Abstract
Aim:
Substance use disorders (SUD) result in substantial morbidity and mortality worldwide. Opioids, and to a lesser extent cocaine, contribute to a large percentage of this health burden. Despite their high heritability, few genetic risk loci have been identified for either opioid or cocaine dependence (OD or CD, respectively). A genome-wide association study of OD and CD related phenotypes reflecting the time between first self-reported use of these substances and a first DSM-IV dependence diagnosis was conducted.
Methods:
Cox proportional hazards regression in a discovery sample of 6,188 African-Americans (AAs) and 6,835 European-Americans (EAs) participants in a genetic study of multiple substance dependence phenotypes were used to test for association between genetic variants and these outcomes. The top findings were tested for replication in two independent cohorts.
Results:
In the discovery sample, three independent regions containing variants associated with time to dependence at P < 5 × 10−8 were identified, one (rs61835088 = 1.03 × 10−8) for cocaine in the combined EA-AA meta-analysis in the gene FAM78B on chromosome 1, and two for opioids in the AA portion of the sample in intergenic regions of chromosomes 4 (rs4860439, P = 1.37 × 10−8) and 9 (rs7032521, P = 3.30 × 10−8). After meta-analysis with data from the replication cohorts, the signal at rs61835088 improved (HR = 0.87, P = 3.71 × 10−9 and an intergenic SNP on chromosome 21 (rs2825295, HR = 1.14, P = 2.57 × 10−8) that missed the significance threshold in the AA discovery sample became genome-wide significant (GWS) for CD.
Conclusions:
Although the two GWS variants are not in genes with obvious links to SUD biology and have modest effect sizes, they are statistically robust and show evidence for association in independent samples. These results may point to novel pathways contributing to disease progression and highlight the utility of related phenotypes to better understand the genetics of SUDs.
Keywords
Substance use disorders, cocaine use disorders, opioid use disorders, genetics, FAM78B, GWASIntroduction
The opioid epidemic continues to cause human and economic devastation in the United States and elsewhere [1]. U.S. deaths due to the misuse of opioids reached a record high of more than 42,000 in 2016, which prompted the U.S. Department of Health and Human Services to declare opioid abuse to be a public health emergency in 2017. Several factors led to the epidemic, including the over-prescription of opioid analgesics, increased availability of heroin and illicit synthetic opioids [2], and possibly progressively worsening labor market opportunities, especially for those with low levels of education [3]. Despite early interventions to curb over-prescription, the epidemic continued to worsen [4]. Cocaine dependence (CD) has received less attention recently but its U.S. prevalence (likely significantly underreported [5]) remains at about 1%, resulting in a significant public health burden [6]. Cocaine users experience mortality rates four to eight times higher than the general population [7] and have an increased risk of suicide [8]. In contrast to opioid dependence (OD), pharmacological treatments for CD do not exist [9].
Moderate to high heritability estimates for both OD (43–50%) [10] and CD (65–78%) [11, 12] strongly suggest a genetic component to risk. Several OD risk genes have been identified through modestly-sized genome-wide association studies (GWAS) [13–16], but they have not been replicated and explain only a tiny fraction of the total trait heritability. Similarly, CD risk genes have been identified and there is evidence of genetic overlap between CD and other psychiatric disorders [17, 18], but collectively these results explain little trait heritability. Two relatively large OD GWAS papers have been published recently [Polimanti et al. [16] (4,503 OD cases) and Zhou et al. [19] (28,317 OD cases)]. These studies identified a single genome-wide significant (GWS) association for OD: a coding variant in the μ-opioid receptor gene OPRM1 (opioid receptor mu 1 gene). The sample sizes available for these studies still lag far behind those available for other complex diseases with a similar public health impact, and no large scale GWAS for CD has been performed. This problem is exacerbated by the fact that controls exposed to illicit drugs (a prerequisite for developing OD or CD) represent a small subset of the total control population. They may also be limited by the lack of differentiation between dependence upon prescription opioids and heroin, which may have a different set of risk factors.
