Abstract
Aim:
Cervical squamous cell carcinoma (CESC) is a highly prevalent women’s gynecologic disease. Because the specific mechanisms associated with the illness are still mostly unclear, more investigation is needed to comprehend the triggers of CESC’s initiation and progression. Circular RNAs (circRNAs) are a new type of RNA that control microRNAs’ (miRNAs) expressions. Although particular circRNA–miRNA–mRNA regulatory axes for CESC have been defined, little is known about this field of research. Therefore, the current study aimed to identify new circRNA–miRNA–mRNA axes in CESC.
Methods:
GSE102686 and GSE169057 GEO datasets were utilized to identify differentially expressed circRNAs (DEcircRNAs). The Cancer Genome Atlas (TCGA) database was used to detect differentially expressed miRNAs (DEmiRs) and mRNAs (DEmRNAs). Various in silico tools were used to identify interactions between circRNAs, miRNAs, and their potential target genes in CESC. Moreover, enrichment analysis, correlation analysis, and survival analysis were performed on potential target genes.
Results:
Utilizing publically available data, we found dysregulated circRNAs, miRNAs, and mRNAs in CESC. We showed that the circRNA hsa_circ_0000711 may be involved in the CESC process by inhibiting many target genes via hsa-miR-338-3p and/or hsa-miR-361-3p. Moreover, we found that hsa_circ_0000515 circRNA can contribute to CESC by modulating the expression of some target genes via hsa-miR-296-5p.
Conclusions:
The findings of this work contribute to a better understanding of the circRNA–miRNA–mRNA regulation processes in CESC.
Keywords
Cervical squamous cell carcinoma, circRNA, miRNA, mRNAIntroduction
Cervical cancer (CC) is the fourth most common female malignancy. CC death rates rise every year because of a lack of early detection [1, 2]. Despite surgery, radiation, or chemotherapy optioning for 80% of initial stages CC cases, there is still an enormous percentage of advanced-stage individuals who have poor outcomes [3]. The most prevalent histological subgroup of CC is cervical squamous cell carcinoma (CESC) [4]. The main serious issues with CESC are metastasis and recurrence. Because the particular pathways remain unclear, more study into the underlying tumor initiation and development processes is required for CESC [3, 4]. Non-coding RNAs are well-known actors in cancer biology, serving as a critical part of gene regulation that, when disrupted, contributes to the development of cancer [5]. microRNAs (miRNAs), which are non-coding RNAs with a length of around 20–25 nucleotides, function as critical regulators in the cell by controlling target genes’ expressions [6, 7]. Circular RNAs (circRNAs) are covalently closed, endogenous biomolecules in cells with tissue-specific and cell-specific expression patterns that function through specific miRNAs [5, 8, 9]. Unlike ordinary linear RNA, circRNAs have a characteristic closed-loop structure [10]. As a result, circRNAs are resistant to destruction and are expressed in a wide range of organisms. Following miRNA binding by miRNA response elements (MREs), circRNAs may be essential in tumor development as tumor suppressors or proto-oncogenes [11, 12]. Determining the circRNA–miRNA–mRNA axes is critical for understanding the molecular mechanism of cancers. For instance, circ_VPRBP has been found to reduce cell proliferation, migration, and invasion and also induce CC cell apoptosis via modulating the miR-93-5p/FRMD6 [13]. circRNA_101996 has been shown to increase the development of CC by influencing the miR-1236-3p/TRIM37 axis [14]. In another investigation, circRNA_400029 has been shown to increase the aggressiveness of CC by controlling the miR-1285-3p/TLN1 axis. Recent studies have uncovered several circRNA–miRNA–mRNA pathways in CC. However, we are still in the early stages of unraveling the complicated interactions between thousands of circRNAs, miRNAs, and mRNAs found in the cells. Therefore, in the current study circRNA–miRNA–mRNA axes were investigated using various bioinformatics data.
Materials and methods
Selection criteria for circRNA–miRNA–mRNA interactions
The recognized functioning principles of the circRNA–miRNA–mRNA axis guided the selection. Before selecting circRNAs, miRNAs, and mRNAs from the relevant Gene Expression Omnibus (GEO) datasets, Log2 Fold Change (log2FC) and p-values were considered. The overexpressed circRNAs were the intended focus of this research. Downregulated miRNAs were considered for sponge miRNAs. The selection of mRNAs was also limited to elevated ones.
