Abstract
Depression is characterized by affective symptoms and neuropsychological deficits. After treatment, affective symptoms frequently remit, but cognitive alterations may remain affecting the lives of people and having an impact on health, economy, and societies. The present work describes the methods, procedures, and equipment for a randomized three-arm controlled experiment designed to measure the effects of exergames on executive functions (EFs) under depression. EFs are complex cognitive functions that are essential for organizing information, planning an action, reasoning, decision-making, and problem-solving. Literature shows that EFs are compromised in depression, affecting daily-life activities and other cognitive domains. To conduct the experiment, depressed middle-aged adults will be randomly distributed into three experimental groups: an intervention group training with exergames, an active control group training only with cognitive video games, and a passive control group or wait-list. EFs are evaluated at three different time points: pre-post intervention, and three months follow-up, using the Wisconsin Card Sorting Test (WCST). Researchers will collect cognitive-behavioral and neural information [event-related potentials (ERPs)]. Results will be explored by performing analysis of variance to compare the outcomes of the diverse groups at the three different time points, understanding the benefits of exergames in terms of EFs under depression. The study of simultaneous multidomain interventions in depression holds promise for the development of novel approaches, to implementing psychological and neuropsychological health [this experiment is part of a registered clinical trial entitled “MUDgame” (ClinicalTrials.gov identifier NCT06536530). Registered on August 2024, https://clinicaltrials.gov/study/NCT06536530].
Keywords
Depression, exergames, neuropsychology, neuroplasticity, cognitive neuroscience, executive functions, event-related potentialsIntroduction
Background
During recent decades, societies have improved their efforts to build sustainable societies where people can experience well-being and happiness. Nonetheless, people suffer increasing levels of sadness, worry, and anxiety [1, 2].
Depressive disorders are estimated to affect 300 million people worldwide [3]. For instance, in the United States, Spain, Austria, Portugal, and Australia, depression has an estimated prevalence rate of approximately 5% [4]. Additionally, recent epidemiological studies show a significant increase in depression, anxiety, and further psychological conditions [5–7].
Depression is characterized by affective-related problems and cognitive deficits (i.e., changes in attention, memory, process of information, or decision-making) [8–10]. However, the cognitive alterations associated with depression are not usually evaluated at the beginning or after remission of the clinical symptoms, compromising the lives of people and having an impact on societies, work performance, and the economy [11–13].
Objectives
The current work consists of an experiment to assess how executive functions (EFs) under depression can benefit from a cognitive intervention consisting of exergames and video games integrating simultaneous cognitive and psychomotor stimulation.
EFs are related to the ability to organize information, planning, reasoning, decision-making, and problem-solving. These functions are essential in our daily life activities. They are complex mental abilities that include cognitive domains, such as executive attention, working memory, cognitive control, inhibition, cognitive flexibility, updating, or shifting [14].
Experimental and review studies show that depression is associated with an impairment in EFs. Deficits are related to the domain of shifting or switching between tasks, a dysfunction in the ability of inhibition (cease actions or thoughts that are no longer useful), or a broad impairment of EFs in depressed individuals [15–17].
Nowadays, interventions based on video games are facing troubles with motivation, engagement, and adherence associated with previous cognitive stimulation programs [18]. Regarding depression, past research analyzed video games’ global positive effects, but there are no studies systematically revising specific contributions of video games to cognitive domains altered in depression [19].
Using exergames with sound methods may allow us to overcome previous limitations and reach targeted effects. Physical training has been demonstrated to have a positive effect on cognition in people suffering from depression [20], and multidomain training (combining cognitive and physical stimulation) has shown higher effects than unidomain interventions [21].
As depression is characterized by a decrease in energy and psychomotor functioning [22], physical training could be excessively demanding, thus exergames combining cognitive stimulation and moderate physical activity may be a better suited novel approach.
Previous studies analyzing the effects of exergames on depression are scarce and cross-sectional, and they do not focus on the cognitive impairment associated with depression. In addition, these studies do not include an active control group that trains in similar conditions as the intervention group, and they do not measure the placebo effects depending on previous experience, expectations, motivation, and engagement, so it is difficult to accurately ascertain the effects of the programs [23].
In this study, we overcome previous limitations by including a longitudinal randomized controlled experiment focalized on the benefits of exergames on EFs in depression, with appropriate control groups and placebo-controlled. In addition, neural activity will be explored to understand the underlying mechanisms associated with the effects derived from the intervention.
To assess cognitive-behavioral and neural changes, the Wisconsin Card Sorting Test (WCST) is used. The studies comparing EFs between people suffering from depression and healthy participants frequently show a different performance in multiple measures of the WCST: the number of categories completed, total correct responses, errors, perseverative responses, and so forth [24–27].
Moreover, after remission, previously depressed individuals continue showing alterations in a variety of executive abilities such as updating information, cognitive control, shifting, or inhibition [15, 28–31].
From a cognitive neuroscience perspective, the WCST has been used to explore the electrophysiological components associated with EFs [32–34] and is a tool that has been extensively used to assess damage and deficits associated with the frontal lobes [35].
