Abstract
Aim:
Skin lesion segmentation is critical for early skin cancer detection. Challenges in automatic segmentation from dermoscopic images include variations in color, texture, and artifacts of indistinct lesion boundaries. This study aims to develop and evaluate MUCM-Net, a lightweight and efficient model for skin lesion segmentation, leveraging Mamba state-space models integrated with UCM-Net architecture optimized for mobile deployment and early skin cancer detection.
Methods:
MUCM-Net combines Convolutional Neural Networks (CNNs), multi-layer perceptions (MLPs), and Mamba elements into a hybrid feature learning module.
Results:
The model was trained and tested on the International Skin Imaging Collaboration (ISIC) 2017 and ISIC2018 datasets, consisting of 2,000 and 2,594 dermoscopic images, respectively. Critical metrics for evaluation included Dice Similarity Coefficient (DSC), sensitivity (SE), specificity (SP), and accuracy (ACC). The model’s computational efficiency was also assessed by measuring Giga Floating-point Operations Per Second (GFLOPS) and the number of parameters. MUCM-Net demonstrated superior performance in skin lesion segmentation with an average DSC of 0.91 on the ISIC2017 dataset and 0.89 on the ISIC2018 dataset, outperforming existing models. It achieved high SE (0.93), SP (0.95), and ACC (0.92) with low computational demands (0.055–0.064 GFLOPS).
Conclusions:
The model’s innovative Mamba-UCM layer significantly enhanced feature learning while maintaining efficiency that is suitable for mobile devices. MUCM-Net establishes a new standard in lightweight skin lesion segmentation, balancing exceptional ACC with efficient computational performance. Its ability to perform well on mobile devices makes it a scalable tool for early skin cancer detection in resource-limited settings. The open-source availability of MUCM-Net supports further research and collaboration, promoting advances in mobile health diagnostics and the fight against skin cancer. MUCM-Net source code will be posted on https://github.com/chunyuyuan/MUCM-Net.
Keywords
Medical image segmentation, lightweight model, mobile health, machine learning, skin cancerIntroduction
Skin cancer is primarily classified into two major types: melanoma and non-melanoma. Despite melanoma accounting for only 1% of skin cancer cases, it is responsible for the majority of skin cancer-related fatalities due to its aggressive behavior. In 2022, melanoma led to approximately 7,800 deaths in the United States, with an estimated 98,000 new cases anticipated in 2023 [1]. The lifetime risk for Americans developing skin cancer is alarmingly high, with current statistics showing that one in five individuals will be affected. This underscores the critical need for effective diagnostic and treatment approaches. The financial impact is also considerable, with skin cancer treatment in the U.S. exceeding $8.1 billion [2]. Malignant melanoma, in particular, is notorious for its rapid progression and high mortality rate, making early and accurate detection essential for improving patient outcomes. Dermatoscopy and dermoscopy play a vital role in the clinical evaluation of skin lesions, helping dermatologists to identify malignant characteristics [3–5]. However, manual interpretation can be time-consuming and error-prone, dependent on the clinician’s expertise. Recent advancements have introduced machine learning-driven techniques into clinical practice to improve diagnosis accuracy and efficiency. These techniques are particularly beneficial in computationally constrained environments like mobile health applications [6, 7].
Manual interpretation can be time-consuming, error-prone, and heavily dependent on the clinician’s expertise. Furthermore, certain medical samples present considerable challenges [8]. These challenges include indistinct boundaries where lesions merge seamlessly with the surrounding skin, making it difficult to delineate them clearly. Variations in lighting can alter the appearance of lesions, affecting the consistency of their visual features. Obstructions such as hair and bubbles may obscure lesion edges, complicating accurate segmentation. Additionally, variations in lesion size and shape, along with inconsistencies in imaging conditions and resolutions, can lead to inaccuracies in the segmentation process. Age-related skin changes can impact texture and appearance, further complicating detection. Complex backgrounds and differences in skin tone, influenced by factors such as race and environmental conditions, also contribute to the difficulty of achieving precise segmentation. Addressing these issues requires advanced techniques that can adapt to these varying conditions and ensure accurate diagnosis. Figure 1 presents some representative complex skin lesion samples.