In light of the deficits in our understanding of the genetic factors contributing to OD and CD risk, we have sought to derive phenotypes that are under more direct genetic control that could facilitate understanding the disorders and identification of potential drug targets and risk pathways. Our group recently identified variants near OPRM1 associated with usual methadone dosage in a sample of individuals with OD [20]. Here, we report findings from a GWAS of two related phenotypes: the time between first reported use and first DSM-IV diagnosis of OD and CD. We analyzed each substance independently. Both cocaine and opioids are highly addictive [21]. Approximately 50% of individuals who ever use heroin develop OD [22], while about four percent of people who try cocaine become addicted within two years, with another 16% in a prodromal stage of addiction [23]. A few studies (one conducted in the primary cohort analyzed here) have examined risk factors associated with rapid progression to dependence. Conduct disorder and childhood physical abuse predicted rapid more development of OD and CD, while and alcohol and nicotine dependence diagnoses were associated with slower progression, and African Americans (AAs) progressed to OD more rapidly than European Americans (EAs) [24].
There is also an interplay between use of medically indicated or recreationally used prescription opioids or heroin in determining who develops OD. Prescription opioids used for pain relief are generally safe when taken for a short time and as prescribed by a doctor. In a U.S. veteran population, individuals who used prescription opioids recreationally had 19 times the odds of initiating heroin use, fewer than four percent of people who abuse prescription opioids initiate using heroin within five years [25]. Despite our inability to distinguish between disease course with regard to specific opioids and their pattern of initiation, these related phenotypes have two properties that make them attractive for variant discovery: namely (1) they do not include individuals who were never exposed to opioids or cocaine and (2) they may be better indicators of an individual’s genetic risk for OD/CD than case-control status because they distinguish between cases who develop the disorder slowly (i.e. are more genetically resistant) and those who develop it rapidly (i.e. are highly genetically susceptible), assuming these differences are partially under genetic control.
Materials and methods
Participants and diagnostic procedures
Subjects for the study were recruited from three sources. The Yale-Penn discovery sample includes 6,188 AAs and 6,835 EAs participants who were ascertained for genetic studies of dependence on opioids, cocaine or alcohol between 2000 and 2017 by advertisement and through treatment settings at Yale University School of Medicine, University of Pennsylvania, University of Connecticut Health Center, the Medical University of South Carolina, University of Pennsylvania, and McLean Hospital in Belmont, Massachusetts [26, 27]. Psychiatric interviews were conducted using the Semi-Structured Assessment for Drug Dependence and Alcoholism (SSADDA) [28, 29].
A replication sample of EAs, informative for opioids but not cocaine, was derived from the Comorbidity and Trauma Study (CATS) [30, 31]. Briefly, opioid-dependent cases age 18 or older were recruited from opioid agonist treatment (OAT) clinics in metropolitan Sydney, Australia. Persons who had recent suicidal intent or psychosis were excluded. Controls were recruited from neighborhoods geographically proximal to the cases and excluded those who used opioids recreationally more than five times. Subjects were interviewed using the Semi-Structured Assessment for the Genetics of Alcoholism-Australia (SSAGA-OZ) [32] to derive DSM-IV substance use disorders (SUDs) diagnoses.
A second independent sample used for replication came from the Collaborative Study on the Genetics of Alcoholism (COGA), a large family-based study that recruited alcohol-dependent AA and EA probands from treatment facilities across seven sites in the United States [33, 34]. Probands and their families were invited to participate. Additional individuals and their families were recruited from the same communities. All participants were administered a version of the SSAGA [32, 35] which also queried about illicit drugs including opioids and cocaine.
Institutional review boards at all sites for all three studies approved the protocol and consent forms, and all participants provided written, informed consent according to the Helsinki Declaration Code of Ethics.
Phenotype definition
Analyses for cocaine and opioids were conducted independently; separate GWAS were performed for each outcome. Although individuals exposed to both opioids and cocaine contributed to both analyses, cocaine exposure/dependence did not inform analyses of opioids and vice versa. Time-to-dependence (TD) was defined as the interval between self-reported age at first opioid/cocaine use and the age at first self-reported endorsement of three or more DSM-IV OD/CD criteria within the same month. Individuals who used opioids or cocaine at least once but never became dependent were censored at their age at interview. No differentiation was made between exposure to or dependence on prescription vs. illicit opioids, but the majority (65%) of users in the Yale-Penn sample reported heroin as their most heavily used opioid.