Frontal lobes play a fundamental role in filtering and selecting information that is later processed and integrated with other associative brain areas. Thus, frontal lobes have been related to EFs or the ability to organize information, planning, decision-making, cognitive control, mind-shifting, and so on [36, 37].
Functional neuroimaging studies show that depression is associated with patterns of abnormal brain activation in different regions, including frontal areas: the dorsolateral prefrontal cortex, superior temporal cortex, cingulate cortex, insula, hippocampus, etc. Registrations show hypoactivity or hyperactivity of these brain networks [38].
From a structural approach, young and older adults suffering from depression show smaller gray-matter volumes relative to controls in the frontal-limbic regions: the right dorsolateral prefrontal cortex, the orbitofrontal cortex, cingulate, cerebellar, temporal, and parietal regions [39–41]. Meanwhile, reduced white matter was also observed in the frontal-limbic circuit, extending from the genu of the corpus callosum to the inferior fasciculus and posterior thalamic radiation [42, 43].
In addition, electroencephalographic (EEG) studies have shown differential brain activity in depressive subjects. Spectral analysis that shows the power amplitudes of each band associated with brain mechanisms reports that alpha, beta, gamma, theta, and delta activity can work as biomarkers that allow us to differentiate between depressive and nondepressed participants [44].
The present experiment explores neural activity through electroencephalography, a non-invasive and easy-access technique with high temporal resolution. Previous studies show alterations in depression regarding brain components linked to EFs: P300, P200, N100, mismatch negativity (MMN), error-related negativity (ERN), etc. Thus, we will explore the neuro-cognitive effects of exergames in depression concerning this brain activity.
P300 or P3 is a positive deflection that occurs 200–500 ms after a stimulus. Its amplitude and latency are related to information processing, stimulus evaluation, categorization, decision-making, and working memory. P3 is frequently elicited in a task where the target stimuli are rarely presented among repetitive standard stimuli, and it is frequently modality independent.
The distribution of P3 is frontal, parietal, and central, and larger P3 (P3b) amplitudes are associated with set-shifting and memory update. Different experimental designs can be used to study the subcomponents of P3, including P3a and P3b.
The P3b component has a centro-parietal scalp distribution, occurs approximately 300–500 ms after the target stimulus, and is related to the allocation of attentional resources, working memory operations, and the decision-making process.
Moreover, attention ability seems to be related to shifting [45]. Indeed, some researchers have demonstrated using the WCST that P3b modulation can be attributed not only to the selection or storage of sensory stimuli but also to an internal shift in the perceptual rule that is being used to sort the cards [34].
P3b reflects top-down processing, whereas P3a has a frontocentral scalp distribution, occurs earlier than P3b, and reflects the bottom-up capture of attention and orientation after an odd stimulus is presented. Different studies have shown that people suffering from depression exhibit decreased P3 amplitudes and prolonged latency [46–49].
Further brain components have been associated with EFs (i.e., the N1 and its subcomponents). The lateral occipital visual N1 subcomponent is related to attention and discriminative processing [50–52]. Some studies have found a significant correlation between target-locked N100 and information processing P3. Authors hypothesize that a lower amplitude of N100 could be associated with a dysfunction in attention and perception, affecting later cognitive processes linked to information-updating in working memory [53].
Additionally, under depressive conditions, the N1 component helps to differentiate between depressive and healthy participants. Showing higher latency and lower amplitude in N1 under depressive conditions [48, 54–56].
Taking into consideration the auditory sensory system, the MMN response is registered when subjects are exposed to identical stimuli with occasional mismatching stimuli (e.g., a sequence of 800-Hz tones and occasional 1,200-Hz tones). The mismatching stimuli elicit a negative-going wave that frequently peaks between 160 and 220 ms at midline scalp sites. This activity reflects an automatic process that compares new stimuli to an auditory sensory memory of previous stimuli [57].
However, some works have demonstrated that MMN is not just associated with an automatic pre-attentive response to deviant auditory stimuli, but also with voluntary effort, related to attention switching or mental shift.
Toyomaki et al. [58] found a correlation between low MMN amplitude and poor EFs performance in schizophrenia patients using nonauditory tasks: the WCST for exploring cognitive switching, and the Stroop test to assess cognitive control or response inhibition.
MMN may receive some contribution from frontal sources. People with dorsolateral prefrontal cortex lesions showed reduced MMN amplitude. Toyomaki et al. [58] suggested that the frontal component of MMN might be associated with a conscious perception of attention switching to stimulus change. In fact, they found a significant association with increased perseveration errors in the WCST.
These perseverative errors can be explained by a lack of attention switch and/or mental set shift and difficulty of response inhibition (registered by Stroop test). The authors suggested that the prefrontal cortex might work in connection with auditory areas in the temporal cortex, contributing to sensory-perceptual processes for MMN generation and further higher-cognition processes [58].
Under depression, participants completing an auditory task show significant differences in the amplitude of MMN at frontal and frontocentral electrodes, which can be related to cognitive deficits associated with preattentive states, complex cognitive-perceptual processes, and EFs [59–62]. However, further research is needed to understand the relationship between depression, EFs, and MMN.