Complex skin lesion samples. (A) Artifacts arising from the image acquisition process; (B) lesions obstructed by hair; (C) subtle differences between lesion and skin color; (D) low contrast between the wound and surrounding skin
Note. Source from International Skin Imaging Collaboration (ISIC) datasets [18–21]
Recent advancements in artificial intelligence (AI) have increasingly integrated computer-aided tools into clinical practice to enhance skin cancer diagnosis [9, 10]. A crucial technique in this process is skin cancer segmentation, which accurately delineates the boundaries of skin lesions in medical images. This segmentation is vital for accurately assessing lesion characteristics, monitoring their progression, and guiding treatment decisions. With rapid advancements in AI techniques and the widespread adoption of smart devices, such as point-of-care ultrasound (POCUS) devices or smartphones [11–13], AI-driven approaches for skin cancer detection have become popular.
Patients now enjoy enhanced access to medical information, remote monitoring, and tailored care, which has improved their overall satisfaction with healthcare services. Despite these positive changes, certain obstacles remain, particularly in medical diagnostics. A notable issue is the precise and efficient segmentation of skin lesions, which is critical for diagnosis but challenging to implement on devices with limited computational resources. Most AI-driven medical applications rely on deep learning techniques described in detail by [14]. These methods typically require significant computational power and extensive learning parameters to deliver accurate predictions, posing a challenge for integration into devices with constrained hardware capabilities [15, 16].
In this study, we extend our previous method UCM-Net [17], and introduce MUCM-Net, a lightweight, robust, and Mamba-powered approach for skin lesion segmentation. MUCM-Net leverages a new novel hybrid module that combines Convolutional Neural Networks (CNNs), multi-layer perceptions (MLPs), and Mamba to enhance feature learning. Utilizing new proposed group loss functions, our method surpasses existing Mamba-based techniques in skin lesion segmentation. Figure 2 presents the visualization of comparative experimental results on the International Skin Imaging Collaboration (ISIC) 2017 dataset [18, 19]. More results will be provided in the following “Results and Discussion” sessions.
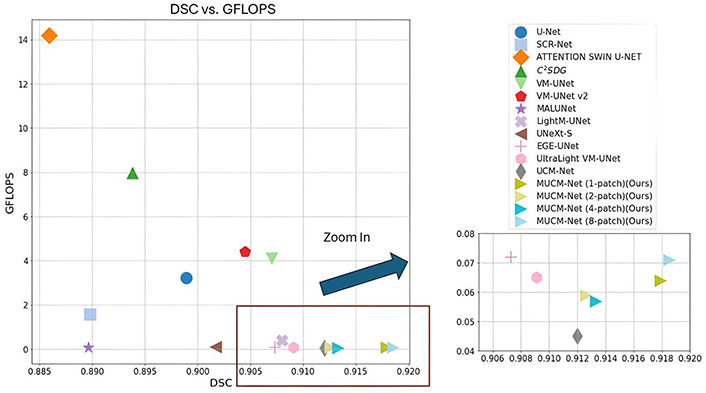
Comparative results on the International Skin Imaging Collaboration (ISIC) 2017 dataset. The X-axis shows the Dice Similarity Coefficient (DSC, higher values indicate better performance), and the Y-axis represents Giga Floating-point Operations Per Second (GFLOPS, lower values indicate greater efficiency). LightM-UNet’s result is out of range in the zoom-in window due to GFLOPS
Key contributions of MUCM-Net include:
Hybrid feature learning: The MUCM-Net block integrates CNN, MLP, and Mamba elements, enhancing the learning of complex and distinct lesion features. We explore the application of the Mamba structure to increase the feature learning ability.
