Abstract
Aim:
Lung cancer is the leading cause of cancer-related deaths worldwide. Non-small cell lung cancer (NSCLC) is the most common subtype. Despite recent advancements in diagnostics and therapies, only a small percentage of patients benefit from immunotherapies. This underscores the urgent need to identify prognostic biomarkers for accurately assessing outcomes and providing treatment recommendations for NSCLC patients. Single-cell RNA sequencing (scRNA-seq) has revealed the heterogeneity of tumor-associated macrophages. Macrophages consist of M0, M1, and M2 subsets. M1 macrophages are often associated with improved clinical outcomes in various malignancies. However, there are no systematic studies on risk biomarkers for prognosticating NSCLC.
Methods:
CIBERSORT was used to calculate the macrophage subset infiltration percentage in bulk RNA-seq data from TCGA and GEO. The M1-related module was identified using the WGCNA algorithm. Potential M1 macrophage prognosis-associated genes were defined as the overlapping genes between marker genes in the M1 subpopulation from scRNA-seq data and prognosis-associated genes in M1 infiltrating cells.
Results:
Four risk genes (ADAM19, ICAM3, WIPF1, and LAP3) were identified through LASSO and multivariate Cox regression analysis. Forest plots demonstrated that the scoring model was an independent risk factor. A nomogram was provided to predict the prognosis of NSCLC patients. Finally, we validated the four risk genes at the protein expression levels and for copy number variations.
Conclusions:
In summary, our studies identified four risk genes related to M1 macrophages and presented a risk-scoring system to predict the outcomes of patients with NSCLC by integrating bulk and single-cell data.
Keywords
NSCLC, M1 macrophage, prognosis, TCGA, scRNA-seq, WGCNAIntroduction
With an estimated 1.8 million deaths annually from lung cancer, it is the most common type of cancer [1]. Regardless of the type, the 5-year survival rate for all lung cancer patients is 22%. Non-small cell lung cancer (NSCLC) has a 26% 5-year survival rate, compared to a 7% rate for SCLC [2–4]. Lung adenocarcinoma (LUAD) and lung squamous cell carcinoma (LUSC) are the most prevalent histological subtypes of NSCLC, accounting for about 85% of cases [5]. With the rapid development of precision medicine, new therapeutic approaches, particularly immunotherapies, have been suggested to improve clinical outcomes for NSCLC patients [6]. However, only a subset of patients benefit from immunotherapies, underscoring the urgent need to identify suitable biomarkers for accurate prognostic prediction.
In recent years, macrophages have emerged as novel therapeutic targets for cancer treatment. Tumor-associated macrophages (TAMs) are particularly crucial targets due to their influence on the tumor microenvironment (TME), tumor immunity, and response to immunotherapy [7]. Solid tumors and metastasis are prominent contributors to fatalities in NSCLC [8]. The TME plays an essential role in tumor cells’ growth and metastatic progression, which interact with immune cells [8, 9]. Leader et al. [10] highlighted that the composition of immune cells correlated with tumor mutational burden (TMB) serves as a crucial indicator of immunotherapy response in NSCLC, based on analysis of 35 matched single-cell RNA sequencing (scRNA-seq) samples. Macrophages exist in various states including non-activated M0, pro-inflammatory (M1), and anti-inflammatory (M2) consist of macrophages [11]. For many primary solid tumors such as ovarian cancer, a high M2/M1 ratio correlates with poor prognosis [8]. Zhao et al. [12] demonstrated that miR-934 promotes M1 depolarization, thereby facilitating colorectal cancer liver metastasis. In this study, we focused on M1 macrophages due to their high expression of major histocompatibility complex molecules and their effectiveness in killing cancer cells [13].
Weighted gene co-expression network analysis (WGCNA) is a widely used algorithm for clustering genes to identify modules highly correlated with clinical characteristics [14]. WGCNA has diverse applications, including discovering disease-associated gene modules, distinguishing gene sets specific to disease subtypes, and identifying gene lists involved in biological processes [15–20]. For instance, ITGAX, CCL14, ADHFE1, and HOXB13 were proposed as early molecular biomarkers for diagnosing gastric cancer by analyzing expression patterns using WGCNA [16]. Niemira et al. [15] used WGCNA to differentiate gene modules and functional pathways between LUAD and LUSC by investigating differentially expressed genes (DEGs) from bulk transcriptomic data. Similarly, essential gene modules and key genes were identified in breast cancer through WGCNA analysis [20]. In this study, we constructed a scale-free network using WGCNA to identify a group of co-expressed genes related to M1 macrophage in NSCLC patients. This approach is based on the hypothesis that co-expressed genes within the same module share comparable biological properties [21, 22].
Single-cell technology represents a sophisticated, powerful, and up-to-date technology for addressing issues of heterogeneity [23]. Until recently, researchers identified diagnostic and therapeutic molecular biomarkers using traditional bulk RNA-seq technology, which detected DEGs at an “average” status, requiring large sample sizes. The challenge lies in identifying specific cell types involved in cancer progression and development, particularly with scarce and challenging-to-obtain samples. Nowadays, single-cell genomics enables examination of patient intra-diversity at the resolution of individual cells [24]. Analysis of extensive scRNA-seq data from cancer, para-cancerous tissues, and peripheral blood samples is gradually illuminating the TME and disease mechanisms [25–27]. Hence, we now possess the capability to identify key marker genes in NSCLC patients using readily accessible scRNA-seq data.
The combined research of bulk RNA-seq and scRNA-seq is widely applied in cancer-related studies to better understand cancer heterogeneity and provide insights for precision medicine. For example, Zheng et al. [28] identified a cancer-associated fibroblast signature consisting of nine genes, which serves as a robust and reliable prognostic biomarker for colorectal cancer. Wang et al. [29] developed a machine-learning model by integrating scRNA-seq with bulk RNA-seq data, revealing that high-risk cohorts had lower immune infiltration and poorer prognosis in ovarian cancer. Bao et al. [30] suggested that a TAM-associated signature could predict immune response and aggressiveness in triple-negative breast cancer. Twenty-seven natural killer (NK) cell-associated genes were screened to develop a risk score model for prostate cancer, demonstrating reasonable predictive performance for patient outcomes [31]. However, the development of an M1-targeting algorithm for identifying prognostic risk-scoring systems in NSCLC has yet to be achieved through the integration of bulk and single-cell technologies.
In the current study, we constructed a classification system based on the percentage of M1 macrophage immune infiltration, concluding that high M1 infiltration is associated with a better prognosis compared to low M1 infiltration. A gene module related to the M1 trait was identified using WGCNA analysis. By integrating scRNA-seq data, we identified maker genes from the M1 subcluster. Eleven risk genes that play important roles in NSCLC through M1 immune cells were identified. After removing highly correlated risk genes using the LASSO algorithm, a risk score model was generated for the prognosis of NSCLC patients based on four M1 macrophage-related genes (ADAM19, ICAM3, WIPF1, and LAP3). Finally, these four genes were validated using immunohistochemistry (IHC) results from the Human Protein Altas (HPA) database. Our results provided a prognostic signature, identified potential therapeutic targets, offered a fresh perspective for understanding the mechanisms of NSCLC, and provided medical recommendations for NSCLC patients.
