Abstract
Aim:
Pancreatic ductal adenocarcinoma (PDAC) is a leading cause of cancer-related mortality and is characterized by T-cell exhaustion, particularly in effector CD8+ T-cells. This exhaustion, driven by persistent immunosuppressive signals in the tumor microenvironment, impairs immune function and hinders effective immunotherapy. This study aimed to identify key exhaustion-related marker genes in CD8+ T-cells linked to PDAC and assess the potential of repurposing anti-inflammatory drugs to counteract T-cell exhaustion and enhance immune responses against PDAC.
Methods:
We employed a multi-omics approach, integrating single-cell RNA sequencing data with whole genome sequencing to identify dysregulated exhaustion-related immune markers in CD8+ T-cells in PDAC. We examined gene expression profiles and conducted functional enrichment analysis to evaluate their roles in immune exhaustion. We analyzed mutations in the shortlisted biomarkers from The Cancer Genome Atlas (TCGA) and performed in silico mutational analysis using Maestro to evaluate the impact of an IL7R mutation (K110N) on protein function. Virtual screening using a deep learning framework, GNINA, explored the inhibitory features of the anti-inflammatory drugs oxaprozin and celecoxib on IL7R.
Results:
Key dysregulated exhaustion-related immune markers were identified including PRF1, GZMA, CD8A, CD3D, NKG7, IL7R, and IL2RG. Pathway enrichment analysis indicated significant involvement in T-cell receptor signaling, Th1 and Th2 differentiation, and Th17 differentiation pathways, correlating with reported poor survival outcomes in PDAC patients. Mutational analysis of IL7R revealed a likely pathogenic mutation (K110N) located in the IL-7Ralpha fibronectin type III domain. Drug repurposing of oxaprozin and celecoxib showed favorable binding interactions with both wild and mutant IL7R proteins.
Conclusions:
The K110N mutation, despite not causing significant structural changes, may impact T-cell and B-cell homeostasis and development. Our findings suggest that oxaprozin and celecoxib could effectively inhibit T-cell exhaustion through favorable interactions with IL7R. Further clinical studies are necessary to validate the therapeutic potential of these anti-inflammatory drugs in enhancing immune responses in pancreatic cancer.
Keywords
Pancreatic ductal adenocarcinoma, effector CD8+ T-cells, immunosuppression, exhaustive immune biomarkers, anti-inflammatory drugsIntroduction
Pancreatic ductal adenocarcinoma (PDAC), originating from the exocrine pancreas, is a cancer with relatively low incidence but high mortality rates. By 2030, it is projected to become the second-leading cause of cancer-related deaths in the United States and the seventh most common form of cancer worldwide, with an overall 5-year survival rate of around 10% in the United States. Men tend to have higher incidence and mortality rates, both in terms of crude numbers and when adjusted for age, compared to women. The median age at which PDAC is typically diagnosed in advanced stages is 70 years [1].
PDAC is one of the most lethal solid tumors despite the use of multi-agent conventional chemotherapy regimens. PDAC typically originates from non-invasive precancerous lesions, classified as low-grade or high-grade based on epithelial dysplasia. Pancreatic intraepithelial neoplasms (PanINs), also known as primary precursor lesions, are found in pancreatic ducts. Similarly, the intraductal papillary mucinous neoplasms (IPMNs) are responsible for causing a smaller proportion of 10% of PDAC cases [2]. The dense tumor environment is primarily composed of approximately 90% of fibrous tissues. Thus, these factors result in clinical challenges in the development of effective therapies, contributing to PDAC being recognized as one of the most formidable diseases to treat. Despite the cytotoxic treatments, low significant improvement in survival rates of pancreatic cancer patients is observed [3].
Among the aforementioned complexities, the dysregulation of the immune system in PDAC creates an immunosuppressive tumor microenvironment. The dense fibrous stroma, immune cell infiltration, and a network of blood vessels in the tumor microenvironment of PDAC result in tumor growth and progression. These microenvironmental complexities create challenges for therapeutic intervention leading to high activity of immune checkpoint pathways that impair the ability of the body to effectively mount the antitumor immune responses. Moreover, these factors play crucial roles in PDAC progression and resistance to therapeutic interventions [4].
Among these factors, T-cells play a critical role in adaptive immune responses through direct destruction of cancerous cells and regulation of immune activity through cytokine release. However, T-cell exhaustion, marked by prolonged expression of inhibitory receptors, is a major obstacle in immunotherapy, leading to treatment failure and inadequate control of cancers. Targeting exhausted T-cells is challenging due to their heterogeneous nature and varied responses to interventions [5]. Single-cell RNA sequencing (scRNA-seq) can play a crucial role in identifying such heterogeneous roles and gene expression levels of these exhausted immune cells, leading to the identification of immunotherapeutic targets.
Effector CD8+ T-cells are important for the protective immunity against tumors. CD8+ T-cell exhaustion is driven by massive immunosuppressive signals, including nutrient deficiency, hypoxia, pro‐inflammatory cytokines, and chronic T-cell receptor signaling in the tumor microenvironment regarded as the main responder to ICB (immune checkpoint blockade), and correlated with poor prognosis in numerous cancers. This excessive amount of signals often leads CD8+ T-cells to gradual deterioration of T-cell function, a state called exhaustion [6].
Exhausted effector CD8+ T-cells are characterized by progressive loss of cytokine production and killing function, dysregulated metabolism, poor memory recall response, and homeostatic proliferation. These altered functions are closely related to altered transcriptional programs and epigenetic landscapes that clearly distinguish exhausted T-cells from normal effector and memory T-cells [6].