Genotyping, imputation and quality control (QC)
DNA specimens in the Yale-Penn sample were genotyped on three microarrays: Yale-Penn 1 on the Illumina HumanOmni1-Quad v1.0 microarray (OMNI), Yale-Penn 2 on the Illumina Infinium Human Core Exome microarray (HCE), and Yale-Penn 3 on the Illumina Multi-ethnic Global Array (MEGA). QC of genotype data was performed as previously described [13]. Briefly, individuals and single nucleotide polymorphisms (SNPs) with a call rate < 98% and variants with minor allele frequency (MAF) < 1% were excluded prior to imputation. Pairwise identity-by-decent (IBD) was calculated with PLINK to determine genetic relatedness among individuals in the sample and individuals with a pairwise IBD estimate > 25% were assigned to the same family. Self-reported males with X chromosome heterozygosity > 20% and self-reported females with X chromosome heterozygosity < 20% were excluded. SNP genotype imputation was performed using the March 2012 1,000 Genomes reference panel (1,000 Genomes Project, 2012; http://www.1000genomes.org/) and IMPUTE2 [36] separately in AAs and EAs implemented on the Michigan imputation server (https://imputationserver.sph.umich.edu).
DNA specimens from the CATS sample were genotyped at the Center for Inherited Disease Research (CIDR) using the Illumina Human660W-Quad BeadChip. Genotype and sample QC was as described previously [9]. After initial QC, data were imputed to the 1,000 Genomes Phase 3 European ancestries reference panel using the Michigan Imputation Server. Details on the genotyping QC, and imputation of the COGA samples are described in detail elsewhere [37]. Briefly, samples were genotyped on multiple arrays in multiple labs. A subset of 47,000 common, independent, and high-quality SNPs that were genotyped across all arrays were used to assess duplicate samples, confirm the reported pedigree structure and compute ancestral principal components (PCs). After assignment of individuals in a family to a specific population, family-wise ancestry was designated according to the majority of individual family members. Genotypes were imputed to 1,000 Genomes using the cosmopolitan reference panel (Phase 3, version 5, NCBI GRCh37) using SHAPEIT2 [38] and Minimac3 [39].
After applying QC filters and phenotypic criteria, the Yale-Penn discovery sample included in analyses contained 1,307 AA and 2,340 EA OD cases and 974 AA and 768 EA opioid-exposed controls and 3,554 AA and 2,712 EA CD cases and 478 AA and 915 cocaine-exposed controls. The CATS replication sample contained 1,217 EA OD cases and 88 opioid-exposed controls. Demographic information and mean TD or censoring in the three cohorts are shown in Tables 1 (CD) and 2 (OD). The COGA replication sample included 144 AA and 334 EA OD cases and 354 AA and 1,305 opioid-exposed controls, 572 AA and 759 EA CD cases and 416 AA and 1,620 EA cocaine-exposed controls.
Demographic information on opioid-exposed individuals contributing to analysis of the trait time to opioid use disorder
Cohort | AA | EA | ||||||
---|---|---|---|---|---|---|---|---|
Age μ (SD) | Sex N (%) male | OD cases (N, mean years to dependence) | OD controls (N, mean years to censoring) | Age μ (SD) | Sex N (%) male | OD cases (N, mean years to dependence) | OD controls (N, mean years to censoring) | |
Yale-Penn | 42.2 (9.0) | 1,500 (66) | 1,307 (3.6) | 974 (14.9) | 36.7 (10.8) | 1,977 (64) | 2,340 (3.8) | 768 (13.9) |
COGA | 56.6 (13.7) | 325 (65) | 144 (4.3) | 354 (13.8) | 49.7 (14.6) | 1,047 (64) | 334 (3.4) | 1,305 (13.6) |
CATS | NA | NA | NA | NA | 36.5 (8.6) | 778 (59.6) | 1217 (3.8) | 88 (10.5) |
Statistical analysis
OD and CD TD analyses were performed separately and without considering an individual’s exposure or dependence status for the other drug. Analyses were stratified by genotyping platform and genetically determined ancestry and combined with inverse variance weighted meta-analysis. Variants with P-values < 1.0 × 10−5 were tested in the replication samples. Association tests were performed using Cox proportional hazards regression [40] implemented in the R package Survival (https://cran.r-project.org/web/views/ Survival.html). Cases had a lifetime DSM-IV OD or CD diagnosis and controls did not, although they could have endorsed two or fewer of the dependence criteria. All controls were exposed to cocaine or opioids (at least one reported use, including prescription) in their respective analyses. Imputed allele dosage was the predictor variable and models were adjusted for age (in COGA and CATS only, ten-year-age cohorts in COGA), sex, and the first five PCs (calculated within each ancestry group). The cluster option was used to account for the presence of related individuals in the samples by generating robust variance estimates. OD and CD TD analyses were performed separately and without considering an individual’s status on exposure to or dependence on the other drug. Analyses in the Yale-Penn and COGA samples were stratified by population and genotyping chip (in Yale-Penn) and all results were combined via inverse variance weighted meta-analysis using METAL (https://genome.sph.umich.edu/wiki/METAL) [41]. Analyses were stratified by cohort and ancestry (where applicable) and combined with inverse variance weighted meta-analysis. Variants with P-values < 1.0 × 10−5 were tested in the replication samples. The proportional hazards assumption was checked by verifying that the Schoenfeld residuals for each term in the model were independent of time. Both age at interview and age at fist cocaine/opioid use were significantly associated with TD in Yale-Penn but both violated the proportional hazards assumption and were not included in the final analysis in Yale-Penn. The top results were very similar with and without each age term in the model, but including an age by time interaction term attenuated them substantially.