Regarding the ability to detect negative feedback (NF) or error processing, conflict monitoring, information update, switching, and decision-making, another brain component has been extensively studied: ERN, discovered by Falkenstein et al. [63].
This component refers to a negative-going deflection at frontal and central electrodes that peaks at 100 ms of error commission in simple decision tasks [57, 63, 64]. It happens after responding, and it is frequently followed by a positive deflection, associated with the evaluation of the erroneous response [65].
Some studies have shown an effect of depression on ERN, registering more negative amplitudes in some cases, reduced amplitudes in other situations, or no differential effects between healthy and depressive participants [66, 67]. However, the role of ERN in depression still needs to be clarified.
Furthermore, there are experiments showing that amplitudes of the CRN (correct response negativity) and the ERN, summate with stimulus-locked event-related potentials (ERPs) as the P3, showing altered response-related ERPs in depression that are not present in controls [68].
Continuing with NF, specifically explored with the WCST, several studies have shown that the brain activation response is different at several stages of the experimental task.
Switch-NF requires more attention and inhibition than learn-NF, and it is associated with different evoked potentials (EPs) [69] and distinct errors (perseverative errors and non-perseverative errors), evoking distinct ERP patterns on the frontal regions and nonfrontal brain regions.
Perseverative errors or continued responding to a classification rule that is no longer useful, appear to be related to a disruption in the frontal-extrastriatal network, which is involved in the selection of visually relevant information. These errors are characterized by an absence of extrastriate N1 that temporarily precedes a significant reduction in P2 over the right frontal region.
On the other hand, the ERP patterns evoked by distractions or non-perseverative errors show a different activation. They occur in more central and frontal areas, and enhancements of P2 are registered during distractions. This fact can be associated with a loss of inhibitory control for incoming stimuli [33].
With the current experiment, we will contribute to the understanding of the underlying neural mechanisms associated with EFs under depression and we will measure the effects of exergames for improving recovery.
Materials
Experimental task to measure EFs: WCST
In this randomized controlled experiment, the effects of exergames on EFs are measured by using a classical experimental task: the WCST [70–72], which consists of classifying a card following changing rules according to three different criteria: matching the color, the shape, or the number of symbols.
Therefore, the screen shows a card; each card presents a different number of symbols (one, two, three, or four symbols) with different colors (red, blue, yellow, or green), and shapes (circle, cross, triangle, or star), but all the symbols in the card have the same shape and color, i.e., two green stars (Figure 1).
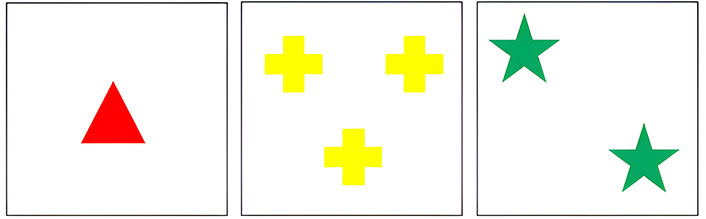
Three examples of principal cards from the Wisconsin Card Sorting Test (WCST). Principal cards appear individually in each trial
Then, the participant must choose from a group of four cards presented at the same time on the screen. The participant does not know the criteria to classify the principal card and must infer it by trying, receiving positive or NF, and adjusting the response. After several trials using the same classification rule, it can change without notice, and the participant again will readjust the response to determine the new criteria (Figure 2).
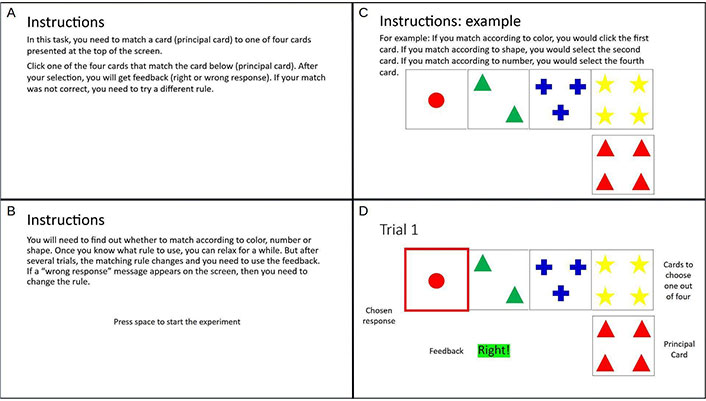
Example of the first trial in an experiment of Wisconsin Card Sorting Test (WCST). We can see the following elements: A, B: instructions; C: principal card and four cards to choose one out of four; D: a chosen response given by the participant, and the feedback
Outputs of the WCST
To perform this task, participants need to activate diverse domains and components of EFs. They must understand instructions, organize information, focus on the principal card, plan an action, choose the matching card, consider the feedback, infer the classification criteria, remember the rules, manipulate information, shift their mind when the rule changes, update, and inhibiting response to a previous rule. It is possible to check the enumeration and description of the outputs of the WCST in the current publication (Table 1) and the Supplementary material.