Hence, there is a dire need to biologically evaluate these exhausted and heterogeneous effector CD8+ T-cells for effective therapeutic interventions by identifying dysregulated immune biomarkers. Therefore, this study employed a multi-omics approach to investigate the exhaustive immune marker genes in effector CD8+ T-cells by using scRNA-seq analysis and single nucleotide polymorphisms from PDAC patients obtained from The Cancer Genome Atlas (TCGA). The identification of exhaustive immune marker genes and their domains will give insights into the pro-cancer pathways leading to an exhaustive immune system. Lastly, drug repurposing was performed to identify anti-inflammatory drugs as potential inhibitors of both wild-type and mutant protein structures. The findings from this study have the potential to facilitate the development of new therapeutic strategies for pancreatic cancer by inhibiting the exhaustive immune marker genes in the effector CD8+ T-cells.
Materials and methods
scRNA-seq data retrieval and processing
The scRNA-seq raw data, comprising 27 PDAC tumor samples (accession ID: GSE205013), were sourced from the publicly available National Center of Biotechnology Information (NCBI) Gene Expression Omnibus (GEO), a public database storing extensive collections of high-throughput functional genomics and expression datasets [7]. The dataset was acquired through 10X Genomics and Illumina NextSeq, HiSeq4000 platform was used to sequence the samples.
To analyze the raw sequencing FASTQ files, the solo submodule of the STAR splice-aware aligner tool was employed. Key parameters included "--soloType CB_UMI_Simple" for defining the structure of cellular barcodes and unique molecular identifiers (UMIs), along with "--soloCBwhitelist", using the 10X Genomics V2 whitelist for barcode validation. Additional settings comprised "--soloBarcodeReadLength 0" to automatically adjust barcode read length, "--soloUMIdedup 1MM_All" to allow deduplication of UMIs within a 1-mismatch threshold, and "--soloCBmatchWLtype" to ensure strict matching of barcodes to the whitelist. UMI filtering was conducted with "--soloUMIfiltering MultiGeneUMI", and cell barcode filtering followed the CellRanger 2.2 approach via "--soloCellFilter CellRanger2.2". The reads were decompressed using "--readFilesCommand zcat", and the maximum output of spliced junctions was set with "--limitOutSJcollapsed 2000000" [8]. The reference genome index for GRCh38 Homo sapiens was generated using the STAR "star-build" command. Post-alignment, UMIs associated with individual cells were quantified, and valid cell barcodes were identified, resulting in a sparse cell-by-gene count matrix. Three output files were generated for each sample in Matrix Market (MTX) format, including the count matrix ("matrix.mtx"), cell barcodes ("barcodes.tsv"), and gene identifiers ("features.tsv").
Additionally, Seurat (version 4.4.0) and scType R packages were used for the quality control and downstream analysis [9]. Low-quality cells were removed using three parameters, including the number of UMIs identified per cell barcode (i.e., library size), the number of genes called per barcode (nFeature_RNA > 200 & nFeature_RNA < 6000) for the filtration of cells expressing too few or too many genes, as fewer than 200 gene expressing cells may represent dead or damaged cells, while cells expressing more than 6,000 genes may represent doublets or multiplets of two or more cells, and the proportion of UMIs mapping to mitochondrial genes (< 10%).
Additionally, the high mitochondrial content was filtered out using the condition "percent.mt < 10", as it can indicate damaged or low-quality cells. Lastly, the low RNA content cells were filtered out using the condition "nCount_RNA > 1000", which can indicate that low RNA content might have undergone apoptosis or may have low-quality data [10]. Additionally, to exclude outlier distributions (no potential doublets/multiples and cells with higher mitochondrial reads > 10%), the visual analysis of the violin plots for each metric was performed.
The raw counts were normalized using the “LogNormalize” method, which involves taking the natural logarithm of the raw numbers and adding a pseudocount of 1. This is done by dividing the result by the total number of counts for that cell. Scaling the expression numbers and adding a pseudo count to account for technical variability improves the comparability of gene expression data across cells.
Clustering and dimension reduction
The highly variable characteristics were subsequently determined using the “findvariablefeatures” function from the Seurat package. Specifically, 2,000 genes expressing substantial very high to very low deviation in expression across different cells were identified. Dimensionality reduction was performed using t-distributed stochastic neighbor embedding (t-SNE) to compute the first 20 principal components (PCs) for clustering and visualization [11]. Additionally, cell clustering was performed using the standard Louvain clustering technique [9].
Cell annotation and differential expression analysis
Cell annotation based on marker genes from the “immune system” was performed using an automated supervised clustering pipeline, utilizing an experimentally validated cell marker database, through the scType R package [12]. The differential expression analysis was subsequently performed to determine significant marker genes (p-value < 0.05 and logFC > 0.25 or logFC < –0.25) through the Wilcox method for each cell type as well as effector CD8+ T-cells. Additionally, module score was calculated based on the exhaustive markers given below by utilizing the AddModuleScore within Seurat package, whereas grouping was done based on each cell type for statistical comparison using the ggpubr package (https://cloud.r-project.org/web/packages/ggpubr/index.html).
