Abstract
Aim:
This study aimed to investigate the relationship between heart rate variability (HRV) parameters and performance in soccer players.
Methods:
This study used a cross-sectional design to assess HRV parameters in a cohort of twenty-nine male athletes, aged 18 to 20 years, randomly selected from the Macapá Sports Club team in the Amazon region. Resting HRV data for ten minutes while maintaining normal breathing, acquired with a Polar V800 heart rate monitor recording at a sampling rate of 1,000 Hz, were analyzed using Kubios HRV software to extract time domain: mean of the normal sinus intervals (MRR), the standard deviation of normal sinus (NN) intervals (SDNN), root mean square of successive differences (RMSSD), the percentage of times that the change in consecutive normal sinus intervals exceeded 50 ms (pNN50), and frequency domain: low frequency (LF), high frequency (HF), and LF/HF ratio parameters. Factor analysis was then performed using principal component (PC) extraction and varimax rotation. The logarithmic transformation [normalized LF/HF by logarithmic transformation (LF/HFNormlog)] was applied to address this non-normality before factor analysis.
Results:
The first two PCs showed that 87.4% of the total variance was explained by the original variables. The LF (–0.93), HF (0.93), and LF/HFNormlog (–0.92) parameters contributed significantly to PC1, also known as the frequency domain component. In contrast, the MRR (0.60), SDNN (0.91), RMSSD (0.89), and pNN50 (0.79) parameters contributed to PC2, also known as the time domain component.
Conclusions:
This study provides valuable evidence of the complex relationship between autonomic factors affecting HRV parameters in soccer players. Identifying two distinct PCs related to sympathetic and parasympathetic activity highlights the importance of monitoring HRV to optimize performance and recovery. Machine learning is important to monitor these changes in the possible molecular mechanisms controlling HRV in soccer players.
Keywords
Heart rate variability, autonomic nervous system, soccer, performance, factor analysisIntroduction
Cardiology studies the heart and circulatory system, including the diagnosis, treatment, and prevention of cardiovascular disease [1–3]. In particular, cardiovascular exercise physiology complements cardiology by studying the effects of exercise on heart rate and oxygen consumption [4, 5].
Heart rate variability (HRV) is a non-invasive physiological measure that reflects the oscillations between consecutive heartbeats, and R-R interval waves of the QRS complex on an electrocardiogram (ECG), as a simple measure of autonomic impulses [6]. HRV is one of the most promising quantitative markers of the sympathetic-vagal balance of the autonomic nervous system (ANS) [7] for health, high HRV generally signifies a dominant parasympathetic response [8], while low HRV suggests a heightened sympathetic response, linked to disease [9, 10].
In sports, especially in soccer, the relationship between HRV and athletic performance has aroused growing interest among researchers and professionals in the field, as HRV reflects the heart’s ability to adapt to the physiological demands imposed during sports practice, making it a valuable tool for monitoring the response of cardiac impulses to physical stress [11, 12].
In this context, the use of advanced technologies such as heart rate monitors in the acquisition of the R-R interval for the measurement of HRV in real-time, for example, Polar® heart rate monitor showed the reliability of the mean R-R interval compared to a resting ECG [13]. Incorporating HRV monitoring into training programs can lead to improved performance and fitness [11, 14, 15], reduced injury risk and overtraining [16, 17], and enhanced mental resilience [18–20], ultimately contributing to the success of individual players and the team as a whole [21].
Artificial intelligence is transforming the game of soccer through applications that improve player performance [22], injury prevention [23], tactical strategy [24], and sports medicine [25]. Specifically, machine learning techniques using factor analysis and unsupervised multivariate statistics are used to uncover underlying relationships between observed variables for dimensionality reduction and feature extraction of the data, thereby facilitating interpretation and analysis [26]. In the context of soccer analysis, this technique can be used to identify latent factors contributing to autonomic HRV data.
However, until the present study, it has not been investigated which HRV parameters are associated with soccer players using the factor analysis after a biomedical literature review from Medline PubMed, which makes this study justified. This study aims to elucidate the complex interplay of factors that contribute to autonomic factors affecting HRV parameters in soccer players.