Outputs of the Wisconsin Card Sorting Test (WCST)
Outputs | Description |
---|---|
1 | Number of categories completed |
2 | Number of items or trials to complete the first category |
3 | Number of trials to complete the WCST |
4 | Total correct responses |
5 | Total errors or incorrect responses |
6 | Percentage of total errors |
7 | Total perseverative responses |
8 | Percentage of perseverative responses |
9 | Total perseverative errors |
10 | Percentage of perseverative errors |
11 | Total non-perseverative errors |
12 | Percentage of non-perseverative errors |
13 | The frequency of failure to maintain set or set-loss errors |
14 | Conceptual-level responses |
15 | Percentage of conceptual-level responses |
Data acquisition
As this experiment is part of a project that measures diverse cognitive functions, the order of presentation of the tasks, including the WCST, will be counterbalanced across subjects. Indeed, all the methodological designs of the outcome measures will be constructed using the rules of counterbalancing and stimulus rotation. In addition, response keys will be counterbalanced across conditions.
WCST will be programmed with E-Prime 2.0 (Psychology Software Tools Inc., Pittsburgh, PA, USA), and the statistical analyses of the behavioral and electrophysiological results will be conducted with different data-analysis software.
After screening, participants will be randomized into three groups. Then, pre-evaluation will take place, collecting data about the primary and secondary outcomes. Thereafter, the intervention will be developed over a period of eight weeks, with two sessions per week, for a total of 40–50 min per session.
After intervention, we will perform the post-evaluation. Three months after finishing the intervention, we will measure the effects of the follow-up through the final assessment.
Stimuli
We will use a computerized version of WCST that is a part of the stimulus presentation software of the NeuroScan NuAmps EEG amplifier and NeuroScan audio-video simulator (Stim II software): “CARDSORT”, a version based on Berg and Grant, 1948 [32]. The software allows to adapt the experiment by choosing the number of trials and the rule-change conditions. In this experiment, the rule is modified after a number of correct responses, and this number changes randomly between six and nine trials, avoiding the prediction of a new sorting category.
In each trial, a single choice card (principal card) will be presented in the bottom right corner of the screen, and the participant must match it to one of the four reference cards displayed in the upper part of the screen (Figure 2). After participant responds, written feedback (positive or negative), will be provided for correct or incorrect answers. The feedback appears on the left part of the screen, and the interval between feedback offset and the next WCST trial will vary randomly between 2 and 3 s. Then, a new trial will start.
Participants use a response panel with four buttons aligned. The far-left button corresponds to the reference card on the far left of the screen, the far-right button corresponds to the reference card on the far right of the screen. Subjects must use their thumbs to respond.
To ensure that participants understand the instructions, they will practice for approximately five minutes. Then, the formal experiment starts. It consists of eighteen series. As mentioned before, the series varies randomly between six and nine trials. The average duration of the task is around 20 min.
ERPs recording
Continuous electroencephalograph activity will be recorded while performing the WCST using a NuAmps amplifier (Neuroscan Inc.). Testing will take place in an electrically shielded, quiet, and dimly illuminated room. To record brain activity, we will use a 34-channel elasticized Quik-Cap with Ag/AgCl sintered electrodes (Neuroscan Inc.), positioned according to the extended 10–20 system [73], and referenced to linked mastoids (A1, A2). Participants will be grounded to the AFz electrode, and signals will be bandpass filtered (0.1–70 Hz), notch filtered (50 Hz), and amplified at a sampling rate of 250 Hz.
The electrooculogram (EOG) is recorded to correct the influence of ocular artifacts. Vertical and horizontal EOGs are recorded in two bipolar channels. Eye blinks and vertical eye movements are monitored via electrodes located below and above the supraorbital ridge of the left eye. Horizontal artifacts are monitored via electrodes on the outer canthus of each eye. The existence of blinks and other ocular movements will not be a criterion for epoch rejection. These artifacts will be eliminated using independent component analysis (ICA) decomposition [74–77].
After ICA decomposition, artifact components will be removed by inspection of their scalp topography and spectral power [78]. EOG correction and muscle or movement artifacts will be discarded. Those trials exceeding EEG amplitudes of ± 75 mV at any active electrode or response latencies longer than 4 s will also be discarded. Only artifact-free epochs will be selected for average.
After filtering, signals are extracted in epochs ranging from –200 to 1,000 ms after feedback onset. Then, the extracted epochs are averaged in each condition for analysis. Analysis is performed using the EEGLAB toolbox [79] and ERPLAB plugin for EEGLAB [80], both running under the MATLAB environment (The MathWorks Inc.).
Training intervention
The intervention group will train with an exergame. An adapted version of ExerCube by Sphery Ltd. [81] will be used. This program provides simultaneous physical and cognitive training through video games in an immersive gaming environment and allows the user to adapt to the intensity and physical demand from moderate to high intensity, which is especially important for people suffering from depression [82]. We will use a combination of training programs provided by ExerCube (Racer, Roboshop, Beat Squash, Fight the Dome, and ExergG).