Functional enrichment analysis of effector CD8+ T-cells dysregulated genes
The functional enrichment analysis of the effector CD8+ T-cells dysregulated genes was performed using the online tool GeneCodis4. GeneCodis 4 (https://genecodis.genyo.es/) is a web-based tool that integrates information from various biological databases and tools to perform functional enrichment analysis based on different annotation sources, such as Gene Ontology (GO) terms and KEGG pathways. It extracts sets of significant concurrent annotations and assigns a statistical score to evaluate those that are significantly enriched in the input list [13]. Moreover, these pathways were further evaluated through literature review whether they are reported for poor survival by their dysregulation.
Literature review and shortlisting of exhausted genes
An extensive literature review used Google Scholar and PubMed databases to shortlist the exhaustive immune marker genes in different T-cell types involved in various pro-cancer pathways leading toward an exhaustive immune system. Among all the genes, only genes reported in the literature to cause an exhaustive immune system in effector CD8+ T-cells were shortlisted for further analysis. To extract information about these genes, several search terms were used, such as “T-cells”, “immune cells”, “T-cell exhaustion Genes”, and “CD8+ T-cell exhaustion”.
Expression validation
GEPIA2 web server (http://gepia2.cancer-pku.cn/), a highly cited and valuable resource for gene expression analysis from TCGA and GTEx databases, was used to validate the gene expression levels of the shortlisted genes identified to be dysregulated in effector CD8+ T-cells PDAC tumor samples [14]. The expression levels of the shortlisted exhaustive genes were validated through GEPIA2 based on their expression levels in normal and tumor samples in pancreatic adenocarcinoma (PAAD).
Identification of reported mutations in whole genome sequencing data and virtual mutagenesis
The Genomic Data Commons (GDC) Data Portal (https://portal.gdc.cancer.gov/) comprising data from TCGA (https://www.cancer.gov/ccg/research/genome-sequencing/tcga) and Therapeutically Applicable Research to Generate Effective Treatments (TARGET) program (https://www.cancer.gov/ccg/research/genome-sequencing/target) was used to identify the clinically reported deleterious and possibly damaging mutations in the shortlisted exhaustive genes [15]; on the other hand, the AlphaMissense database (https://alphamissense.hegelab.org/) which predicts the pathogenicity of the missense variants [16] was utilized to evaluate the pathogenicity of clinically identified mutations from the GDC. The genes that were likely pathogenic among both databases were selected for further analysis.
The 3D structure of the selected protein was retrieved using the AlphaFold Protein Structure Database (https://alphafold.ebi.ac.uk/), which predicts the 3D structure by using the amino acid sequence of the protein [17]. Moreover, its protein name and sequence length were also observed from the UniProt database (https://www.uniprot.org/). Additionally, Maestro 12.0 (version 12.0.012; Schrödinger, LLC, New York, NY) was utilized to generate the mutant model of the selected protein from the wild-type protein structure.
Furthermore, the InterPro database (https://www.ebi.ac.uk/interpro/), which predicts domains and provides functional analysis of the proteins by classifying them into families [18], was utilized to retrieve the domain information of the selected protein.
Lastly, the visualization tool PyMOL (The PyMOL Molecular Graphics System, Version 3.0 Schrödinger, LLC) was utilized to superimpose the mutant model of the selected protein on the wild-type model, resulting in root mean square deviation (RMSD) value and structural differences between both models; additionally, domain regions were also colored.
Retrieval of anti-inflammatory drugs
Anti-inflammatory drugs that have the potential to inhibit the inflammatory reaction proteins were identified through a literature search using search terms such as “anti-inflammatory drugs” and “drugs against inflammatory proteins”. Furthermore, the PubChem database (https://pubchem.ncbi.nlm.nih.gov/), comprising chemical molecules and their activities [19], was utilized to retrieve the Simplified Molecular Input Line Entry System (SMILES) of the anti-inflammatory drugs that were converted to PDB-format through a custom Python script using RDKit (RDKit: Open-Source Cheminformatics Software, https://www.rdkit.org).
Virtual screening for drug repurposing of anti-inflammatory drugs
The virtual screening of the retrieved anti-inflammatory drugs with the wild-type and the mutant model of the IL7R was performed using the molecular docking tool GNINA utilizing a group of conventional neural networks (CNNs) as a scoring function [20]. The top three best binding affinity-scored complexes were visualized on Maestro 12.0 (version 12.0.012; Schrödinger, LLC, New York, NY) to identify the protein-ligand interactions.
The 2D interaction analysis of the top three highest-affinity complexes was conducted using Discovery Studio Visualizer (BIOVIA, San Diego, CA, USA). PDB-format complexes were imported, and 2D illustrations of interactions between protein residues and the ligand were generated.
Results
Pro-tumor CD8+ T-cells dynamic expression profile in tumor microenvironment
Sequencing data processing included mapping and quantifying reads with UMIs, yielding a dataset of 156,559 cells and 36,601 RNA features. After normalization, 2,000 highly variable genes were identified to detect elevated expression within tumor cells, including JCHAIN, COL3A1, COL1A2, COL1A1, CCL18, IGKC, IGHG1, APOC1, SPP1, and OLFM4, shown in Figure 1A.
