is HS-SPME, applied in various matrices including table olives [43, 89, 90, 92, 95–97]. It is characterized by simplicity, does not involve solvent extraction and is applied for the analysis of volatiles in the vapor phase of the sample [98]. Another solventless extraction strategy applied in this field is sequential sorptive extraction. The latter consists of the stir-bar sorptive extraction (SBSE) for semi volatile compounds followed by headspace sorptive extraction (HSSE) for highly volatile compounds [90, 91]. Furthermore, SBSE exhibits higher sensitivity than SPME thanks to a bigger sorbent phase volume. Nevertheless, SPME can be accomplished in a fully automated way and the solid phase used can be selected according to the chemical features of the metabolites to be analyzed [98].
After the extraction has taken place, the volatile compounds are separated through gas chromatography (GC) and then are usually analyzed in a MS, for their detection and identification [99] (step 3). The most frequently chosen technique for the analysis of volatiles is GC followed by mass spectrometry (GC-MS) [100]. A widely used analytical strategy for the determination of volatile compounds in table olive research and in the food field in general is the combination of SPME and/or HS-SPME with GC-MS [39, 46, 89, 101]. The combination of these techniques can lead to both the qualitative and quantitative analysis of individual volatile compounds found in a complex mixture [85, 98]. The GC-MS technology provides insights into the overall aroma profile of a sample and is characterized for its reproducible results. Its potential for high level separation and identification of individual compounds is aided due to extensive libraries of mass spectral data [98, 100]. It also produces a “spectral fingerprint” of the volatile and semi-volatile compounds that can even be evaluated for food authentication purposes [98].
An emerging machine olfaction technology, the electronic nose (e-nose) is also being applied to volatilomics research in table olives. It consists of powerful electromechanical sensors that simulate the biological olfactory that can discriminate the aroma profiles of different food matrices [100, 102]. The volatiles detected can be used to identify and investigate the changes in the quality of products, due to their unique composition. In comparison to GC-MS, it offers real-time monitoring of the quality [100]. Sánchez et al. [102] developed a protocol based on the e-nose technology, by which they detected olfactory defects derived from abnormal fermentations such as zapatera, butyric and putrid spoilage during the fermentation of Spanish‐style green olives. This approach could potentially be applied for the monitoring of the fermentation process to prevent an abnormal fermentation and ensure a final product of high quality. The e-nose was also applied by Sánchez et al. [103] for the discrimination of defective olives inoculated with mold strains, as an alternative to traditional sensory evaluation analysis. Machine olfaction proved to be a satisfactory approach to discriminate the olives according to their health-status as well as the volatiles produced during the fermentation of olives inoculated with different mold strains.
Workflow of bioinformatics for analysing and interpreting volatilomics data of table olives microbiome
Volatilomics, just like metagenomics, is associated with the generation of extensive datasets. The management of volatilomic data requires efficient processing [85, 100]. Bioinformatics is the essential tool for the management of the GC-MS data. The bioinformatics workflow for the interpretation of volatilomics data could be summarized into two primary stages, the preprocessing, where feature extraction, alignment, annotation, and the use of spectral databases take place and the analysis and interpretation stage, in the course of which statistical analysis and integration with other data types are conducted [99].
Once the data have been acquired, pre-processing takes place (step 4 in Figure 3) with the aid of specialized software and online platforms [85, 100]. This step involves the clean-up and transformation of the raw data produced in suitable format to be used in the later data analysis procedure [98, 104]. The basic requirements for this step encompass peak deconvolution, peak picking, and peak alignment [105]. More specifically, peak deconvolution leads to the resolving of the overlapping of co-eluted peaks in the case of a GC-MS chromatograph, using MS as the second dimension. Peak picking involves the selection of the peak that represents the signal of the metabolite in the sample. During peak alignment the retention time shifts are corrected and the chromatographs are matched so that each signal has the same retention time in each sample. Frequently, the annotation process that follows overlaps with the data preprocessing procedure [99, 105]. Metabolite annotation is characterized as the appointing of the tentative metabolites to the signal, by matching their masses according to databases or entries in libraries to lead to a list of candidate matches [106].