Materials and methods
Data collection and preprocessing
In total, four bulk datasets and one single-cell dataset for NSCLC were collected and combined in this research.
The TCGAbiolinks R package (version: 2.25.0) was used to download the clinical characteristics and gene expression data of LUAD and LUSC patients from the TCGA database [32]. We retained only the expression data that had matching clinical traits. GSE13213 and GSE31210 are two external datasets in this study. The GSE13213 dataset, based on the Agilent platform, contains gene expression microarray profiles and clinical information for 117 patients with LUAD [33]. The GSE31210 dataset, based on the Affymetrix platform, contains gene expression microarray profiles and clinical information for 226 LUAD samples included in our study [34, 35]. The removeBatchEffect() function in the limma R package (version: 3.52.1) was used to remove the batch effect among the four bulk RNA-seq datasets (TCGA-LUAD, TCGA-LUSC, GSE13213, and GSE31210).
There are 190 single-cell datasets, comprising 6,297,320 cells, available from TISCH2 (Tumor Immune Single-Cell Hub, http://tisch.comp-genomics.org/) [36].
We processed data from the study with accession number GSE117570, which includes 11,485 cells from four patients [37, 38].
Macrophage subclusters infiltration analysis
To assess the proportions of M0, M1, and M2 macrophage subclasses in NSCLC samples from four bulk datasets—two TCGA RNA-seq datasets and two external GEO microarray datasets, the CIBERSORTx R tool (https://cibersortx.stanford.edu/) was used to estimate their abundance. This tool, designed and developed by Prof. Alizadeh and Prof. Newman, is a popular method for providing the percentages of 22 different immune cell types by analyzing gene expression profile data [39, 40].
Samples were divided into two groups based on the median immune score of each macrophage subcluster type as follows (Algorithm 1).
WGCNA analysis
First, we filtered the genes based on the following criteria: genes in the top 75% of the median absolute deviation (MAD) and with MAD greater than 0.01. We removed genes that exhibited minimal variation across all samples because they were considered to have little effect on distinguishing between high- and low-M1 macrophage patient groups. Then, samples were screened to remove outliers. Finally, WGCNA was performed using the WGCNA R package (version 1.71) to identify gene modules most associated with M1 macrophage after dividing patients into high- or low-M1 macrophage content groups based on the median M1 infiltration score [14].
The pickSoftThreshold() function of WGCNA was used to determine the soft power value (beta). The next step was to use hierarchical clustering to find gene modules, with a minimum of 100 genes per module (minModuleSize = 100). Subsequently, the relationships between modules and sample characteristics were analyzed. Finally, the genes in the most relevant module were identified and defined as M1-related genes.
scRNA-seq analysis
The R package Seurat (version: 4.1.1) was used to conduct scRNA-seq data analysis [41–44]. Genes detected in fewer than three cells and cells with fewer than 200 detectable genes were disregarded, and the proportion of mitochondrial genes was restricted to under 30%. The doubletFinder_v3() function of the DoubleFinder R package (version: 2.0.3) was used to identify and remove doublet cells in the scRNA-seq data [45]. The LogNormalize method was selected for normalizing data using the NormalizeData() function. We analyzed the four scRNA-seq datasets from four patients separately and then merged them.
The criteria for removing principal components (PCs) were as followings: (1) a cumulative contribution rate greater than 90%; (2) each PC contributing less than 5% to the variance; (3) a difference of less than 0.1% between two consecutive PCs. The resolution was determined using the clustree R package (version: 0.5.0). Increasing the resolution resulted in more interlacing of clusters, indicating that our classification was becoming excessive.
Annotation was performed using the SingleR (version: 1.10.0) and celldex (version: 1.6.0) R packages [46]. The HumanPrimaryCellAtlasData() dataset served as the reference. tSNE was used for dimensionality reduction. Marker genes in subpopulations were identified using the FindAllMarkers() function in the Seurat R package with the Wilcox test. Cutoff values were set at log fold change threshold (logfc.threshold) = 0.25 and minimum percent (min.pct) = 0.25. Finally, only positively expressed genes were retained (only.pos = TRUE).
A similar protocol was used for recluster analysis of the macrophage subpopulation. The difference was in the annotation process, where cell types were assigned based on a combination of marker genes and functional analysis results, rather than auto-annotation. To assess the precision of our reclustering system, we utilized AUCell (version: 1.18.1) in our study [47]. AUCell enables the identification of cells with active, specific gene expression signatures. RUNX3, ADAM19, LIMD2, PRKCB, CYTIP, FNBP1, ICAM3, WIPF1, TAP1, PSMB9, and LAP3 were defined as M1 marker genes. Additionally, the topGO R package (version: 2.48.0) was employed to explore the functional characteristics within each cluster.
Identification of M1-related risk genes
The M1-related risk genes were defined as a class of group genes that do not exhibit linear relationships. They are overlapping genes between the first gene list from the M1-related gene co-expression module and the second gene list from marker genes in the M1 subcluster. First, we intersected the two gene lists and then removed co-linear genes based on LASSO analysis. LASSO analysis was performed using the glmnet (version: 4.1.4) and survival (version: 3.4.0) R packages to avoid overfitting problems.
Construction of the prognostic signature
By concentrating on significant M1-related macrophage risk genes, identified from the overlapping genes between the pink module from WGCNA and maker genes from the M1 subcluster, we created a prognosis-associated signature for NSCLC patients. Overall survival (OS) was the outcome of this study, and prognostic M1-related risk genes were examined using a multivariate Cox regression model on the training dataset. Genes were considered candidate prognostic genes if they had a p-value < 0.1 in the regression model.
The mathematical formula used to calculate the M1-related prognostic signature was:
where βi represents the coefficient value from Cox regression analysis, expi represents the expression levels for gene i, and n represents the number of significant risk genes.
The schematic workflow for designing and validating the prognostic signature in NSCLC is shown in Figure 1.
