Abstract
As an integral part of human chronobiology, the circadian system plays a crucial role in regulating key biological functions, including sleep and the intricate hormonal rhythms of melatonin (MLT) and cortisol (CORT). Scholars have increasingly recognized environmental stressors as significant contributors to disturbed sleep patterns. Albeit vigorously discussed individually, the literature lacks comprehensive insights into the synergistic effect of artificial light at night (ALAN) and noise. The aim of this review is to look into the intricate interplay of the ALAN effects on sleep architecture, the modulation of circadian function, and how this influences homeostatic sleep. Furthermore, ALAN suppresses MLT secretion, which is most pronounced in response to short wavelengths of light. In addition, this review will demonstrate how exposure to noise during sleep elevates CORT and noradrenaline levels, which contributes to stress-related diseases and sleep disturbances. ALAN and noise, persistently emitted into the environment, share intrinsic mechanisms with comparable characteristics. Therefore, understanding their combined impact has become increasingly urgent. Pre-sleep exposure to both ALAN and noise acts as a potent stressor, with the potential to disrupt sleep patterns. Interestingly, during sleep, noise emerges as the predominant influence on sleep quality. Moreover, these stressors often synergize and amplify one another’s adverse effects. Thus, limiting their exposure is crucial for cultivating a sustainable environment conducive to quality sleep and overall well-being.
Keywords
Artificial light at night, light pollution, noise pollution, sleep, circadian rhythms, melatonin, cortisol, public healthIntroduction
At the dawn of time, there was neither light nor noise pollution [1–4], but a natural environment for Homo sapiens to evolve in [5, 6]. Under these conditions, humans gravitated towards diurnal activity when sunlight was available and sought rest under the gentle cloak of night [7]. Driven by the relentless forces of natural selection [8], humanity developed in perfect harmony with the slow cadence of life, seamlessly orchestrating the delicate balance between activity and sleep [9]. Thus, through evolution, light became the synchronizer of circadian rhythms [10–12], while sounds served both as a communication tool and a warning of potential danger, triggering survival instincts and biological responses such as increased levels of cortisol (CORT, stress hormone) [13, 14].
However, over the past three centuries, rapid technological advancements have disrupted this delicate balance [15, 16]. The “Industrial Revolution” accelerated urbanization, introduced shift work, and exposed people to increasingly intrusive artificial sounds from machinery [15], later from home appliances [16], and from rising traffic [17, 18]. Simultaneously, the discovery and widespread use of electricity led to pervasive artificial light at night (ALAN) [9, 19]. Both noise and ALAN pollution have since significantly disrupted human circadian rhythms and sleep patterns [20, 21].
Derived from the Latin word circā diēm (about a day), the circadian system is a self-sustained [22] bio-oscillator generating a daily cycle of approximately 24.18 h. This non-24-hour period can vary due to genetic differences [22, 23]. Aligning the human circadian rhythm to a 24-hour cycle is achievable through the entrainment of environmental synchronizers, including light, body temperature, social interactions, and behaviors such as metabolic intake and rest-activity patterns [24–26]. By providing consistent and predictable environmental cues, these synchronizers help properly stage circadian rhythms, supporting sleep-wake cycles and activity routines [22].
In humans, the circadian time-keeping system is governed by the suprachiasmatic nucleus (SCN), a bilateral structure located in the hypothalamus (HYP) [6, 24]. The SCN is synchronized in response to light input, which is recognized as the primary zeitgeber (the time-giver) [10–12]. Functioning as the “master clock” [8, 25, 26], the SCN controls a network of peripheral clocks present in mammalian tissues [26–28], predominantly in peripheral organs such as the liver, spleen, kidneys, heart, and lungs [29]. This regulation occurs through chemical and/or neuronal intermediates, e.g., glucocorticoid receptors, retinoic acid receptors, G-protein-coupled receptors, tyrosine kinase receptors, and Ca2+ channels [26, 27]. Additionally, body temperature and feeding are also recognized as dominant zeitgebers for peripheral clocks [26, 30, 31].
The circadian rhythms regulate several key functions, including behavioral patterns such as mood and sleep-wake cycles, as well as physiological processes, e.g., metabolic activities [22, 32–34]. They also govern intricate hormonal rhythms, i.e., melatonin (MLT) [33] and CORT [35], which serve as markers of the circadian day-night cycle [26]. MLT levels peak during the night, while CORT peaks at the beginning of the circadian cycle, preparing the body for wakefulness and activity [26, 31]. Therefore, shifts in the circadian cycle are often associated with changes in body temperature and the timing of MLT secretion relative to an endogenous circadian oscillation reference point [22].
As an integral part of human chronobiology [36], the circadian system plays a crucial role in regulating sleep [22, 37]. Normal sleep involves two distinct states: rapid eye movement (REM) and non-REM (NREM). NREM is intricately categorized into four stages, further classified as NREM stages 1 (N1) to N3, with N3 encompassing stages three and four (Table 1), which are commonly recognized as slow-wave sleep (SWS) [38]. Sleep typically starts with NREM, progressing through these stages before transitioning into REM sleep [38]. This cyclical progression between stages continues throughout the night [39], delineating what is often referred to as sleep architecture [40], as described in Table 1 [38–41].
State | Stage | % of total sleep1 | Body and brain activity | Mechanism and benefits | |
---|---|---|---|---|---|
NREM | 1 | N1 | 2–5 | Low-voltage, mixed-frequency brain waves | Transitions from wakefulness and low arousal threshold |
2 | N2 | 45–55 | Low-voltage, low-frequency brain activity with spindles; reduces muscle tension | Associated with memory consolidation and learning | |
3 | N3 | 3–8 | Increased high-voltage SWS activity | Transition to stage 4; HGH release [41] | |
4 | 10–15 | Increased high-voltage, SWS; some body movements | HGH release [41] | ||
REM | 20–25 | Low-voltage, mixed-frequency brain activity; muscle atonia; bursts of REM; irregular HR and breathing (including short breathing apnea) | Dreaming, mood regulation, and antidepressant effects are most often associated with REM sleep; memory consolidation in REM is still debated |
HGH: human growth hormone; HR: heart rate; NREM: non-rapid eye movement; REM: rapid eye movement; SWS: slow-wave sleep; 1 Estimated for healthy young adults [38]
Empirical evidence highlights that circadian dysfunction and impaired sleep can stem from numerous factors. These include substance use, which disrupts REM sleep [42–45], triggers nightmares, causes insomnia [46], and induces circadian rhythm shifts [37]. Mental health conditions also significantly impact sleep quality [47–49]. Additionally, circadian disruptions, insomnia, sleep breathing disorders, and hypersomnia [50] are linked to viral infections [51], cardio-respiratory disorders [51–55], such as COVID-19 [56], and degenerative diseases [57], i.e., Parkinson’s and Alzheimer’s [58, 59]. Behavioral factors like shift work [60], sleep deprivation [61], irregular sleep-wake patterns [62], and jet lag [63] can also cause “circadian misalignment” (the discrepancies between the circadian rhythm and circadian entrainment). Socioeconomic status [64], demographic factors [65, 66], and environmental stressors [67] may also play a role.
Scholars have increasingly recognized urban-related stressors as significant contributors to disturbed sleep patterns and circadian function. Notably, ALAN often termed light pollution [9, 19, 68, 69], and noise pollution [21, 70–73] have garnered attention. These factors have been linked to disturbances in the secretion of key hormones, i.e., MLT [74, 75] and CORT [35, 76], further underscoring their impact on health, sleep, and the circadian system as a whole [8, 25, 37, 77–79].
Albeit being vigorously discussed individually, the literature lacks comprehensive insights into the synergistic interplay between ALAN and noise, which are common attributes of real-life exposure in urban environments. This gap in knowledge limits understanding of their overall impact on public health, including crucial aspects such as sleep quality and well-being.
This review aims to deepen the scientific understanding of the effects of ALAN and noise on sleep, MLT, and health while elucidating their intricate interplay through three key steps: first, exploring the anatomical pathways supporting vision and hearing; second, examining the individual effects of each stressor on sleep, MLT, CORT and health; and finally, analyzing the combined impact of ALAN and noise on sleep.
Methodology
To deepen the understanding of the acute effects of uncontrolled exposure to ALAN and noise, this review synthesizes both theoretical and empirical findings. It also clarifies the characteristics of these exposures, as identified by scholars. Although it deviates from traditional systematic review methodology and structure, a similar approach was employed. Initially, a comprehensive survey of the literature was conducted using key terms and their combinations, including “circadian”, “sleep”, “ALAN”, “noise”, “health effect”, “eye”, “cones”, “rods”, “melanopsin”, “MLT”, “CORT” and “ear”. This survey encompassed papers published in Google Scholar from 1970 through 2024. Studies were then screened based on criteria such as publication date (favoring recent studies), relevance (human-based studies), peer review, and methodological robustness.
This resulted in the inclusion of 19 studies on ALAN, 19 studies on noise, and 7 studies on the combined effects of ALAN and noise on sleep. Figure 1 presents a breakdown of the studies by category and research type. The full list of studies, organized by category (ALAN, noise, and combined effect) and publication year, is provided in Table 2. This table includes details on methodologies, main results, and limitations. Most of the studies on ALAN and noise were conducted under controlled laboratory conditions, comprising 68.4% and 47.4%, respectively. These studies are recognized for their limitations, including small cohort sizes [80] and difficulties in reflecting real-life conditions [80, 81]. Field-based research accounts for only 21.1% of ALAN studies and 31.6% of noise studies, typically involving small cohorts and the use of expensive, complex, and intrusive gold-standard equipment (e.g., [82, 83]). Moreover, studies on the combined effect of ALAN and noise are scarce, accounting for only 15.6% of all reviewed studies, with only two studies conducted in real-life field conditions and only one study [54] introducing real-time measurements. This underscores the pressing need for large-cohort field research conducted in real-world settings.