GEO is a publicly available functional genomic data resource. Microarrays or sequence-based studies are accepted. Users can use the supplied tools to query and download experiments and determine gene expression profiles. Identifying molecules (circRNA, miRNA, mRNA, etc.) whose expressions alter in common among various datasets may be useful to obtain more accurate information about the association between these molecules and diseases before in vitro or in vivo studies.
Identification of circRNAs
Gene Expression Omnibus 2R (GEO2R) is an interactive web application that enables users to compare two or more sets of samples from a GEO series to find differentially expressed genes, miRNAs, circRNAs, etc. GSE102686 dataset (including 5 CC patient’s tissue samples and 5 adjacent normal tissue samples) and GSE169057 (including 3 CC patient tissue samples and 3 adjacent normal tissue samples) datasets were downloaded from the National Center for Biotechnology Information (NCBI) Geodatabase and the differential expression of circRNAs between tumor and normal samples was analyzed via GEO2R. When selecting upregulated circRNAs from the datasets, first we paid attention to the log2FC and p-value of circRNAs (log2FC > 1, p < 0.001). circRNAs that met these criteria and overlapped in both datasets were selected.
Detection of miRNAs
The Cancer Genome Atlas (TCGA) (https://cancergenome.nih.gov/) is a fundamental cancer genomics program, that molecularly analyzed approximately 20,000 primary cancers and matched normal samples from 33 different types of cancer. In the TCGA database, miRNAs with log2FC > 2 and p < 0.05 were identified in 307 CESC tissue samples and matched 3 normal tissue samples. UALCAN [15] and CancerMIRNome [16] are user-friendly platforms for investigating miRNA dysregulation in various cancer samples from the TCGA. For miRNA expression analysis these web tools were used.
mRNA selection
The Gene Expression Profiling Interactive Analysis (GEPIA2) [17] is beneficial and well accepted online tool for gene expression, survival analysis, etc., using tumor and normal specimens from the TCGA and Genotype-Tissue Expression (GTEx) databases. Overexpressed genes with log2FC > 2 and p < 0.01 criterion were chosen in TCGA constructed from 307 CESC patients and 3 adjacent normal tissue samples using GEPIA2 tool (ANOVA method was selected using GEPIA2 tool).
Determination of circRNA–miRNA–mRNA interaction
circRNA interactome (CircInteractome) identifies miRNAs that might possibly target the circRNAs using the Targetscan prediction tool [18]. The CircInteractome web tool was used to identify potential sponge miRNAs of selected circRNAs from the GSE102686 and GSE169057 datasets. miRNet 2.0 is a user-friendly, web-based tool that integrates user data with existing information using network-based visual analytics to assist in unraveling miRNA functions [19]. The putative target genes of the selected miRNAs were defined via miRNet 2.0. Then, those matched genes with the log2FC > 2, p < 0.01 criterion in the TCGA database were chosen.
Enrichment analysis
The biological processes, cellular components, and molecular activities in which the selected genes were involved were determined by creating the EnrichR tool (https://maayanlab.cloud/Enrichr/, pathways with p < 0.05 were considered).
Correlation analysis
Selected genes’ correlation analysis was constructed via Correlation AnalyzeR [20] and GEPIA2 (http://gepia2.cancer-pku.cn/) tools.
Survival analysis
Kaplan-Meier plotter (KM plotter) is an online platform for determining genes/miRNAs’ expression and survival rates in many cancer types. It also enables publicly accessible transcriptome data, such as TCGA. The prognostic values of chosen miRNAs in CESC were determined utilizing the KM plotter database. In brief, the miRNAs were entered into the database. Then KM survival plots were constructed, with the hazard ratio (HR), 95% confidence intervals (CI), and log-rank p-value provided on the website. p < 0.05 were considered statistically significant.
Statistical analysis
Publicly available platforms were used to conduct functional enrichment assessments. When choosing upregulated circRNAs from the datasets, we evaluated the log2FC and p-value (for GSE102686 analysis: log2FC > 1, p < 0.001; for GSE169057 dataset: log2FC > 1; p < 0.001). For DEmiRs log2FC > 2 and p < 0.05; overexpressed genes with log2FC > 2 and p < 0.001 were used. The KM method was utilized for determining overall survival (OS), and the log-rank test was used to determine differences (via KM plotter tool). The statistical cut-off for OS analysis and enrichment analysis in tools was set at a p < 0.05. GraphPad Prism 10 was employed to perform statistical calculations and visualize figures.