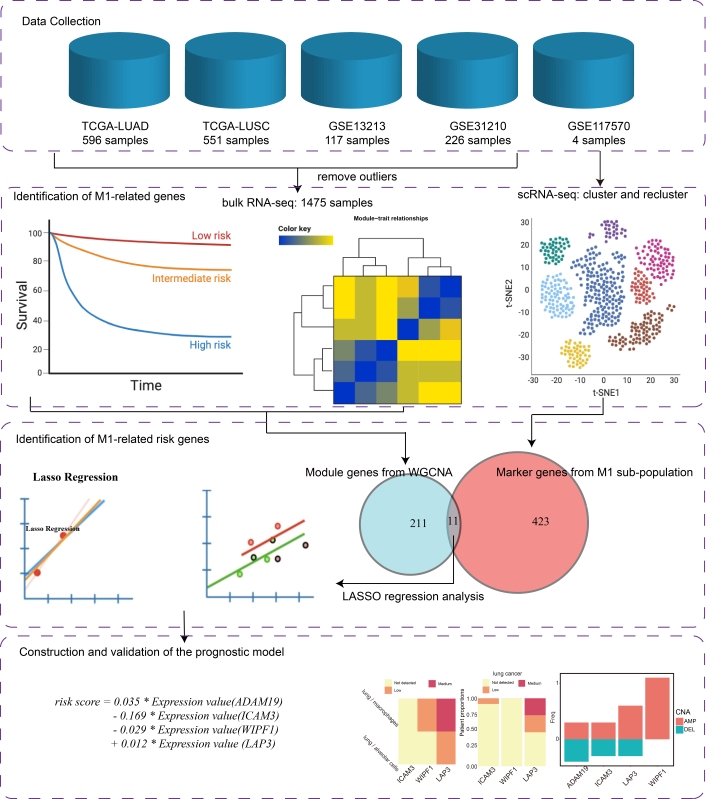
The schematic workflow of a comprehensive study on constructing and evaluating a risk score model based on M1 macrophage-related genes for calculating prognostic risk in NSCLC patients. Step 1. Four bulk RNA-seq and one scRNA-seq datasets were collected for this study. Step 2. Two M1-related gene lists were identified separately using WGCNA and maker gene screening algorithms. Step 3. The candidate M1-related factor genes were defined as the overlap between module genes and marker genes in the M1 sub-population. These factor genes were then filtered using LASSO regression analysis. Step 4. A risk score model was constructed using Cox regression analysis and validated by protein and copy number variation (CNV) levels
Univariate and multivariate Cox regression analysis
Two R packages, survival (version: 3.4.0) and survminer (version: 0.4.9), were used to determine the best cutoff of risk scores. NSCLC patients were then divided into high- and low-risk groups based on the optimal risk score cutoff.
Univariate and multivariate Cox regression analyses were conducted to evaluate whether age, gender, stage, and risk score are independent risk factors. Two R packages, survival (version: 3.4.0) and forestplot (version: 2.0.1), were used for assessment and visualization. Risk factors with p-value < 0.05 and HR > 1 were regarded as significant independent risk factors for poor prognosis in NSCLC patients.
Protein expression validation
The protein expression levels of risk genes in the risk score model were obtained and compared using the HPAanalyze R package (version: 1.14.0) [48]. HPAanalyze provides an interface for accessing and visualizing data from the HPA database (https://www.proteinatlas.org/). HPA is an open-source website for downloading and visualizing human protein data in cell lines, normal tissues, and tumors [49–55]. The protein expression profiles of risk genes in lung cancer and normal lung tissue were calculated and visualized separately using the hpaVisPatho() and hpaVisTissue() functions from quantified IHC staining results. A heatmap displaying the expression of relevant proteins in low, medium, and high levels was created.
Functional enrichment validation
To comprehensively validate the functional differences between high- and low-risk score groups, we included three popular functional enrichment analysis methods in our study: Metascape [56], GSEA, and ssGSEA.
DEGs between high- and low-risk score groups were identified using the limma R package (version: 3.52.1) [57]. Genes with |Fold change| > 1.5 and p-value < 0.05 were treated as significant DEGs. Up-regulated and down-regulated genes in the high-risk score group were separately uploaded to Metascape (https://metascape.org/gp/index.html#/main/step1). Enriched terms were downloaded in a bar graph summary format.
KEGG pathways gene sets were downloaded from the MSigDB database (https://www.gsea-msigdb.org/gsea/msigdb) [58–60]. Enrichment analysis and figure plotting were performed using the GSEA() and gseaplot2() functions from the clusterProfiler (version: 4.4.4) and enrichplot (version: 1.16.1) R packages.
Cancer hallmark gene sets were collected from the MSigDB database. The gsva() function in GSVA (version: 1.44.2) and GSEABase (version: 1.58.0) packages were used to calculate the enrichment score of each gene set in each sample using the ssGSEA algorithm . The fortify_mantel() function in the ggcor R package (version: 0.9.7) was employed to visualize the relationship between enrich score and risk score.
Other bioinformatics and statistical analysis
This study utilized R language (version 4.2.2) for bioinformatics and statistical analysis. The significant cutoff for p-values was set at 0.05. Kaplan-Meier analysis was performed to assess survival differences between patient groups. Additionally, a t-test was employed to analyze gene scores among M0, M1, and M2 groups. The nomogram illustrating the relationship between the sum of risk factors scores and survival time was generated using the regplot R package (version: 1.1). Genomic variation analysis included the collection of copy number variation (CNV) data in NSCLC from the cBioPortal database (https://www.cbioportal.org/). The frequencies of CNV changes for risk genes were shown by the ggplot2 R package (version: 3.3.6). The VennDiagram R package (version: 1.7.3) was utilized to compute and visually represent overlapping genes between the M1 characteristics-related gene list and the M1 marker gene list.
Results
M0, M1, and M2 infiltration percentage and survival analysis
In total, the GSE13213 dataset contained 117 samples and 19,412 genes, while the GSE31210 dataset included 226 samples and 20,857 genes. The TCGA-LUAD dataset comprised 596 samples and 59,427 genes, and the TCGA-LUSC dataset had 551 samples and 59,427 genes. These four datasets were integrated to mitigate batch effects (Figure S1). Finally, after removing samples without clinical information and excluding normal samples, we obtained data from 1,475 samples representing 1,432 patients and 15,596 genes for further prognostic analysis.
The expression profile was input into the CIBERSORT() function of the CIBERSORTx R tool (https://cibersortx.stanford.edu/). This allowed the calculation of the immune score for each cell type in each sample. Subsequently, samples were grouped based on the median immune score for M0, M1, and M2 categories individually. The survival curve in the M1 classification system exhibited the most significant differences between high and low immune score groups compared to other macrophage subclusters (Figure 2A–C). Therefore, our focus shifted to identifying M1-related risk genes for subsequent prognostic analysis in NSCLC.