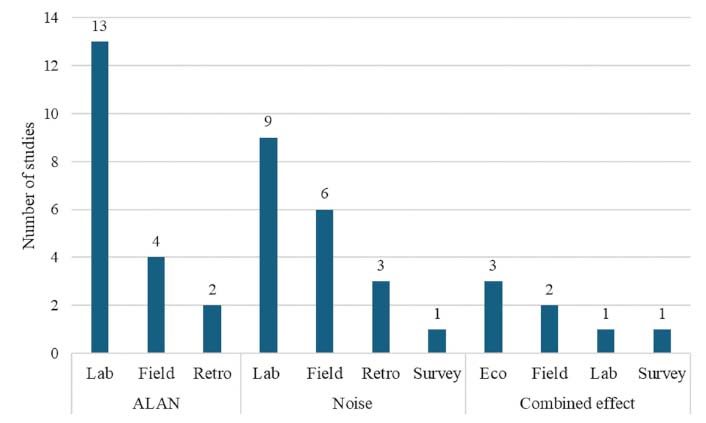
Classification of studies by category and research type. This classification excludes reviews, letters, and books, focusing solely on studies investigating the relationship between artificial light at night (ALAN) and noise with the circadian cycle, sleep, and melatonin (MLT). Additionally, the “laboratory” category encompasses research conducted in medical institutions, research facilities, and controlled clinical settings. Furthermore, the “ecological” category includes both individual-based and population-based epidemiological studies. The full list of studies is detailed in Table 2. Eco: ecological; Lab: laboratory; Retro: retrospective
List of reviewed studies, organized chronologically by publication year1
REF2 | Year | Study type3 | Cohort size and study design | Main results | Limitations |
---|---|---|---|---|---|
ALAN | |||||
| |||||
[111] | 2002 | Field | 154 postmenopausal women, with a mean age of 66.7 years, underwent one-week continuous recordings of light exposure and sleep using wrist actigraphy. Mood was evaluated using a questionnaire. | Pre-bedtime illumination (median 24 lux) had no significant impact on sleep. However, total 24-hour light exposure correlated with shorter sleep latency (P < 0.01), wakefulness within sleep (P < 0.05), and depressed mood (P < 0.01) | A homogeneous cohort in age and gender may influence light preferences and health. Actigraphy sensors may not fully capture light exposure, and ambient light recordings are limited. Spectral composition was also not considered |
[10] | 2013 | Lab | 10 healthy volunteers were exposed to two conditions over two nights: no light and 40 lux luminance, while PSG monitored sleep parameters. | Nights with lights-on were associated with increased N1 sleep, decreased SWS, and increased arousal. Moreover, brain activity was observed to decrease during NREM sleep under lights-on conditions. | Small cohort sizes and controlled conditions may not fully reflect real-world scenarios. The study did not account for spectral composition and relied solely on a single light intensity. |
[114] | 2013 | Lab | 30 individuals were exposed to light sources with temperatures of 6,500 K, 2,500 K, and 3,000 K at intensities ranging from 27 to 29.2 lux. The study protocol included dark conditions followed by 2 h of exposure sleep metrics were monitored using a PSG device. | All-night NREM EEG power density showed lower frontal power with 6,500 K light compared to 2,500 K (0.8%) and 3,000 K (0.2%). Additionally, SWS activity decreased by 0.4% and 0.3%, and total sleep time was shorter by 4 min and 12.7 min with 6,500 K light compared to 2,500 K and 3,000 K, respectively. | A small cohort size and controlled conditions may not accurately reflect real-world scenarios. Additionally, the study did not account for changes in light intensity. |
[110] | 2017 | Field | 20 healthy volunteers were monitored for three weeks using PSG and actigraphy to collect sleep metrics. Their light exposure was monitored using wrist actigraphy. | Later circadian phases were linked to lower light intensity (P < 0.01), delayed light exposure (P < 0.001), and later sleep timing (P < 0.001). Light exposure above 10 lux increased awakenings (P < 0.05), while delayed exposure reduced REM latency (P < 0.05). Lower light levels were associated with more REM sleep (P < 0.001), and higher light intensity with early exposure led to greater SWS accumulation (P < 0.05). | Limitations include a small cohort, potential obstruction of actigraphy light sensors, and reliance on ambient light measurements that may not accurately reflect perceived light. Additionally, the study did not account for the light’s spectral composition. |
[115] | 2017 | Lab | 19 volunteers (mean age 24.3 years) were exposed to light from computer screens with varying intensities (80 lux and 350 lux) and wavelengths (460 nm and 650 nm). Sleep metrics were collected with PSG, MLT levels from urine, and body temperature were recorded at set times. Behavioral evaluation was performed using questionnaires. | SWL exposure was linked to shorter sleep duration (8.6 min to 16.4 min), increased WASO by 2.1% to 3.1% (P < 0.01), decreased SE by 2.1% to 3.1% (P < 0.01), and reduced SWS by 6.5% to 7.7% (P < 0.05). On SWL exposure nights, body temperature was higher, MLT levels were lower (P < 0.05), and subjective sleep quality, mood, and attention were worse, with attention impairment significant (P < 0.05). | The 22-inch LED screens used may not represent other technologies or sizes. Measurements were limited to one night per light condition, restricting long-term effects. Daytime exposure, which influences MEL levels and circadian function, was not recorded. The small cohort and controlled setting may not reflect real-world scenarios. |
[112] | 2023 | Field | 59 volunteers wore wrist actigraphy to measure light exposure and collect sleep metrics over seven days, from February to July 2022. Personal attributes were collected using questionnaires and sleep diaries | Earlier bedtimes were linked to afternoon light exposure (13:00–17:00). Longer bedtimes corresponded to extended sleep on high light exposure (> 1 lux) days, while more natural light was associated with earlier sleep onset. ALAN exposure above 10 lux before sleep was tied to earlier sleep onset, peaking in the last 30 min before bedtime. Each 1 log lux increase in light exposure extended sleep onset by 0.5 h (P < 0.05). | The five-month data collection period may introduce seasonal bias. Questionnaires are prone to omission and recall biases. Actigraphy light sensors could be obstructed, recording ambient rather than perceived light. Additionally, the spectral composition was not considered |
| |||||
[119] | 1980 | Lab | 6 volunteers underwent a two-night protocol: 500 lux on the first night and 2,500 lux on the second. Two volunteers continued for two additional nights, with 1,500 lux on the third night and darkness on the fourth. Light intensities were measured at eye level, and MLT synthesis was assessed via blood samples. | MLT levels decreased (P < 0.05) within 10 min to 20 min of exposure to 2,500 lux incandescent light, nearing daytime levels within 1 h (P < 0.01). MLT levels quickly rebounded to pre-exposure levels within 40 min after returning to darkness. Exposure to 500 lux did not affect MLT levels significantly. Subjects exposed to 1,500 lux light showed intermediate MLT levels, with recovery similar to that after exposure to 2,500 lux. | The small cohort size and controlled conditions may not accurately reflect real-world scenarios. Additionally, the study did not account for the spectral composition or personal confounders of the volunteers. |
[124] | 1995 | Lab | 11 blind patients with no conscious perception of light and 6 healthy men aged 20 years to 25 years were exposed to 10 lux to 15 lux during baseline and 6,000 lux to 13,700 lux during stimulus exposures. Body temperature and blood samples for plasma MLT evaluation were collected at fixed intervals | All healthy individuals showed MLT suppression in response to bright light, with levels averaging 66% lower during the last 60 min of exposure compared to 24 h earlier. Blind patients who did not experience sleep difficulties, also showed MLT suppression, with a 69% decrease following bright light exposure. These blind patients maintained stable entrainment to a 24-hour day in repeated measurements | A small cohort size and controlled conditions, which may not fully represent real-world scenarios. |
[120] | 2001 | Lab | 15 volunteers (mean age 31.8 years) were exposed to light spectra (470 nm to 660 nm) aimed at the pupil. Over a 6-week protocol, they experienced various spectral conditions. Saliva MLT was sampled at fixed intervals. | The shorter wavelengths (470 nm, 497 nm, and 525 nm) caused significant MLT suppression, ranging from 65% to 81% (P < 0.0001). They also resulted in the greatest delay in simulated light MLT onset on the second night, ranging from 27 min to 36 min. | A small cohort size and controlled conditions may not accurately represent real-world scenarios. |
[12] | 2005 | Lab | 48 individuals, with a mean age of 23 years, were exposed to light intensities ranging from < 0.03 lux to 9,500 lux over three consecutive days. During the study, body core temperature and blood plasma MLT levels were monitored at regular intervals. | Significant shifts in MLT peak midpoint were seen at all light intensities compared to < 0.03 lux (P < 0.05). Suppression was minimal below 15 lux but complete above 200 lux. The circadian system responds logarithmically, with half-maximal effects at 50–160 lux and saturation at 1,400 lux. MLT suppression reached half-max effects at 10–200 lux and saturation at 1,100 lux. | Background light could significantly influence the responsiveness of the circadian timing system. Additionally, the study did not account for spectral composition. Lastly, the study was conducted under controlled conditions, which may not accurately reflect real-world scenarios. |
[125] | 2007 | Lab | 2 blind subjects participated in parallel experiments: a 56-year-old male and an 87-year-old female, both without light perception. In Experiment #1, the male underwent a 14-day inpatient study with random exposure to 555 nm and 460 nm light for 6.5 h, with plasma MLT measured via blood samples and sleep assessed by EEG. In Experiment #2, the female’s pupil constriction response to various monochromatic light wavelengths and irradiances was tested for 10 s. | In Experiment #1, 555 nm light had no effect on plasma MLT, while 460 nm light reduced it by 57% and caused a 1.2-hour phase delay in the circadian MLT rhythm, compared to a 0.4-hour delay with 555 nm light. Blue light increased alpha EEG activity and reduced subjective sleepiness, consistent with its effects in sighted individuals.In Experiment #2, irradiance-response curves matched vitamin A opsin-pigment nomograms (P < 0.01) for all three cones, indicating pupil constriction was driven by a photopigment with a peak at 476 nm. This suggests that ipRGCs mediate both pupillary and circadian responses to light, even in the absence of rods and cones. | A small cohort size and controlled conditions may not fully represent real-world scenarios. Additionally, the male subject received a pupil dilator before each experimental light exposure. While this approach is acceptable in experimental settings, it may not fully replicate real-world conditions of light exposure. |
[129] | 2011 | Retro | 116 healthy volunteers aged 18 to 30 participated in two inpatient studies. In Study #1, 104 volunteers followed a 9–10 day protocol involving enforced wakefulness for 30–50 h. In Study #2, 12 volunteers were exposed to 200 lux daily for 14 days, followed by a 40-hour constant routine under the same light intensity. Blood samples were collected at intervals to monitor plasma MLT concentrations. | In Study #1, MLT onset occurred 23 min before sleep under room light conditions (< 200 lux) but 1 h and 57 min before bedtime under dim light (P < 0.05), marking a 94-minute earlier onset. Room light reduced MLT secretion duration by 1 h and 32 min compared to dim light (P < 0.05). Additionally, 78.6% of volunteers had earlier MLT onset by over an hour, and MLT concentrations were reduced by 71.4% on average under room light. In Study #2, four of five individuals showed significant MLT suppression with room light exposure, while seven of eight experienced greater suppression with higher light intensities (~200 lux). | Study conditions, specifically dim and bright light exposures, were not balanced, which could affect the accuracy of comparisons. Additionally, the study did not account for spectral composition. Lastly, the studies were conducted under controlled conditions that may not fully reflect real-life scenarios. |
[122] | 2013 | Lab | 9 volunteers (mean age 26.3 years) were exposed to six light conditions, varying in intensity from < 10 lux to 500 lux and temperature from 2,000 K to 6,000 K, over six days. MLT concentration was measured from saliva, and alertness was assessed via questionnaires. | Comparisons showed significant decreases in MLT levels with three of the four lamps containing blue components (P < 0.03). The 500 lux, 6,000 K condition did not significantly affect MLT levels (P = 0.07), and the 130 lux, 2,000 K condition had no effect. Subjective alertness significantly increased after exposure to the 130 lux, 6,000 K; 500 lux, 6,000 K; and 500 lux, 5,000 K conditions (P < 0.05). | The study’s small cohort size and controlled conditions may not fully represent real-world scenarios. Additionally, as suggested by the authors, it lacks outcome variables such as sleep parameters and objective measures of behavioral variables. |