The training regime consists of 40–50 min sessions, two sessions per week, for a total of eight weeks of individual training. We use a training regime that is not too long to avoid loss of motivation and attrition.
On the other hand, the active control group will train in similar conditions as the experimental group, but with an intervention program different from an exergame. In this case, participants will practice with adapted cognitive video games, designed to improve mental performance. Participants will play short brain games designed by Lumosity, a commercial platform (http://www.lumosity.com/).
The selection of games per day is random, and participants play individually for 40–50 min per session, two sessions per week for a total of eight weeks in a similar setting as the experimental group.
Finally, the passive control group consists of a wait-list group.
Procedure
Study design
The present experiment measures how exergames can have a positive effect specifically on EFs in people suffering from depression.
The experiment is a three-arm parallel-group randomized study. We compare three different interventions (training with an exergame, training with a cognitive game, and a wait-list condition) by randomizing participants to each group with equal probability. To randomly classify the participants into three groups, we use block randomization, where each participant is randomly assigned to one of the three equally sized blocks. These three blocks are created using the web applications available at http://random.org.
Moreover, this experiment is a double-blind design, where participants are blind to the intervention, and researchers are blind to intervention allocation. In addition, it is a longitudinal study with a pre-post intervention and 3-month follow-up. All procedures will be approved by a certified scientific board, and the participants will provide written informed consent to be considered for the experiment.
Eligibility and exclusion criteria
All the members in a population between 20 and 60 years old have the same chance to be selected for this study; participants will be volunteers, and will be recruited by online and media posting, local newspaper advertisements, flyers posted in campus buildings, and local businesses.
Sociodemographic variables depending on education, socioeconomic status, participant sex, work status, ethnicity, and age will be controlled. Prevalence studies have reported that women are frequently at a higher risk of depression than men, but recently, dominance analysis has shown that the variation in the prevalence of depression seems to be explained by similar factors in women and men [83]. To control these effects, we ensure the proportional gender ratio and the gender balance, and we will analyze the data in a gender-sensitive way.
Participants suffering from depression with comorbidities (bipolar disorder, obsessive-compulsive disorder, neurological disorder, or traumatic brain injury) will be excluded. Another exclusion criterion will be < 20/60 vision with or without correction, inability to complete the activities, or communication problems. The current use of any antidepressant medication did not function as an exclusionary criterion.
The inclusion and exclusion criteria for persons suffering from depression will be validated by a professional through an interview and the use of reliable and valid psychological assessment tools.
Beck Depression Inventory-Second Edition
We will use the Beck Depression Inventory-Second Edition (BDI-II) [84] to measure the severity of depression. It consists of twenty-one items. Each item is scored on a Likert-type scale ranging from 0 to 3 (e.g., “0 = I do not feel sad, 1 = I feel sad, 2 = I am sad all the time and I can’t snap out of it, and 3 = I am so sad and unhappy that I can’t stand it.”). The score considered for inclusion criteria was depression (≥ 28 points on BDI-II).
The test has been adapted to Spain (BDI-IA [85]; BDI-II [86]). The adaptation of the BDI-II has shown high internal consistency (coefficient alpha of 0.89) [86].
Sample size
To calculate the value of a sufficient sample size, we have conducted a priori power analysis using the software G-Power 3.1.9.7 [87, 88]. The power of a test estimates the probability of correctly rejecting the null hypothesis when it is false. We calculated the sample size using a model for differences between three groups based on analysis of variance (ANOVA), using an alpha of 0.05, a power of 0.80, and a large effect size (f = 0.40) [89, 90]. The result obtained was a total sample size of at least 144 subjects for ten primary outcomes and three secondary outcomes, or approximately fifty participants per group (Figure 3).
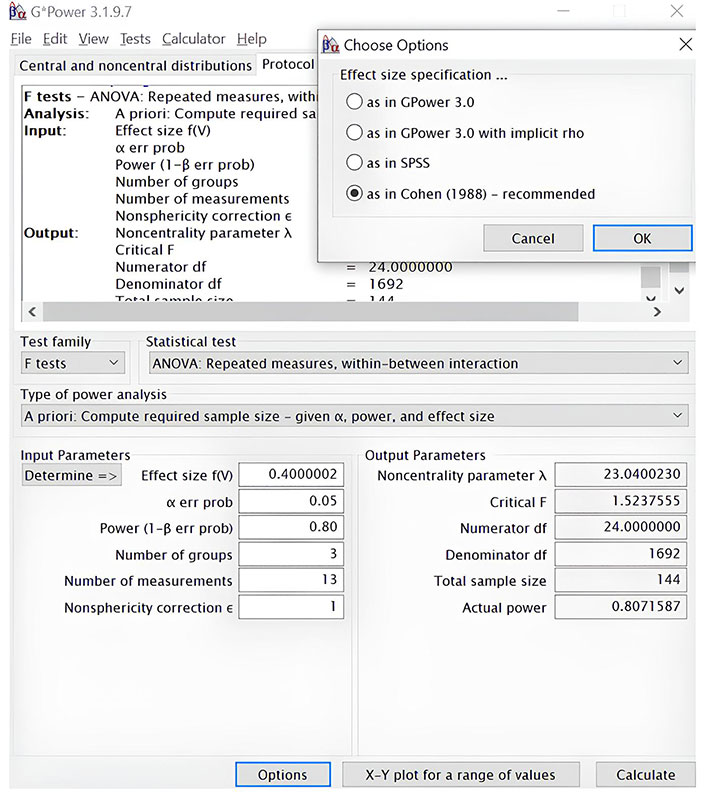
Estimation of sample size with a priori power analysis using the software G-Power 3.1.9.7. ANOVA, using an alpha of 0.05, a power of 0.80, and a large effect size (f = 0.40). ANOVA: analysis of variance
We also plan to perform analysis of covariance (ANCOVA) to compare post-test scores across conditions while controlling for pre-test scores, so we calculated the sample size for the three groups by conducting a priori power analysis (compute needed sample size, given alpha = 0.05, power = 0.80, and effect size f = 0.40), using ANCOVA fixed effects, main effects, and interactions as statistical tests. In this case, we obtained a sample size of sixty-five participants (Figure 4).