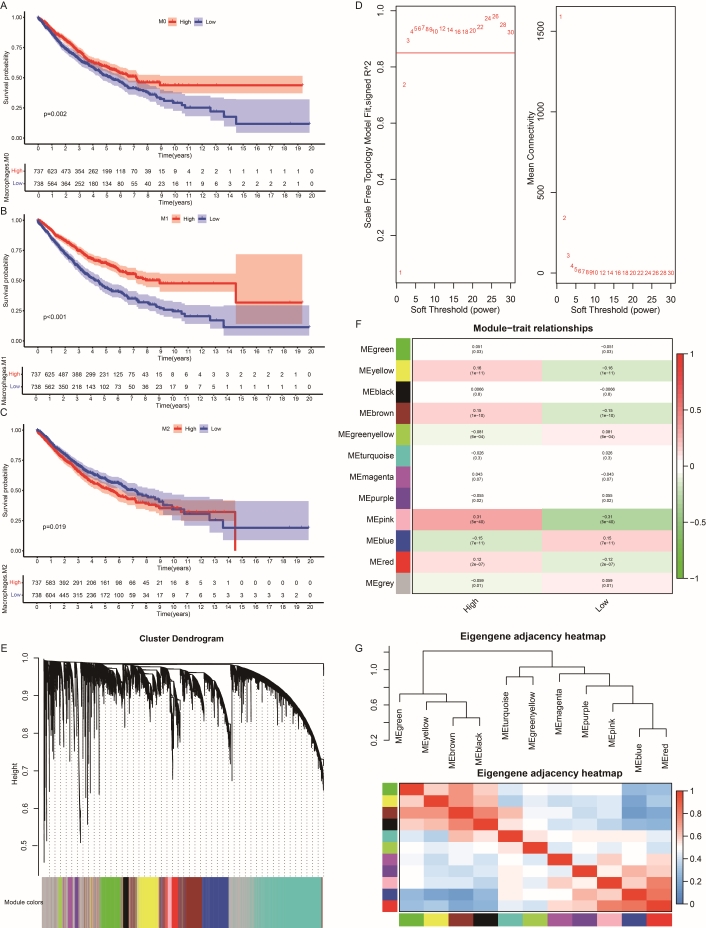
The survival analysis of M0, M1, and M2 macrophage subclasses and identification of M1 macrophage genes in NSCLC. Kaplan-Meier survival curves of patients with different macrophage infiltration subclasses: (A) M0 macrophage. (B) M1 macrophage. (C) M2 macrophage. (D) Selection of the soft threshold to achieve a scale-free topology in the network. Left plot: scale-free topology fit index as a function of the soft-thresholding power. Right plot: mean connectivity as a function of the soft-thresholding power. (E) Hierarchical clustering of the TOM matrix and visualization of gene module hierarch, excluding genes in the grey module that cannot be classified into color modules. (F) Visualization of the module-trait relationship to identify which module is most correlated with M1 macrophage characteristics. (G) Clustering heatmap depicting the relationship between gene modules and traits, highlighting the significant correlation of the pink module with M1 macrophage traits
Identification of M1 macrophage-related genes by bulk dataset
After filtering genes based on the cutoff of MAD across all samples, 11,697 genes were retained. Outliers were removed, resulting in 1,773 samples. As visualized in Figure 2D, a soft power beta value of 3 was selected as optimal. Setting the minimum module size to 100 (Table 1) yielded 12 modules; genes in the grey module, which could not be classified into color modules, were ignored (Figure 2E). The pink module showed the strongest correlation with the M1 trait and no significant association with other modules (Figure 2F, G). Therefore, 222 genes in the pink module were identified and defined as M1 macrophage-related genes for NSCLC patients.
The number of genes in WGCNA modules
Module | The number of genes |
---|---|
Black | 234 |
Blue | 1,363 |
Brown | 1,179 |
Green | 873 |
Greenyellow | 141 |
Grey | 2,950 |
Magenta | 197 |
Pink | 222 |
Purple | 159 |
Red | 532 |
Turquoise | 2,880 |
Yellow | 967 |
Identification of M1 macrophage-related marker genes by scRNA-seq
The identification of M1 macrophage-related marker genes from scRNA-seq data in NSCLC patients involves two main steps. First, macrophage subpopulations are isolated from all single cells within NSCLC patients. Second, M1 macrophage-related marker genes are identified.
After filtering doublets, genes, and samples, we obtained the following datasets: 6,610 genes across 1,788 cells for the first patient, 9,317 genes across 1,250 cells for the second patient, 3,205 genes across, 319 cells for the third patient, and 7,454 features across 1,364 cells for the last patient (Figure S2A–C). The optimal number of PCs and resolution were set at 14 and 0.6, respectively (Figure S2D–G). Four Seurat objects were normalized and integrated, resulting in 21 clusters (Figure 3A, Figure S2H). Additionally, seven main cell subpopulations were identified through SingleR annotation: epithelial cells (1,851 cells), monocyte (957 cells), NK cells (676 cells), macrophage (980 cells), B cells (170 cells), fibroblasts (52 cells), and endothelial cells (71 cells), as shown in Figure 3B. The tSNE plot depicted the distribution of immune cells in the scRNA-seq data (Figure 3C). The classification of immune cells into various subtypes (e.g., T cells, B cells, macrophages) is crucial for understanding the composition of immune cell populations in samples. This classification is particularly important in studying diseases such as cancer, where the immune response plays a critical role. By categorizing immune cells, we gain insights into how the immune system is responding. Single-cell analysis enables the identification of rare or novel immune cell subpopulations that may significantly impact disease progression or treatment response. Furthermore, immune cell classification provides crucial context for interpreting other study results. For instance, variations in immune cell composition may correlate with clinical outcomes or other molecular findings. To validate our classification approach, a bubble plot featuring three marker genes per cell type was generated (Figure 3D). The distribution of each cell type was visualized in a stacked percentage bar plot (Figure 3E), revealing similar proportions across patients. Additionally, enriched functional pathways associated with different cell types were illustrated in Figure S3A–B (GO-MF and KEGG).
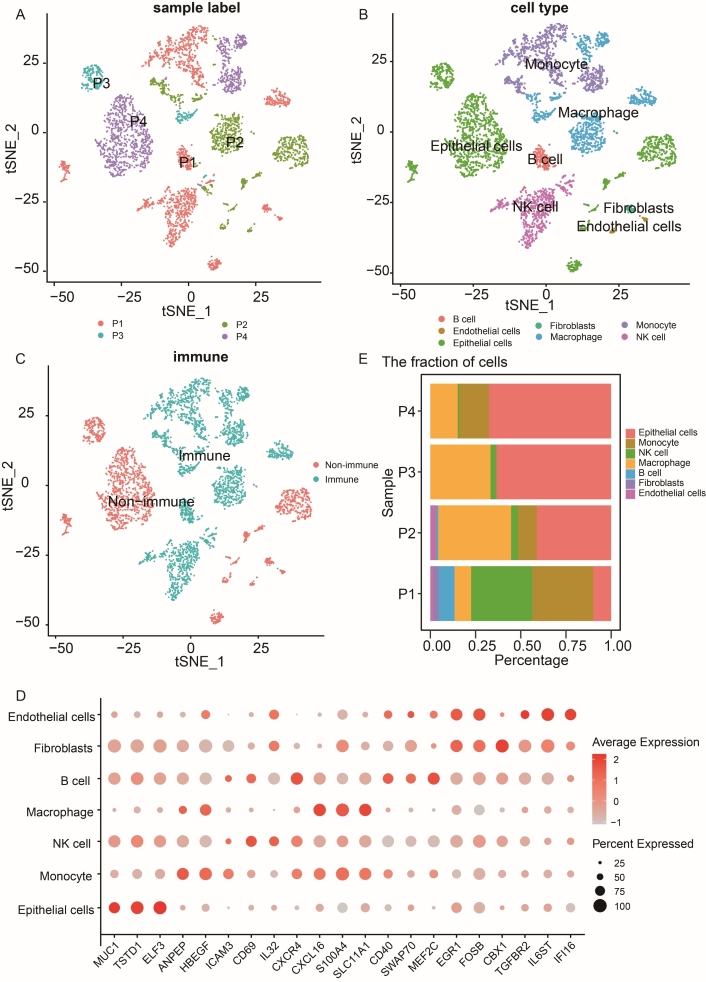
scRNA-seq analysis of NSCLC and identification of macrophage-related marker genes. (A) tSNE plot depicting the integration of 4,721 cells from four NSCLC patients. (B) tSNE plot displaying the main seven cell clusters identified in NSCLC samples based on 10× data. (C) tSNE plot illustrating the classification of cell clusters into immune and non-immune cells. (D) The bubble plot of representative three maker genes for seven cell clusters in NSCLC patients. (E) Stacked bar plot showing the proportion of seven cell clusters across the four NSCLC patients
The macrophage subpopulation was isolated from all single cells for further analysis (Figure 4A). We determined the optimal number of PCs and resolution to be 11 and 1.6, respectively. Figure 4B depicts the cell distribution of M0, M1, and M2 using tSNE visualization. Each cell’s AUCell score was calculated using the AUCell R package, with cells from the M1 cluster exhibiting higher scores than those from the other clusters (Figure 4C, D). Figure 4E illustrates the distribution of individual heterogeneity within cell clusters. Additionally, six marker genes and the top six DEGs are presented in Figure 4F. Enriched pathways for M0, M1, and M2 are detailed in Figure S3C–D, encompassing GO-MF and KEGG pathways. These findings collectively affirm the reliability of our cell type classification system.