Results
circRNA identification
In GSE102686, 137 upregulated circRNAs met the log2FC > 1, p < 0.001 criteria among the circRNAs whose expression changed in cancer tissue samples compared to normal tissue samples; in GSE169057, 145 upregulated circRNAs met the log2FC > 1, p < 0.001 condition. In both datasets, three overlapping circRNAs (hsa_circ_0000711, hsa_circ_0000515, and hsa_circ_0001855) have been chosen by the required parameters (Table 1).
Overlapping circRNAs between GSE169057 and GSE102686 datasets
circBase ID | GSE169057 | GSE102686 | ||
---|---|---|---|---|
p-value | log2FC | log2FC | p-value | |
hsa_circ_0000711 | 4.28e–02 | 1.57 | 1.37 | 7.30e–02 |
hsa_circ_0000515 | 3.66e–03 | 3.75 | 1.28 | 4.39e–06 |
hsa_circ_0001855 | 2.99e–02 | 1.79 | 1.02 | 4.52e–02 |
miRNA selection results
Following study on the circinteractome tool that suggested potential targets of the three selected circRNAs, 26 miRNAs were identified as common targets as seen in Figure 1. Three hundred fifty-nine upregulated miRNAs met the log2FC > 2 and p < 0.05 requirements in the TCGA database in CESC tissue samples compared to normal tissues. The 26 miRNAs were screened among these 359 miRNAs to detect overlapping miRNAs. Six possibly sponge miRNAs were overlapped. Among these 6 miRNAs, 3 miRNAs (hsa-miR-296-5p, hsa-miR-338-3p, and hsa-miR-361-3p) with decreased expression were selected (because selected circRNAs were upregulated) (Table 2).
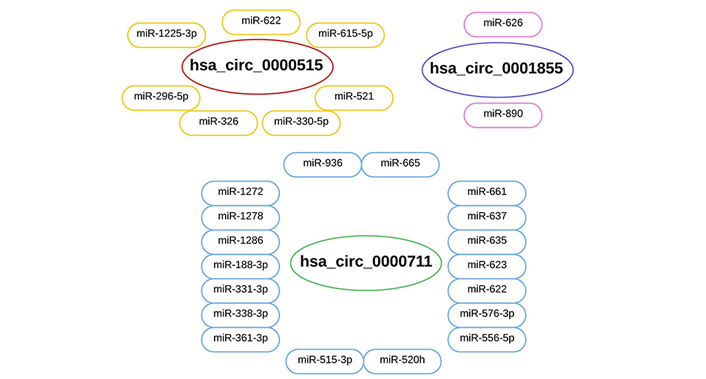
Potential sponge miRNAs of three hsa_circRNAs. According to the circinteractome and circMine tools, it is estimated that hsa_circ_0000711 has 18 potential sponge miRNAs, hsa_circ_0000515 has 7 potential sponge miRNAs, and hsa_circ_0001855 has 2 potential sponge miRNAs
Overlapping miRNAs between TCGA database and selected upregulated circRNAs’ potential sponge miRNAs in circinteractome and circMine tools
miRNAs | Log2FC | Adj. p-value |
---|---|---|
hsa-miR-296-5p | –2.18 | 1.43e–12 |
hsa-miR-326 | 2.12 | 1.39e–33 |
hsa-miR-330-5p | 5.41 | 1.27e–21 |
hsa-miR-331-3p | 4.47 | 2.40e–12 |
hsa-miR-338-3p | –8.9 | 3.75e–15 |
hsa-miR-361-3p | –7.72 | 1.45e–28 |
mRNAs analysis results
Among the overexpressed genes in CESC tumors compared to normal tissues in the TCGA database, it was defined that 778 genes met the conditions of log2FC > 2 and p < 0.05.
circRNA–miRNA–mRNA interaction results
Using miRNet 2.0 and miRTarBase v8, the target genes of the 3 selected miRNAs were examined and 355 genes matching these miRNAs were identified (Figure 2). Seven hundred seventy-eight genes which were identified from the TCGA database were compared to 355 genes shown as probable targets of 3 chosen miRNAs, in the end, 10 overlapping genes were selected (Table 3). The heatmap of these 10 genes that are upregulated in CESC is shown in Figure 3. In summary, three circRNAs were chosen because they had the potential to regulate the expression of ten genes via three miRNAs.