Computational efficiency: MUCM-Net’s design, based on Mamba-UCM blocks and UCM-Net, prioritizes accuracy and efficiency. It achieves high prediction performance with low computational demands [approx. 0.055–0.064 Giga Floating-point Operations Per Second (GFLOPS)], making it suitable for various deployment scenarios.
Enhanced loss function: A novel loss function integrates output and internal stage losses, ensuring efficient learning during the model’s training process.
Superior results: MUCM-Net achieves exceptional results on the ISIC2017 [18, 19] and ISIC2018 datasets [20, 21], outperforming previous Mamba-based methods on metrics like Dice similarity, sensitivity, specificity, and accuracy.
Related works
Tiny machine learning (TinyML) for healthcare: Biomedical imaging segmentation, which involves accurately delineating anatomical structures and pathological regions from medical images, is essential for precise diagnostics. Recent advances in AI have significantly improved segmentation methods, enhancing both their accuracy and efficiency. A burgeoning area of research in this field is the integration of TinyML into healthcare, particularly for applications like lesion segmentation, offering exciting possibilities for both research and practical use.
TinyML refers to deploying machine learning models on low-power, compact hardware. This technology holds the potential to revolutionize healthcare by enabling advanced analytical capabilities directly at the point of care. It facilitates real-time, on-device processing, making sophisticated medical image analysis feasible even in settings with limited traditional computing resources or in mobile healthcare environments. For instance, using TinyML for lesion segmentation could provide immediate diagnostic feedback during patient consultations or in remote locations, significantly reducing dependence on extensive infrastructure typically needed for detailed analyses.
The incorporation of TinyML into medical devices is expected to enhance diagnostic accuracy, improve patient outcomes, and broaden access to advanced medical technologies in underserved regions. To optimize the deployment of TinyML in these critical applications, researchers are exploring advanced methods such as hyper-structure optimization [22] and leveraging efficient techniques like binary neural networks [23].
Hyper-structure optimization focuses on reducing the model’s parameter count without sacrificing performance, ensuring the models remain both practical and lightweight for use on miniature devices. Moreover, implementing binary neural networks helps streamline computations, further enhancing the practicality of TinyML applications in resource-constrained settings. As we delve into optimizing and applying models like MUCM-Net in healthcare. This research not only underscores the transformative possibilities of TinyML but also guides future explorations in deploying compact, efficient AI solutions in medical settings.
Supervised methods of segmentation: As AI technology continues to advance, the approaches for medical image segmentation have evolved significantly. Initially, the field heavily relied on CNNs such as U-Net, which is essential in medical image segmentation [24], and its attention-enhanced variant, Att-UNet [25], which incorporates attention mechanisms to further refine the segmentation accuracy by focusing on relevant features within the images. The development of hybrid architectures marks a further evolution in segmentation techniques. There are some hybrid-based UNets for medical image segmentation: (1) Transformers-related: such as TransUNet [26], TransFuse [27], and SANet [28]; (2) MLP-related: such as ConvNeXts [29], UNeXt [30], MALUNet [31] and its extended version EGE-UNet [32]. Recently, as Vision Mamba [33]’s image processing ability with fewer parameters and lower computations, Mamba-based hybrid structure UNets are becoming popular such as VM-UNet [34], VM-UNet V2 [35], LightM-UNet [36], and UltraLight VM-UNet [37].
State-space models (SSMs): SSMs have recently been recognized for their linear complexity concerning input size and memory usage, establishing them as fundamental components for lightweight model architectures [38]. SSMs are particularly effective at capturing long-range dependencies, offering a critical solution to the convolution challenge of processing information across extensive distances. With the advantage of SSMs, Mamba [39] has been proven to handle textual data with fewer parameters than Transformers. Similarly, the advent of Vision Mamba has advanced the application of SSMs in image processing, demonstrating a significant memory reduction, all without relying on traditional attention mechanisms. This pioneering research bolsters confidence in Mamba’s potential as a critical lightweight model component in future technological advancements.
In this paper, we extend our previous work UCM-Net to propose a new hybrid work MUCM-Net which engages the Mamba’s features learning ability and maintains fewer parameters and lower computations.