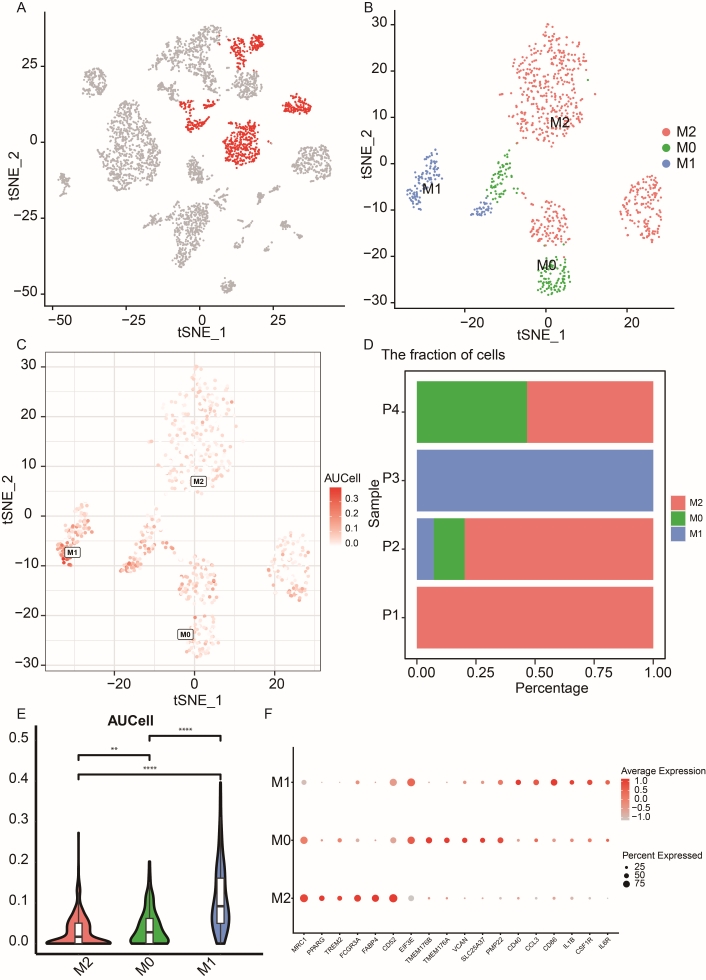
Recluster analysis of macrophage cell types in NSCLC patients. (A) tSNE plot depicting labeled macrophage cell clusters across all single cells. (B) tSNE representation illustrating the three main subpopulations of macrophages, M0, M1, and M2. (C) Analysis of M1-related gene set activity in single-cell RNA-seq data. (D) Stacked bar plot showing the proportion of the three main cell clusters across four NSCLC patients. (E) Violin plot displaying the AUC values of M1-related genes among the three macrophage cell types. The “**” denotes p-value < 0.01, and “****” denotes p-value < 0.0001. (F) Bubble plot presenting the expression levels and percentages of six marker genes for each macrophage subpopulation
Construction of the prognostic model by M1 macrophage-related risk genes
Eleven M1 macrophage-related risk genes were identified by intersecting the genes from the M1 trait-related and M1 marker gene lists (Figure 5A). LASSO regression analysis was subsequently applied to these 11 genes (RUNX3, ADAM19, LIMD2, PRKCB, CYTIP, FNBP1, ICAM3, WIPF1, TAP1, PSMB9, and LAP3) to mitigate model overfitting issues (Figures 5B, C). After eliminating genes with collinear associations, namely ADAM19, ICAM3, WIPF1, and LAP3, four risk genes remained significant with a p-value < 0.1. Table 2 summarizes the results from the Cox regression model. The risk score was defined based on the outcomes of the Cox regression analysis.
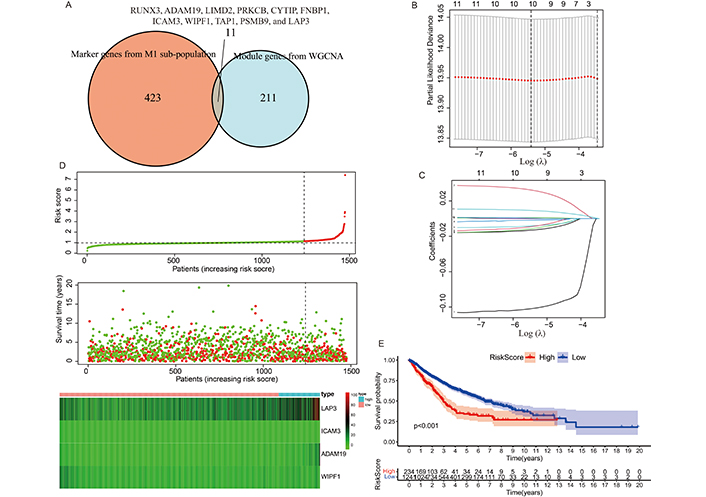
Selection of M1-related prognostic biomarkers and analysis of prognostic signatures. (A) Venn diagram illustrating the overlap of candidate M1-related genes identified from M1 trait-related and M1 marker gene lists. The overlapping 11 genes were RUNX3, ADAM19, LIMD2, PRKCB, CYTIP, FNBP1, ICAM3, WIPF1, TAP1, PSMB9, and LAP3. (B) (C) LASSO regression plots depicting dimension reduction to refine the selection of prognostic biomarkers. (D) Risk plot displaying the relationship between risk score and expression levels of four risk genes in the risk score model. (E) Kaplan-Meier survival curves comparing survival outcomes between low- and high-risk score groups in NSCLC patients
The coefficient of the risk factors in multivariate Cox regression model
Risk factor | Coefficient value | HR value | 95% CI (Low) | 95% CI (High) | p-value |
---|---|---|---|---|---|
ADAM19 | 0.035 | 1.035 | 1.012 | 1.059 | 0.003 |
ICAM3 | –0.169 | 0.845 | 0.719 | 0.993 | 0.041 |
WIPF1 | –0.029 | 0.972 | 0.941 | 1.004 | 0.083 |
LAP3 | 0.012 | 1.012 | 1.005 | 1.019 | 0.001 |
The first column indicates the risk gene, the second column indicates the coefficient value corresponding to its risk gene, the third column indicates the HR value, and the fourth and fifth columns show the HR value’s low and high 95% confidence interval, the last column shows the p-value
Subsequently, the risk score for each NSCLC patient was computed using the formula above. The risk plot demonstrated a correlation between risk score and gene expression levels, highlighting that elevated expression levels of ADAM19 and LAP3 are linked to poor prognosis in NSCLC patients, whereas lower expression levels of ICAM3 and WIPF1 are associated with favorable prognosis (Figure 5D). Figure 5E further illustrates a significant difference in survival time between low- and high-risk score groups among NSCLC patients.