The Yale-Penn results for CD and OD in EAs, AAs, and the combined meta-analyses were uploaded to the Functional Mapping and Annotation (FUMA) of GWAS portal [42], which performs functional mapping, gene based tests, and gene set enrichment analyses using GWAS summary statistics. Regional Manhattan plot were generated using Locuszoom software [43].
Results
Across all cohorts, the mean TD was shorter for opioids than cocaine (Tables 1 and 2). In Yale-Penn, older age at interview was strongly associated with longer TD for both CD [hazard ratio (HR) = 0.97 per year, P = 3.51 × 10−97] and OD (HR = 0.98 per year, P = 3.02 × 10−27). EA ancestry was associated with a longer TD for CD (HR = 0.79, P = 3.70 × 10−18) and a shorter TD for OD (HR = 1.43, P = 1.20 × 10−21). Female sex was associated with longer TD for CD (HR = 0.97, P = 0.046) and shorter TD for OD (HR = 1.12, P = 0.001). Shorter TD was significantly correlated with a higher number DSM-IV dependence criteria endorsed (i.e. more severe dependence), with Pearson correlation coefficients of 0.54 and 0.53 in AAs and EAs, respectively, for OD and 0.44 and 0.48 in AAs and EAs, respectively, for CD. The distribution of DSM-4 CD and OD criteria counts in Yale-Penn are shown in Supplemental Figure 1.
Demographic information on cocaine exposed individuals contributing the time to cocaine use disorder analysis
Cohort | AA | EA | ||||||
---|---|---|---|---|---|---|---|---|
Age μ (SD) | Sex N (%) male | CD cases (N, mean years to dependence) | CD controls (N, mean years to censoring) | Age μ (SD) | Sex N (%)male | CD (N, mean years to dependence) | CD controls (N, mean years to censoring) | |
Yale-Penn | 42.5 (8.3) | 2,419 (60) | 3,554 (5.5) | 478 (17.8) | 38.0 (11.1) | 2,237 (62) | 2,712 (4.9) | 915 (16.3) |
COGA | 56.9 (10.1) | 568 (57) | 572 (4.6) | 416 (13.8) | 52.1 (13.1) | 1380 (58) | 759 (3.9) | 1,620 (13.3) |
Boxplots for the distributions of age at onset and the TD for each substance in Yale-Penn are shown in Supplemental Figure 2. There were 4,989 individuals exposed to both cocaine and opioids that contributed to both analyses. There were 2,908 individuals with a lifetime DSM-4 dependence diagnosis for both drugs.
Discovery GWAS results
In the discovery sample, we identified tone region containing variants associated with TD at a GWS level (P < 5 × 10−8) in the gene family with sequence similarity 78-member B (FAM78B) on chromosome 1 (rs61835088, P = 2.96 × 10−8) for CD in the combined EA and AA sample. Each copy of minor (C) alleles at rs61835088 was associated with 0.57 fewer years between first use and CD diagnosis among those who converted. The Manhattan plots for CD and OD in Yale-Penn AAs and EAs are shown in Supplemental Figure 3. There was no evidence for inflation of the test statistics for either substance in either EAs or AAs (Supplemental Figure 4).
Gene and gene set analyses
No significant gene-based tests were observed after correcting for the number of valid gene-based test results, which varied by population and dependence outcome. The top ranked gene (P = 4.5 × 10−6) in gene based tests was calcium voltage-gated channel subunit alpha1 B (CACNA1B) in the EA CD analysis. One gene set (membrane depolarization) showed a significant enrichment, with six of 83 genes, including CACNA1B, showing nominally significant gene based test results after Bonferroni correction (P = 0.04) for CD in EAs.