Materials and methods
MUCM-Net
Network structure design: Figure 3 presents a detailed overview of the structural framework of MUCM-Net, a cutting-edge architecture featuring a unique U-shape design, building upon UCM-Net. MUCM-Net consists of a down-sampling encoder and an up-sampling decoder, forming a robust network for skin lesion segmentation. The network is composed of six encoder-decoder stages, with channel capacities set at {8, 16, 24, 32, 48, 64}. The first stage in the encoder-decoder sequence is a convolutional block, responsible for extracting and capturing critical features. The subsequent stages incorporate our novel UCM-Net blocks, enhancing the network’s performance.
Convolution block: In our design, the first encoder-decoder stage employs a standard convolution layer with a 3 × 3 filter. This convolution block utilizes a 3 × 3 kernel, which is widely used to capture spatial relationships within input features. This kernel size is especially beneficial in the initial layers of the network, where maintaining the spatial integrity of feature maps is crucial for decoding complex input patterns. From the 2nd to the 6th stage, we incorporate a 1 × 1 filter convolution layer, which supports the subsequent Mamba-UCM block. This adjustment significantly reduces the number of learnable parameters and the overall computational load, optimizing the network’s efficiency.
Mamba-UCM block: The 2nd–6th stages mainly use the Mamba-UCM block for feature learning. The Mamba-UCM block showcases an advanced strategy that merges UCM-Net block, which contains CNNs with MLPs and Mamba block, to enhance feature learning. There are two advantages in the hybrid structure: (1) CNNs module can enable the model to extract the spatial feature; (2) MLPs and SSM can power the model’s pattern recognition capabilities. The process begins by reshaping the initial input feature map to meet the distinct requirements of CNNs, MLPs, and Mamba. This adaptation involves converting a four-dimensional tensor suitable for CNN processing into a three-dimensional tensor appropriate for MLP and Mamba operations. Inspired by UltraLight VM-UNet and Vision Mamba, we proposed four versions of MUCM-Net with different patch processing. Figure 4 presents the visible differences between the UCM-Net block and the two visions of Mamba-UCM blocks. The PyTorch-style pseudocode in Figure 5 presents our defined sequence of operations, which is how we combine the UCM block and the Mamba block for feature learning.
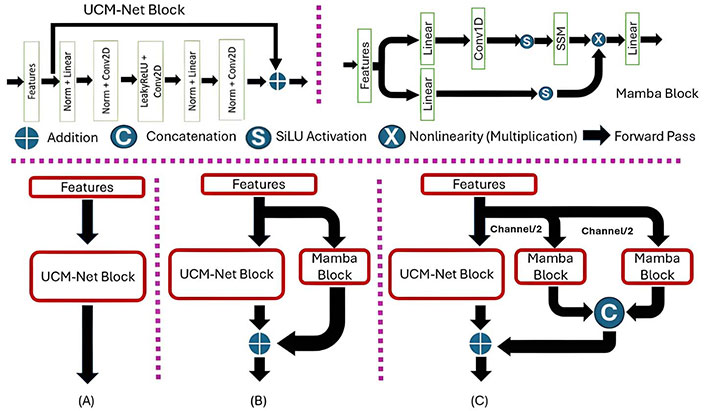
MUCM-Net Structure. (A) UCM-Net pipeline; (B) MUCM-Net (1-patch) pipeline; (C) MUCM-Net (2-patch) pipeline. SSM: state-space model; Norm: normalization; Conv2D: 2-dimensional convolution
Loss functions
In our solution, we designed a new group loss function similar to those used in TransFuse, EGE-UNet, and our previous work, UCM-Net. However, different from theirs, our proposed base loss function is calculated from Binary Cross-Entropy (BCE) loss (1) and Dice loss (2) components and square Dice loss (3) components to calculate the loss from the scaled layer masks in different stages compared with the ground truth masks.