Software that has been reported for the use of data preprocessing of volatilomics data deriving from the fermentation of table olives include the MassHunter [107], PerkinElmer Turbomass, XCMS [10], GC/MSD ChemStation [96], MSWS [95], while it is also not uncommon to use the instrument data system [43]. The identification of volatile compounds in table olive fermentation is usually accomplished based on mass spectra matching with the standard National Institute of Standards and Technology (NIST) Mass Spectral Library and on the comparison of retention indices (RI) sourced from NIST Standard Reference [10, 39, 40, 43, 46, 95, 97, 107].
Further analysis of the processed volatilomics data to identify statistically significant metabolites (step 5 in Figure 3) usually takes place with the application of multivariate statistical analysis [85, 104], most frequently after the application of one-way analysis of variance (ANOVA). The most common methods used to analyze the volatilomics data deriving from table olive fermentation include principal component analysis (PCA) [43, 95–97, 102, 108], partial least squares (PLS) discrimination analysis [91, 97, 102], hierarchical cluster analysis (HCA) [97], through the utilization of appropriate software [99]. The most frequently reported are the SIMCA-P [95, 97, 100], XLSTAT [91, 92, 101], STATISTICA [46, 96, 108, 109] and Matlab software [101, 102].
The last step of the volatilomics analysis workflow is the integration of volatilomics data with other omics data (step 6 in Figures 2 and 3) such as metagenomics, metaproteomics, etc. [104]. Since individually, each omic technology describes a part of a fermentation system, the combination of different omics data can investigate the interactions among the various parts of the system at the molecular level [99], as well as offer a reliable and comprehensive view of the metabolic changes that take place [104].
Integrated metagenomic and volatilomic analysis in table olive research
In the past few years various research papers have revealed strong relationships between the microbial volatile profile and the microbial composition of table olive fermentation system (brine and olives) employing conventional culture-dependent techniques and/or culture-independent techniques during controlled and spontaneous fermentations (Table 3).
Positive correlations (P < 0.05) between the microbiota composition and the microbial volatilome of fermented table olives
Processing method | Olive cultivar | Starter culture strain/indigenous microbiota | Volatile organic compounds in olives and/or brines | References |
---|---|---|---|---|
Greek-style | Bella di Cerignola | (Former) Lactobacillus plantarum starter | Ethyl acetate, ethanol, acetic acid, D-limonene | De Angelis et al. [46] |
Combination of (former) Lactobacillus plantarum starter, Wickerhamomyces anomalus DiSSPA73 | Ethyl acetate, ethanol, acetic acid, isobutanol, isoamyl and amyl alcohols | |||
Combination of (former) Lactobacillus plantarum starter, Wickerhamomyces anomalus DiSSPA73, Lactobacillus plantarum DiSSPA1A7, Lactobacillus pentosus DiSSPA7 | Ethyl acetate, ethanol, acetic acid, D-limonene | |||
Natural green | Nocellara Etnea | Proteobacteria | Octanal, nonanal, decanal, 1-nonanol, 1-undecanol, 1-dodecanol, 1-octanol | Randazzo et al. [39] |
(Former) Lactobacillus paracollinoides, (former) Lactobacillus acidipiscis, Pediococcus parvulus | Ethyl-acetate, ethyl-propanoate, ethyl-butanoate, ethyl-lactate, ethyl-benzoate, butanoic acid 2-methylester, butanoic-acid 3-methylester, ethyl decanoate | |||