The risk score signature is an independent prognostic factor
To integrate multi-omics perspectives, we incorporated protein expression data from the HPA database using the HPAanalyze R package. Figure 6A presents an accumulated percentage bar chart for three of the four prognostic risk genes (the protein expression data of ADAM19 is unavailable from the HPA database.). Consistent with dysregulation observed in transcriptomic analysis, WIPF1 protein levels were notably decreased in tumor lung samples compared to normal lung samples. Additionally, genomic variations were detected in these prognostic genes. For instance, we observed an increased frequency of copy number amplification for the LAP3 gene in the LUAD and LUSC cohort from cBioPortal (Figure 6B). Independent prognostic analysis was conducted to validate whether clinical characteristics and risk scores could serve as independent prognostic factors for NSCLC patients (Figure 6C, D). These results confirmed that age, gender, stage, and risk score are indeed independent risk factors. This suggests that these factors alone can predict outcomes in NSCLC patients without needing additional factors. A nomogram was generated using age, gender, stage, and risk score factors (Figure 6E). The nomogram facilitates the assessment of 3-, 5-, and 10-year survival rates for each NSCLC patient.
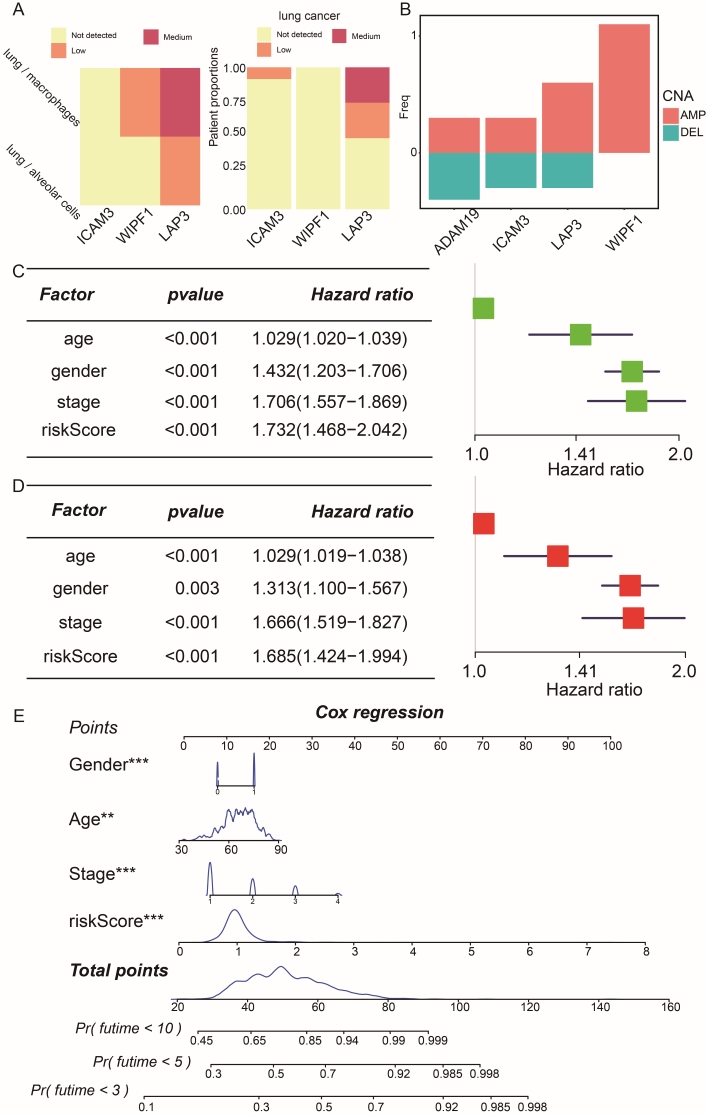
Performance of the risk score model constructed by prognostic genes. (A) Protein expression profiles of risk genes in normal lung tissue and NSCLC samples, highlighting dysregulation compared to normal cells. (B) Copy number variation (CNV) differences in risk genes within the risk score model. The horizontal axis represents the risk genes, while the vertical axis depicts the frequency of CNV events. Red indicates amplification, and green indicates deletion. Forest plot illustrating results from univariate (C) and multivariate (D) Cox prognostic regression models, displaying risk factors, 95% CIs, and HR values. HR < 1 and p-value < 0.05 indicate significantly better outcomes. (E) Nomogram for assessing the outcome of NSCLC patients, integrating age, gender, stage, and risk score into a prognostic risk score model. The “**” denotes p-value < 0.01, “***” denotes p-value < 0.001, and “****” denotes p-value < 0.0001
Dysfunctional pathways in high- and low-risk score groups
The differential expression analysis between high- and low-risk groups was conducted, and the results are presented in Figure 7A. The high-risk group exhibited 86 up- and 239 down-regulated genes. The up-regulated genes showed enrichment in pathways related to cell structure development, immune responses, and several signaling pathways, including skin and cartilage development, as well as cellular responses to interferon-gamma, IL-17, and Wnt signaling pathways (Figure 7B). Conversely, the down-regulated genes were enriched in pathways associated with disorders such as dopaminergic neurogenesis (Figure 7B). Additionally, GSEA corroborated these findings, highlighting enriched functional pathways among up-regulated genes, including the cell cycle, cytokine receptor interaction, antigen processing and presentation, and toll-like receptor signaling pathway.