The advantages of using different loss functions are as follows:
BCE loss: Widely used in classification tasks, including biomedical image segmentation, these losses are highly effective for pixel-level segmentation.
Dice loss: Commonly used in biomedical image segmentation, Dice loss addresses class imbalance by focusing on the overlap between predicted and true regions.
Square Dice loss: Further enhances the Dice loss by emphasizing the contribution of well-predicted regions, thereby improving stability and performance.
where N is the total number of pixels (for image segmentation) or elements (for other tasks), yi is the ground truth value, and pi is the predicted probability for the i-th element.
where we used a smooth constant, which equals 1e-5, to enhance numerical stability.
where the equation represents an improvement over the standard Dice loss by placing greater emphasis on the squared terms of intersections and unions.
Equations 1, 2, and 3 define the base loss function (Equation 4) for our proposed model, incorporating the Dice loss, and square Dice loss components. λi represents the weight assigned to different stages. We explore adding the difference between the up-sample from the stage with ground truth to the final loss. Because the large stage’s result should be close to the final prediction, we set λi to 0.1, 0.2, 0.3, 0.4, and 0.5 based on the i-th stage, as illustrated in Figure 3.
Equation 7 defines our proposed group loss function, which calculates the loss based on the scaled layer masks at various stages compared to the ground truth masks. Equations 5 and 6 detail the stage loss for different intermediate layers and the output loss for the final layer, respectively.
To prove the effectiveness of our proposed methods, we did an ablation experiment of various base loss functions and the experimental results are reported in the following Discussion section.
Results
Datasets: To evaluate the efficiency and performance of our proposed model with other published models, we picked the two public skin segmentation datasets from the ISIC, namely ISIC2017 and ISIC2018. The ISIC2017 dataset contains 2,000 dermoscopy images, while the ISIC2018 dataset includes 2,594 images. The ISIC2017 dataset was randomly divided into 1,250 for training, 150 for validation, and 600 for testing. The ISIC2018 dataset was randomly divided into 1,815 for training, 259 for validation, and 520 for testing.
Evaluation setting: Our MUCM-Net is implemented with the PyTorch [40] framework. All experiments are conducted on the instance node at Lambda [41] that has a single NVIDIA RTX A6000 GPU (24 GB), 14vCPUs, 46 GiB RAM, and 512 GiB SSD. We normalized and resized the images to 256 × 256 pixels. To enhance data sample diversity, we applied basic data augmentations, including horizontal and vertical flipping, as well as random rotation. This is because, although there are various and effective image augmentation techniques in the current literature [42], they may lead to an increase in the computational cost. We selected AdamW [43] for the optimizer, initialized with a learning rate of 0.001 and a weight decay of 0.01. The CosineAnnealingLR scheduler [44] is used with a maximum number of iterations set to 50 and a minimum learning rate of 1e-5. The model is trained for a total of 200 epochs with a training batch size of 8 and a testing batch size of 1.
Evaluation metrics: The model’s performance is evaluated using the Dice Similarity Coefficient (DSC), sensitivity (SE), specificity (SP), and accuracy (ACC). Furthermore, the model’s memory consumption is assessed based on the number of parameters and GFLOPS. DSC measures the degree of similarity between the ground truth and the predicted segmentation map. SE is used to measure the percentage of true positives in relation to the sum of true positives and false negatives. SP measures the percentage of true negatives in relation to the sum of true negatives and false positives. ACC measures the overall percentage of correct classifications. The formulas used are as follows:
where TP denotes true positive, TN denotes true negative, FP denotes false positive, and FN denotes false negative. In our benchmark tests, we evaluate the effectiveness of our approach and contrast it with other existing high-performing models.
Experiments results: Tables 1 and 2 present the segmentation prediction performance, while Table 3 provides an overview of the models’ physical performance.