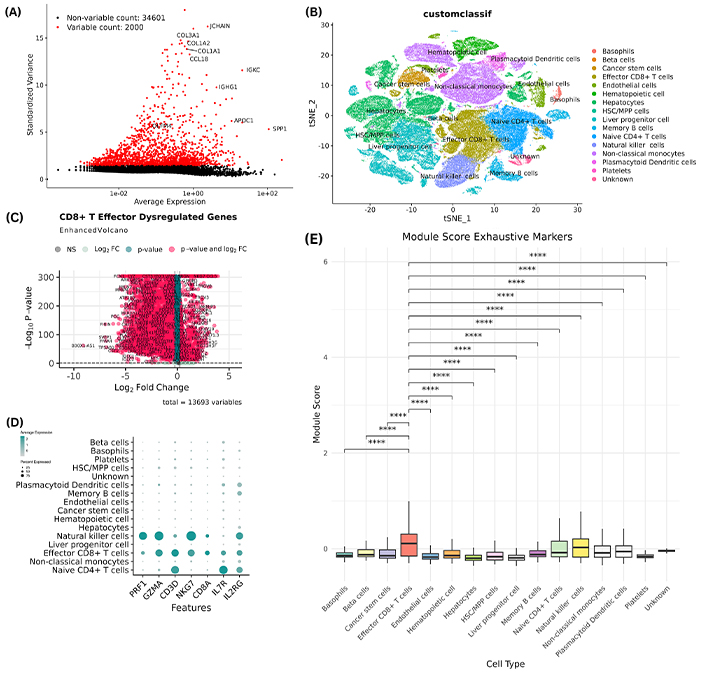
Genes that expressed in tumor microenvironements. (A) The top 2,000 highly variable genes were determined for the subsequent analysis. (B) t-SNE visualization of the distinct cell subpopulations. (C) Volcano plot depicting the differentially expressed genes in CD8+ T effector cells, red dots indicate the genes meeting the differential expression thresholds, light teal indicates the genes that meet p-value threshold, light green indicates the genes that meet the logFC threshold whereas light gray indicates the genes that do not meet any threshold and are therefore non-significant. (D) Dot plot of the 7 shortlisted genes showing the expression levels of each gene in all cell subpopulations indicating a higher expression of these genes in CD8+ T effector cells compared to most other cell types. (E) Module score for each cell type based on 13,691 gene markers that show statistically significant (p < 0.05) higher average expression in CD8+ T effector cells compared to all other cell types. The module score is a measure of the degree to which a set of genes is co-expressed in a given sample. This finding suggests that the genes in the module are important for the function of CD8+ T effector cells. ****: denotes highly statistically significant results (p < 0.0001)
Using the scType R package and immune system marker database, cells were annotated and clustered, identifying cell types such as basophils, beta cells, cancer stem cells, effector CD8+ T-cells, endothelial cells, hematopoietic cells, hepatocytes, hematopoietic stem cells/multipotent progenitor cells (HSC/MPP) cells, liver progenitor cells, memory B cells, naive CD4+ T-cells, NK cells, non-classical monocytes, plasmacytoid dendritic cells, and platelets, visualized with t-SNE in Figure 1B. However, effector CD8+ T-cells were chosen for further analysis due to their central role in combating cancer cells. Additionally, these cells play a crucial part in exhaustion mechanisms during cancer progression, contributing to immune system exhaustion. Differential gene expression analysis on effector CD8+ T-cells identified 3,888 overexpressed (logFC > 0.25) and 6,894 underexpressed (logFC < –0.25), a volcano depicting these differentially expressed genes is shown in Figure 1C. The top 25 markers for CD8+ T-cells are given in Table 1.
Top 25 markers of effector CD8+ T-cell
Gene | AvgLog2FC | p-value (FDR) |
---|---|---|
CD8A | 3.674801457 | < 0.0001 |
GZMK | 3.646602714 | < 0.0001 |
CD8B | 3.643712642 | < 0.0001 |
CCL5 | 3.318077103 | < 0.0001 |
KLRG1 | 3.072229198 | < 0.0001 |
GZMA | 3.041245437 | < 0.0001 |
KLRK1 | 2.882004347 | < 0.0001 |
GZMH | 2.864935094 | < 0.0001 |
CD3D | 2.724396613 | < 0.0001 |
CD3G | 2.693499069 | < 0.0001 |
SH2D1A | 2.677929871 | < 0.0001 |
CD96 | 2.641160589 | < 0.0001 |
CD2 | 2.46276313 | < 0.0001 |
APOBEC3G | 2.414205027 | < 0.0001 |
CD3E | 2.379812082 | < 0.0001 |
PYHIN1 | 2.365613166 | < 0.0001 |
CST7 | 2.353722473 | < 0.0001 |
PTPN22 | 2.310352522 | < 0.0001 |
IKZF3 | 2.301985575 | < 0.0001 |
ITM2A | 2.277711796 | < 0.0001 |
TRAC | 2.277620352 | < 0.0001 |
GZMM | 2.244593676 | < 0.0001 |
CCL4 | 2.234407187 | < 0.0001 |
RUNX3 | 2.182604495 | < 0.0001 |
LCK | 2.15254322 | < 0.0001 |
An extensive literature review identified a total of 17 genes (PRF1, GZMA, IFNG, CD160, TBX21, ZEB2, NR4A2, SLAMF7, CD8A, CD3D, NKG7, CCR7, IL7R, ID2, IL2RB, IL2RG, and TGFB1) primarily involved in the exhaustion of different T-cells, including effector CD8+ T-cells. These identified genes were queried against our data using the ‘grep’ Linux command, resulting in 7 common genes (PRF1, GZMA, CD8A, CD3D, NKG7, IL7R, and IL2RG). Subsequently, these common exhaustive genes were selected for further validation. The dot plot of the shortlisted genes is depicted in Figure 1D which shows overexpression of these markers in effector CD8+ T-cells compared to other cell types. To evaluate the state of CD8+ T cells, we analyzed the expression of known exhaustion markers, including TOX, PDCD1, LAG3, TIGIT, EOMES, and RBPJ. By calculating the average expression of these genes across the entire cell type module, we found that CD8+ T cells exhibited a higher average module score than all other cell types for these markers. This suggests that CD8+ T cells have elevated expression levels of exhaustion-related genes, indicating a more pronounced state of exhaustion compared to other cell types; module score is shown in Figure 1E.