[126] | 2018 | Lab | 18 healthy blind subjects were exposed to 6,807 lux from a 4,100 K source. The study included 5–6 days of inpatient observation followed by a 38-day protocol where 10 volunteers adjusted to a 28-hour “day” which included light stimuli. Saliva and blood samples were collected to measure MLT concentration, and body core temperature was recorded at set intervals. | During 6.5 h of light exposure, MLT concentrations decreased by an average of 71.6% in five blind participants, with each showing reductions over 33%. Four had a normally phased 24-hour rhythm in urinary MLT before the study, while the fifth, with an irregular rhythm and opaque scleral shells, showed a positive response after removing the shells. Among those without MLT suppression, only one had a 24-hour urinary rhythm prior to the study, likely due to non-photic cue entrainment. | A small cohort size and controlled conditions, which may not fully represent real-world scenarios. |
[121] | 2019 | Retro | To model the relationship between alpha-opic illuminances and MLT suppression, early study results were reanalyzed and validated. | The study found the highest R² value (0.87) with melanopic lux and melanopsin-weighted irradiances, indicating that MLT suppression is strongly predicted by melanopsin-weighted illuminance. MLT suppression was found to be most sensitive at 480 nm SWS light and is enhanced by blue-enriched light stimuli. Moreover, the model demonstrated good predictive performance. | The model is based on a single dataset capturing a narrow circadian phase, limiting its applicability to other phases and conditions. Moreover, its reliance on controlled settings may not reflect real-world scenarios. |
[123] | 2022 | Lab | 100 healthy volunteers (aged 18 to 30) completed a nine-day protocol involving 30- and 50-hour constant routines and exposure to various light spectra and intensities. Saliva and blood samples were collected to assess MLT secretion. | MLT suppression and circadian phase resetting were best modeled with peak sensitivities at 481 nm and 483 nm, respectively. Initially, MLT suppression peaked at 441 nm and 550 nm, indicating significant early contributions from S and L+M cones. Over time, the peak shifted to 485 nm, reflecting a predominant influence of melanopsin. Similarly, circadian phase resetting showed peak sensitivities from 445 nm to 487 nm, with a shift from S-cones to melanopsin as exposure continued. | The study was conducted under controlled conditions, and participants used a pupil dilator, which may not reflect real-life scenarios. Additionally, it was not documented whether maximum pupil dilation was maintained throughout the 6.5-hour light exposure, potentially affecting the results. |
[127] | 2022 | Field | 580 participants (mean age 71) monitored short-wavelength ALAN exposure and sleep metrics over two days using a headband for light, actigraphy for sleep data, and a PSQI questionnaire for subjective evaluation. Daily urine samples assessed MLT levels. The study was conducted from November 2012 to March 2014. | Increased short-wavelength ALAN was significantly linked to higher global PSQI scores and sleep disturbances (P = 0.004 and P = 0.006). Multivariable analysis showed a higher OR for disturbances in the highest tertile compared to the lowest (P = 0.006). MLT levels were significantly lower in the highest tertile (P = 0.039). Objective sleep measures revealed longer sleep onset latency (P < 0.001), increased WASO (P < 0.05), and decreased SE (P < 0.001) in the highest tertile. | The study’s extended duration raises concerns about seasonal effects. Despite a large cohort, each volunteer was observed for only two days, limiting individual data. Inconsistent MLT and sleep measurements, a cross-sectional design, and reliance on a homogeneous elderly population limit generalizability and introduce potential bias. |
| |||||
[132] | 2007 | Lab | 10 volunteers (mean age 21.9) were asked to wear wrist actigraphy for one week to measure light exposure and to sleep in the lab during a night session with 2 h of exposure to 1,000 lux bright light. Saliva samples were collected for MLT measurement, and subjective sleep data were obtained via questionnaires. The study, conducted in winter and summer, to assess seasonal effects | Awakening occurred about 30 min earlier in winter (P < 0.05), with higher MLT amplitude. MLT concentration decreased with light exposure, but the suppression percentage was significantly greater in winter (66.6%) compared to summer (37.2%) (P < 0.01). Winter ambient light levels were about half those of summer (P < 0.01). There was considerable variability in MLT levels, light exposure, and suppression among individuals. Other sleep and MLT metrics showed no significant seasonal differences. | The small cohort size and controlled conditions may not accurately reflect real-world scenarios. Additionally, using a wrist-worn device to measure light could be inaccurate if the light sensor becomes obstructed. Moreover, the study did not account for variations in light spectra, which could differ between ALAN sources and sunlight across different seasons. |
[157] | 2019 | Lab | 11 healthy volunteers (mean age 23 for the control group, 27 for the exposure group) underwent a 4-day simulated night shift protocol. After a 24-hour baseline, participants followed a 10-hour delayed sleep schedule. The control group was exposed to dim light (< 10 lux), while the exposure group received 6,500 lux of bright light. Blood samples were for MLT measurement and microarray analysis. | In a study of 492 probe sets with a 24-hour rhythm, 67% showed altered circadian expression under bright light during a simulated night shift, with gene rhythms delayed by 7.8 h on average. The bright light group had a significantly greater delay in the midpoint of MLT secretion (7.6 h) compared to controls (0.4 h, P = 0.014). Gene analysis indicated bright light's strong phase-shifting effect, affecting genes related to immune processes, lipid metabolism, and circadian rhythm regulation. | The study is limited by a small cohort size, a narrow age range (18–30 years), and a skewed sex distribution (10 males vs. 1 female). Furthermore, the controlled conditions employed may not fully replicate real-world scenarios. |
Noise | |||||
| |||||
[193] | 1983 | Field | 26 healthy individuals, who had lived near a busy street slept under various conditions, including changes in bedroom location, earplugs, and double-glazed windows, to simulate both quiet and typical nighttime environments. Their sleep behaviors were monitored using EEG signals, while sound was recorded with microphones and tape recorders. | Noise peaks of 50.3 dB(A) can cause awakenings, while changes in sleep state occur at 48.5 dB(A), and transient reactions at 47.6 dB(A). The study suggests that limiting noise peaks to below 40 dB(A) can prevent 80% of sleep disturbances and 87% of awakenings while keeping noise below 45 dB(A) can avoid two-thirds of sleep impairments. Additionally, peak noises during quiet conditions caused shifts in sleep at lower intensities [42–44 dB(A)] compared to noisier conditions [50–53 dB(A)]. | Changes in sleep settings affected room temperature and other environmental factors, which may have influenced sleep. This complicates the observed relationship between noise and sleep, as other interactions could have played a role. Additionally, the study’s small sample size limits the generalizability of its findings. |
[194] | 1987 | Lab | The study included a pilot with one participant exposed to noise levels from 40 dB(A) to 70 dB(A) in 5 dB(A) increments, and a main study with 9 individuals exposed to: (a) a single truck pass, (b) continuous all-night traffic noise, and (c) a combination of both. Sleep metrics were recorded using PSG, and mood was assessed via questionnaires. | Awakening reactions in response to car passages were observed at 55 dB(A) (P < 0.05). An increase in noise level from 45 dB(A) to 55 dB(A) significantly affected sleep stages (P < 0.01). Additionally, noise peaks relative to background levels were better predictors of arousal and changes in sleep stages than absolute noise peak levels. Subjective mood and sleep quality questionnaires supported these findings. | The study’s small cohort size and controlled conditions may not fully reflect real-world scenarios. |
[195] | 1990 | Lab | 18 individuals (mean age 20.8) were divided into three groups. The first and second groups were exposed to infrasound and low-frequency sounds (10–63 Hz) at 50–105 dB(A). The third group was subjected to simulated traffic noise (25–1,600 Hz) at 40 dB(A), 50 dB(A), and 60 dB(A) for 30 s every 20 min during sleep. Sleep patterns were monitored using EEG. | The reaction rate during N1 was significantly higher than in other sleep stages, with REM and N2 being more affected than N3 (P < 0.01), indicating that lighter sleep stages are more sensitive to infrasound and low-frequency sounds. However, in groups one and two, the relationship between infrasound, low-frequency sounds, and sleep metrics was insignificant compared to control nights. In group three, exposure to 40 dB(A), 50 dB(A), and 60 dB(A) led to awakening rates of 33%, 50%, and 100%, respectively, with 40 dB(A) also causing changes in sleep stages in 51% of cases. | The study’s small cohort and controlled conditions may not reflect real-world scenarios. It also did not account for indoor noise from appliances, which could impact results. Additionally, variability in noise perception among the three groups may introduce bias and affect the findings. |
[191] | 2001 | Retro | This study is based on the results of road traffic noise and urine catecholamine levels during sleep. Overnight urine samples were analyzed for adrenaline and NA. Noise exposure was assessed through traffic counts, and indoor exposure was evaluated through questionnaires. | A significant increase in renal adrenaline was associated with a higher number of cars per day (P < 0.05). Additionally, subjective perceptions of indoor noise by volunteers were strongly correlated with urinary NA secretion (P < 0.001). Renal adrenaline levels were 26% higher in women experiencing high levels of disturbance compared to those with low disturbance (P < 0.05). | The study lacked data on daytime noise exposure, making it difficult to assess cumulative effects on adrenaline and NA secretion. Additionally, noise exposure was estimated from traffic counts rather than direct measurements, potentially misrepresenting actual noise conditions. |
[192] | 2002 | Field | 16 volunteers were exposed to airport noise for 40 nights, peaking at 65 dB(A). The noise occurred from 23:00 to 06:00, with an average continuous level of 42 dB(A). Urine samples were collected nightly to measure CORT, adrenaline, and NA, and subjective data were gathered via a questionnaire. | Nocturnal noise exposure significantly increased CORT levels in men but not in women, suggesting greater men sensitivity to noise (P = 0.038). Variability in adaptation types was observed, with changes in CORT excretion patterns and significant associations between adaptation differences and CORT variations (P = 0.004). | The study is limited by its small sample size and its exclusive focus on airplane noise, which restricts the generalizability of the findings to other common noise sources, such as road traffic and indoor appliances. |
[206] | 2003 | Lab | 10 volunteers (mean age 24.7) were exposed to 8 h of 75 dB(A) mill noise. Sleep was monitored with PSG and questionnaires, and blood samples were collected to measure CORT levels. | On the exposure night, both REM sleep and total sleep durations were significantly reduced compared to quiet conditions (P < 0.001 and P < 0.05, respectively). REM sleep onset was delayed (P < 0.01), and there were more frequent stage shifts (P < 0.001). SE fell below 80%, and CORT levels were elevated during the exposure night. | The controlled conditions and small sample size limit the study’s applicability and generalizability to real-world settings. Additionally, the brief exposure duration restricts the assessment of continuous effects. |