Comparative prediction results on the ISIC2017 dataset
Dataset | Models | Year | DSC↑ | SE↑ | SP↑ | ACC↑ |
---|---|---|---|---|---|---|
ISIC2017 | U-Net* | 2015 | 0.8989 | 0.8793 | 0.9812 | 0.9613 |
SCR-Net* | 2021 | 0.8898 | 0.8497 | 0.9853 | 0.9588 | |
C2SDG* | 2021 | 0.8938 | 0.8859 | 0.9765 | 0.9588 | |
ATTENTION SWIN U-NET* | 2022 | 0.8859 | 0.8492 | 0.9847 | 0.9591 | |
MALUNet* | 2022 | 0.8896 | 0.8824 | 0.9762 | 0.9583 | |
UNeXt-S# | 2022 | 0.9017 | 0.8894 | 0.9806 | 0.9633 | |
EGE-UNet* | 2023 | 0.9073 | 0.8931 | 0.9816 | 0.9642 | |
VM-UNet* | 2024 | 0.9070 | 0.8837 | 0.9842 | 0.9645 | |
VM-UNet v2* | 2024 | 0.9045 | 0.8768 | 0.9849 | 0.9637 | |
LightM-UNet* | 2024 | 0.9080 | 0.8839 | 0.9846 | 0.9649 | |
UltraLight VM-UNet* | 2024 | 0.9091 | 0.9053 | 0.9790 | 0.9646 | |
UltraLight VM-UNet# | 2024 | 0.9097 | 0.9042 | 0.9804 | 0.9660 | |
UCM-Net (Baseline) | 2024 | 0.9120 | 0.8824 | 0.9877 | 0.9678 | |
MUCM-Net (1-patch) | 2024 | 0.9160 | 0.9090 | 0.9869 | 0.9689 | |
MUCM-Net (2-patch) | 2024 | 0.9126 | 0.9008 | 0.9829 | 0.9679 | |
MUCM-Net (4-patch) | 2024 | 0.9133 | 0.8871 | 0.9870 | 0.9681 | |
MUCM-Net (8-patch) | 2024 | 0.9185 | 0.9014 | 0.9857 | 0.9697 |
* the results cited from UltraLight VM-Net; # the results tested by us. ISIC: International Skin Imaging Collaboration; DSC: Dice Similarity Coefficient; SE: sensitivity; SP: specificity; ACC: accuracy. ↑: the higher the value, the better the performance
Comparative prediction results on the ISIC2018
Dataset | Models | Year | DSC↑ | SE↑ | SP↑ | ACC↑ |
---|---|---|---|---|---|---|
ISIC2018 | U-Net* | 2015 | 0.8851 | 0.8735 | 0.9744 | 0.9539 |
SCR-Net* | 2021 | 0.8886 | 0.8892 | 0.9714 | 0.9547 | |
C2SDG* | 2021 | 0.8806 | 0.8970 | 0.9643 | 0.9506 | |
ATTENTION SWIN U-NET* | 2022 | 0.8540 | 0.8057 | 0.9826 | 0.9480 | |
MALUNet* | 2022 | 0.8931 | 0.8890 | 0.9725 | 0.9548 | |
UNeXt-S# | 2022 | 0.8910 | 0.8577 | 0.9818 | 0.9555 | |
EGE-UNet* | 2023 | 0.8819 | 0.9009 | 0.9638 | 0.9510 | |
VM-UNet* | 2024 | 0.8891 | 0.8809 | 0.9743 | 0.9554 | |
VM-UNet v2* | 2024 | 0.8902 | 0.8959 | 0.9702 | 0.9551 | |
LightM-UNet* | 2024 | 0.8898 | 0.8829 | 0.9765 | 0.9555 | |
UltraLight VM-UNet* | 2024 | 0.8940 | 0.8680 | 0.9781 | 0.9558 | |
UltraLight VM-UNet# | 2024 | 0.8905 | 0.8724 | 0.9766 | 0.9545 | |
UCM-Net (Baseline) | 2024 | 0.9060 | 0.9041 | 0.9753 | 0.9602 | |
MUCM-Net (1-patch) | 2024 | 0.9111 | 0.8953 | 0.9811 | 0.9629 | |
MUCM-Net (2-patch) | 2024 | 0.9040 | 0.9151 | 0.9706 | 0.9588 | |
MUCM-Net (4-patch) | 2024 | 0.9058 | 0.8752 | 0.9846 | 0.9614 | |
MUCM-Net (8-patch) | 2024 | 0.9095 | 0.9046 | 0.9772 | 0.9618 |
* the results cited from UltraLight VM-Net; # the results tested by us. ISIC: International Skin Imaging Collaboration; DSC: Dice Similarity Coefficient; SE: sensitivity; SP: specificity; ACC: accuracy. ↑: the higher the value, the better the performance