Functional enrichment analysis and validation of exhaustive markers of effector CD8+ T-cells
Pathway enrichment analysis was conducted to identify dysregulated pathways associated with the upregulated and downregulated markers of effector CD8+ T-cells. Notably, the upregulated genes were found to be enriched in pathways such as T-cell receptor signaling pathway, Th1 and Th2 cell differentiation, Th17 cell differentiation, and PD-L1 expression and PD-1 checkpoint pathway (Figure 2A). Conversely, pathways such as oxidative phosphorylation and chemical carcinogenesis, reactive oxygen species were found to be enriched for the downregulated genes (Figure 2B). The analysis revealed that the altered expression levels of these marker genes were significantly enriched in pathways associated with poor survival in PDAC patients, as reported in the literature [21–23].
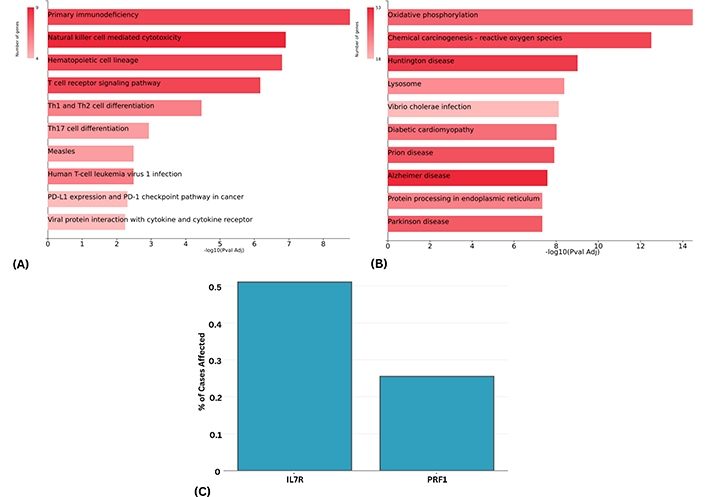
Top 10 upregulated and downregulated genes. (A and B) KEGG pathways analysis depicting the enriched terms based on the upregulated and downregulated genes. (C) Percentage of cases in the TCGA PAAD whole-genome dataset harboring somatic mutations in IL7R and PRF1. A case was considered affected if a mutation was detected in the respective gene. About 0.50% of cases exhibited IL7R mutations, while 0.25% of cases showed PRF1 mutations
In the analysis of TCGA datasets, these markers demonstrated significantly elevated expression levels in PAAD, with the IL2RG gene exhibiting the highest expression at 61.42 TPM, while the PRF1 gene had the lowest at 2.82 TPM. The CD3D gene showed an expression level of 9.98 TPM, and the GZMA, NKG7, and IL7R genes had expression levels of 7.96, 7.93, and 7.61 TPM, respectively, with the CD8A gene expressing at 4.05 TPM in tumor samples. The exhaustive markers and their respective expression levels in PAAD compared to normal are listed in Table 2.
Effector CD8+ T-cell shortlisted exhaustive gene TCGA expression validation analysis
Genes | Tumor | Normal |
---|---|---|
PRF1 | 2.82 | 0.32 |
GZMA | 7.96 | 0.32 |
CD8A | 4.05 | 0.69 |
CD3D | 9.98 | 0.41 |
NKG7 | 7.93 | 0.58 |
IL7R | 7.61 | 0.67 |
IL2RG | 61.42 | 0.75 |
TCGA: The Cancer Genome Atlas
Characterization of mutations in TCGA whole genome sequencing data for PDAC
To integrate our scRNA-seq findings and overexpression of these exhaustive markers with whole genome sequencing data, we analyzed the GDC TCGA PAAD dataset. The analysis concentrated on identifying mutations classified as benign, deleterious, and possibly damaging missense mutations. This investigation revealed that only two genes, PRF1 and IL7R, exhibited such mutations. However, PRF1 showed only one mutation (benign) at the 546 positions where proline was mutated to leucine (P546L), while IL7R showed two mutations (probably damaging and possibly damaging) at 110 and 405 positions where lysine was mutated to asparagine (K110N) and leucine was mutated to methionine (L405M), respectively. Moreover, the percent of cases that are affected by the mutations in IL7R and PRF1 genes are shown in Figure 2C.
Moreover, PRF1 and IL7R genes were further searched on the AlphaMissense database to predict the pathogenicity of PRF1 and IL7R mutations identified in the previous step. The AlphaMissense showed a total of 589 likely pathogenic mutations in the PRF1 gene, but none of those 589 mutations coincided with the mutation (P546L) that we identified in the TCGA dataset. Furthermore, the IL7R gene was observed to have a total of 488 likely pathogenic predicted mutations, whereas only one mutation coincided with our findings, where lysine was mutated to asparagine at position 110. Therefore, only one mutation of the IL7R gene (K110N) was used for further analysis.