[209] | 2006 | Field | 360 individuals, equally divided between children (mean age 10.9 years) and parents (mean age 42 years), provided sleep habit information through questionnaires and interviews. Noise exposure was categorized by road traffic levels [< 55 dB(A), 55–59 dB(A), 60–64 dB(A), and > 64 dB(A)]. | For parents, road traffic noise significantly (P < 0.05) affected sleep quality, awakenings, and perceived interference. Children also showed a notable link between noise and sleep quality, as well as daytime sleepiness. Despite reporting better sleep quality and fewer awakenings, children’s wrist-actigraphy showed that parents had better objective sleep (P < 0.001). Both groups experienced acute sleep disturbances at 60 dB(A) to 64 dB(A) noise levels. | Actigraphy, which tracks body movements, may misclassify children’s sleep due to their higher sleep activity compared to adults. The study’s noise assessment, categorized by road traffic noise, might not accurately reflect direct exposure. Additionally, sleep questionnaires are prone to recall and response biases. |
[72] | 2011 | Lab | 12 volunteers with a mean age of 27 years underwent a 3-day lab exposure protocol, with the first night serving as an adaptation period. On the second and third nights, noise stimuli were presented every 30 s during sleep, starting at 40 dB(A) and increasing in 5 dB(A) increments until arousal, a change in sleep stage, or a maximum of 70 dB(A). PSG was used to collect sleep metrics. | Increased sound levels significantly disturbed sleep, with effects varying by sound type. Arousal probabilities differed among sleep stages: N2 was different from N3 and REM (P < 0.001), while N3 and REM were similar. Arousal rates were lower during N3 compared to N2, and REM had a more consistent arousal response to different sounds. Sound-induced arousals significantly increased HR, with the highest responses during REM, followed by N3 and N2. Additionally, HR response times from arousal onset to peak were shorter during REM sleep. | The small cohort, narrow age range, and controlled conditions limit the study’s generalizability. Real-world noise exposure is more variable and interactive, which may amplify physiological and psychological effects differently than the fixed noise administration used in the study. |
[196] | 2011 | Field | 40 healthy volunteers, with a mean age of 48.5 years, participated in the study. Half of the cohort was exposed to road traffic noise, while the other half was exposed to rail noise. Data were collected over two consecutive nights, including sound recordings and PSG to measure sleep metrics. | Road traffic noise did not affect sleep, but rail noise exposure was significantly linked to increased WASO and reduced REM sleep (P < 0.01). A significant difference was found in arousals and REM sleep between rail noise levels below and above 50 dB(A) (P = 0.02). Specifically, exposure to rail noise above 50 dB(A) resulted in shorter REM sleep, while exposure below 50 dB(A) led to more arousal. | The study did not control for non-traffic noises, and unattended data recording prevented sensitivity adjustments. Personal confounders and individual noise sensitivity were not analyzed. The small sample size and brief duration may not fully capture noise effects or variability, limiting generalizability and may introduce bias. |
[198] | 2012 | Retro | Data from 7,019 participants (mean age 50.5) in the Finnish Public Sector Study Cohort (2004–2009) were analyzed for sleep duration and insomnia. Nighttime traffic noise levels were calculated, and personal confounders and socioeconomic attributes were assessed via questionnaires | Participants exposed to noise levels > 55 dB(A) had significantly higher OR for insomnia symptoms and nonrestorative sleep compared to those exposed to ≤ 45 dB(A). Frequent nighttime awakenings were notably linked to higher anxiety scores at > 55 dB(A). The impact of noise exposure > 55 dB(A) on insomnia was more pronounced among women and obese individuals compared to men and those who were not obese. | The study’s generalizability is limited by its homogeneous population and incomplete traffic data. Key limitations include exposure misclassification, missing nighttime traffic intensity data, and potential bias from variations in noise levels and survey timing. |
[201] | 2014 | Lab | 103 patients, with a mean age of 60 years, across 29 ward rooms, completed the PSQI and the Leeds Sleep Evaluation Questionnaire to evaluate their sleep performance. Noise exposure during one night of sleep was measured using type-1 noise level dosimeters. | The study found significant declines in sleep quality among patients. Overall, 86% reported disturbed sleep, linked to daily average noise levels of 63.5 dB(A) (P < 0.05). Female patients had higher PSQI scores (P = 0.06), and those with severe disease had poorer sleep (P = 0.04). Patients not on sleep-interfering medications fared better (P = 0.08). Noise levels above 49.3 dB(A) during the day and 34.2 dB(A) at night were associated with increased disturbances, with common sources being caregivers, visitors, other patients, toilet flushing, and medical devices. | The small cohort limits statistical power and generalizability. Sleep assessments via questionnaires may suffer from omissions and recall bias. Dosimeters did not capture detailed noise characteristics, and psychological factors like stress and depression, which are common among patients, were not considered. |
[210] | 2015 | Retro | The study, based on a 2007 environmental and health survey in Sweden, included 2,612 participants (median age 46 years) living near rail and noisy roads. Noise exposure was categorized into six levels: < 40 dB(A), 40–44 dB(A), 45–49 dB(A), 50–54 dB(A), 55–59 dB(A), and ≥ 60 dB(A). The survey assessed participants’ annoyance, sleep quality, bedroom window orientation, and subjective noise perception. | Access to a quiet or green space decreased with higher noise levels. A bedroom window facing green space significantly reduced noise annoyance (P < 0.05) and concentration problems. Higher noise exposure was linked to poorer sleep quality. Men reported fewer sleep issues compared to women, while those under financial strain experienced more sleep problems. | Limitations include reliance on potentially biased self-reported data, unconsidered factors like OSA, and unvalidated scales. With a 54% response rate, there may be selection bias, especially in high-noise areas. Noise assessments lacked details on building characteristics and other sources, and combining noise types could skew results. Assigning noise exposure by building rather than individual units may also introduce errors. |
[203] | 2018 | Lab | 64 patients, averaging 63.9 years, completed RCSQ. Noise exposure was recorded for one night using a microphone and laptop. | A daily average sound pressure of 54 dB(A) was associated with poorer sleep (P < 0.05). Additionally, women reported better sleep quality than men (P < 0.01). | Small cohort limits generalizability. Sleep questionnaires may introduce recall and response biases, especially in critically ill patients. A single night’s measurement does not capture long-term effects. |
[207] | 2018 | Lab | 48 day-shift workers (mean age 45.1) were split into two groups: one experienced high noise followed by low noise, and the other the reverse. CORT levels were measured from blood samples, and sleep was recorded using PSG. | Sleeping after exposure to 76.8 dB(A) was linked to longer sleep durations but decreased SWS and SE (P < 0.05). Additionally, blood pressure, sympathetic activity, and CORT levels significantly increased following noise exposure (P < 0.05). | Controlled conditions and a small sample size limit generalizability and may increase Type II errors. Protocol deviations, unmonitored lab noise, and non-representative noise composition may also impact results. |
[208] | 2019 | Field | From May to November 2016, 105 volunteers from 94 households (mean age 52.1 years) wore wrist actimeters for seven consecutive days to monitor sleep. Environmental and room acoustics were assessed through outdoor and sound measurements. | Average noise exposure was not a significant predictor of sleep quality. However, noise during the first 4 h of bedtime reduced sleep latency by 5.6 min per 10 dB(A) increment. Conversely, noise exposure during the final 3 h before waking decreased SE by 2% to 3% per 10 dB(A) increment. | Indoor noise was estimated from outdoor measurements, potentially misrepresenting conditions. Censoring noise levels below 20 dB(A) and missing nightly data on window positions contributed to exposure misclassification, reducing statistical power. |
[220] | 2020 | Lab | 50 volunteers (mean age 51.2 years), including 24 living near a wind turbine, participated in a 3-night study: habituation, wind turbine noise exposure, and quiet control. Sleep was assessed with PSG, CORT levels were measured from saliva samples, and subjective experiences were evaluated with morning questionnaires. | During exposure nights, REM duration increased by 16.8 min, while the amount of REM sleep decreased by 11.1 min (−2.2%). Other objective sleep measures did not differ significantly between nights. Self-reported sleep quality was consistently worse on exposure nights, and individuals living near wind turbines reported poorer overall sleep compared to the reference group. | The study faced potential self-selection bias, as the exposed group included individuals who had complained about wind turbines. The small sample size, deviations from requirements, and laboratory setting may not reflect real-life conditions, introducing bias. Awareness of experimental conditions could also influence self-reported outcomes. |
[211] | 2021 | Survey | A total of 495 participants completed a questionnaire covering sleep performance, occupational strain, and demographic and lifestyle factors. Nocturnal noise exposure was modeled using road traffic data based on the Nordic prediction method. | Nocturnal traffic noise was negatively associated with self-reported poor sleep, while poor sleep was more common in medium- and high-traffic areas. A quiet bedroom façade reduced the incidence of poor sleep, especially at 45–50 dB(A). No difference in poor sleep prevalence was found between windows facing no street and those facing a low-traffic street. | The study relied on self-reported sleep data, which may be biased by misclassification and recall issues. It did not directly link disturbances to road noise, affecting accuracy. A restrictive sleep cut-off and lack of OSA screening could have influenced results. Incomplete data on bedroom orientation and noise exposure, with traffic noise as a proxy, may have biased estimates, and missing details on building specifics and other noise sources could have affected findings. |
[197] | 2022 | Field | 40 participants, mean age of 29.1 years, were monitored with PSG over five consecutive nights. Indoor sound levels were recorded using a type-1 sound level meter, while road traffic counts were obtained via a radar detector and analyzed into sound levels using custom-developed acoustic software. | Traffic noise events were positively associated with REM sleep (P < 0.001) and increased probability of awakening due to maximum noise pressure (P = 0.03) and age (P < 0.005). Conversely, a negative association was observed with SWS (P = 0.017) and the interaction between REM and age (P = 0.017). | The small sample size limits generalizability. Study locations with high noise levels may not represent other urban areas. Noise exposure was assessed from traffic counts rather than direct measurements, and the study did not consider confounders such as occupation, income, or temperature. |