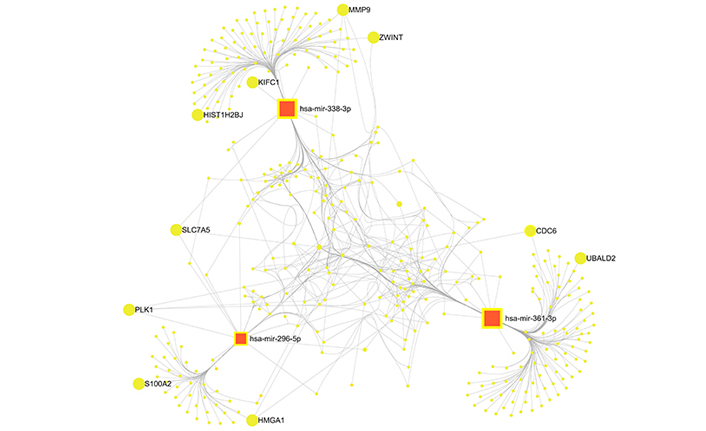
Three selected miRNAs and their possible target genes: a complicated network. Red squares represent selected miRNAs, yellow circles represent possible target genes whereas big yellow circles represent overlapping genes. The figure was created utilizing the miRTarbase v8. and miRNet tools. A p < 0.05 value was used to determine significances
Selected 10 upregulated genes that could be potential targets for selected downregulated 3 miRNAs
Gene | Approved name | Log2FC | Adj. p-value |
---|---|---|---|
S100A2 | S100 calcium binding protein A2 | 9.183 | 2.74e–11 |
ZWINT | ZW10 interacting kinetochore protein | 5.099 | 1.16e–43 |
KIFC1 | Kinesin family member C1 | 4.492 | 6.97e–47 |
MMP9 | Matrix metallopeptidase 9 | 3.920 | 1.01e–9 |
PLK1 | Polo like kinase 1 | 3.732 | 9.80e–32 |
CDC6 | Cell division cycle 6 | 3.678 | 2.47e–29 |
HMGA1 | High mobility group AT-hook 1 | 3.585 | 4.56e–26 |
SLC7A5 | Solute carrier family 7 member 5 | 2.941 | 4.60e–7 |
HIST1H2BJ | H2B clustered histone 11 | 2.333 | 2.42e–4 |
UBALD2 | UBA like domain containing 2 | 2.190 | 1.81e–17 |
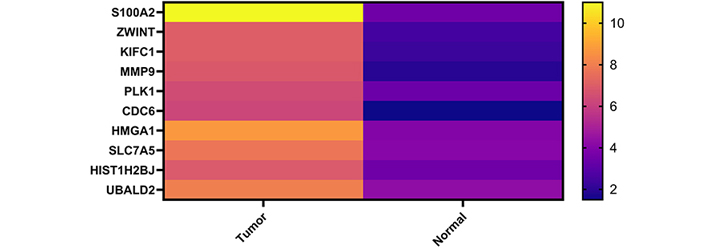
Heatmap image of selected genes. All selected genes were overexpressed in CESC samples. (Tumor and normal samples were compared in heatmap analysis: TCGA Tumor samples vs. TCGA normal and GTEx normal tissue samples)
Enrichment analysis results
Pathway analysis showed that selected potential 10 target genes have been associated with many critical molecular cellular processes like cell cycle, and DNA replication Figure 4A. Enrichment analysis results showed that these genes could have crucial roles in the cancer process as seen in Figure 4B. They are also connected with many essential hub proteins, including the MYC oncogene and the TP53 tumor suppressor gene, as demonstrated in Figure 4C. A correlation study of selected genes revealed that four of them are connected with CDK1 and CDK2 genes, which are known key cancer-related genes in CESC, as can be seen in Figure 5.