Structural comparison of wild-type and mutant (K110N) IL7R protein
The 3D structure of the wild-type IL7R with a sequence length of 459 amino acids was retrieved from the AlphaFold database by utilizing its accession ID (P16871). Furthermore, the InterPro database predicted two domains, IL-7Ralpha, fibronectin type III (FN3) domain in regions 32–127 and 131–231, respectively. The IL7R protein details from the UniProt and InterPro databases are mentioned in Table S1.
Nonetheless, the wild-type IL7R protein structure was utilized to create its mutant model, K110N. Furthermore, both the wild-type and the mutant (K110N) models were superimposed, revealing an RMSD value of 0.218 Å, indicating a slight structural change in the mutant model (Figure 3A). Additionally, the domains, wild-type residue, and mutated residue were colored to highlight the differences in the wild-type and mutant IL7R protein model, shown in Figure 3B and 3C. Moreover, it was observed that the mutation (K110N) lies in the IL-7Ralpha, FN3 domain, indicating potential role in the pathogenicity of the IL7R protein.
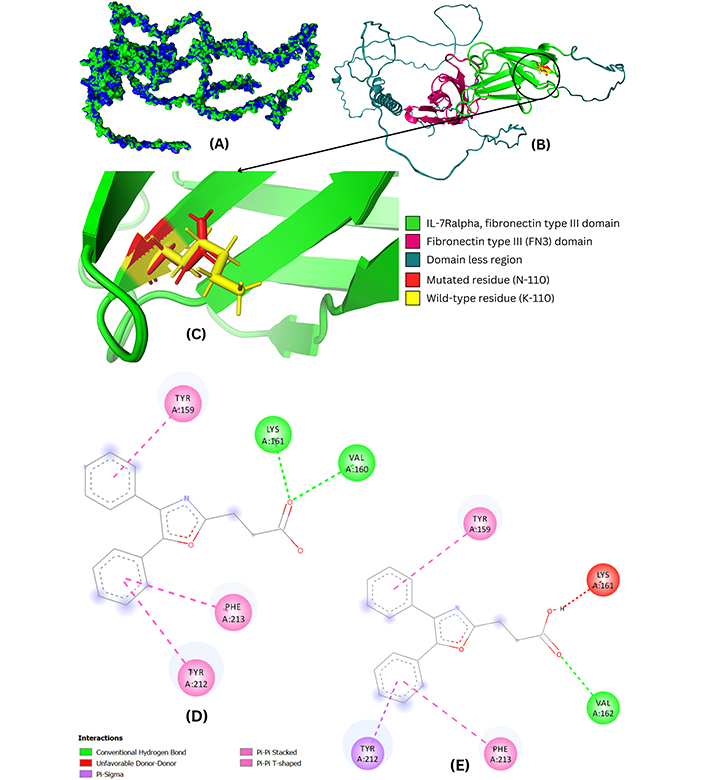
Structural and interaction analysis of wild-type and mutant IL7R-oxaprozin complexes. (A) The superimposed wild-type and mutant model of the IL7R shows the structural changes where green represents the wild while blue corresponds to the mutant model. The region where one color is more prominent than the other indicates deviation in terms of structure compared to the wild model. (B and C) Superimposed wild and mutant models of IL7R indicate small structural variations where mutated residue is colored in red. (D) 2D interaction analysis of the wild-type. The diagram illustrates the various interactions of oxaprozin with the wild-type and mutant protein residues, including conventional hydrogen bonds with LYS-161 and VAL-160, as well as Pi-Pi T-shaped and Pi-Pi stacked interactions involving TYR-159, PHE-213, and TYR-212. (E) 2D interaction analysis of mutant IL7R-oxaprozin complex, showing Pi-Pi stacked and Pi-Pi T-shaped interactions with TYR-159 and PHE-213, Pi-sigma interaction with TYR-212, unfavorable donor-donor interactions with LYS-161, and a conventional hydrogen bond with VAL-162
Retrieval of anti-inflammatory drugs
A total of 20 FDA-approved anti-inflammatory drugs were identified through a literature review, including diclofenac, diflunisal, etodolac, fenoprofen, flurbiprofen, ibuprofen, indomethacin, ketoprofen, ketorolac, mefenamic acid, meloxicam, nabumetone, naproxen, oxaprozin, piroxicam, sulindac, tolmetin, celecoxib, rofecoxib, and valdecoxib. The drug names, their PubChem IDs, SMILES, and 2D illustrations of the retrieved drugs are shown in Figure S1 and Table S1.
Binding affinity assessment of IL7R wild-type and mutant models with anti-inflammatory drugs
The virtual screening of the IL7R wild-type and mutant model with the anti-inflammatory drugs predicted various binding affinities ranging from –4.97 kcal/mol to –7.56 kcal/mol. However, the top three best binding affinities of –7.56 kcal/mol, –7.56 kcal/mol, and –7.53 kcal/mol were shown by wild-type IL7R-oxaprozin complex, mutant (K110N) IL7R-oxaprozin complex, and wild-type IL7R-celecoxib complex, respectively. Importantly, it was observed that the anti-inflammatory drug oxaprozin showed the same binding affinities (–7.56 kcal/mol) with both the wild-type and the mutant (K110N) model. Additionally, we have provided all docking results in Table S1.
Moreover, the 2D interaction analysis revealed that the oxaprozin exhibited Pi-Pi T-shaped, Pi-Pi stacked, and conventional hydrogen bond interactions with the wild-type protein residues, where including LYS-161 and VAL-160, were involved in conventional hydrogen bonds, while TYR-159, PHE-213, and TYR-212 were involved in Pi-Pi T-shaped and Pi-Pi stacked interactions. The 2D interactions of the wild-type IL7R-oxaprozin complex are shown in Figure 3C.