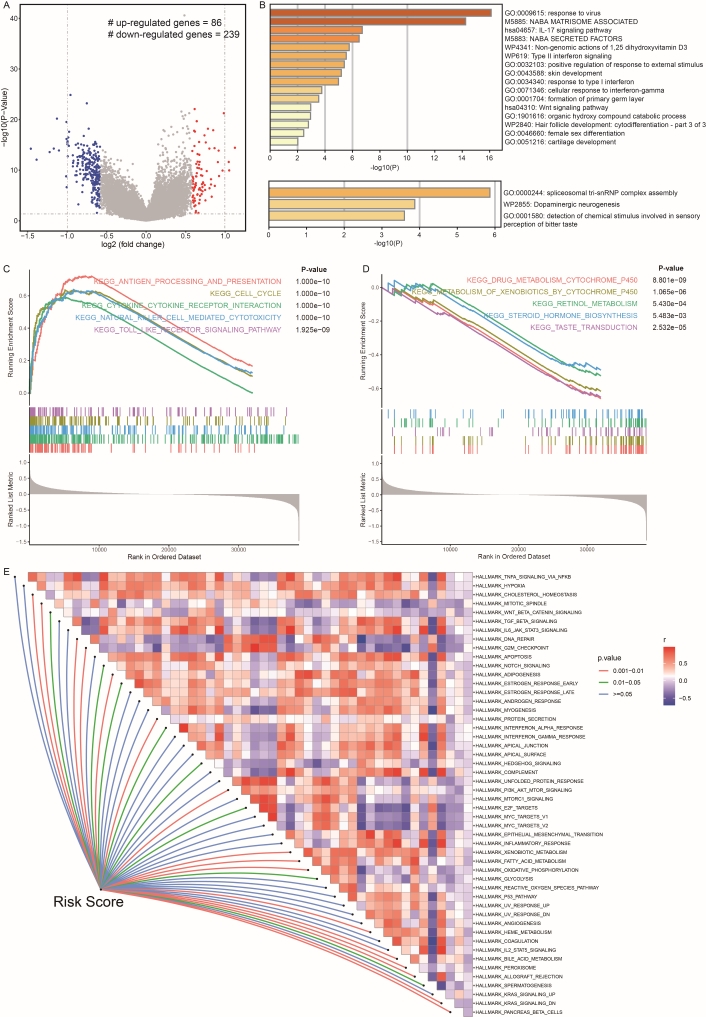
The predictive mechanisms and functional pathways of low- and high-risk score groups. (A) Volcano plot displaying gene expression levels in low- and high-risk score patients. The x-axis represents log2FC, while the y-axis represents –log10(p-value). Red dots indicate significantly up-regulated genes, and blue dots indicate significantly down-regulated genes in high-risk score samples. (B) GO term (molecular function category) enrichment analysis results for up- and down-regulated genes. The upper panel shows pathways enriched by up-regulated DEGs, while the lower panel shows pathways enriched by down-regulated DEGs. GSEA results reveal significant biological pathways enriched in high- (C) and low-risk score groups (D). The horizontal axis depicts the running enrichment score, while the vertical axis displays gene members sorted in a list. (E) ssGSEA results show the correlation between the risk score and activities of 50 cancer hallmark gene set pathways. Red indicates up-regulation in the high-risk score group, while purple indicates down-regulation in the high-risk score group
In contrast, down-regulated genes were enriched in pathways related to drug metabolism and steroid hormone biosynthesis (Figure 7C, D). To investigate the dysregulation pathways influenced by the risk score, we analyzed the relationship between the risk score and 50 cancer hallmarks. Figure 7E indicates that the risk score is significantly positively associated with DNA repair, adipogenesis, PI3K-AKT-mTOR signal, fatty acid metabolism, heme metabolism, bile acid metabolism, and oxidative metabolism. Conversely, the risk score is significantly negatively associated with G2M checkpoint, protein secretion, and peroxisome functions.
Discussion
Lung cancer remains the leading cause of cancer-related deaths worldwide [61, 62]. NSCLC accounts for approximately 80–85% of lung cancer cases [63]. Despite significant progress in understanding the pathological mechanisms of NSCLC over the past decades [5, 64–66], considerable challenges remain. One major limitation of current immunotherapies is their inability to effectively discriminate between targets, resulting in a 5-year survival rate of only 10–30% for all NSCLC patients [67, 68]. Macrophages, which are a crucial component of the human innate immune system, possess powerful capabilities to identify, phagocytize, and eliminate bacteria and foreign substances [69]. Typically, macrophages are classified into three distinct subtypes: M0, M1, and M2 [69]. TAMs are extensively studied in cancer diagnosis and treatment research. For example, monoamine oxidase A has been shown to reprogram TAMs and inhibit tumor cell proliferation in ovarian cancer by increasing oxidative stress, as demonstrated in a series of in vivo experiments [70, 71]. Additionally, autophagy-dependent ferroptosis promotes TAM polarization in pancreatic cancer through the release and absorption of the oncogenic KRAS protein [72]. However, the role of M1 macrophage infiltration in NSCLC remains unclear. To identify the best candidates for immune response-based therapies, it is essential to find accurate prognostic biomarkers that go beyond immune escape mechanisms.
In this study, we classified 1,475 samples into two groups based on the median infiltration levels of M0, M1, and M2 macrophages, respectively. The results of survival analysis curves demonstrated that the subgroups classified according to M1 immune cell infiltration exhibit the most significant difference in survival time. Interestingly, patients with high M1 infiltration have a longer survival time compared to those with low M1 infiltration. Through WGCNA analysis, we identified 222 co-expressed genes in the pink module associated with the M1 trait in NSCLC patients, while scRNA-seq analysis revealed 434 marker genes in the M1 subpopulation. We hypothesize that the overlapping genes play a crucial role in the development and progression of NSCLC by affecting the function of the M1 macrophage subcluster. To avoid overfitting caused by collinearly correlated genes, we employed LASSO regression. To assess the prognosis of NSCLC patients, we constructed a risk score model based on the Cox regression model, which combines the expression levels of risk genes with patients’ clinical information. Additionally, we integrated bulk and single-cell transcriptomic data with proteomic data to support our identified risk genes.
Two of the four M1 macrophage-related genes (ADAM19, ICAM3, WIPF1, and LAP3) have been reported to be associated with NSCLC. (1) ADAM19 (ADAM Metallopeptidase Domain 19), located on chromosome 5q33.3, is a protein-coding gene that is highly expressed in the adrenal glands, lungs, lymph nodes, prostate, and white blood cells, based on the Illumina BodyMap2 transcriptome project (https://www.ncbi.nlm.nih.gov/gene/8728). As a transmembrane protein, ADAM19 possesses both cell adhesion and protease activity due to its disintegrin and metalloprotease domain [73, 74]. ADAM19 is associated with immune cell infiltration and can predict the prognosis of various complex diseases, including osteosarcoma nephrogenesis and renal disease [75–77]. Macrophage infiltration, fibrotic gene expression, and CCL2 are directly enhanced by the upregulation of ADAM19, as demonstrated in vivo experiments [78]. The fibrogenic effects of ADAM19 were mitigated by using clodronate liposomes to deplete macrophages [78]. ADAM gene products have a broad spectrum of biological functions, including myogenesis, fertilization, and inflammatory response [73, 79]. Shan et al. [80] demonstrated that ADAM19 knockdown inhibited the migration and invasion of NSCLC cells in two in vitro experiments using the A549 and H157 cell lines. They found that the upregulation of ADAM19 was significantly associated with lymph node status and tumor stage. Wang et al. [81] reported that miR-145 inhibits cell invasion and metastasis by downregulating ADAM19 in NSCLC. These findings suggest that ADAM19 is a poor prognostic factor in NSCLC, which aligns with our conclusions. (2) ICAM3 (Intercellular Adhesion Molecule 3), located on chromosome 19p13.2, is a protein-coding gene that is highly expressed in the adrenal glands, lungs, lymph nodes, and white blood cells, as indicated by the Illumina BodyMap2 transcriptome project (https://www.ncbi.nlm.nih.gov/gene/3385). ICAM3 is involved in the intercellular adhesion of leukocytes and the maintenance of cell viability. ICAM3 also activates cancer cell proliferation through the PI3K/Akt pathway in lung cancer and induces radiation resistance through the FAK signaling pathway in cervical cancer [82, 83]. According to studies by Cassol and Saha et al. [84, 85], ICAM3 can mediate the polarization of macrophages in vitro. In the NSCLC cell line NCI-H1299, ICAM3 plays a crucial role in developing anti-cancer drug resistance, mediated by the ICAM3/Akt and ERK-CREB-2 protein pathways, independent of p53 and PTEN, according to Ahn et al. [86]. Park et al. [87] also reported that ICAM3 is a cancer diagnostic marker and anti-cancer drug target in NSCLC because it promotes cancer cell invasion and migration through the ICAM3/Akt/CREB/MMP pathway in the absence of p53 and PTEN. For the two remaining M1 macrophage-related genes (WIPF1 and LAP3), no results were available to report their prognostic roles in NSCLC. Thus, further research is necessary to explore how these genes affect the prognosis of patients with NSCLC through M1 macrophage immune regulation.