(Former) Lactobacillus acidipiscis | Acetic acid | |||
Spanish-style | Gordal &Manzanilla | Propionibacterium | Acetic acid, propionic acid, succinic acid, methyl propanoate | de Castro et al. [73] |
Ruminococcus | Propionic acid, butyric acid | |||
(Former) Lactobacillus | (Z)-3-hexen-1-ol, propyl acetate, 3-ethylpyridine | |||
Corynebacterium | 2-Butanol, benzyl, pentanoate, p-cresol, linalool, 3-ethyl-4-methylpyridine | |||
Enterobacteriaceae | 2-Butanol, ethyl hexanoate, p-ethyl guaiacol, 4-ethyl phenol, 3-ethylpyridine, 3-ethyl-4-methylpyridine, 1,4-dimethoxybenzene | |||
Cardiobacteriaceae | Butyric acid, valeric acid, caproic acid, 1-octanol, methyl pentanoate, methyl hexanoate, p-cresol | |||
Candida apicola, Candida etchellsii | Lactic acid, 3,4-dimethylbenzaldehyde | |||
Candida pararugosa | Methyl heptanoate | |||
Pichia membranifaciens | α-Terpineol | |||
Dipodascaceae | Butanoic acid, nonanal, p-cresol, dimethyl sulfide | |||
Natural green | Nocellara Etnea | Combination of Lactobacillus plantarum F3 and Lactobacillus paracasei N24 | Phenylethyl alcohol, methyl 2-methylbutanoate | Pino et al. [101] |
Spanish-style | Manzanilla | Wickerhamomyces anomalus Y12 | 1-Butanol, ethanol, methyl acetate, 2-phenylethyl acetate, ethyl acetate, 2-methyl-1-butanol | Benítez-Cabello et al. [91] |
Spanish-style | Manzanilla | Propionibacterium | Propanoic acid | de Castro et al. [92] |
Lactobacillus pentosus LP99 | 4-Ethylphenol, 1-heptanol, 6-methyl-5-hepten-2-ol, isoamyl acetate, methyl benzoate | |||
Natural black | Nyons | Pichia membranifaciens | Ethyl propanoate, 3-methylacetate, ethyl nonanoate, 2-phenylethylacetate, methyl-3-phenylproapanoate, heptan-2-one, pentan-2-one, 3-methylbutan-1-ol | Penland et al. [10] |
Celerinatantimonas diazotrophica | Hexan-1-ol, methyl hexanoate, phenol | |||
Candida boidinii | Ethyl 2-phenylacetate, ethyl 2-hydroxybenzoate | |||
Priceomyces carsonii | Ethyl(2S)-2-hydroxypropanoate, 1,2-dimethyoxybenzene, ethyl 2-hydroxybenzoate, phenylmethanol | |||
Aureobasidium pullulans | Nonanal, benzaldehyde | |||
Candida boidinii | Ethyl 2-phenylacetate, ethyl 2-hydroxybenzoate, ethyl(2S)-2-hydroxypropanoate, 1,2-dimethyoxybenzene, ethyl 2-hydroxybenzoate, phenylmethanol | |||
Marinobacterium litorale | Methyl octanoate, ethyl octanoate, ethyl-2-phenylactete, ethyl 3-phenylpropanoate, heptan-2-one | |||
Natural green | Nocellara Etnea | Lactobacillus pentosus LPG1 | 2-Phenylethyl acetate, cis-2-penten-1-ol, 2-methyl-3-hexanol, 1-butanol | Garrido-Fernández et al. [90] |
Lactobacillus plantarum Lpl15 | Methanol, 1-butanol, 4-ethyl phenol | |||
Lactobacillus pentosus Lp13 | 1-Butanol | |||
Wickerhamomyces anomalus Y12 | 2-Phenylethyl acetate, cis-2-Penten-1-ol, 2-methyl-3-hexanol | |||
Natural green | Manzanilla | Candida adriatica NC168.4 | Ethyl acetate, ethanol, theaspirane, (Z)-3-hexen-1-ol, acetic acid | Montaño et al. [107] |
Candida boidinii NC168.5 | Ethanol, isopentanol, acetic acid, isopentyl acetate, isobutanol | |||
Zygotorulaspora mrakii NC168.2 | Ethanol, isopentanol, dimethyl sulfide, ethyl acetate, acetaldehyde | |||
Nakazawaea molendinolei NC168.1 | Ethanol, ethyl acetate, phenylethyl alcohol, isobutanol, (Z)-3-hexen-1-ol | |||
Pichia manshurica NC168.3 | Dimethyl sulfide, acetic acid, isopentanol, 2-methylbutanoic acid | |||