Comparative performance results on models’ computations and the number of parameters
Models | Year | Params (millions)↓ | GFLOPS↓ |
---|---|---|---|
U-Net | 2015 | 2.009 | 3.224 |
SCR-Net | 2021 | 0.801 | 1.567 |
C2SDG | 2021 | 22.001 | 7.972 |
ATTENTION SWIN U-NET | 2022 | 46.91 | 14.181 |
MALUNet | 2022 | 0.175 | 0.083 |
UNeXt-S | 2022 | 0.253 | 0.104 |
EGE-UNet | 2023 | 0.053 | 0.072 |
VM-UNet | 2024 | 27.427 | 4.112 |
VM-UNet v2 | 2024 | 22.771 | 4.400 |
LightM-UNet | 2024 | 0.403 | 0.391 |
UltraLight VM-UNet | 2024 | 0.049 | 0.065 |
UCM-Net (Baseline) | 2024 | 0.047 | 0.045 |
MUCM-Net (1-patch) | 2024 | 0.139 | 0.064 |
MUCM-Net (2-patch) | 2024 | 0.100 | 0.059 |
MUCM-Net (4-patch) | 2024 | 0.081 | 0.057 |
MUCM-Net (8-patch) | 2024 | 0.071 | 0.055 |
GFLOPS: Giga Floating-point Operations Per Second. ↓: the lower the value, the better the performance
Discussion
Experimental result analysis
Tables 1 and 2 provide a thorough evaluation of the prediction performance of MUCM-Net, our novel Mamba-based skin lesion segmentation model, in comparison to established models. This assessment is conducted using the widely recognized ISIC2017 and ISIC2018 datasets. Introduced in 2024, MUCM-Net proves to be a robust and highly competitive solution in this domain. The key takeaway from these Tables is MUCM-Net’s ability to outperform all previous models, establishing a new state-of-the-art for lightweight skin lesion segmentation. Our model presents exceptional performance across various prediction metrics, underscoring its advancement in the field and potential to redefine the standard for accurate skin lesion delineation. To assess the physical performance of our proposed model, we compared its computational aspects and number of parameters with those of different segmentation models, as shown in Table 3. Remarkably, MUCM-Net (8-patch) operates with lower GFLOPS compared to other Mamba-based models. This efficiency does not come at the cost of performance, as MUCM-Net maintains high accuracy and robustness in segmentation tasks with the Mamba structure.
Ablation experiment on loss functions
To prove the effects of our proposed loss function, we conducted an ablation experiment using different base loss functions to train our model, MUCM-Net (1-patch). Table 4 presents the experimental results on the ISIC2017 dataset, providing a comparative analysis of the performance metrics for each loss function. The base loss functions evaluated include BCE loss (A), Dice loss (B), and square Dice loss (C). Additionally, combinations of these loss functions were tested: A + B, A + C, B + C, and A + B + C. The performance metrics considered were the DSC, SE, SP, and ACC. The training settings and hardware environment are the same as the benchmarks in Tables 1 and 2.