Replication results
Three hundred and fifty one variants with suggestive P-values < 1.0 × 10−5 in the discovery sample (149 for CD, and 202 for OD) were tested in the replication samples. Several of these did not yield a valid result due to low MAF or imputation quality in their respective ancestry group or substance. Valid results were obtained for 142 CD SNPs and 200 OD SNPs in COGA and 170 OD SNPs in CATS. After correcting for the number of tests performed, none of the GWS hits in the discovery met criteria for replication. Several variants were at or near nominal significance with the same effect direction as Yale-Penn in COGA/CATS and improved the association signal after meta-analysis. The strongest signal in any of the replication samples was in the OD analysis with rs8063946 (P = 3.70 × 10−4 in COGA AAs) in the gene FTO alpha-ketoglutarate-dependent dioxygenase (FTO).
Discovery + replication results
After combining all results, the association of rs61835088 with CD TD strengthened (HR = 0.87, P = 3.71 × 10−9, Table 3, Figure 1). For the same outcome, the association with intergenic chromosome 21 SNP rs2825295 in AAs surpassed the GWS threshold in the combined Yale-Penn/COGA meta-analysis (P = 2.19 × 10−8, Table 3, Figure 1). Each minor (T) allele at rs2825295 was associated with 0.43 fewer years between first cocaine use and dependence among those who converted. The top variant in regions with P-values < 1.0 × 10−6 after combining all data are shown in Tables 3 (CD) and 4 (OD in AAs). For OD TD, all but one of the top associations was in the AA portion of the sample. There was one OD TD signal in the combined EA and AA sample, rs8063946 (P = 1.87 × 10−7) in the gene FTO, which for clarity is not shown in Table 4. Near-GWS evidence of association was obtained in the combined samples with SNPs in three other genes previously associated with addiction phenotypes: rs114341823 in GRIN2B [44, 45], which encodes the glutamate ionotropic receptor NMDA type subunit 2B was associated with OD TD in AAs (P = 1.45 × 10−7); the variant in FTO [46–48] described above; and rs73721103, which is between alpha-aminoadipic semialdehyde synthase (AASS) and LOC102724527 but is ~100 kilobases from PTPRZ1 [49, 50], which encodes the protein tyrosine phosphatase receptor type Z1 with CD TD in AAs (P = 2.81 × 10−7).
Top associations for time to CD
Chr | BP | rsID | A1 | A2 | Gene | Group | Yale-Penn | COGA | Yale-Penn + COGA | ||||||
---|---|---|---|---|---|---|---|---|---|---|---|---|---|---|---|
MAF | AA P | EA P | AA + EA P | AA P | EA P | HR | P | Direction | |||||||
1 | 166038842 | rs61835088 | T | C | FAM78B | All | 0.14 | 5.19E-08 | 2.55E-02 | 2.96E-08 | 0.89 | 0.01 | 0.87 | 3.71E-09 | -------- |
5 | 170894319 | rs112894747 | C | G | FGF18-LOC105377721 | AA | 0.03 | 9.01E-06 | NA | 9.01E-06 | 0.01 | NA | 1.41 | 4.76E-07 | +-++ |
7 | 90978616 | rs74426341 | C | G | LOC105375392 | All | 0.04 | 1.46E-06 | 5.70E-07 | 1.46E-06 | 0.08 | 0.62 | 1.37 | 2.52E-07 | ?+-+++++ |
7 | 90983464 | rs73404786 | A | G | LOC105375392 | EA | 0.04 | 2.75E-01 | 5.20E-08 | 4.19E-07 | 0.08 | 0.74 | 1.38 | 2.37E-07 | ++++ |
7 | 121807926 | rs73721103 | T | G | AASSLOC102724527 | AA | 0.05 | 6.57E-01 | NA | 2.76E-03 | 0.11 | NA | 1.30 | 4.00E-07 | ++++ |
