Abstract
Fracture non-union remains a significant clinical challenge despite considerable advances in diagnostic imaging and treatment modalities. Unpredictable healing, repeated interventions, and prolonged disability contribute to high patient morbidity and increased healthcare costs. Early and reliable prediction of non-union is therefore essential for timely intervention. This review discusses traditional radiographic assessment using the Radiologic Union Scale for the Tibia (RUST), its inherent limitations, and the emerging role of artificial intelligence (AI) and deep learning in fracture analysis. In addition, we review recent studies—including Bayesian classifiers and simulation models—that integrate AI for early prediction of non-union, and we provide an updated summary table of key studies.
Keywords
Deep learning, machine learning, bone, fracture union, non-unionIntroduction
Non-union of bone after a fracture is an orthopedic condition with high morbidity and clinical burden [1]. Despite advances in the understanding of basic science and technology, fracture non-union remains a clinical problem [2]. Non-union is a difficult outcome to predict at the time of injury and throughout the healing process, and although it is an established complication of any fracture, in clinical practice, both clinicians and patients consider it a poor outcome [2]. Fracture non-union is a feared complication that leads to devastating consequences for the patient. Though rare, it requires complex and expensive treatment associated with multiple surgical procedures, prolonged hospital stays, pain, and functional and psychosocial disability. Implementation of treatment protocols can be complex and prolonged. Moreover, the resulting socioeconomic burden should not be underestimated. Being able to immediately identify patients at high risk for non-union will allow for early, appropriate, targeted therapeutic intervention, leading to a successful outcome [3].
The RUST and non-union
The Radiologic Union Scale for the Tibia (RUST) appears to be the most reliable method to routinely assess bone union [4]. The use of the radiologic union scale after non-union surgery may be useful in predicting non-union, and adjunctive therapies such as bone stimulation, autogenous grafting, or the use of bone morphogenic proteins may be considered in patients with possible non-union [5–7].
RUST is a scoring system based on the degree of bridging of the fracture callus and visibility of the fracture line on anteroposterior and lateral radiographs [7, 8]. Although the name of the RUST score varies in the literature, the scoring applied in the detection of union is the same [9–12]. And there is evidence in the literature that this scoring system is successful in predicting non-union. The main problems in the use of RUST are that it is observer dependent and inter-observer agreement is not perfect, it cannot predict the progression of healing in the early stages of union until the callus tissue starts to transform into lamellar bone and becomes visible on radiographs, and in patients with implants, not all cortices can be evaluated because the fracture line and bone cortices are covered by the implant on radiographs. According to RUST, since the union assessment is made by a human, the bridging of the union needs to be visible to the eye. Therefore, even if RUST predicts the possibility of non-union, a certain amount of time must pass over the fracture (the time it takes for union findings to be visible on radiography).
Software can be used to determine fracture union and callus tissue on plain radiographs [13]. The software can detect pixel changes caused by the fracture callus on plain radiographs, the area, volume, and width of the callus. In this way, it can provide quantitative monitoring of the progress in fracture healing [13]. With this feature, software has the ability to detect bridges during union and the progress in union at an earlier time. This can provide earlier radiological prediction of non-union after fracture, thanks to software.
AI, non-union, and literature
There are some studies and artificial intelligence (AI) applications in the literature regarding the use of AI in the detection of non-union [14–18]. These articles suggest that AI can be used to detect and/or predict non-union [14–18]. Stojadinovic et al. [15] evaluated an AI application that could be used to predict non-union by analyzing risk factors using Bayesian statistical methods. Degenhart et al. [18] designed a biomechanical model considering radiographs after intramedullary nailing in patients with femur fractures. And they predicted tissue differentiation in healing in these patients [18]. Although RUST criteria were used in this study, it was not primarily an application aimed at detecting non-union using radiological images. Also, predictions such as the complete tissue differentiation process in fracture healing and callus size were not confirmed in this study [18]. These studies were not primarily based on predicting non-union early using interval radiological images.
Fracture healing, AI, and the RUST score
Software can be used to determine fracture union and callus tissue on plain radiographs [13]. The software can detect pixel changes caused by the fracture callus on plain radiographs, the area, volume, and width of the callus. In this way, it can provide quantitative monitoring of the progress in fracture healing [13]. Deep learning algorithms are used in the field of orthopedics to detect or classify fractures from plain radiographs [19–21]. In addition to this application area, plain X-rays taken regularly and at certain weeks during the follow-up of fractures can be read into a designed deep learning model, and the information about the union or non-union of these fractures can be taught to this model. The success of this deep learning model in predicting non-union can then be tested. If the RUST criteria, in which visible callus bridging on plain roentgenograms is scored, predicts the likelihood of non-union, it may be possible that a deep learning model that can analyze even pixel changes caused by callus tissue can predict non-union in early fracture roentgenograms or predict non-union at higher rates. In addition, it can provide a more standardized union follow-up by eliminating observer-induced scoring errors. Finally, it can provide more reliable information by analyzing very small cortical areas and fracture lines not covered by the implant in patients with implants. This needs to be investigated and proven by scientific studies. A successful deep learning model in this regard may provide clinicians with advantages in terms of cost, time, and treatment options.