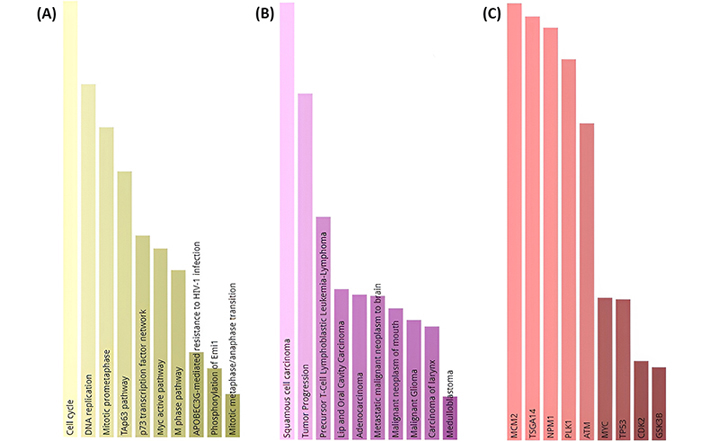
Enrichment analysis. (A) Selected potential 10 target genes have been linked to various fundamental molecular cellular processes. (B) Selected genes have been found to be related to many cancers according to the Disgenet database. (C) Hub proteins of selected 10 genes (Figure has been created using Enrichr. p < 0.05 for each of the figure’s bars)
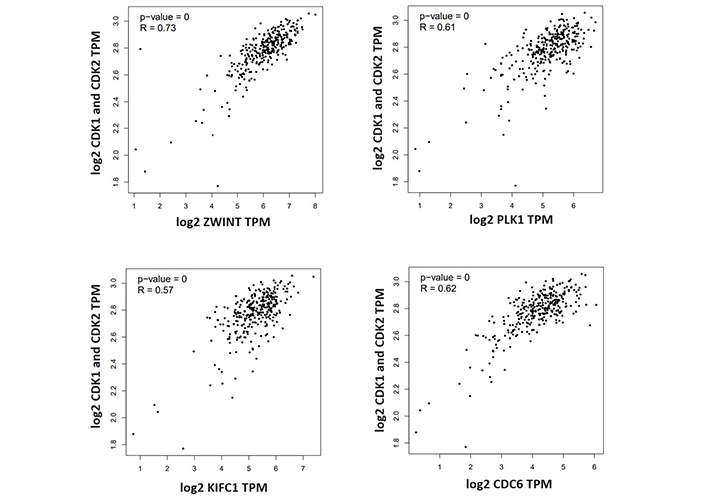
Selected target genes’ correlation analysis with CDK1 and CDK2 genes. ZWINT1, PLK1, KIFC1 and CDC6 genes found to be correlated with CDK1 and CDK2 genes
Expression change in selected miRNAs may affect overall survival
The all 3 selected miRNAs were found to have reduced expression in CESC samples compared to the control group (Figure 6). OS analysis in TCGA for CESC patients was performed for 3 selected miRNAs and 10 genes. It was found that the miR-361-3p and miR-296-5p miRNAs have prognostic importance for the OS rate of CESC patients (Figure 7). However, any one of the genes did not have a statistically significant effect on the OS of the CESC patients.
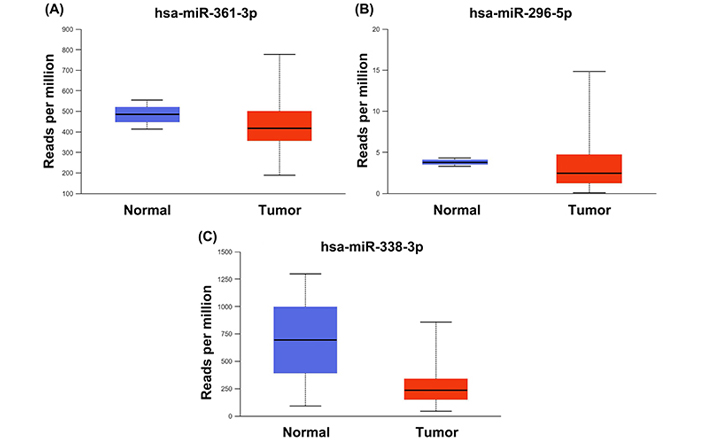
Selected 3 miRNAs’ expression rates in 307 tumor vs. 3 Normal CESC samples in TCGA database and these miRNAs effect on survival rates of CESC patients. Selected (A) hsa-miR-361-3p, (B) hsa-miR-296-5p, (C) hsa-miR-338-3p miRNAs have been found to be significantly downregulated in tumor samples (p < 0.001)
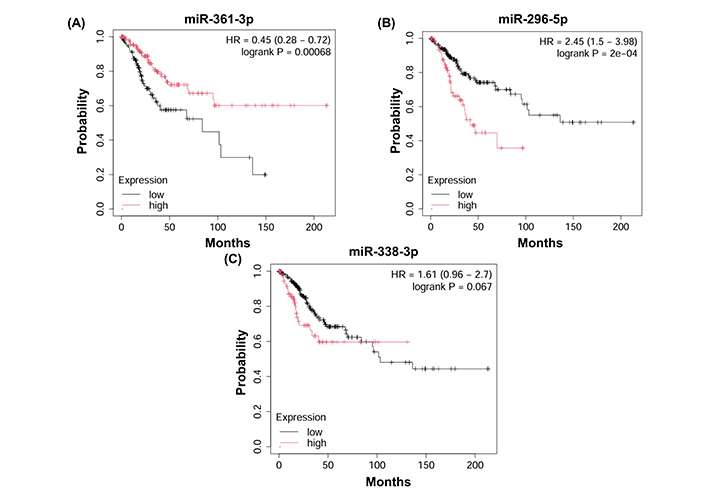
The effect of selected 3 miRNAs’ on overall survival rates of 307 CESC patients in the TCGA database. (A) Downregulated miR-361-3p was related to a lower overall survival rate. (B) A high miR-296-5p level was associated with a decreased overall survival rate. (C) Altered hsa-miR-338-3p expression level was not altered with CESC survival rates
Discussion
In human cells, the number of unique circRNAs formed (~100,000) is significantly greater than that of protein-coding genes (~20,000). Despite their widespread prevalence, the biological significance of most circRNAs remains unclear, and they have not been functionally characterized [21]. Studies have shown that miRNAs can serve as potent regulators of various cellular activities, including cell growth, differentiation, development, and apoptosis in many cancers [22–24]. The circRNA–miRNA–mRNA regulation axis plays a crucial role in the progression of several diseases, including malignancies, diabetes, Alzheimer’s disease, and cardiovascular disease [25–28]. These networks participate in the signaling mechanisms of several human illnesses by controlling the expression profile of genes linked to pathogenicity. To comprehend the complicated biological processes of cancer, the interaction between circRNAs/miRNAs and mRNAs needs to be clarified [29].