The risk score is calculated by a linear combination of the correlation coefficient and the expression of M1-related risk genes. It serves as an independent risk factor for predicting the prognosis of NSCLC. NSCLC patients were divided into two groups based on the risk scoring system using the optimal cutoff. The survival time differed significantly between high- and low-risk groups. The risk genes were validated at both the CNV and protein expression levels. LAP3 and WIPF1 are downregulated in lung cancer, while ICAM3 is upregulated compared to normal lung tissue, based on IHC experiments from the HPA database. WIPE1 and LAP3 exhibit significantly higher copy number amplification, whereas ADAM19 shows considerable copy number deletion in NSCLC patients. Although there is no detailed research on the mechanisms of these genes in lung cancer, they are reported to be critical to cancer development and influence patient outcomes. LAP3 overexpression plays a crucial role in tumor metastasis by promoting the proliferation, angiogenesis, migration, and invasion of malignant cells in breast cancer, hepatocellular carcinoma, and ovarian cancer [88–90]. Zhang et al. [91] reported that hypomethylation and upregulated WIPF1 are therapeutic targets for thyroid cancer activated by the BRAF V600E mutation. In pancreatic ductal adenocarcinoma patients, WIPF1 is associated with poor survival [92]. CNVs are structurally diverse regions where CNVs have been identified between two or more genomes [93]. Pan-cancer research has shown that most genes exhibit a positive linear relationship between copy number and gene expression (copy number amplification is associated with upregulation of the gene, while copy number deletion is associated with downregulation of the gene) [94]. Our conclusion aligns with the general theory about biomarkers in cancer, but further experiments using molecular functional methods are needed.
There are 86 significantly upregulated genes and 239 significantly downregulated genes in high-risk score cohorts compared to low-risk score cohorts. Additionally, the functional pathways between high- and low-risk score groups differ. Upregulated genes are mainly enriched in IL-17, type II interferon, Wnt, and PI3K-AKT-mTOR signaling pathways, and immunological reaction processes, such as cytokine-receptor interaction, antigen processing, and presentation, and cellular response to type I interferon. We also found a significant positive correlation between the risk score and common cancer hallmarks, including DNA repair, adipogenesis, and oxidative metabolism. Stewart [95] observed that WNT1, WNT2, WNT3, and WNT5A, as well as Wnt signaling pathway components Frizzled-8 and TCF4, are overexpressed in NSCLC patients and linked to poor prognosis. Some lncRNAs were identified as prognostic biomarkers for NSCLC. For example, BLACAT1 inhibits epithelial-mesenchymal transition by downregulating the Wnt/beta-catenin signaling pathway and is negatively related to the prognosis of NSCLC patients [96]. PKMY1AR enhances tumor stem cell maintenance by initiating the Wnt signaling pathway [97]. Thompson et al. [98] demonstrated that deficiencies in antigen presentation play a significant role in predicting immune checkpoint blockade in melanoma and lung cancer, summarizing that the antigen processing machinery score is associated with progression-free survival time. Oxidative stress and reactive oxygen stimulate carcinogenesis-related pathways in the respiratory system [99, 100]. Thus, the risk score might be a good system for predicting the outcome of NSCLC patients based on studies at the functional level.
Several limitations exist in the current study. First, the data source is singular, and the sample size is limited, which may introduce bias in the analysis results. Second, our study is retrospective, necessitating further prospective studies to validate the prognostic function of M1 macrophage-related signals. Third, the robustness of our prognostic model needs confirmation in other independent cohorts. Fourth, functional experiments are required to elucidate the potential molecular mechanisms for predicting the effects of M1 macrophage genes.
In conclusion, the four M1 macrophage-related genes and their signature may serve as molecular biomarkers for patients with NSCLC. Our research demonstrated that the risk score model is a reliable prognostic indicator for NSCLC and is associated with immunotherapy and features of immune infiltration. This study contributes to a comprehensive and systematic understanding of the role of M1 macrophages in the prognosis of NSCLC.
Abbreviations
CNV: | copy number variation |
DEGs: | differentially expressed genes |
HPA: | Human Protein Altas |
IHC: | immunohistochemistry |
LUAD: | lung adenocarcinoma |
LUSC: | lung squamous cell carcinoma |
MAD: | median absolute deviation |
NSCLC: | non-small cell lung cancer |
PCs: | principal components |
scRNA-seq: | single-cell RNA sequencing |
TAM: | tumor-associated macrophage |
TME: | tumor microenvironment |
WGCNA: | weighted gene co-expression network analysis |
Supplementary materials
The supplementary materials for this article are available at: https://www.explorationpub.com/uploads/Article/file/1001254_sup_1.pdf.
Declarations
Acknowledgments
The authors would like to thank the computational clusters source support from the Department of Computer Science, City University of Hong Kong.
Author contributions
ZL: Investigation, Formal analysis, Writing—original draft. FL, OOP, MT, and NC: Writing—review & editing. KW, ZZ: Conceptualization.
Conflicts of interest
All authors disclosed no relevant relationships. The authors reported no potential conflict of interest.
Ethical approval
Not applicable.
Consent to participate
Not applicable.
Consent to publication
Not applicable.
Availability of data and materials
The datasets collected in this research are from accessible databases. The article contains the accession number and the names of the databases. All code for data cleaning and analysis associated with the current submission is available at https://github.com/liuzhe93/M1_NSCLC. The raw data supporting the conclusions of this manuscript will be made available by the authors, without undue reservation, to any qualified researcher.
Funding
This research was substantially sponsored by the research projects [No. 32170654; No. 32000464] supported by the National Natural Science Foundation of China and was substantially supported by the Shenzhen Research Institute, City University of Hong Kong. This project is substantially funded by the Strategic Interdisciplinary Research Grant of City University of Hong Kong [No. 2021SIRG036]. The work described in this paper was substantially supported by the grant from the Health and Medical Research Fund, the Food and Health Bureau, The Government of the Hong Kong Special Administrative Region [07181426]. The work described in this paper was partially supported by the grants from City University of Hong Kong [CityU 11203520, CityU 11203221]. The funder had no role in study design, data collection and analysis, decision to publish, or preparation of the manuscript.
Copyright
© The Author(s) 2024.