Natural green | Nocellara Etnea | (Former) Lactobacillus | Ethyl acetate, acetic acid methyl ester, lactic acid ethyl ester, 2-butanone-3-hydroxy, 3-methyl-1-butanol acetate, 2-methyl ester | Vacalluzzo et al. [40] |
Yeasts | Benzoic acid and 2-hydroxy-ethyl ester | |||
Weisella | 1-Octanol, 3,7-dimethyl-muurolene, phenol, 4-ethyl-2-methoxy | |||
Enterobacteriaceae | Ethanol, 1-hexanol, butanoic acid ethyl ester, hexanoic acid, ethyl ester, benzoic acid 2-hydroxy ethyl ester, hexanal, benzeneacetaldehyde dimethyl sulfide | |||
Spanish-style | Chalkidiki | Yeasts | 3-Methyl-1-butanol | Alvanoudi et al. [95] |
LAB | 2-Butanol | |||
Spanish-style | Manzanilla | (Former) Lactobacillus | Acetic acid, butanoic acid, nonanoic acid, benzoic acid, methyl lactate, methyl acetate, ethyl acetate, ethyl 3-methylbutanoate, ethyl 2-hydroxyisovalerat, 1-heptanol | Ruiz-Barba et al. [43] |
Saccharomyces | Octanoic, decanoic acids | |||
Pichia manshurica | Butanoic acid, benzoic acid, isopentanol, decanol | |||
Natural green | Pichia manshurica, Aliidiomarina, Nakazawaea | Carbitol, ethyl phenylacetate, α-terpineol, phenol, 2,3-dihydrobenzofuran | ||
Halomonas | 2-Phenylethyl acetate 1-decanol | |||
Saccharomyces | (Z)-3-hexen-1-ol, 3-octanol, (Z)-3-hexenyl acetate, limonene | |||
Natural green | Gordal | (Former) Lactobacillus and Pediococcus | Isobutanoic, hydrocinnamic and acetic acids, 2-pentanol, acetoin, 4-ethylphenol, creosol | Ruiz-Barba et al. [110] |
Manzanilla & Hojiblanca | Idiomarina | Isobutanol, ethyl octanoate | ||
Halomonas, Marinobacter, Aliidiomarina | 1-Propanol, 1-butanol, 1-heptanol | |||
Natural black &natural green | Taggiasca | Aureobasidium pullulans | Tridecane | Traina et al. [47] |
Wickerhamomyces anomalus | 1-Heptanol | |||
Aureobasidium pullulans, Aureobasidium protae, Wickerhamomyces anomalus | Benzaldehyde | |||
Vishniacozyma carnescens | Trichloromethane | |||
Cystobasidium minutum, Pichia spp., Yamadazyma diddensiae | 1-Heptanol and 3-methyl-benzaldehyde | |||
Yamadazyma diddensiae | Nonanoic acid |
Significant (P < 0.05) positive correlations between the microbiota (innoculum strains and/or indigenous microbiota) and the volatile metabolites of microbial origin are presented. LAB: lactic acid bacteria
The employment of a volatilomics approach could facilitate the comprehension of the factors (i.e., NaCl content in brine) shaping the complex concept of spontaneous fermentation of table olives and identify volatile biomarkers related to the population dynamics of viable-cultivable LAB and yeasts by selective plating methodology during the fermentation stages, as reported by Alvanoudi et al. [95]. The authors highlighted that future studies focusing on the species-level identification of the microbial diversity could determine whether/which species are linked to VOCs. In the scientific literature culture-dependent techniques have been applied to confirm the viability of single- and multi-strain starter cultures throughout the controlled fermentation processes [91, 101, 107, 109]. In the majority of those studies, the relationship between starter cultures and their VOCs that facilitate the enhancement of the flavor profile of the final products is highlighted. Despite all the interesting remarks mentioned (e.g., detection of potent biomarkers for monitoring of the fermentation [95], discrimination of fermentation stages [109]) the application of culture-dependent techniques did not allow the exploration of the contribution of the background microbiota (indigenous strains) to the VOCs.