To our knowledge, there is no AI application that predicts non-union by analyzing fracture healing, considering only radiographic healing. RUST criteria can predict non-union. However, RUST criteria have this prediction when the bridging in fracture healing can be evaluated by seeing it with the human eye. If we assume that computer software can detect signs of fracture healing on X-rays earlier than the human eye can see, it may be possible to make this detection using AI applications earlier than the time we can detect non-union, according to RUST (Figure 1). Therefore, studies on AI applications in the detection of fracture non-union in serial roentgenograms are needed. Being able to achieve this may bring up cost-effective early surgical approaches for non-union.
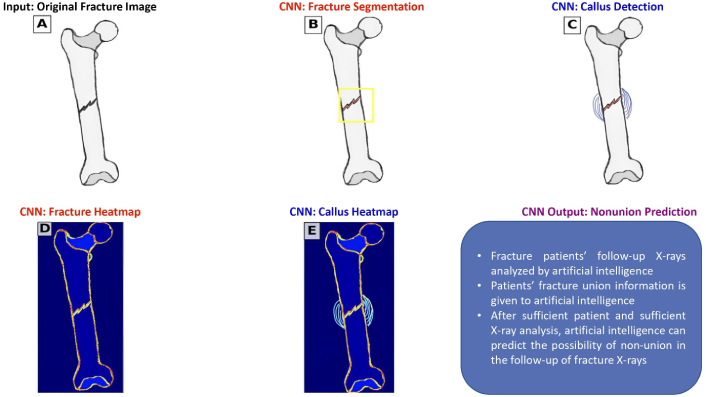
CNN and X-Ray processing. Anteroposterior and lateral X-rays of fracture patients are input into an artificial intelligence (AI) system (A). The AI system identifies and detects the fracture line and surrounding callus tissue (B and C). The AI analyzes changes in the fracture line and callus formation in sequential X-rays taken at regular intervals (D and E). The system is then provided with data on whether these fractures ultimately achieved union or non-union. By analyzing this comprehensive dataset, the AI can evaluate fracture healing progression and predict the likelihood of non-union during the early stages of fracture healing. CNN: convolutional neural network
Summary of key studies
To facilitate a clearer understanding of the current landscape, Table 1 summarizes several key studies that explore the application of deep learning in fracture detection and healing prediction.
Summary of key studies in deep learning for fracture analysis and non-union prediction
Reference | Study focus | Main finding | Performance parameters |
---|---|---|---|
Porter et al. (2016) [13] | Automated measurement of fracture callus using portable software | Quantitatively monitored callus progression, indicating potential for early non-union detection | Improved measurement consistency |
Kalmet et al. (2020) [19] | Deep learning for fracture detection | Demonstrated reliable fracture detection on radiographs | Enhanced sensitivity and specificity over traditional methods |
Chung et al. (2018) [20] | Automated detection and classification of proximal humerus fractures | Achieved high accuracy in detecting and classifying proximal humerus fractures | Accuracy metrics comparable to expert assessments |
Tanzi et al. (2020) [21] | X-ray bone fracture classification using deep learning | Established a baseline for fracture classification accuracy with potential for further improvement | Accuracy values in line with clinical expectations |
Stojadinovic et al. (2011) [15] | Prognostic naïve Bayesian classifier for non-union prediction | Developed a Bayesian model to predict fracture healing outcomes following shock wave therapy | Specific performance metrics are not detailed |
Degenhart et al. (2023) [18] | Computer-based mechanobiological fracture healing model for predicting non-union | Proposed a simulation model predicting healing outcomes after intramedullary nailing with promising results | Preliminary findings: detailed metrics require further validation |
Conclusions
Integrating deep learning into fracture healing assessment offers the potential to overcome many limitations of traditional methods. These technologies can enable earlier and more standardized predictions of non-union status, significantly impacting clinical decision-making processes, leading to improved patient outcomes and more efficient use of healthcare resources. This perspective aims to outline the current landscape and inspire further research toward integrating deep learning into daily orthopedic practice.
Abbreviations
RUST: | Radiologic Union Scale for the Tibia |
AI: | artificial intelligence |
Declarations
Author contributions
AY: Conceptualization, Investigation, Writing—original draft. HY: Conceptualization, Investigation, Writing—review & editing. AM: Validation, Writing—review & editing, Supervision. All authors read and approved the submitted version.
Conflicts of interest
The authors declare that they have no conflicts of interest.
Ethical approval
Not applicable.
Consent to participate
Not applicable.
Consent to publication
Not applicable.
Availability of data and materials
Not applicable.
Funding
Not applicable.
Copyright
© The Author(s) 2025.
Publisher’s note
Open Exploration maintains a neutral stance on jurisdictional claims in published institutional affiliations and maps. All opinions expressed in this article are the personal views of the author(s) and do not represent the stance of the editorial team or the publisher.