Materials and methods
Subjects
The study protocol was ethically approved by the Human Research Ethics Committee of the Federal University of Amapá (CAAE: 50150121.1.0000.0003, n° 5.121.013). Written informed consent was obtained from all participants. This study complied with the ethical guidelines of the Declaration of Helsinki [27] and Resolution 510/2016 of the National Health Council.
This was a cross-sectional study of twenty-nine male athletes (18.6 ± 0.78 years old, 68.2 ± 6.8 kg, and 174.0 ± 7.7 cm) from the Macapá Sports Club team in the city of Macapá, in the state of Amapá, located in the northwestern region of the North Region of Brazil, also known as the Amazon Region. Exclusion criteria were that the volunteers could not be younger or older than the proposed age, could not have performed strenuous exercise in the 48 hours before the test, could not have consumed caffeine-containing compounds, could not have eaten in the 2 hours before the test, could not have used ergogenic aids or anabolic steroids, and could not have a history of cardiovascular disease.
Anthropometric measurements
Prior to study participation, all potential volunteers underwent a comprehensive orientation. Participants were given detailed information about the test procedures, including the estimated time required. They were instructed to remove their shoes, wear light clothing, and not carry any objects. Height was measured in centimeters and weight in kilograms using certified and calibrated mechanical scales manufactured by Filizola (Brazil). To ensure consistency and data reliability, all anthropometric measurements were performed by the same experienced evaluator throughout the study.
Heart rate variability analysis
Subjects were instructed to sit quietly in the supine position for 10 minutes at rest with spontaneous breathing, and the first five minutes of signal acquisition were discarded. A Polar V800 heart rate monitor (Polar, Finland) was used to acquire the R-R signal, positioned on the xiphoid process of the sternum, with a sampling frequency of 1,000 Hz to record the R-R intervals. The R-R interval tacho-grams were transferred via a Bluetooth interface device to Polar FlowSync software (Polar, Finland), which automatically corrects the signals based on the averaging filter and stored in ‘.txt’ files.
After signal filtering, the HRV of the last 5 minutes of the R-R interval signal was analyzed and first estimated using the Kubios HRV Standard software (version 3.5.0) to obtain the classical time domain parameters: (a) MRR—the mean of the normal sinus (NN) intervals; (b) SDNN—the standard deviation (SD) of all NN intervals; (c) RMSSD—the root mean square of successive differences between adjacent NN intervals; and (d) pNN50—the percentage of times that the change in consecutive NN intervals exceeded 50 ms. Spectral analysis was performed using the Welch periodogram method, 256 points segments with 128 points overlapping using the Hanning window, to obtain the normalized spectral indices: low frequency (LF) 0.04–0.15 Hz, high frequency (HF) 0.15–0.40 Hz, and LF/HF ratio. All parameters were calculated according to the recommendations of the Task Force of the European Society of Cardiology and the North American Electrophysiological Society [28].
Data analysis
Factor analysis is a statistical technique used to reduce a large set of variables into a smaller number of underlying factors or components. These factors represent the shared variance among the original variables, effectively simplifying complex data. This process involves analyzing the covariance or correlation matrix is decomposed into eigenvalues and eigenvectors or latent factors [26].
The eigenvalues indicate the importance of each factor in the amount of variance explained, while the eigenvectors, through their elements, the factor loadings, show how the original variables relate to the factors. The strength of these relationships is represented by factor loadings, which act as regression coefficients between the observed variables and the common factors. To simplify the interpretation of factors, a process called factor rotation is often applied, where each factor primarily influences only a few observed variables [29]. The eigenvectors corresponding to these selected eigenvalues define the retained latent factors.
Statistical analysis
Descriptive statistics were used to summarize the data, expressed as mean ± SD or standard error. The Shapiro-Wilk test was used to assess whether the HRV parameters were from a normally distributed population. The post hoc power (1-beta error level) sample analysis determined the effect size [30] by G*Power software version 3.1.9.2 (University Kiel, Germany).