[204] | 2022 | Lab | 148 patients in intensive care units, scheduled for surgery, with a mean age of 63 years, completed the RCSQ on their first night of admission. Noise levels were monitored over six weeks using a type-2 sound meter. | The study found no overall correlation between nighttime sound levels and sleep quality. However, patients with a Visual Analog Scale (VAS) score > 3 or those on hypnotic drugs had lower RCSQ scores (P < 0.05 and P < 0.01, respectively). Excluding these patients showed that each 1 dB(A) increase in nighttime noise was associated with a 3.92-point drop in RCSQ scores (P < 0.05). | The study did not account for pre-existing sleep disorders, age, obesity, or disease severity. The sound meter mostly captured staff noise rather than patient-specific sources. Assessments were based on the first night of admission, missing long-term effects, daytime sleep, and circadian disruptions, and lacked patient feedback on perceived sleep disruption causes. |
| |||||
[83] | 1999 | Field | In a 4-year study of 184 residents (mean age 83.9 years) from eight nursing homes, participants were divided into an intervention group and a control group. The intervention aimed to reduce noise and ALAN exposure. Light and noise levels were recorded with a light meter and microphone, while sleep metrics were collected via actigraphy. | While their behavioral intervention resulted in significant reductions in ALAN and noise exposure compared to the control group (P < 0.01), no significant changes were found in sleep patterns. | Noise intensity remained high post-intervention, with peaks over 50 dB(A), possibly impacting sleep and reducing effectiveness. The study also overlooked daytime napping, personal factors like medical conditions, and recorded ambient rather than direct exposure, potentially introducing bias. |
[223] | 2016 | Eco | 19,136 individuals aged 18 to 99 years completed questionnaires, including self-assessed noise levels. ALAN data were obtained from the Defense Meteorological Satellite Program using NASA’s VIIRS instruments. | Increased exposure to outdoor ALAN correlated with delayed bedtimes, later wake-up times, shorter sleep durations, and increased daytime sleepiness (P < 0.01). Intriguingly, noise levels showed no statistically significant correlation with sleep metrics. | Self-reported data may suffer from recall and misclassification biases. Relying on self-reported noise and average ALAN measurements could introduce exposure bias. The study also did not analyze the combined impact of ALAN and noise. |
[70] | 2021 | Eco | 7,230 individuals with a mean age of 34.5 years completed a national survey focusing on health and well-being, including sleep patterns. Outdoor ALAN exposure was assessed using NASA’s VIIRS data, while noise exposure was interpolated from road density maps. | Increased road density and ALAN intensity were associated with reductions in sleep duration (4.5% and 3%, respectively) and increases in sleep difficulty (3.5% and 11%, respectively) (P < 0.01). An interaction effect showed that higher ALAN in noisy areas led to longer sleep but greater sleep difficulty (P < 0.01), possibly due to ALAN penetrating sleeping areas where residents close shades due to heat and humidity. | This ecological study combines individual data with environmental estimates, risking misspecification and uncontrolled confounding, which prevents the establishment of causality. Additionally, relying on outdoor ALAN and noise estimates rather than direct indoor measurements may introduce exposure bias. |
[229] | 2021 | Lab | 103 patients in the intensive care unit, with a mean age of 53.6 years, completed the RCSQ. Their exposure to noise and ALAN was monitored hourly over one night using a Type 1 noise meter and a light meter. | The study revealed a mean noise level of 63.9 dB(A), with peak noise reaching 102 dB(A). The measured ALAN intensity averaged 104.1 lux, peaking at 271 lux. Both stressors were identified as significant predictors of poor sleep (P < 0.001). | As a cross-sectional study, causality is unclear. Self-reported data and ambient exposure measurements may introduce bias. Medication, psychological conditions, and the hospital setting might also affect sleep, limiting real-life applicability. |
[153] | 2022 | Eco | Data from 51,562 individuals (mean age 66.8 years) in the California Teacher Study cohort were analyzed to evaluate sleep performance. ALAN exposure was assessed using the New World Atlas , and noise levels were derived from the US National Park Service Sound Map. Environmental factors, such as green spaces and air pollution, along with demographic and socioeconomic attributes, were also considered. | ALAN and air pollution were linked to shorter sleep durations (< 7 h), while noise was associated with longer sleep onset latency (> 15 min) per 10 dB(A) (P < 0.05). Conversely, green space was associated with increased sleep duration and decreased latency. | Self-reported data may be affected by recall and misclassification biases. The study lacks information on the timing of outdoor light exposure and did not consider pre-bedtime device use. Additionally, the US Parks noise model may be less accurate for smaller roadways, and data on ALAN and noise exposure during the night is missing, which could contribute to exposure bias. |
[228] | 2022 | Survey | 552 volunteers, with a mean age of 36.7 years, completed questionnaires detailing demographic attributes and reported exposure to ALAN and noise. They also filled out the PSQI, Munich Chronotype Questionnaire, Cognitive Failures Questionnaire, and General Health Questionnaire. | The perception of ALAN was significantly associated with poorer sleep quality (P < 0.002), while individuals reporting noise in their bedroom had higher PSQI scores (P = 0.018), suggesting poorer sleep. However, noise within the bedroom showed no significant correlation with sleep duration. | As a cross-sectional study, it cannot determine causality. Additionally, self-reported data may introduce recall bias and misclassification. Perceptions of ALAN and noise were not measured directly, which may lead to exposure bias. Moreover, ALAN measurements did not include pre-bedtime exposure. |
[71] | 2023 | Field | 72 volunteers, with a mean age of 44 years, from 44 localities in Israel, wore smartwatches to record their sleep metrics and used a smartphone to log exposure to ALAN and noise for 30 consecutive nights. Diaries recorded subjective data, and questionnaires were used to gather demographic, behavioral, habitual, and health information. | Exposure to ALAN and noise before bedtime significantly reduced sleep duration by 9.7% to 9.9% and SE by 2.4% to 2.8% (P < 0.01). While ALAN exposure during sleep had no significant impact, noise was notably disruptive, reducing sleep duration by 13.5% and SE by 4.4%. The combined effect of ALAN and noise led to a substantial decrease in sleep metrics, with reductions of 15.3% in duration and 8% in SE before sleep, and 14% and 9%, respectively, during sleep. The results suggest that ALAN and noise may amplify each other’s effects, potentially worsening health outcomes. | The study’s limitations include its short duration (one month) and single-country scope, which may limit generalizability. Most participants were under 60, potentially overlooking age-related impairments. Variability in personal smartphones and smartwatches, as well as the lack of seasonal and spectral considerations for light and noise, may also affect results. |
ALAN: artificial light at night; CORT: cortisol; Eco: ecological; EEG: electroencephalographic; WASO: wakes after sleep onset; HR: heart rate; ipRGCs: intrinsic photosensitive retinal ganglion cells; S: shor wavelengths of light; L: long wavelengths of light; M: medium wavelengths of light; Lab: laboratory; MLT: melatonin; NREM: non-rapid-eye-movements; N1–N3: NREM 1 to 3 sleep stages; OR: odd ratio; OSA: obstructive sleep apnea; PSG: polysomnography; SWL: short wavelength light; PSQI: Pittsburgh sleep quality index; RCSQ: Richards-Campbell sleep questionnaire; REM: rapid eye movements; Retro: retrospective; SE: sleep efficiency; LED: light emitted diode; SWS: slow-wave sleep; NA: noradrenaline; VIIRS: Visible Infrared Imaging Radiometer Suit; 1 Arranged from oldest to newest and excludes reviews, letters, or books, focusing primarily on studies investigating the relationship with circadian cycles, sleep, and MLT; 2 Ref numbers are organized in the order they appear in the text; 3 The category “laboratory” covers research conducted in medical institutions, research facilities, and controlled clinical settings, whereas the “ecological” category includes both individual-based and population-based epidemiological studies
The photic pathway
In the early 20th century, a new perspective on the mechanics of light emerged through Einstein’s quantum theory [84]. This groundbreaking work revealed light as electromagnetic radiation with a captivating duality [85], existing as both particles and waves [84]. Consequently, light can be quantified through various means, including its illuminance intensity, measured in lux, lumens, or candles, as well as its spectral composition, i.e., the amount of energy (number of photons) as a function of wavelength, assessed in nanometers (nm) [86].
The daily light and dark cycles govern the regulation of circadian rhythms [87] and are calibrated by the eye in response to changes in light intensity and spectral composition [24, 25, 77, 88]. The human eye is recognized as one of the most complex organs in the body [89]. Light enters the eye and meets the cornea, which refracts and transmits the light to the lens, continuing to the inner surface and up to the retina [89]. The retina encompasses three types of photoreceptor cells: rods, cones, and melanopsin [90] (see Figure 2A). Rods facilitate scotopic vision, enabling sight in low-light conditions and discerning black-and-white imagery [91], with maximal sensitivity at 507 nm [24]. Alternatively, cones specialize in photopic vision, enabling the perception of colors [92]. There are three distinct types of cone cells responsive to short (S), medium (M), and long (L) wavelengths, with peak sensitivities at 420 nm, 534 nm, and 546 nm, respectively [24, 33].
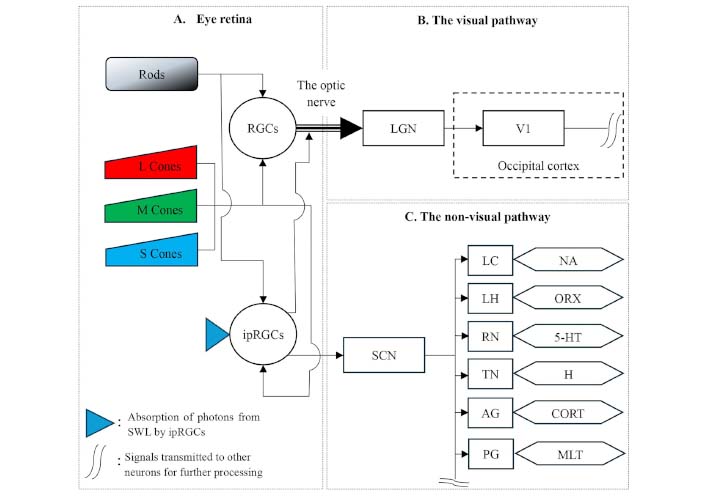
Schematic representation of the photic pathway. Light reaches the retina, which houses rods for low-light vision [94] and cones sensitive to short (S), medium (M), and long (L) wavelengths of light, enabling color differentiation (A) [92, 96]. These photoreceptors transmit signals to retinal ganglion cells (RGCs), which relay visual information via the optic nerve to the lateral geniculate nucleus (LGN) [33, 92, 96] (B). From the LGN, signals are processed in the occipital cortex, including the primary visual cortex (V1), and continue to higher-order brain regions [97] (B). Concurrently, signals from rods and cones reach intrinsic photosensitive RGCs (ipRGCs) [100], which contain melanopsin that peaks at 480 nm [92]. IpRGCs directly transmit signals to the suprachiasmatic nucleus (SCN) as part of the non-visual pathway [6, 92] (C). Melanopsin signals also influence visual perception in V1 [100, 101] (B). The SCN activates various neuronal clusters involved in neurotransmitter secretion, including the locus coeruleus (LC) for noradrenaline (NA), the lateral hypothalamus (LH) for orexin (ORX), the raphe nuclei (RN) for serotonin (5-HT), the tuberomammillary nucleus (TN) for histamine (H), the adrenal gland (AG) for cortisol (CORT) [10, 102, 104], and the pineal gland (PG) for melatonin (MLT) [74, 103, ] (C)
These traditional photoreceptors [33] are connected to retinal ganglion cells (RGCs), which facilitate the transmission of visual stimuli through the optic nerve to the lateral geniculate nucleus (LGN) along the optic chiasm [24, 33, 89]. Information processed within the LGN then travels to the primary visual cortex (V1) and onward to the secondary visual cortices and higher-order brain regions for further processing [93] (Figure 2B).
Alternatively, as suggested by Blume et al. [33] and Mirjam et al. [24], signals from rods and cones are also transmitted to intrinsic photosensitive RGCs (ipRGCs) situated in the inner retina [94]. The ipRGCs contain the light-sensitive photopigment melanopsin [95], which peaks at 480 nm [24]. Signals from the ipRGCs are directly transmitted to the SCN, constituting the non-visual pathway (Figure 2C). Furthermore, melanopsin signals reach V1, where they contribute to visual perception [96, 97] (Figure 2B).