Large-scale bioinformatics databases have been increasingly used in medical research since genetic screening techniques were developed. Among these, GEO datasets stored in the NCBI and TCGA may be useful in the quantitative investigation and analysis of alterations in gene expressions of many diseases, including CESC [30]. For instance, based on GEO and the TCGA databases, Gao et al. [31] identified 4 miRNAs (miR-223, miR-99a, miR-188, and miR-125b) influencing the survival rate of individuals with CESC. In another study, Qi et al. [32] revealed 3 novel miRNAs (miR-7641, miR-585-5p, and miR-216b-5p) expression patterns might be used as a biomarker to indicate the poor prognosis of CESC. Recent studies have shown that circRNAs play key roles in CESC processes by altering the regulation of numerous oncogenes or tumor suppressor genes via miRNAs. For example, it has been found that circ0001955 enhances CESC carcinogenesis and metastasis via the miR-188-3p/NCAPG2 axis [33]. The circ_POLA2/miR-326/GNB1 axis has been found to be important in the development and progression of CESC [34].
Using various in silico approaches, circRNAs–miRNAs–mRNAs axes that may be significant in the CESC cancer process have been identified in current study. 3 circRNAs (hsa_circ_0000711, hsa_circ_0000515, and hsa_circ_0001855) that may be more associated with CESC were identified. To our knowledge, two studies in the literature regarding the relationship between hsa_circ_0000711 circRNA and cancers exist. However, there is no information about the relationship between hsa_circ_0000711 and CESC. In the study of Li et al. [35], it has been suggested that hsa_circ_0000711 may have a significant role in colorectal cancer and may be a possible biomarker for colorectal cancer diagnosis and prognosis. The increased expression of hsa_circ_0000711 has been shown to promote hepatoma cell proliferation and prevent apoptosis by binding has-miR-103a-3p [36]. Among the selected miRNAs in the current study, hsa-miR-338-3p and hsa-miR-361-3p, which are among the candidate potential sponges of hsa_circ_0000711, were shown to be downregulated in many cancers, including CESC. For instance, according to Luan et al.’s study [37], miR-338-3p was considerably downregulated in CC cells and tissues, while lncRNA XLOC_006390 was found to act as a ceRNA and accelerate CC progression and metastasis by reverse regulating miR-338-3p expression. In the study of Meng et al. [38] circUBAP2 has been shown to be a prognostic marker and it may contribute to tumor growth and metastasis in CESC by influencing the miR-361-3p/SOX4 axis. In Pubmed, there are two research that indicate a link between ‘hsa_circ_0000515’ and cancers. Cai et al. [39] showed that hsa_circ_0000515 is involved in developing breast cancer via the miR-296-5p/CXCL10 axis. Another study emphasized that hsa_circ_0000515 may play a role in the development of CESC through miR-326/ELK1 axis [40]. miR-296-5p found a candidate sponge miRNA of hsa_circ_0000515 in the current study is an important miRNA in many cancers including CESC. For example, KCNQ1OT1 has been demonstrated to accelerate the malignant behavior of CESC and promote tumor formation by altering the miR-296-5p/HYOU1 axis. The third circRNA that may be associated with CESC, ‘hsa_circ_0001855’, has not yet been associated with any cancer in the literature, and only one study has stated that it may be associated with preeclampsia [41].