Furthermore, oxaprozin also exhibited Pi-Pi stacked, Pi-Pi T-shaped, Pi-sigma, unfavorable donor-donor, and conventional hydrogen bond interactions with the mutant model (K110N) protein residues, where TYR-159 and PHE-213 showed Pi-Pi stacked and Pi-Pi T-shaped interactions, TYR-212 showed Pi-sigma, LYS-161 showed unfavorable donor-donor interactions, and lastly, VAL-162 exhibited conventional hydrogen bond. The 2D interactions of oxaprozin with the mutant model (K110N) are shown in Figure 3D and 3E.
However, the visualization of the top three best-binding affinity complexes showed that oxaprozin and celecoxib exhibited a single hydrogen bond in all three complexes. The oxaprozin exhibited hydrogen bond interaction with protein residues K-161 and V-162 of wild-type and mutant (K110N) models with a bond distance of 1.89 and 2.33, respectively. Finally, celecoxib exhibited a hydrogen bond with the protein residue P-222 of wild-type protein with a bond distance of 1.83. The visualization of the best binding affinity complexes is shown in Figure 4A and 4B.
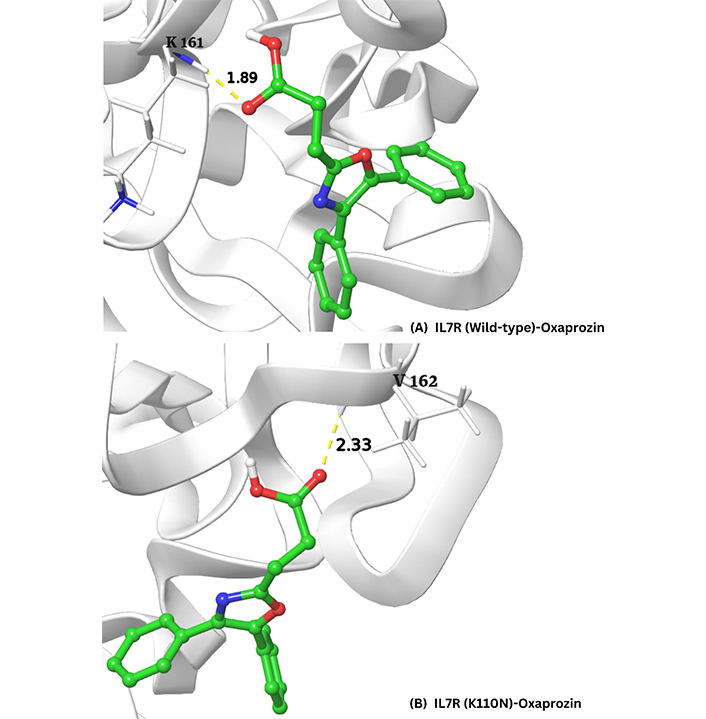
Visualization of the top three best-binding affinity complexes of oxaprozin and celecoxib with the wild-type (A) and mutant (K110N) (B) models of the IL7R protein, as analyzed using Desmond. The hydrogen bond interactions are highlighted, with oxaprozin forming bonds with residues K-161 (1.89 Å) and V-162 (2.33 Å)
Nonetheless, it was observed that in all three complexes, the anti-inflammatory drugs (oxaprozin and celecoxib) interacted with the wild-type and the mutant (K110N) model within the FN3 domain region, for both models. Moreover, the FN3 domain is known to be involved in cell adhesion, cell morphology, thrombosis, cell migration, tumor metastasis, inflammation, and embryonic differentiation, indicating that the binding of oxaprozin and celecoxib in this domain can inhibit the crucial function of this domain.
Discussion
T-cell exhaustion imposes a major hurdle to cancer immunotherapy, while the therapeutic efficacy of the immunotherapy is negatively related to T-cell exhaustion in tumors [20]. The T-cell exhaustion results in a lower response as the tumor-infiltrating lymphocytes (TILs) become exhausted and incapable of controlling the tumor progression [24]. The hallmark of T-cell exhaustion is characterized by the dysfunctioning of T-cells by low expression of cytokine production and enhanced expression of inhibitory receptors; however, it is reported that the T-cell exhaustion in PDAC is driven by multiple mechanisms hence, to control the tumor progression, reversing or inhibiting the T-cell exhaustion might be a viable option by targeting the exhaustive genes involved in T-cell exhaustion [25, 26].
As previously reported that immune marker genes (PRF1, GZMA, CD8A, CD3D, NKG7, IL7R, and IL2RG) of the effector CD8+ T-cells, which were involved in the pathways such as T-cell receptor signaling, Th1 and Th2 cell differentiation, and Th17 cell differentiation pathway which were proved to be involved in the poor survival [21–23].
The PRF1 gene is implicated in the granule-dependent killing of the NK cells and cytotoxic T lymphocytes (CTLs) [27]. GZMA is implicated in the inhibition of tumor growth, inducing apoptosis and antigen-specific cytotoxic CD8+ T-lymphocytes [28]. CD8A gene is involved in the cell-cell interactions within the immune system [29]. CD3D gene plays its role in the development of T-cells and signal transduction [30]. NKG7 is implicated in the regulation of the cytotoxic granule exocytosis in effector lymphocytes [31]. IL2RG encodes protein, which is a signaling component of various interleukin receptors [32]. IL7R gene encodes a protein that is a receptor for the IL-7 gene, while IL7R is a common marker of the lymphoid progenitor cells, and signaling of IL-7 leads to phosphorylation of STAT5 and proliferation of T and B cells [33]. This suggests that the shortlisted exhaustive immune marker genes in the effector CD8+ T-cells are deprived of performing their function effectively due to their exhaustiveness, which is also reported in several studies [34–37], eventually making an advantageous milieu for the tumor cells to proliferate and progress rapidly.