The SCN coordinates various diurnal brain activities, including thermoregulation, heart rate (HR) control, and activation of key neuronal clusters such as the locus coeruleus (LC), lateral HYP (LH), raphe nuclei (RN), tuberomammillary nucleus (TN), and adrenal gland (AG). These regions are instrumental in producing wake-promoting neurotransmitters, including noradrenaline (NA), which enhances alertness but can impair cognition and contribute to the development of Alzheimer’s and Parkinson’s diseases [98]; orexin (ORX), which is involved in the maintenance of arousal, emotions, and behavior [99]; serotonin (5-HT), which affects emotion, reward, pain, cognition, and sleep-wake patterns [100]; histamine (H), which plays a role in the immune response [101]; and CORT, which influences stress levels, blood sugar regulation, and immune system function [102]. Furthermore, the rhythmic activity of the SCN governs the pineal gland (PG), stimulating the secretion of MLT [74, 103, 104] (see Figure 2C).
Noteworthy is the recognition by Mirjam et al. [24] of undefined neurons, organs, and cells contributing to the sleep-wake cycle [24]. Additionally, several scholars have suggested that light can penetrate the skull and reach the HYP, thereby amplifying SCN exposure to light and affecting circadian rhythms and MLT secretion [105–108]. While most studies have been conducted on animals, it should be acknowledged that the level of penetration varies with wavelength and intensity and may differ among species and across ages [109], hence stressing the need for further research.
ALAN and sleep
Considering the intricate anatomy of the eye and the subsequent sensitivity of the SCN to ALAN, researchers have delved into their interconnected dynamics. In an early study, Wams et al. [110] found that exposure to ALAN affected sleep architecture, modulated circadian function related to sleep, and influenced homeostatic sleep pressure [110]. Furthermore, Cho et al. [10] discovered that exposure to dim 40 lux fluorescent light during sleep resulted in longer N1 sleep, shorter SWS, and increased arousal, leading to shallow and unstable sleep [10]. These studies emphasize the profound effect of ALAN intensity during sleep.
Additionally, Wallace-Guy et al. [111] examined the association between ALAN exposure, sleep, and depressed mood. Their results indicated that pre-sleep exposure (three hours before sleep, with a median of 24 lux) had no significant correlation with sleep or depression. However, average daily exposure to ALAN was found to be statistically significant, with shorter sleep (P < 0.01), reduced wake within sleep (P < 0.05), and increased depressed mood (P < 0.01) [111]. In contrast, Didikoglu et al. [112] found a correlation between pre-sleep ALAN exposure and longer sleep onset, particularly when the exposure occurred 30 min before sleep (P < 0.05) [112]. Although poor sleep may be influenced by MLT and the circadian phase-response curve to ALAN [12, 113], discrepancies in results highlight the need for further research. This research should aim to elucidate the circadian response curve by comparing daily exposure with pre-sleep exposure—considering cumulative vs. direct effects—while addressing factors such as exposure onset, duration, and dosage (spectra and intensity).
In another study, Chellappa et al. [114] compared the effects of three polychromatic light sources: 2,500 K (27 lux), 3,000 K (29.8 lux), and 6,500 K (27.6 lux). They observed that exposure to the blue-enriched 6,500 K source for 2 h led to lower NREM electroencephalographic (EEG) density (0.8% and 0.2%), reduced SWS activity (0.4% and 0.3%), and a shorter average total sleep time by 4 and 12.7 min compared to the 2,500 K warm light and 3,000 K classic light, respectively, suggesting an acute effect of blue-spectrum light on sleep architecture [114]. Additionally, Green et al. [115] investigated the impact of short wavelength light (SWL) on sleep. They examined the effect of light emitted from computer screens simulating exposure to different compositions: 80 lux and 350 lux, representing low and high intensities, respectively, combined with wavelengths of 460 nm and 650 nm, representing SWL and long-wavelength spectra. Volunteers were exposed to different combinations of light, which were randomly changed daily. The exposure started at 21:00 and ended at 23:00, after which volunteers were allowed to sleep. They found that SWL exposure correlated with shorter average sleep duration (8.6 min to 16.4 min), an increased number of wakes after sleep onset (WASO, 2.1% and 3.1%), and decreased sleep efficiency (SE, 2.1% and 3.1%, P < 0.01). Moreover, SWL was found to reduce the proportion of SWS in the sleep architecture (6.5% and 7.7%, P < 0.05) when comparing low and high intensities of light [115], highlighting the potential health concerns linked to SWL emitted from computer screens, smartphones, and tablets [115].
ALAN and MLT
Discovered by the American physician Aaron Lerner [116], N-acetyl-5-methoxy-tryptamine commonly known as MLT, is often referred to as the “hormone of darkness” [117]. It is primarily secreted by the PG, though not exclusively [118]. Lewy et al. [119] were the first to observe that exposure to light suppresses MLT secretion in humans. They found that exposure to 2,500 lux for an hour reduced MLT concentrations to nearly daytime levels [119]. Subsequent studies have further elucidated this phenomenon. For example, Zeitzer et al. [12] discovered that plasma MLT suppression began at 10 lux and increased with light intensity, with complete suppression occurring above 200 lux [12]. Moreover, several studies have highlighted the acute impact of SWL, specifically in the range of 470–525 nm [120] and even at a lower wavelength of 460 nm [115], underscoring its association with melanopsin [121, 122]. Recent research by St. Hilaire et al. [123] has shed further light on the intricate interplay between spectral exposure and MLT secretion. Their findings suggest an initial influence of cone photoreceptors in driving MLT suppression and phase resetting, followed by the dominance of melanopsin, which is particularly responsive to light at 481 nm, during prolonged exposures [123].
Interestingly, MLT suppression is not exclusively associated with normal vision. Czeisler et al. [124] demonstrated a 69% decrease in plasma MLT concentration in 3 of 11 blind patients after exposure to bright light, mirroring findings from healthy volunteers who experienced an average 66% decrease [124]. In a later study, Zaidi et al. [125] observed that exposure to monochromatic blue light at 460 nm reduced MLT secretion by 57%, delayed the MLT circadian rhythm by 1.2 h, and increased alertness, as indicated by EEG tests among blind volunteers with non-functional retinas [125]. Hull et al. [126] further confirmed these results, noting a reduction of more than 33% in MLT concentration among five blind patients following exposure to bright light [126].
This evolving understanding underscores the profound impact of light, both in intensity and spectrum, on MLT dynamics, shaping the sleep-wake cycles [127]. Additionally, it highlights the intrinsic role of the ipRGCs via the non-visual pathway and sensitivity to SWL, which suppress MLT, elevate alertness, and impede sleep [125, 126].
MLT concentration fluctuates throughout the day, typically rising one to two hours before bedtime and sharply declining in response to light exposure [128]. Moreover, Gooley et al. [129] discovered that the timing of light exposure influences MLT secretion. They found that pre-sleep exposure to 200 lux delayed MLT onset by 94 min and shortened its duration by 92 min (P < 0.05) compared to exposure to dim light (less than 3 lux) [129]. Therefore, MLT response to ALAN involves both a dose-response inhibition of MLT [123, 130] and a phase response in the temporal modulation of the rhythm [11, 120].
Swop et al. [131] pointed out that MLT sensitivity to light may differ based on factors such as age, gender, and the presence of mental or physical disorders [131]. Furthermore, Higuchi et al. [132] emphasized MLT’s responsiveness to changes in daily light patterns due to seasonal variations. These variations inform the biology of the time of year, helping to synchronize reproductive and biological activities accordingly [132, 133].
MLT exhibits anti-inflammatory, immunomodulatory, and antioxidant properties [134, 135]. It plays a significant role in immune system function [136], promotes tissue regeneration [137], acts as an anti-aging agent [138], reduces macromolecular damage by scavenging harmful free radicals [139], protects DNA integrity against malicious bindings, and helps suppress cancer resistance to treatments [140]. Consequently, suppression of MLT due to ALAN exposure could potentially compromise immune function, leading to an increased risk of early mortality [141].
Health effect of ALAN on MLT, and sleep
Given SCN function as the regulator of key neurotransmitters i.e., NA, ORX, 5-HT, H, CORT, and MLT [74, 93, 103, 104], alongside its central role as the circadian pacemaker, scholars have directed their attention toward unraveling this intricate mechanism shedding light on the health implication of ALAN exposure and consequently MLT suppression for human well-being.
Numerous studies have linked ALAN exposure to hormone-dependent cancer, including breast cancer [142–145] and prostate cancer [146, 147], both of which are associated with MLT suppression [9, 148]. Additionally, MLT suppression and circadian rhythm disruption have been associated with overweight, obesity [149–151], and diabetes [130, 152, 153]. Moreover, MLT plays a crucial role in the synthesis, secretion, and action of insulin [154]. Therefore, circadian misalignment can lead to late-night snacking, contributing to metabolic dysfunction [155]. Furthermore, ALAN has been associated with fatigue [156], circadian rhythm phase shifts [157], and molecular changes in blood vessels and the heart [158], which can contribute to cardiovascular disease development [130, 152, 153, 159]. These conditions collectively have the potential to disrupt sleep [6, 54, 70, 71].
Furthermore, insufficient exposure to sunlight has been linked to vitamin D deficiency, which also suppresses MLT and impedes sleep, leading to similar health dysfunctions [160–163]. The most recent study by Sirhan-Atalla et al. [149] identified ALAN, rather than vitamin D, as a more significant predictor of these effects (P < 0.05).
Notably, the effect of light exposure may vary with age [164], influenced by age-related degradation of the eye, manifested by yellowing of the lens [165]. Nevertheless, invasive interventions, e.g., cataract surgery, have demonstrated a positive effect on sleep [166, 167]. Moreover, as suggested by Shishegar et al. [168], daily exposure to varying color temperatures of light, from 6,500 K in the morning to 2,700 K in the evening, at intensities of 500 lux and 100 lux, respectively, can enhance sleep quality and reduce disturbance among the elderly compared to a constant 2,700 K lighting setup [168]. Additionally, Chellappa [169] identified several factors that may contribute to individual sensitivity to light and consequently affect sleep, including gender, chronotype, genetic haplotypes, individual circadian hallmarks, and retinal sensitivity [169]. Similarly, Xiao et al. [170] reported that elderly women (aged 50–71 years) sleep more than men, and the association between ALAN and shortened sleep is more pronounced in neighborhoods with higher poverty levels [170].
The auditory pathway
Sound is the result of waves and pressure fluctuations produced by mechanical movements and propagated through air or other media. It is characterized by frequency (Hz), wavelength (m), pressure (Pascal), and intensity, also known as sound pressure level (Lp), measured in decibels (dB) [171, 172]. Perception of sound varies subjectively [3], with noise typically classified as unwanted sound [73]. The human hearing threshold ranges between 20 Hz and 20 kHz but can vary with age and health [73, 171]. The sound pressure levels reported herein are A-weighted, which adjusts for the human ear’s varying sensitivity to different frequencies. This provides a decibel reading that more accurately reflects perceived loudness, denoted as dB(A) [173].