The genes in our study’s proposed axes are involved in critical biological functions such as cell division and DNA replication and are linked to various malignancies (Figure 4). All of the selected 10 genes for our investigation have previously been proven to be elevated in many cancers including CESC. For instance, a recent study identified S100A2 as a hypoxia-induced immunosuppressive factor that regulates the expression of PD-L1. S100A2 has also been identified as an oncogene that enhances the proliferation and migration of CC cells, suggesting that it might be a potential therapeutic target for CESC [42]. There is sufficient evidence supporting PLK1 overexpression in CESC, and it has been documented that PLK1 overexpression contributes to the clinical progression of CESC patients [43]. ZBRK1 has been implicated as inhibiting CC metastasis, perhaps by modulating MMP9 expression [44].
Increasing evidence indicates that circRNAs have the potential to serve as indicators for early cancer detection, clinical diagnosis, prediction, and even in the evaluation of therapy response [21]. To reveal the effects of the hsa_circ_0000711/hsa-miR-338-3p/HMGA1, HIST1H2BJ, ZWINT, MMP9, KIFC1 and hsa_circ_0000515/hsa-miR-296-5p/S100A2, PLK1, SLC7A5, HMGA1 axes in CESC, it is necessary to conduct both in vitro and in vivo research. If the appropriate confirmation is achieved, these axes may be used as a biomarker for early diagnosis and in creating targeted therapy.
Conclusions
According to the findings, this reserch suggest that hsa_circ_0000711 may be involved in the CESC process hsa-miR-338-3p/HMGA1, HIST1H2BJ, ZWINT, MMP9, KIFC1 axis and also via hsa-miR-361-3p/KIFC1, UBALD2, CDC6 axis. Moreover, the results suggest that hsa_circ_0000515 may affect CESC processes by changing the expressions of S100A2, PLK1, SLC7A5, and HMGA1 genes through hsa-miR-296-5p. These circRNAs–miRNAs–mRNAs axes, which are considered to be associated with CESC, should be confirmed in vitro and in vivo with new investigations.
Limitations of the study
Although this study was conducted using TCGA data containing a large number of patient samples in mRNA and miRNA selection, GSE102686 and GSE169057 GEO datasets containing a small number of patient samples were used in determining circRNAs. The small number of patient samples can be considered as a limitation of the study. Despite this, circRNA is a new topic, we considered that our study can be considered a clue for in vitro and in vivo studies.
Abbreviations
CC: | cervical cancer |
CESC: | cervical squamous cell carcinoma |
CircInteractome: | circRNA interactome |
circRNAs: | circular RNAs |
GEO: | Gene Expression Omnibus |
GEPIA2: | The Gene Expression Profiling Interactive Analysis |
KM plotter: | Kaplan-Meier plotter |
Log2FC: | log2 Fold Change |
miRNA: | microRNA |
OS: | overall survival |
TCGA: | The Cancer Genome Atlas |
Declarations
Author contributions
MK: Conceptualization, Data curation, Formal Analysis, Investigation, Methodology, Resources, Validation, Visualization, Writing—original draft, Writing—review & editing.
Conflicts of interest
The author declare that he has no conflicts of interest.
Ethical approval
The study was prepared using publicly available bioinformatics data and does not require ethical approval.
Consent to participate
Not applicable.
Consent to publication
Not applicable.
Availability of data and materials
The datasets generated and analyzed for this study can be found in the GEO (https://www.ncbi.nlm.nih.gov/gds) and TCGA (https://cancergenome.nih.gov/). The author of this publication will provide the raw data to any competent researcher without hesitation.
Funding
Not applicable.
Copyright
© The Author(s) 2024.