Unraveling microbial composition and dynamics during table olive fermentation
The application of metagenomics has taken things a step further, allowing a more comprehensive understanding of the microbial ecology and a holistic monitoring of the fermentation process, exceeding the limitations of the culture-dependent approaches applied in the papers mentioned in the above section. The following studies combine amplicon metagenomics with volatilomic analysis to investigate the dynamics of microbial composition of table olives and association with the quality attributes of the final products. Thus, a deeper understanding of the fermentation system can be achieved and the findings could open the possibility to design starter cultures for the tailored production of VOCs, hence providing the ability to model the flavor and quality aspects of table olives [90, 91]. Details regarding the positive correlations between the microorganisms and the VOCs are thoroughly presented in Table 3.
De Angelis et al. [46] evaluated the effect of starter cultures to improve the fermentation process and organoleptic properties of Greek-style Bella di Cerignola table olives. Their study is the first to employ sequencing based meta-omics technologies to explore the occurrence of specific starter cultures on the microbial system of table olives. This allowed the detection of genus Hafnia, not previously detected in table olives and specifically in this cultivar. This genus was found in high abundance in the raw olive fruit and became dominant in the uninoculated samples after one day of fermentation. Moreover, a simplified microbial profile was revealed in the inoculated samples, attributing antimicrobial activities in the starter culture strains. On the contrary, the uninoculated sample exhibited higher abundances of the Enterobacteriaceae family and the genera Propionibacterium and Clostridium at the end of the fermentation that were associated with high levels of propionic acid. The study of Randazzo et al. [39] focused on the bacterial community dynamics during the fermentation of natural green Nocellara Etnea table olives and its impact on the formation of VOCs. The species (former) L. acidipiscis was uncovered for the first time through amplicon metagenomics in brine samples. A rare species in green table olive fermentation of Spanish and Italian cultivars, P. parvulus, along with the species (former) L. paracollinoides and the genus Hafnia were also reported during fermentation. All the above microorganisms were associated with the production of esters attributing fruity and floral notes (e.g., ethyl acetate, ethyl-propanoate, ethyl-butanoate, etc.). Vacalluzzo et al. [40] investigated the impact of spontaneous and controlled (selected L. plantarum strains) fermentation also of natural green Nocellara Etnea table olives on their microbial and VOCs profile. In the inoculated samples the dominance of the (former) Lactobacillus genus was unveiled by amplicon metagenomics, while in uninoculated samples it revealed a high abundance of the Enterobacter genus persisting throughout the fermentation. On the other hand, culture-dependent microbiological analysis accentuated their total inhibition in the final product. The compounds 3-methyl-1-butanol acetate and the 2-methyl ester which are associated with desirable flavors, were primarily detected in the inoculated samples. The research of Penland et al. [10] focused on the monitoring of the evolution of the naturally black Nyon table olives microbiota, regarding the final product’s organoleptic properties. Although culture-dependent analysis disregarded the occurrence of C. diazotrophica, the amplicon metagenomics approach revealed its dominance from the beginning till the end of the fermentation, which presented strong correlations with various VOCs (Table 3) and interestingly with lactic acid. The dominant yeast species P. membranifaciens and C. boidinii exhibited significant correlations with various VOCs, attributing fruity and flowery notes and are overall considered to majorly contribute to the aroma of black table olives. Ruiz-Barba et al. [43] combined amplicon metagenomic and volatilomic analysis along the spontaneous fermentation of cv. Manzanilla table olives, processed according to the Spanish and natural style in parallel. They reported significant differences in each microbial profile, which in turn affected the volatilome of the fermentation systems. The microbiota was associated with aroma-active VOCs, exhibiting among others fruity, flowery, and green notes. A noteworthy observation is that although Saccharomyces and P. manshurica appeared in high abundance in both processing types, their correlations with volatile compounds formed were distinctively different as presented in Table 3. This finding can probably be attributed to interactions with other members of the fermentation system. Recently, the same group of researchers, Ruiz-Barba et al. [110] employed the same approach to investigate the effect of indigenous