The human ears are situated within the temporal bone on both sides of the skull, comprising three primary sections: the outer, middle, and inner ear. They are asymmetric, thus enabling the localization of sound in space [174]. Incoming sound waves first reach the external pinna (auricle). The pinna collects incoming sound waves and channels them through the concha—a shell-shaped cavity—towards the opening of the ear canal. Subsequently, the sound waves travel through the ear canal, which is “S”-shaped, and reach the tympanic membrane, also known as the eardrum, separating the outer ear from the middle ear and vibrating in response to pressure variations carried by sound waves [174] (see Figure 3A). Within the ear canal lies the cartilaginous structure, where cerumen (wax) is secreted and together with hair follicles, aids in skin moisturization and trapping airborne particles [175]. This intricate design of the outer ear facilitates the amplification of sound pressure by approximately 10 dB(A) to 15 dB(A) and the protection of the tympanic membrane [176].
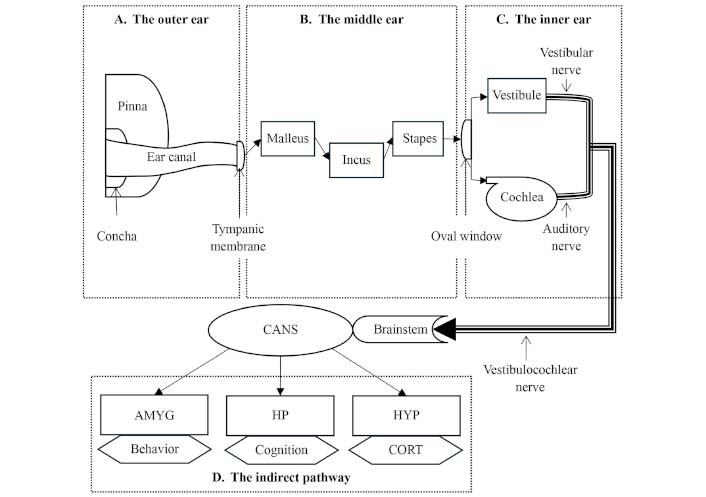
Schematic representation of the auditory pathway. Sound waves enter through the outer ear, where the pinna and concha collect the sound waves, which are then directed to the tympanic membrane (eardrum) [174] (A). Within the middle ear, acoustic pressure is transduced into amplified mechanical pressure through the three ossicles (malleus, incus, and stapes) [174, 176, 177] (B). This amplified pressure is then transferred to the inner ear through the oval window. In the inner ear, mechanical pressure is transformed into electrical signals by the cochlea. These signals, combined with vestibular inputs, are transmitted via the vestibulocochlear nerve to the brainstem nuclei and the central auditory nervous system (CANS) [174, 176] (C). An indirect pathway involves subcortical areas encompassing the amygdala (AMYG), hippocampus (HP), and hypothalamus (HYP), influencing behavior, cognition, and cortisol (CORT) secretion [178–182] (D)
Within the tympanic cavity, nestled in the middle ear, reside the three smallest bones in the human skeleton: the malleus (hammer), the incus (anvil), and the stapes (stirrup) [177]. Together, they form the ossicle chain, which transfers subtle air pressure fluctuations captured by the tympanic membrane to the oval window, demarcating the boundary between the middle and the inner ear [174] (see Figure 3B). This mechanical arrangement serves as a transducer, bridging the gap between the air-filled environments of the outer ear and the fluid-filled inner ear. The mechanical motion of these bones increases acoustic pressure, effectively addressing the impedance mismatch between the air-filled and fluid-filled media [176].
The inner ear consists of two distinct fluids—the perilymph, rich in sodium, and the endolymph, high in potassium. This chemical contrast establishes an electrical potential difference supporting the sensory function of the inner ear organs [174]. Positioned in the upper region of the inner ear, the vestibular system governs balance, while the cochlea houses the sensory organs responsible for hearing—the organs of Corti. Hair cells within the organs of Corti translate mechanical movements of the cochlear fluids into electric signals, which are then transmitted via the auditory nerves [176]. The vestibulocochlear nerve, formed by the auditory and vestibular nerves, conveys this collective data through the brainstem to the central auditory nervous system (CANS) in the brain, where auditory sensation is processed [174] (see Figure 3C).
Recent studies [178–182] indicate the projection of auditory inputs into non-auditorial subcortical regions via neuronal connections [178]. These regions include the amygdala (AMYG), the hippocampus (HP) [183], and the HYP [179]. The AMYG, nestled in the limbic region, plays a key role in regulating emotions and consequent behaviors. Therefore, noise exposure can evoke feelings of fear, alter human behavior [184], and subsequently impact sleep patterns [185]. Another integral limbic structure, the HP, is primarily associated with learning, cognition [186], and memory consolidation during sleep [187]. Finally, the HYP, one of the oldest and smallest parts of the brain, governs various body functions, including metabolism, thermoregulation, SCN function, and sleep (see explanation above—“the photic pathway”) [188], extending to the regulation of CORT secretion by the AG (see Figure 3D).
Noise, sleep, and CORT
In their report, the World Health Organization (WHO) identified noise as a significant health stressor, with traffic highlighted as the most potent source [189]. Specifically, Ising and Braun [190] shed light on the detrimental effects of noise pollution on the human body, indicating that exposure to continuous sound levels equal to or exceeding 30 dB(A) and maximal noise exceeding 55 dB(A) during sleep elevated CORT and NA levels, contributing to stress-related diseases [190]. Later studies by Babisch et al. [191] and Maschke et al. [192] have corroborated these results.
Noise can affect sleep architecture, alter sleep behavior, and potentially induce insomnia. Vallet et al. [193] indicated a significant phase shift in sleep architecture at average continuous sound levels of 48.5 dB(A), accompanied by awakening events observed at 50.3 dB(A) (P < 0.01) [193]. Additionally, Eberhardt et al. [194] identified changes in sleep patterns at 45 dB(A) (P < 0.01) and an arousal response at continuous sound levels exceeding 55 dB(A) (P < 0.05) [194], while Okada et al. [195] noted changes in sleep patterns even at lower noise intensities of 40 dB(A) [195]. Aasvang et al. [196] revealed a negative association between exposure to railway noise above 50 dB(A) and REM sleep (P = 0.02) [196]. Moreover, noise peaks during the night correlated (P < 0.05) with increased awakening, higher REM sleep, and reduced episodes of SWS and sleep spindles, both considered sleep-protective processes [197]. Insomnia was found to be significantly associated with noise levels exceeding 55 dB(A) (P < 0.05), as established by Halonen et al. [198].
Noise in hospitals has been recognized as a significant stressor in public health, potentially impeding sleep, slowing recovery, and affecting medical staff performance [199, 200]. Thus, Buxton et al. [72] investigated changes in sleep architecture in response to hospital noise stimuli and found an increase in arousal during N2 sleep. Moreover, this noise caused a greater and more sustained elevation in instantaneous HR, suggesting stress and annoyance [72]. In another study, Park et al. [201] assessed the effect of noise on sleep using the Pittsburgh Sleep Quality Index (PSQI) [202]. They discovered that an average daily noise level of 63.5 dB(A) measured in hospital wards reduced sleep quality among 86% of the patients and was significantly associated with sleep disturbance (P < 0.05) [201]. Similarly, Simons et al. [203] indicated that daily exposure to 54 dB(A) had a negative impact on sleep quality (P < 0.05). Guisasola-Rabes et al. [204] further elucidated these findings, highlighting that for every 1 dB(A) increase in noise, a hospitalized patient's score on the Richards-Campbell Sleep Questionnaire (RCSQ) [205] decreased by 3.92 points, indicating poorer sleep quality (P < 0.05) [204].
Prolonged exposure to noise has been found to acutely affect sleep. Gitanjali and Ananth [206] showed that exposure to continuous occupational noise exceeding 75 dB(A) for eight hours during the day reduced SE. Additionally, this exposure significantly decreased the duration of both REM sleep and SWS while also delaying the onset of REM sleep (P < 0.01) [206]. Similarly, a later study by Lin et al. [207] found consistent results, revealing that individuals exposed to an average noise level of 76.8 dB(A) had a lower percentage of SWS and decreased SE (P < 0.05) compared to a low-noise group with an average level of 61.0 dB(A). Furthermore, post-exposure assessments showed associations with increased CORT levels, heightened sympathetic activity, and elevated blood pressure (P < 0.05) [207]. These results underscore the association between pre-sleep exposure to noise and its detrimental effects on sleep quality and physiological responses, which can lead to psychological outcomes such as stress and mental dysfunction.
Röösli et al. [208] suggested that the timing of noise exposure during sleep may influence sleep patterns. They found that exposure to noise within the first four hours of sleep increased sleep latency by 5.6 min per 10 dB(A). Additionally, an increase of 10 dB(A) during the three hours before waking reduced SE by 2–3% (P < 0.05) [208].
Age-related differences in noise sensitivity were highlighted by Öhrström et al. [209]. They compared children (mean age 10.8 years) with their parents (mean age 41.9 years) and found better sleep performance among the children. They also observed an acute effect on sleep metrics at continuous sound levels of 60 dB(A) to 64 dB(A) over 24 h, affecting both children and parents [209].
Bedroom window location may contribute to better sleep, as indicated by Bodin et al. [210], particularly in rooms facing quiet yards, water, or green spaces (P < 0.05). However, a later study by Bartels et al. [211] found no significant differences in self-reported poor sleep between bedroom windows facing streets with no traffic and those facing low-traffic streets. The researchers acknowledged that “streets with no traffic” do not necessarily guarantee a quiet façade. Despite this limitation, sleeping in bedrooms with windows facing streets with no traffic still yields improved sleep quality [211].
Due to the proliferation of wind turbines, noise frequency has been recognized as a potential risk factor. Initial studies suggested that low-frequency noise might affect humans more significantly than infrasound [212]. However, recent research contradicts this notion, highlighting the adverse impact of infrasound [213]. Additionally, low-frequency noise has been linked to increased CORT secretion [214, 215] and is subjectively associated with changes in sleep quality, mood, and annoyance [216–219]. Nevertheless, its correlation with objective sleep metrics remains inconclusive due to insufficient empirical support [216, 220–222].
The combined effect of ALAN and noise
As discussed earlier, both ALAN and noise are recognized as significant disruptors of sleep with negative health consequences. In everyday life, these stressors continuously emanate from myriad sources, including electronic devices, household appliances, advertising billboards, road illumination, motor traffic, commercial areas, and recreational spaces. Consequently, individuals are exposed to a constant barrage of these stressors, similar to uncontrolled emissions stimulating the senses—eyes and ears—with varying intensities and frequencies, encompassing both ALAN and noise.