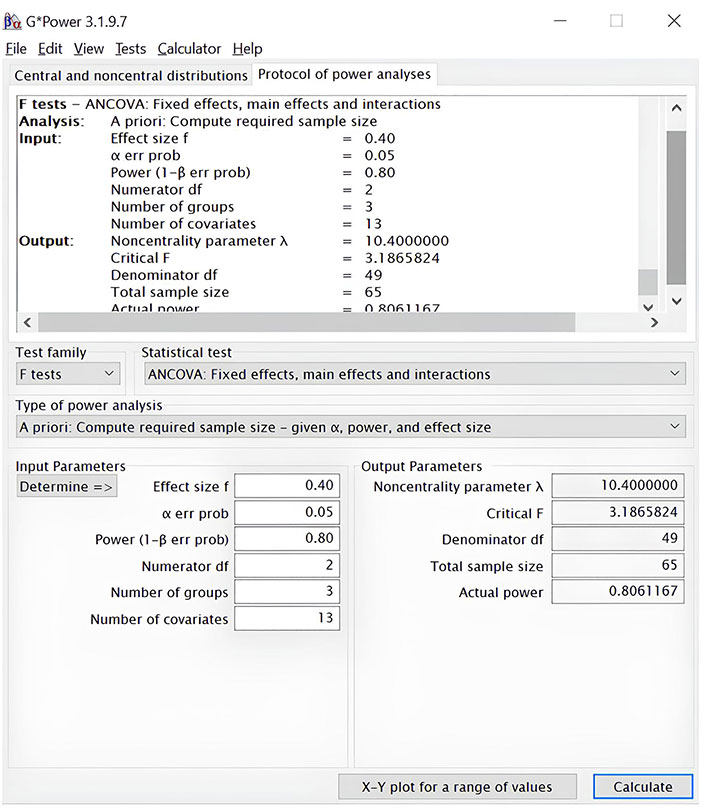
Estimation of sample size with a priori power analysis using the software G-Power 3.1.9.7. Analysis of covariance (ANCOVA), using an alpha of 0.05, a power of 0,80, and a large effect (f = 0.40)
In conclusion, a total sample size between 75 and 150 subjects, or between 50 and 60 participants per group, is required to have 80% power to show the cognitive benefits of exergames over placebo conditions in terms of EFs measured through the WCST.
Primary outcomes and analysis
Effects of exergame training on EFs: behavioral analysis
With the WCST, we collect information about the outputs previously enumerated and described (Table 1, Supplementary material), where there are ten primary measures associated with EFs. These measures are collected using the WCST at three different moments: before the intervention, after the intervention, and three months after the intervention.
We want to assess whether there is a difference in performance after the intervention, and 3 months later, for each group and primary outcome [in terms of correct responses, errors, and reaction times (RTs)].
Moreover, we want to analyze whether the results of each group in the pre-assessment could be related to further results.
Finally, we will measure the differences among groups, comparing the experimental group’s performance and the control groups’ performance before, after the training, and 3 months after finishing the intervention.
To do so, mixed ANOVA with 3 groups (experimental, active control, and passive control) as a between-subjects factor and 3 assessment sessions (before training, after training, and 3-month follow-up) as the within-subjects factor, will be performed for each primary outcome to check whether there is a significant difference between the groups and sessions for each WCST measure.
The effect size is calculated using the partial eta squared (ηp2) to solve the problem related to population variance overestimation, where a value less than 0.01 will be considered trivial, a value of 0.01 will be considered small, a value equal to 0.06 will represent a medium effect and a ηp2 of 0.14 will be considered a large effect. We use post hoc pairwise comparisons to analyze where the differences occur. In all analyses, we will use an alpha of 0.05, and all statistical tests will be Bonferroni corrected for multiple comparisons.
In randomized studies, ANCOVA is considered a better suited method than change from baseline to enhance the statistical power when the groups have equivalent designs [91].