yeast strains as starter culture on the controlled fermentation of natural green olives, cv. Gordal, Manzanilla and Hojiblanca. The viability of the yeast strains throughout the fermentation was confirmed with amplicon metagenomics. This approach also revealed a high abundance of the genera Halomonas, Marinobacter, Aliidiomarina and Idiomarina in both fermentation systems, in contrast to the culture-dependent approach that failed to detect them. The genus Idiomarina exhibited important correlations with isobutanol and ethyl octanoate, while the former genera were significantly correlated with 1-propanol, 1-butanol and 1-heptanol. Unlike all the above-mentioned studies, Traina et al. [47] focused exclusively on the monitoring of the yeast ecology of spontaneously fermented Greek-style Taggiasca table olives, highlighting their contribution to the organoleptic profile of the final product through associations with various VOCs. Although culture-dependent techniques revealed the microbial diversity at a satisfying level (identification of 13 yeast/fungi species), amplicon metagenomics led to an even deeper knowledge of the microbiota (5 genera and 25 yeast/fungi species), that also exhibited significant correlations with VOCs (Table 3). Such is the case of Y. diddensiae, reported for the first time in fermented olives, yet it was strongly correlated with nonanoic acid in brine samples. Evidence also suggested its possible contribution to the acidification of the brine.
These papers confirm the potential of omics technologies to exceed the limitations of culture-dependent techniques. It was highlighted that they allowed a deeper investigation of the microbiota during fermentation that could otherwise remain underexplored and turned out to contribute substantially to the sensory quality of the final product. The fact that Vacalluzzo et al. [40] detected the Enterobacteriaceae family only through amplicon metagenomics and not by culture-dependent techniques was attributed to the fact that culture-independent techniques cannot distinguish alive and from dead microorganisms. This finding is in agreement with Kazou et al. [37] who highlight that the low pH values of brines and olives ensure the safety of the final product, therefore supporting the absence of culturable enterobacteria. They also highlight that culture-independent techniques lead to the amplification of the DNA of both active and dead or compromised cells, a disadvantage of these techniques. Furthermore, it is noteworthy that culture-independent techniques do not facilitate the isolation and selection of autochthonous strains for starter cultures with an active role during fermentation Botta & Cocolin [17]. Therefore, many authors [37, 40, 47] highlight the significance of employing a dual approach, incorporating culture-dependent and independent techniques, to investigate such an intricate microbial ecosystem. Another beneficial approach would be employing a multi-omics strategy which will also include metatranscriptomics. The DNA is notably stable in the environment, unlike RNA that can be found only in actively growing cells. While the DNA allows the investigation of the microbial diversity, analysis of the RNA indicates the metabolically active part of this microbial community which in return is the one contributing to the fermentation process [111]. As previously mentioned, this approach was employed by De Angelis et al. [46], resulting in the differentiation of the microbiota based on the total bacteria detected (DNA sequencing) and the metabolically active ones (RNA sequencing).
Highlighting the spoilage- and authentication-related microbial and volatile profile of table olives
Microbial spoilage in table olives during uncontrolled and abnormal fermentation has a direct impact on the chemical diversity of the VOCs of the final product. There are three primary malodorous spoilage types, the zapatera spoilage, the butyric and the putrid spoilage relating to VOCs produced mainly by bacteria of the genus Clostridium and Propionibacterium [7]. Besides certain major volatile compounds that have been associated with each type of microbial spoilage, other volatile metabolites could also be responsible that have yet to be identified. Therefore, a deeper understanding of the microbial composition and volatilomic profile of table olives could provide a better insight into each type of spoilage [73]. In this scope, de Castro et al. [73] combined amplicon metagenomic and volatilomic technologies to evaluate the microbial and volatile profile of spoiled Spanish-style olives cv. Gordal and Manzanilla. Their results indicate correlations between the genus Propionibacterium with volatiles (e.g., acetic acid, propionic acid, methyl propanoate, etc.) contributing to the zapatera spoilage descriptor. Although the genus Ruminococcus exhibited marginally significant correlations with propionic and butyric acids, it was strongly associated with the butyric spoilage descriptor as revealed by statistical analysis.