21 | 20404237 | rs2825295 | T | G | AL157359.3-AP000431.1 | AA | 0.35 | 1.45E-07 | 3.15E-02 | 1.04E-07 | 0.07 | 0.83 | 1.14 | 2.57E-08 | ++++ |
The order of the symbols corresponds to Yale-Penn 1, Yale Penn 2, Yale-Penn 3, COGA. If AAs and EAs are combined, the AA symbol precedes the EA symbol. A1: effect allele; A2: non-effect allele; MAF: minor allele frequency in the subset of the Yale-Penn cohort indicated in the Group column; P: P-value; Direction: effect direction of A1
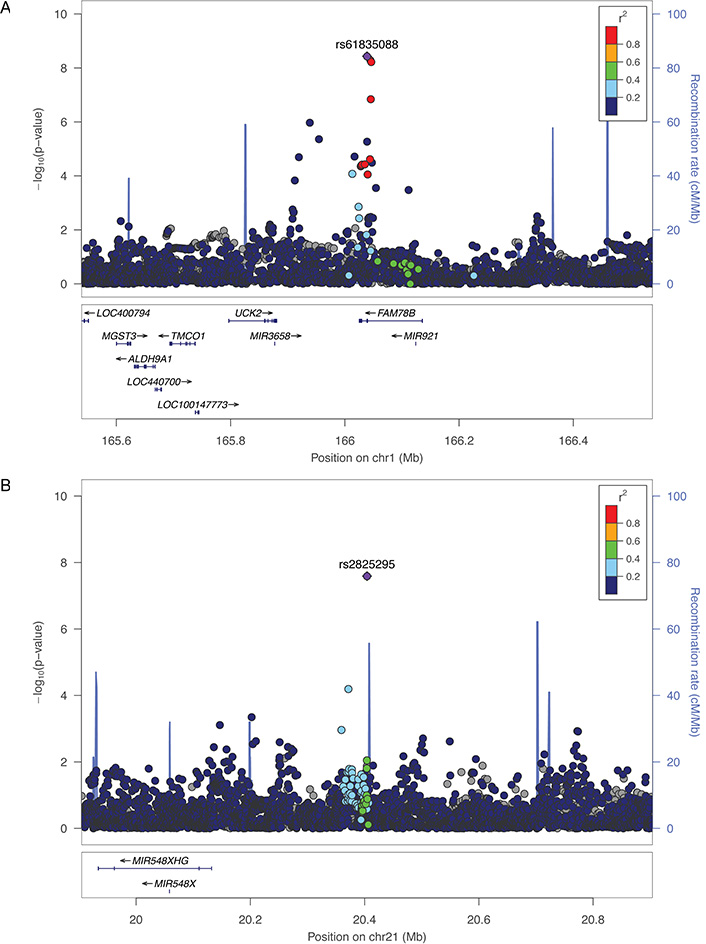
Regional Manhattan plots for the FAM78B region on chromosome 1 (A) and the intergenic region on chromosome 21 (B) for time to CD in AAs in the Yale-Penn cohort. SNPs are color coded according to the correlation coefficient (r2) in the 1,000 Genomes African reference panel with the top-ranked SNP. Light blue line indicates the observed recombination rate (right-side y-axis)
Top associations for time to OD in AAs
Chr | BP | rsID | A1 | A2 | GENE | Yale-Penn | COGA | Yale-Penn + COGA | |||
---|---|---|---|---|---|---|---|---|---|---|---|
MAF | P | P | HR | P | Direction | ||||||
2 | 8653036 | rs113142991 | A | C | LINC00299-LINC0184 | 0.07 | 1.81E-07 | 8.30E-01 | 1.57 | 6.48E-07 | +++- |
4 | 59826282 | rs4860439 | T | C | RP11-506N2.1 | 0.31 | 1.02E-07 | 6.50E-01 | 0.80 | 1.06E-07 | ---- |
4 | 95747400 | rs11728570 | T | C | BMPR1B | 0.37 | 1.08E-05 | 4.90E-02 | 0.82 | 1.07E-06 | ---- |
9 | 118707243 | rs115391335 | T | C | LINC00474-LOC105376236 | 0.03 | 5.55E-07 | 1.80E-01 | 1.72 | 1.11E-07 | +++- |
10 | 49574826 | rs1881736 | A | T | MAPK8 | 0.04 | 1.47E-05 | 7.60E-03 | 0.63 | 3.73E-07 | --+- |
12 | 14086651 | rs114341823 | T | C | GRIN2B | 0.03 | 8.75E-07 | 9.20E-02 | 1.70 | 1.77E-07 | ++++ |
12 | 53305274 | rs114198947 | A | G | KRT8 | 0.03 | 2.68E-06 | 8.80E-01 | 1.67 | 7.35E-07 | ++++ |
The order of the symbols corresponds to Yale-Penn 1, Yale Penn 2, Yale-Penn 3, COGA. A1: effect allele; A2: non-effect allele; MAF: minor allele frequency in the AA subset of the Yale-Penn cohort; P: P-value; Direction: effect direction of A1
Functional annotation
None of the variants we identified result in amino acid changes and any function they might have is likely regulatory, or as a result of linkage to a functional variant. Several of the top CD TD SNPs showed evidence for regulatory potential in various databases. According to the Genotype Tissue Expression database (https://gtexportal.org/home/) rs61835088 is a significant eQTL for FAM78B in tibial nerve tissue. This variant was also an eQTL for that gene in prefrontal cortex and blood according to QTLbase (http://mulinlab.org/qtlbase) [51]. Several variants, including rs61835088, rs73404786 were significant methylation QTLs (mQTL) in prefrontal cortex. Rs73721103 was a significant eQTL for the gene ring finger protein 133 (RNF133) in blood. Only one OD TD SNP showed evidence for regulatory potential: rs11728570 as a significant mQTL in blood. Neither SNP Function Prediction (https://snpinfo.niehs.nih.gov/) nor Braineac (http://braineacv2.inf.um.es/) provided any additional information about potential functionality of the top results.