Ablation experiments on loss functions
Dataset | Base loss function | DSC↑ | SE↑ | SP↑ | ACC↑ |
---|---|---|---|---|---|
ISIC2017 | BCE loss (A) | 0.9130 | 0.8878 | 0.9867 | 0.9680 |
Dice loss (B) | 0.9152 | 0.9008 | 0.9814 | 0.9681 | |
Square Dice loss (C) | 0.9155 | 0.9051 | 0.9831 | 0.9684 | |
A + B | 0.9147 | 0.9078 | 0.9820 | 0.9680 | |
A + C | 0.9158 | 0.9010 | 0.9858 | 0.9677 | |
B + C | 0.9101 | 0.8854 | 0.9859 | 0.9669 | |
A + B + C (our proposed) | 0.9160 | 0.9089 | 0.9868 | 0.9690 |
ISIC: International Skin Imaging Collaboration; BCE: Binary Cross-Entropy; DSC: Dice Similarity Coefficient; SE: sensitivity; SP: specificity; ACC: accuracy. ↑: the higher the value, the better the performance
Table 4 reveals that individual loss functions and their combinations exhibit varying performance on the ISIC2017 dataset. Among the individual loss functions, BCE loss (A) demonstrates a solid overall performance with a high SP of 0.9867 and ACC of 0.9680. However, its SE is relatively lower at 0.8878. Dice loss (B) improves SE to 0.9008 and maintains high ACC (0.9681), while square Dice loss (C) further enhances SE to 0.9051 and retains high SP (0.9831) and ACC (0.9684). Combinations of these loss functions generally yield better results, with A + B and A + C showing improvements in DSC and SE. The proposed combination of A + B + C achieves the best overall performance, with the highest DSC (0.9160), SE (0.9089), SP (0.9868), and ACC (0.9690). This indicates that integrating all three loss functions leverages their strengths, resulting in a more robust model performance across all evaluation metrics.
In conclusion, this paper introduces MUCM-Net, an innovative and efficient solution that integrates CNN, MLP, and Mamba to offer robust feature learning while keeping parameter counts low and computational demands minimal. Applied to the challenging task of skin lesion segmentation, MUCM-Net has been rigorously tested across various evaluation metrics, demonstrating superior performance compared to other recent lightweight or Mamba-based models.
Looking ahead, we plan to extend the application of MUCM-Net to other critical medical imaging tasks, aiming to advance the field and explore its potential across a broader range of healthcare applications. Additionally, we plan to explore how MUCM-Net can be effectively integrated with established hand-crafted segmentation methods (e.g., from [45, 46]) to leverage their complementary strengths and potentially achieve even higher segmentation accuracy. We will also investigate methods to address adversarial noise attacks on skin cancer segmentation models [47, 48], enhancing MUCM-Net’s robustness against potential manipulations that could compromise its performance. Our goal is to push the boundaries of deep learning in healthcare applications, making significant contributions to medical imaging as well as other areas of healthcare.
Abbreviations
ACC: | accuracy |
AI: | artificial intelligence |
BCE: | Binary Cross-Entropy |
CNNs: | Convolutional Neural Networks |
DSC: | Dice Similarity Coefficient |
GFLOPS: | Giga Floating-point Operations Per Second |
ISIC: | International Skin Imaging Collaboration |
MLPs: | multi-layer perceptions |
SE: | sensitivity |
SP: | specificity |
SSMs: | state-space models |
TinyML: | tiny machine learning |
Supplementary materials
The supplementary figures for this article are available at: https://www.explorationpub.com/uploads/Article/file/1001250_sup_1.pdf.
Declarations
Author contributions
CY: Conceptualization, Investigation, Writing—original draft, Writing—review & editing. DZ: Validation, Writing—review & editing. SSA: Validation, Writing—review & editing, Supervision. All authors read and approved the submitted version.
Conflicts of interest
The authors declare that they have no conflicts of interest.
Ethical approval
Not applicable.
Consent to participate
Not applicable.
Consent to publication
Not applicable.
Availability of data and materials
The datasets analyzed for this study can be found in ISIC17 Dataset: https://challenge.isic-archive.com/data/#2017; ISIC18 Dataset: https://challenge.isic-archive.com/data/#2018; Code Repo: https://github.com/chunyuyuan/MUCM-Net.
Funding
Not applicable.
Copyright
© The Author(s) 2024.