Food authenticity has become an issue of significant attention globally, with the misleading geographic origin to be one of the most popular types of fraud. Within the European Union, the institution of protected geographical indication (PGI) and protected designation of origin (PDO) are essential tools for the protection and assurance of economically significant products, such as table olives [112]. To the best of our knowledge, no paper combining amplicon metagenomics and volatilomics for the discrimination of table olives based on the growing region or cultivar has been published so far. However, single omics approaches have been employed. Within this context, Argyri et al. [45] investigated the premise that the diversity of the microbiota of table olives is associated with biogeographic patterns through the application of amplicon metagenomic analysis. They reported differences in the microbial composition between table olives cv. Konservolia grown in Magnesia and Fthiotida, as well as cv. Chalkidiki from Chalkidiki region and Kavala, leading to a distinctive discrimination between olives of the same cv., but of different geographical origin. Similarly, amplicon metagenomics was also implemented by Kazou et al. [37] to differentiate cv. Kalamata grown in two different regions, Aitoloakarnania and Messinia/Lakonia. In both cases the dominant genera (Lactobacillus, Celerinatantimonas, Propionibacterium, etc.) were common but in different abundances according to the growing region. Unique genera for each case were also detected but in considerably lower abundances. In some cases though, the sole approach of amplicon metagenomic analysis for a discrimination based on the geographical region is not adequate. Kamilari et al. [48] did not report a sufficient discrimination among cv. Cypriot, Kalamata and Picual, from different geographical regions based on amplicon metagenomic analysis, as all the samples exhibited a similar bacterial profile. However, a wide yeast and fungal diversity was reported among the three varieties. Another interesting study is that of Mikrou et al. [113] who investigated the volatile profile of table olives from cv. Kalamata, Konservolia and Chalkidiki, grown in different geographical regions implementing only the volatilomic approach. Firstly, they reported noticeable differences among olive cultivars that allowed their discrimination. The main differences among the samples based on the olive cultivar was attributed to the higher concentration of trans-β-ocimene and ethanol for cv. Kalamata, of α-muurolene and α-farnesene for cv. Konservolia and of guaiacol and 4-methylguaiacol for cv. Halkidiki. Similarly, differences in the VOCs among olives of the same cultivar, led to their discrimination based on growing region.
Despite the successful discrimination of table olives solely by amplicon metagenomics achieved by Argyri et al. [45] and Kazou et al. [37] and by volatilomics by Mikrou et al. [113], the importance of integrating the volatile with the microbial profile of table olives through multi-omics technologies in future studies is highlighted. The application of advanced technologies could offer a comprehensive understanding of the microbial composition of table olives and assist in deciphering the development of different organoleptic properties among olives grown in different locations, thus enhancing their PDO or PGI character and significantly endorsing the local economy [48]. Moreover, it is commonly agreed that further studies are needed, that will include comparison among different batches, growing regions, cultivars, and treatments to eliminate false results relate d to the biological variability among the samples [37, 48, 85]. Figure 4 summarizes the potential applications of the integration of metagenomic and volatilomic data in table olives as were reported in all of the above-mentioned papers.