Moreover, ALAN and noise share intrinsic mechanisms and exhibit comparable characteristics. They affect similar sub-cortical structures, such as the HYP, and contribute to hormonal imbalances, including MLT suppression and CORT hypersecretion. They also lead to behavioral changes, cognitive impairment, stress induction, and a weakened immune response [58, 71, 77, 156–160]. As a result, their combined effects may exacerbate the impact of each individual stressor. Therefore, understanding their combined impact has become increasingly urgent and essential.
In an earlier study, Ohayon and Milesi [223] investigated the relationship between exposure to outdoor ALAN and sleep patterns within a cohort of 19,136 individuals aged 18 to 99 years. Leveraging questionnaires, they collected sleep data and potential confounding factors, including self-reported noise levels. External assessments of ALAN were derived from Defense Meteorological Satellite Program data, using NASA’s Visible Infrared Imaging Radiometer Suite (VIIRS) instruments [224]. They found that increased exposure to outdoor ALAN correlated with delayed bedtimes, later wake-up times, shorter sleep durations, and increased daytime sleepiness (P < 0.01). Intriguingly, noise levels showed no statistically significant correlation with sleep metrics [223].
In contrast, Zhong et al. [153] amalgamated sleep data from the California Teacher Study cohort [225] with assessments of ALAN exposure from the New World Atlas [226] and noise evaluations derived from the US National Park Service Sound Map [227]. Their study revealed that decreased sleep duration was linked to increased ALAN exposure and found a significant correlation between noise levels and sleep latency (P < 0.05) [153].
Cleary-Gaffney et al. [228] explored the subjective interplay of ALAN and noise with psychological distress and sleep using a self-reported questionnaire and the PSQI questionnaire. They found that the perception of ALAN was significantly associated with poorer sleep quality (P < 0.002), while individuals reporting noise in their bedroom had higher PSQI scores (P = 0.018), suggesting poorer sleep. However, noise within the bedroom showed no significant correlation with sleep duration [228].
Schnelle et al. [83] tested a behavioral intervention aimed at limiting exposure to ALAN and noise among nursing home residents. They measured ALAN and noise levels using professional instruments—a light meter and a microphone—and recorded sleep metrics using actigraphy under controlled conditions. While their behavioral intervention yielded significant reductions in ALAN and noise exposure compared to the control group (P < 0.01), no significant changes were observed in sleep patterns. The authors suggest that the sleep results may have been influenced by individuals’ daytime napping and medical conditions, which were not considered in the study. Additionally, despite a significant decrease, the noise intensity after the intervention remained relatively high, with peaks exceeding 50 dB(A).
In another study, Younis et al. [229] explored the effects of ALAN and noise on patients sleeping in intensive care units using a light meter and a sound meter. Sleep quality was assessed through a self-reported questionnaire. The study revealed a mean noise level of 63.9 dB(A), with peak noise reaching 102 dB(A). The measured ALAN intensity averaged 104.1 lux, with peaks reaching 271 lux. Both stressors were identified as significant predictors of poor sleep (P < 0.001) [229].
These studies offer insights into the relationship between ALAN and noise and their effects on sleep. However, they do not thoroughly explore the mechanisms underlying their combined impact. Additionally, while most studies indicate a statistical association, these studies lack precise quantitative measures of this relationship. To address this gap, Gabinet and Portnov [70] conducted a comprehensive examination of the association between ALAN and noise exposure and their combined effects on sleep, controlling for various confounding factors, including age, gender, behavior, health, education, and income [71].
In the first study, Gabinet and Portnov [70] juxtaposed outdoor ALAN exposure derived from NASA’s VIIRS archive [224] with noise data interpolated from road densities based on road maps, comparing these with sleep data collected through questionnaires. Their findings revealed that both increased road density and ALAN intensity were linked to reductions in sleep duration of 4.5% and 3%, respectively, and heightened reported sleep difficulty of 3.5% and 11%, respectively (P < 0.01). Moreover, they uncovered an interaction effect between ALAN and noise. Specifically, they noted that an increase in ALAN within the high-noise exposure group enhanced sleep duration but concurrently increased reported sleep difficulty (P < 0.01) [70]. This correlation may be attributed to the exposure mechanism, where ALAN, constantly emitted into the environment, may infiltrate sleeping rooms, especially in places where residents are reluctant to lower their shades due to hot and humid weather conditions [230].
In their subsequent empirical study, Gabinet and Portnov [71] collected data from 72 volunteers who used smartphones to record ALAN and noise exposures. Sleep metrics were tracked via smartwatches. They found that exposure to ALAN and noise before bedtime led to a significant reduction in sleep duration by 9.7% to 9.9% and SE by 2.4% to 2.8%, respectively (P < 0.01). These results are coherent with earlier studies linking pre-sleep exposure with MLT suppression [12, 120] and CORT hypersecretion [207]. Interestingly, while ALAN exposure during sleep time showed no significant impact on sleep, noise exerted a dominant influence, resulting in a notable reduction in sleep duration by 13.5% and SE by 4.4% [71]. This is similar to the findings observed by Foo et al. [231]. Additionally, the combined effect of ALAN and noise led to a substantial decrease in sleep metrics. Concurrently, during the pre-sleep phase, sleep duration and SE were reduced by 15.3% and 8%, while during the sleep phase, reductions were 14% and 9%, respectively. Based on these findings, the authors conclude that ALAN and noise tend to amplify one another, potentially exacerbating health-related issues [71].
Conclusions
Throughout their lifespan, healthy individuals typically devote roughly one-third of their lives to the essential activity of sleeping [39]. Recognizing the profound significance of sleep for human health [232], sleep hygiene has emerged as a focal point among scholars and medical professionals. It serves as a proactive therapeutic approach aimed at ensuring longevity and maintaining a high quality of life [233].
As urbanization expands and population density increases, urban habitats are inundated with ALAN [19] and disharmony of unnatural sounds from technological marvels such as light emitted diodes (LEDs), smartphones, and tablets, which serve as sources of SWL [6, 115], along with an increased volume of motor traffic [3, 21]. These stressors are now recognized for their detrimental effects, supported by a growing body of research (see, inter alia, [9, 18, 76, 144, 146, 149, 190, 198, 234–243]). Empirical studies presented herein have elucidated how both ALAN and noise disrupt the natural circadian rhythm [25, 33, 38, 244–249], interfere with sleep patterns [10, 111, 112, 114, 192–195, 209], suppress the secretion of MLT [12, 119, 120], trigger the hypersecretion of stress hormones including CORT and NA [190–192], and lead to various health and mental impairments, such as nausea and fatigue [250, 251], cardiovascular disease [82, 130, 152, 153, 159, 252], hearing loss [239, 248], hormone-dependent cancers [9, 142–145, 147, 149, 253–255], and obesity and overweight [149–151].
Both ALAN and noise often emanate from similar sources and persistently emit into the environment. They share similar intrinsic mechanisms, potentially influencing each other’s effects [24, 74, 93, 178–182]. Several scholars have tried to disentangle this interconnected association by studying both ALAN and noise, yielding varied results. While some have suggested that both stressors contribute to poor sleep [70, 153, 228], others have proposed that only ALAN is associated with sleep disturbances [223]. However, these findings often stem from ecological studies that rely on satellite data to estimate nighttime exposures in sleeping environments. These studies typically lack control for individual-level confounders and may suffer from misclassification biases [256]. Personal dosimetry is ideal, as exemplified by Gabinet and Portnov [71], who conducted a real-life study using real-time measurements. This study underscores that pre-sleep exposure to both ALAN and noise acts as a potent stressor with established potential to disrupt sleep patterns. Interestingly, during sleep, noise emerged as the predominant influence on sleep. Furthermore, these stressors frequently synergize, amplifying one another’s adverse effects [71], which can influence overall health.
The present review pioneers the exploration of the combined effects of ALAN and noise on sleep, along with their intricate interaction, shedding light on the prevailing mechanisms underlying these stressors. In doing so, it offers novel insights into the existing body of knowledge. Furthermore, it meticulously outlines recent research on sleep and health dysfunctions linked to these stressors, thereby providing a necessary framework for reshaping current policies and standards.
While comprehensive, it is important to acknowledge the possibility of overlooking pertinent studies, which could result in a knowledge gap and limit the scope of the review. To address this issue, the author has implemented a methodological approach aimed at minimizing the likelihood of missing relevant publications.
Despite the well-established findings outlined, there is a clear need for further research to enrich current understanding [24]. This research can utilize advanced approaches such as smart technology [71] and citizen science principles [257–259] to enable unobtrusive, large-cohort field studies. Such studies would enhance comprehension of the underlying physical processes and stressors associated with sleep [220–222], refine existing models, elucidate the mechanisms of synergy [71], and introduce other potential physical variables, e.g., temperature [260], humidity [261], and air pollution [67]. Furthermore, these research endeavors are fundamental in fostering the development of innovative solutions and approaches, including the use of ALAN and noise as therapeutic modalities [262–276].
Translating research into actionable strategies for driving sustainable environmental change demands a comprehensive approach. This approach includes limiting exposure while advocating for healthy sleep hygiene practices [233, 277]. It can be achieved through strategically integrating policies that engage key stakeholders, foster improved communication about environmental challenges, minimize resistance or barriers to change, and establish common ground that encourages economic investment [278].
Such policies may necessitate the enforcement of more stringent environmental standards [235], e.g., adjusting noise and light thresholds to facilitate recovery and healing in hospitals and nursing homes [83, 229, 279], or securing a healthy environment in urban areas [280, 281]. This could involve advocating for legislative requirements for acoustic materials to ensure a noise-free environment [236, 237], as well as promoting technological advancements, which may include the development of monitoring and control systems [238]. Additionally, initiatives like smart lighting, which adjusts the intensity to align with residents’ needs while preserving environmental health [282], can be encouraged.
Lastly, investing in public awareness campaigns is crucial for promoting responsible behavior [240, 241], and for cultivating a sustainable environment conducive to quality sleep and overall well-being.
Abbreviations
ALAN: | artificial light at night |
AMYG: | amygdala |
CORT: | cortisol |
ipRGCs: | intrinsic photosensitive retinal ganglion cells |
MLT: | melatonin |
N1-N3: | non-rapid eye movement stages 1 through 3 |
NA: | noradrenaline |
NREM: | non-rapid eye movement |
PSQI: | Pittsburgh sleep quality index |
REM: | rapid eye movement |
SCN: | suprachiasmatic nucleus |
SE: | sleep efficiency |
SWL: | short wavelength light |
SWS: | slow-wave sleep |
Declarations
Acknowledgments
The author wishes to express sincere gratitude to Boris A. Portnov from the University of Haifa for his invaluable insights, which have significantly enhanced this review. Special thanks are also extended to Noa Weiss and Noga Becker for their valuable assistance with language editing.
Author contributions
NMG: Conceptualization, Investigation, Methodology, Writing—review & editing, Writing—original draft.
Conflicts of interest
The author declares that he has no conflicts of interest.
Ethical approval
Not applicable.
Consent to participate
Not applicable.
Consent to publication
Not applicable.
Availability of data and materials
Not applicable.
Funding
Not applicable.
Copyright
© The Author(s) 2024.