Discussion
Here we present the results from GWASs of CD and OD related phenotypes measuring the time interval between first use of cocaine or opioids and a DSM-IV dependence diagnosis on the respective drug. These analyses, made possible by the extensive phenotyping performed on three independent cohorts, identified two GWS associations, one specific to AAs and one in the full sample, between relatively common variants and enhanced susceptibility to CD that were not detected at GWS in GWAS that used the bivariate case-control status for dependence as outcomes. Both of these were strengthened after combining meta-analysis with the replication sample. To our knowledge, this is the first time these trait definitions have been studied using GWAS. Although none of the GWS associations in the discovery sample were replicated after correcting for the number of variants tested in the COGA and CATS cohorts, 13 (9%) of the CD TD SNPs and 11 (5.5%) of the OD TD SNPs were nominally significant in one of those samples with the same effect direction.
The function of FAM78B, associated with progression from cocaine use to dependence, is not well understood but it is highly expressed in the brain [52] and interacts with several other proteins with diverse functions [53]. According to the STRING protein-protein interaction database (as https://string-db.org) [54], the proteins with which it is predicted to interact include ones related to mitochondrial function (ATP5F1), neurofilament network integrity (SNCG), and NOTCH signaling (XXYLT1).
Other genes that were among the top hits, but not GWS, have functions with potential links to SUDs. The genes GRIN2B and FTO, associated with progression from opioid use to dependence; and PTPRZ1, associated with cocaine TD have all previously been linked to SUD traits. Different variants than the one identified in this study in GRIN2B have been associated with OD [45] and chronic ketamine use [44]. Its protein product directly binds calcium/calmodulin-dependent protein kinase 2-alpha (encoded by CAMK2A), which can lead to synaptic long-term potentiation by facilitated CAMK2 response to synaptic calcium [55]. We previously identified this pathway as an important modulator of OD risk [13] and this result strengthens the evidence that an individual’s propensity to establish and reinforce substance-specific neural circuits may be an important factor driving their genetic predisposition to SUDs, and that calcium homeostasis may at least partially drive that propensity. FTO has also been associated with addictive behaviors. Although initially identified as a regulator of eating and obesity traits [56, 57], though probably not through an effect of FTO itself but rather as a consequence of variants in the gene that affect expression of IRX3 and IRX5 [56, 58]. FTO has also been associated with alcohol dependence [46–48] and connectivity in a dopamine-dependent reward circuit of meso-striato-prefrontal regions of the human brain [59]. PTPRZ1 binds to two neurotrophic cytokines [pleiotrophin (PTN) and midkine (MK)] that, along with other functions, contribute to the extinction of cocaine and amphetamine-seeking behaviors [49, 50] and limit morphine withdrawal syndrome [60]. The variants we identified, however, were ~100 KB away from the gene and may not strongly suggest a role for this gene in SUD risk. The top gene identified through gene based tests, CACNA1B in EAs for CD TD, encodes a pore-forming subunit of the presynaptic neuronal voltage-dependent calcium channel. Genes from this family of neuronal regulators of calcium and potassium levels were associated with their respective traits in our previous OD and CD GWAS papers [13, 18]. This, along with the fact that the membrane polarization gene set was significantly enriched among our results provides further evidence for the role of genetically determined differences in synaptic membrane potential acting as mediators of addiction risk.
We also looked up the results for the top TD SNPs in our previously published CD [18] and OD [13] GWAS of case-control status where controls were exposed to cocaine or opioids. There was modest evidence for association of each TD GWS variant identified with risk of dependence (not TD) on the respective substances, although not at the GWS level. Rs61835088 (P = 0.007) and rs2825295 (P = 0.001) were associated with binary CD diagnosis, and rs4860439 (P = 1.11 × 10−6) and rs7032521 (4.10 × 10−7) with OD diagnosis. The OPRM1 OD variant (rs1799971) identified by Polimanti et al. [16] and Zhou et al. [19] was nominally associated with OD TD in Yale-Penn EAs (P = 0.05).