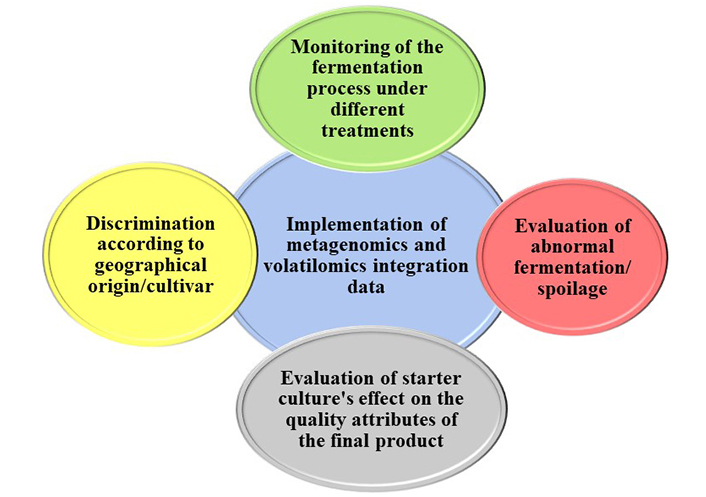
Potential applications of the implementation of amplicon metagenomic and volatilomic analysis in table olives fermentation
Conclusions and future perspectives
In this review, we presented, a collection of studies combining amplicon metagenomics along with volatilomics concerning table olive research to date. The workflow of each of the above omics technology was presented along with the usual bioinformatics pipeline used in table olives. Evident became the variations in various steps of the workflow, e.g., the sequencing of different regions of the 16S rRNA gene to unravel the microbial diversity of table olives. Further research comparing and detecting the most suitable region could lead to the establishment of a standardized workflow to uphold the quality and interoperability of the generated amplicon metagenomic data. Such a consistent pipeline could also be applied to volatilomics, up to the bioinformatics section of the analysis to achieve the same purpose. Furthermore, the combined strategy of amplicon metagenomics and volatilomics revealed the relationships between the microbiota composition and the microbial volatilome. The reported studies achieved to evaluate the influence of starter cultures and/or of the indigenous microbiota on the volatiles profile of table olives, by demonstrating significant changes in the VOCs pattern and resulting in an enriched flavor profile. Potential implementations of this approach could also include monitoring of the fermentation process, designing of starter cultures to model the quality attributes of the final product and assessment of the microbiological quality (e.g., spoilage microorganisms), through the discovery of biomarkers (VOCs) indicative of the presence of specific microbes. Despite the fundamental importance of sequencing-based meta-omics techniques as highlighted in the papers mentioned in this review, the combination of culture-independent along with culture-dependent techniques is crucial for a deeper investigation of the microbial ecology including both viable and non-viable populations.
Both amplicon metagenomics and volatilomics technologies indicated potentials to be used for the promotion of the unique regional character of table olives by discrimination based on the geographical origin and cultivar. The need for a multi-omics approach has been highlighted but not yet extensively applied. The integration of metagenomic with volatilomic data, but also with other omics technologies (e.g., metatranscriptomics, shotgun metagenomics, metaproteomics, etc.) could pave the way for a comprehensive understanding of the mechanisms of the complex system of fermented table olives and offer a holistic representation of this ecosystem. This integration is bound to further allow the successful monitoring of novel fermentation processes (e.g., starter culture and low salt fermentation), reveal the interactions between the members of the table olives microbial consortium, their functional properties and their impact on the quality attributes of table olives, as well as assist in the production of high and consistent quality final products through the discovery of biomarker molecules.
Abbreviations
cDNA: | complementary DNA |
e-nose: | electronic nose |
FROGS: | Find Rapidly OTUs with Galaxy Solution |
GC-MS: | gas chromatography-mass spectrometry |
HCA: | hierarchical cluster analysis |
HS-SPME: | headspace solid-phase microextraction |
LAB: | lactic acid bacteria |
mRNA: | messenger RNA |
NGS: | Next Generation Sequencing |
OTUs: | Operational Taxonomic Units |
PCA: | principal component analysis |
PCR: | polymerase chain reaction |
PLS: | partial least squares |
QIIME: | Quantitative Insights Into Microbial Ecology |
rRNA: | ribosomal RNA |
SBSE: | stir-bar sorptive extraction |
SPME: | solid-phase microextraction |
VOCs: | volatile organic and compounds |
Declarations
Author contributions
FTM: Conceptualization, Validation, Writing—review & editing, Supervision. DL: Investigation, Writing—original draft, Writing—review & editing. Both authors read and approved the submitted version.
Conflicts of interest
The authors declare that they have no conflicts of interest.
Ethical approval
Not applicable.
Consent to participate
Not applicable.
Consent to publication
Not applicable.
Availability of data and materials
Not applicable.
Funding
Not applicable.
Copyright
© The Author(s) 2024.