Furthermore, the mutational analysis of these exhaustive immune marker genes resulted in one significant, likely pathogenic mutation in the IL7R protein where lysine was mutated to asparagine at position 110 (K110N). Although the mutation did not result in a major structural change in the mutant (K110N) model of IL7R protein (0.218 Å RMSD), a study reported that the IL7R mutations are involved in the tumor development and proliferation in T-cell acute lymphoblastic leukemia (T-ALL) [38]. Similarly, the AACR Project GENIE Consortium reported that IL7R is mutated in 2% of all cancers, including PAAD [39].
However, in this study, two domains, IL-7Ralpha and FN3 domain, indicated that the mutation was found to be in the IL-7Ralpha, FN3 domain. This domain is implicated in the homeostasis and development of T and B cells through signaling cascades by forming a complex with the IL-7 gene, while the development of T and B cells is inhibited by the mutations in the IL7R ectodomain region [40], suggesting that the mutation (K110N) may disrupt the function of the IL7R protein. This indicates that although the in-silico mutational analysis in our study did not result in any major structural changes, there may be a possibility of gain or loss of function in the mutant model, leading to the development and progression of tumor cells.
Moreover, to repurpose the anti-inflammatory drugs, virtual screening and the 2D interaction analysis indicated that the anti-inflammatory drugs oxaprozin and celecoxib interacted with the protein residues of the wild-type and mutant (K110N) model within the FN3 domain. Furthermore, this domain is implicated in cell migration, cell morphology, embryonic differentiation, thrombosis, and cell adhesion, while it also exhibits functional and structural modularity [41, 42]. This indicates the potential of oxaprozin and celecoxib, interacting within the FN3 domain, in inhibiting the wild-type and mutant (K110N) models.
Lastly, extensive studies have been conducted on anti-inflammatory drugs to improve cancer therapy for over four decades [43]. Oxaprozin is an oral, long-acting, non-steroidal anti-inflammatory drug used for the treatment of rheumatoid arthritis and osteoarthritis and has a lower incidence of gastrointestinal side effects [44]. It is reported to be used in cancer treatment by targeting the matrix metalloproteinase 9 (MMP9) gene, which is implicated in various cancers, including breast, lung, colon, and PAAD [45, 46]. Additionally, the anti-inflammatory, analgesic, and antipyretic drug celecoxib is used for the treatment of rheumatoid arthritis, osteoarthritis, breast cancer, colon cancer, and UVB radiation-induced skin cancer [47]. It is also reported to significantly inhibit tumor development and is involved in the reduction of drug resistance through multiple molecular mechanisms [48].
In conclusion, we propose that T-cell exhaustion plays a significant role in immune system dysregulation, contributing to the development and progression of PDAC. We identified seven exhaustive immune marker genes (PRF1, GZMA, CD8A, CD3D, NKG7, IL7R, and IL2RG) were identified, which are associated with poor survival outcomes in pancreatic cancer patients. A notable mutation (K110N) in the IL7R protein was found in its FN3 domain, with oxaprozin and celecoxib identified as potential inhibitors for both the wild-type and mutant IL7R protein. This suggests that these anti-inflammatory drugs may help alleviate T-cell exhaustion and improve immune responses against PDAC. Further clinical validation is needed to assess their efficacy in targeting these immune biomarkers. This study highlights the need for additional research on the molecular and functional aspects of immune biomarkers in pancreatic cancer progression.
Abbreviations
FN3: | fibronectin type III |
GDC: | Genomic Data Commons |
PAAD: | pancreatic adenocarcinoma |
PDAC: | pancreatic ductal adenocarcinoma |
RMSD: | root mean square deviation |
scRNA-seq: | single-cell RNA sequencing |
SMILES: | Simplified Molecular Input Line Entry System |
TCGA: | The Cancer Genome Atlas |
t-SNE: | t-distributed stochastic neighbor embedding |
UMIs: | unique molecular identifiers |
Supplementary materials
The supplementary Table S1 for this article is available at: https://www.explorationpub.com/uploads/Article/file/1003179_sup_1.xlsx. The supplementary Figure S1 for this article is available at: https://www.explorationpub.com/uploads/Article/file/1003179_sup_2.pdf.
Declarations
Author contributions
RA: Conceptualization, Formal analysis, Investigation. RM: Data curation, Methodology, Software. SAS: Validation, Visualization, Writing—original draft. AAS: Supervision, Project administration, Writing—review & editing.
Conflicts of interest
The authors declare that they have no conflicts of interest.
Ethical approval
Not applicable.
Consent to participate
Not applicable.
Consent to publication
Not applicable.
Availability of data and materials
The raw data supporting the conclusions of this manuscript will be made available by the authors, without undue reservation, to any qualified researcher.
Funding
Not applicable.
Copyright
© The Author(s) 2025.
Publisher’s note
Open Exploration maintains a neutral stance on jurisdictional claims in published institutional affiliations and maps. All opinions expressed in this article are the personal views of the author(s) and do not represent the stance of the editorial team or the publisher.