Limitations
The primary limitation of this work is the relatively small sample size compared to other GWAS of complex disease. Also, the Yale-Penn sample had exclusion criteria for major psychiatric comorbidities including schizophrenia and suicidal ideation, which could have led to the exclusion of people with severe, possibly highly ‘genetically-driven’ CD or OD. Third, we did not detect GWS associations with our TD phenotypes with the top genes identified through the corresponding binary trait analyses, or vice versa. While we hypothesize that we may be detecting biologically distinct pathways and/or achieved better power (given the sample sizes) by using survival models, an alternative hypothesis is that our models were more prone to error and false positives.
In conclusion, although our findings were derived from a relatively small sample compared to those used for GWAS of other complex psychiatric diseases, we identified significant associations in genes never before implicated in SUD risk, one of which, in contrast to the top findings from published dependence diagnosis GWAS papers, showed evidence for association in both AA and EA ancestry groups. Although the effect size is small and the effect of the variant has little if any implication for the prediction or treatment of SUDs, it may point to a novel SUD-relevant pathway. Measuring gene and gene network expression changes after perturbing FAM78B in cell lines or mouse models would be a logical next step to determine if such a pathway exists. This work also highlights the value of analyzing related phenotypes for addictive disorders given the scarcity of risk genes identified through dependence diagnosis GWAS.
Abbreviations
AAs: | African Americans |
AASS: | alpha-aminoadipic semialdehyde synthase |
CACNA1B: | calcium voltage-gated channel subunit alpha1 B |
CATS: | the Comorbidity and Trauma Study |
CD: | cocaine dependence |
COGA: | the Collaborative Study on the Genetics of Alcoholism |
EAs: | European Americans |
FAM78B: | family with sequence similarity 78-member B |
FTO: | FTO alpha-ketoglutarate-dependent dioxygenase |
GWAS: | genome-wide association studies |
GWS: | genome-wide significant |
IBD: | identity-by-decent |
MAF: | minor allele frequency |
mQTL: | methylation QTLs |
OD: | opioid dependence |
OPRM1: | opioid receptor mu 1 gene |
PC: | principal components |
QC: | quality control |
SNP: | single nucleotide polymorphism |
SSADDA: | Semi-Structured Assessment for Drug Dependence and Alcoholism |
SSAGA: | Semi-Structured Assessment for the Genetics of Alcoholism |
SUD: | substance use disorder |
TD: | time-to-dependence |
Supplementary materials
The supplementary materials for this article are available at:
Declarations
Author contributions
JG, LAF, HRK, HJE, EN, NGM, and LD contributed conception and design of the study; CZ, EJ, and LW, and RS performed the statistical analysis; RS wrote the first draft of the manuscript; all authors contributed to manuscript revision, read and approved the submitted version.
Conflicts of interest
Dr. Kranzler is a member of an advisory board for Dicerna Pharmaceuticals and a member of the American Society of Clinical Psychopharmacology’s Alcohol Clinical Trials Initiative (ACTIVE Group), which over the past three years was supported by Alkermes, Amygdala Neurosciences, Arbor Pharmaceuticals, Ethypharm, Indivior, Lundbeck, Mitsubishi, and Otsuka. Drs. Kranzler and Gelernter are named as inventors on PCT patent application #15/878,640 entitled: “Genotype-guided dosing of opioid agonists”, filed January 24, 2018. In the past three years, Dr. Degenhardt has received untied educational grant funding from Indivior and Seqirus. No other authors reported potential conflicts of interest.
Ethical approval
This study was approved by the Institutional Review Boards of Boston University, Yale University and the University of Pennsylvania.
Consent to participate
Informed consent to participate in the study was obtained from all participants.
Consent to publication
Not applicable.
Availability of data and materials
Summary statistics for GWAS analyses will be provided by the corresponding author upon request.
Funding
This study was supported by National Institutes of Health grants RC2 DA028909, R01 DA12690, R01 DA12849, R01 DA18432, R01 AA11330, R01 AA017535, 2P50-AA012870, VA Connecticut Healthcare Center, Philadelphia VA MIRECCS, and National Center for Post Traumatic Stress Disorder. The funders had no role in study design, data collection and analysis, decision to publish, or preparation of the manuscript.
Copyright
© The Author(s) 2021.