Thus, we firstly perform ANOVAs to analyze the main effects and interactions between groups and within sessions, and later, if the required assumptions for ANCOVAs are met [the assumption of no relationship between the intersubject factor (group), and the covariates (p > 0.05), and the equality of slopes (p > 0.05)], ANCOVAs will be used to analyze the differences between groups in all the outcomes, using as covariates the pretest scores for those measures.
Finally, it is important to mention that outliers will be reported but not included in the analyses, avoiding the influence of these measures in the main effects and interactions. In some cases, we plan to assess outliers by searching for confidence intervals (CIs) that fell outside the limits of the effect size with 95% CIs. In other situations, we will explore the data-removing trials with a cutoff of 2.5 standard deviations (SDs) from the mean [92].
Statistical analysis of electrophysiological data
To explore the effects of exergames on EFs under depression in terms of brain activity, we will perform several statistical analyses with the brain data collected while performing the WCST.
We will perform mixed ANOVAs to compare the latency and amplitude of the different brain components associated with executive deficits under depression: P3, P3a, P3b, P2, N1, MMN, ERN, CRN, switch-NF, and learn-NF; at different brain areas: frontal and prefrontal regions, central, parietal, posterior, temporal areas, and hemisphere (left, middle, and right), for each group (experimental group, active control group, and passive control group); and at three different moments: preintervention, postintervention, and three-month follow-up.
After that, we will compare the differences in brain activity between the three groups at pre-test and post-test. Finally, we will analyze the brain activity by comparing the three groups at the three given time points.
To control the signal-to-noise ratio, for RTs, we will adapt to the task an interval window of 200 ms and 1,500 ms to exclude outliers. RTs faster than 200 ms and slower than 1,500 ms could be related to distraction according to previous research. Therefore, they will be excluded from the RT analysis. Moreover, we plan to control the outliers by searching for CIs that fell outside the limits of the effect size with 95% CIs or exploring the data and removing trials with a cutoff of 2.5% SD from the mean [92].
Significant effects will be set at p < 0.05, and we will use post hoc pairwise comparisons to analyze where the differences occur, where all statistical tests will be Bonferroni corrected for multiple comparisons. In addition, the effect size will be calculated using the ηp2 to solve the problem related to population variance overestimation, where a value less than 0.01 will be considered trivial, a value of 0.01 will be considered small, a value equal to 0.06 will represent a medium effect, and a ηp2 of 0.14 will be considered a large effect.
Finally, to analyze relationships between brain components and EFs (behavioral results), Pearson correlation coefficients will be calculated, using the mean amplitude (locations depending on the brain component) and peak latency (location depending on the brain component) of the given brain component from difference waves.
Secondary outcomes and analysis
The control of placebo effect depending on the intervention’s expectations, motivation, and engagement is an important issue in cognitive training [93–95]. Therefore, to explore whether these variables account for possible training-related improvements, participants will report their expectations before and after evaluation using a 5-point Likert-type scale (1 = the results will be much worse, 3 = there will not be any change, 5 = the results will be much better). Moreover, they will provide information about their motivation and engagement during training using a similar 5-point Likert-type scale (1 = not motivated to 5 = extremely motivated, 1 = not engaged to 5 = extremely immersed).
The reliability of the expectations scale has been previously calculated by combining the items in a global index and computing Cronbach’s alpha. The results showed a reliability of 0.64 for expectations [96]. In addition, some researchers have adapted similar questionnaires [93] producing the expectation assessment scale and analyzing the psychometric properties [97]. They obtained an internal consistency of 0.87.
Regarding the motivation and engagement scales, we will use questionnaires based on other studies that did not report validity coefficients [92, 96, 98].
Regarding the data analysis, mixed measures ANOVAs with three groups (experimental group, active control group, and passive control group) × 2 sessions (pre-post) will be conducted separately for motivation, engagement, and expectations. Post hoc pairwise comparisons will account for the differences. In all analyses, we will use an alpha of 0.05, and all statistical tests will be Bonferroni corrected for multiple comparisons. In addition, the effect size will be calculated using the ηp2 to solve the problem related to population variance overestimation, where a value less than 0.01 will be considered trivial, a value of 0.01 will be considered small, a value equal to 0.06 will represent a medium effect, and a ηp2 of 0.14 will be considered a large effect.
Expected results
This section should provide the expected results of performing an experiment following the protocol. This section can be divided into subheadings. Tables, figures, photographs, and other appropriate forms can be used and numbered in order. Depression is characterized by emotional, thinking, affective, social, and cognitive changes. In clinical settings, cognitive deficits are usually unexplored, having an impact on recovery and recurrence of depression.
The present work consists of a randomized clinical trial to assess the benefits of an exergame-based intervention which simultaneously stimulates cognitive and psychomotor functions.
It represents a novel approach to treatment of depression and implements the methodological limitations of previous research by incorporating adequate control groups, measuring placebo effect depending on expectations, motivation, and engagement, and analyzing brain mechanisms associated with cognitive changes.
The study consists of a longitudinal, double-blind, and three-arm parallel-group randomized experiment, where we compare three different interventions (training with an exergame, training with a cognitive game, and a wait-list condition).
The experimental group trains with an adapted version of ExerCube by Sphery Ltd. [81], which provides simultaneous physical and cognitive training and allows the user to adapt the intensity and physical demand from moderate to high intensity, which is especially important for people suffering from depression [82].
The training regime consists of 40–50 min sessions, two sessions per week, for a total of eight weeks of individual training.
In addition, the active control group will train in similar conditions to the experimental group, playing short brain games designed by Lumosity, a commercial platform (http://www.lumosity.com/). And the passive control group will be a wait-list group.
To our knowledge, previous research assessed whether video games have a global positive effect on depression, but there are no studies analyzing specific contributions of video games to cognitive domains altered in depression with a special concern about the quality of the methodology used.
Besides, we collect brain activity to analyze the neuronal substrate of the cognitive and behavioral changes after the different interventions, which have not been done before.
Another strength of this study is not only to evaluate the immediate effects of the distinct interventions, but also the effect of time on the learning process, establishing a follow-up assessment after three months of finishing the interventions.
Once analyzing and comparing the WCST performance of the three groups at three time points in terms of cognitive-behavioral responses and brain activity, and controlling for placebo effect dependent on expectations, engagement, and motivation, we expect the experimental group benefits deferentially in terms of EFs.
Behavioral improvements are expected concerning the outputs of WCST related to EFs. We expect an increase in total correct responses and categories completed after training, and a decrease in perseverative answers and errors. Besides, we hope that the benefits endure after intervention, showing maintenance during the follow-up assessment.
These results will show how the training could have a positive impact on a variety of domains of EFs (i.e., switching, updating information, inhibition, flexibility, and cognitive control).
Regarding neural activity, we also expect to register benefits derived from exergames in brain components associated with EFs.
First, as depression is characterized by decreased P3 amplitudes and prolonged P3 latency, which explains difficulties in set-shifting, memory update, executive attention, and working memory operations, it is expected that training intervention could optimize neuro-cognitive processing, having a significant effect on P3 and registering higher amplitudes and shorter latency.
It is also expected an increment of N100 amplitudes and a decrement of latency, as result of a more efficient discrimination processing of information after exergames intervention, which will directly affect the ability to update information and adjust correctly the response.
Moreover, we plan to explore the relationship between N1 and P2 to understand whether an increase in N1 amplitudes could be related to an enhancement in P2 amplitudes over frontal and central regions, diminishing errors of discrimination and palliating deficits in inhibitory control.
Furthermore, it will be important to explore MMN and voluntary effort, associated with cognitive switching, cognitive control, or response inhibition. The frontal component of MMN seems to be related to an increase in the number of perseverative errors in the WCST. Depression processing shows significant differences in the amplitude of MMN at frontal and frontocentral electrodes, and we expect this brain activity could also be implemented after training.
Lastly, we expect to register improvements to detect negative and positive feedback, (ERN and CRN), and to make the appropriate decision to adjust the response for implementing outcomes.
Conclusively, by exploring the effects of exergames on cognition in participants with depression through a well-structured randomized study, we will understand the mechanisms that allow for integral recovery and remission, which may improve treatment for depression.
Abbreviations
ANCOVA: | analysis of covariance |
ANOVA: | analysis of variance |
BDI-II: | Beck Depression Inventory-Second Edition |
CIs: | confidence intervals |
CRN: | correct response negativity |
EEG: | electroencephalographic |
EFs: | executive functions |
EOG: | electrooculogram |
ERN: | error-related negativity |
ERPs: | event-related potentials |
ICA: | independent component analysis |
MMN: | mismatch negativity |
NF: | negative feedback |
RTs: | reaction times |
SDs: | standard deviations |
WCST: | Wisconsin Card Sorting Test |
ηp2: | partial eta squared |
Supplementary materials
The supplementary materials for this article are available at: https://www.explorationpub.com/uploads/Article/file/100668_sup_1.pdf.
Declarations
Author contributions
ERM: Conceptualization, Investigation, Methodology, Writing—original draft, Project administration, Visualization, Funding acquisition, Writing—review & editing.
Conflicts of interest
The author declares that do not have conflicts of interest.
Ethical approval
The present experiment and the whole project will be conducted in accordance with the Declaration of Helsinki. It will be presented to the Ethics Committee of the Centre designated for the development of the project for protocol approval, and all participants will sign a written informed consent and will be informed of their right to cease participation in the study at any time.
Consent to participate
Informed consent to participate in the study will be obtained from all participants.
Consent to publication
Informed consent to publication will be obtained from participants.
Availability of data and materials
The data that support the findings of this study are openly available in the Open Science Framework (https://osf.io/YBDCT/). This work is available as preprint at Open Science Framework (Preprint DOI).
Funding
This work was developed independently, with the support of MAP Institute Centre of Psychology. The funder had no role in study design, data collection and analysis, decision to publish, or preparation of the manuscript.
Copyright
© The Author(s) 2024.