Factor analysis was then performed using eigenvalue decomposition of the covariance matrix data [29]. Pearson’s correlation plays an essential role in factor analysis by providing information about the relationships between variables, guiding factor extraction, and helping to interpret the final factor structure. Bartlett’s sphericity test and Kaiser-Meyer-Olkin were used to assess the factorability of the data and the appropriateness of the sampling. Principal component analysis (PCA) was used for extraction [10], followed by varimax rotation [31]. The PCs were ranked according to their eigenvalues, with the highest eigenvalue representing the first PC, and so on. Relevant PCs for analysis were determined using a combination of broken stick test criteria, scree plot visualization, and eigenvalues greater than 1 [32]. All procedures were performed using Matlab 2020.b (Mathworks, USA) with a significance level of P < 0.05.
Results
Table 1 shows the HRV parameters of the participants. The low SD values indicate a homogeneous sample, the P-values for each variable confirmed that the data showed a normal distribution. The sample size effect was considered large with an actual power of 0.99. The LF/HF parameter of the HRV showed non-linearity for the data distribution. To apply factor analysis, LF/HF parameter was normalized by logarithmic transformation (LF/HFNormlog).
The heart rate variability parameters of the participants
Variables | Mean ± SD | 95% CI | P-value |
---|---|---|---|
MRR | 911.3 ± 109.0 | 869.8–952.8 | 0.206 |
SDNN | 60.6 ± 25.7 | 50.8–70.4 | 0.665 |
RMSSD | 60.6 ± 25.7 | 50.8–70.4 | 0.643 |
pNN50 | 30.6 ± 19.8 | 23.0–38.1 | 0.197 |
LF | 56.5 ± 18.2 | 49.5–63.4 | 0.271 |
HF | 43.7 ± 18.6 | 36.6–50.8 | 0.159 |
LF/HF | 1.98 ± 1.95 | 1.23–2.72 | < 0.001 |
Where MRR is the mean of the normal sinus (NN) intervals; SDNN is the standard deviation (SD) of all NN intervals; RMSSD is the root mean square of the successive differences between adjacent NN intervals; pNN50 is the percentage of times that the change in consecutive NN sinus intervals exceeded 50 ms; LF is low frequency; HF is high frequency. Values are mean ± SD, 95% CI is the confidence interval around 95% of the mean and the P-value of Shapiro-Wilk test
Table 2 shows the correlation matrix between HRV parameters (P < 0.01). The SDNN, RMSSD, pNN50, and HF are highly correlated with values above 0.80. The LF is strongly negatively correlated with SDNN, RMSSD, pNN50, and HF (–0.55 to –0.99).
Correlation matrix of heart rate variability parameters
Variables | MRR | SDNN | RMSSD | pNN50 | LF | HF | LF/HFNormlog |
---|---|---|---|---|---|---|---|
MRR | 1.00 | 0.41 | 0.51 | 0.56 | –0.43 | 0.43 | –0.46 |
SDNN | 0.41 | 1.00 | 0.96 | 0.78 | –0.55 | 0.55 | –0.55 |
RMSSD | 0.51 | 0.96 | 1.00 | 0.87 | –0.67 | 0.67 | –0.68 |
pNN50 | 0.56 | 0.78 | 0.87 | 1.00 | –0.73 | 0.71 | –0.73 |
LF | –0.43 | –0.55 | –0.67 | –0.73 | 1.00 | –0.99 | 0.99 |
HF | 0.43 | 0.55 | 0.67 | 0.71 | –0.99 | 1.00 | –0.98 |
LF/HFNormlog | –0.46 | –0.55 | –0.68 | –0.73 | 0.99 | –0.98 | 1.00 |
Values are Pearson’s correlation. Strong positive correlations and strong negative correlations (above 0.8) are shown in dark black. MRR is the mean of the normal sinus (NN) intervals; SDNN is the standard deviation (SD) of all NN intervals; RMSSD is the root mean square of the successive differences between adjacent NN intervals; pNN50 is the percentage of times that the change in consecutive NN sinus intervals exceeded 50 ms; LF is low frequency; HF is high frequency, and LF/HFNormlog is normalized LF/HF by logarithmic transformation
Bartlett’s sphericity tests indicated that the dataset was suitable for factor analysis (P < 0.001) and sampling adequacy (0.81). The Broken Stick test revealed that two PCs were most representative of the eigenvalues based on the inflection point, as depicted in Figure 1A. Additionally, the eigenvalues were greater than 1, with 87.4% of the total variance explained by the original variables (Figure 1B).
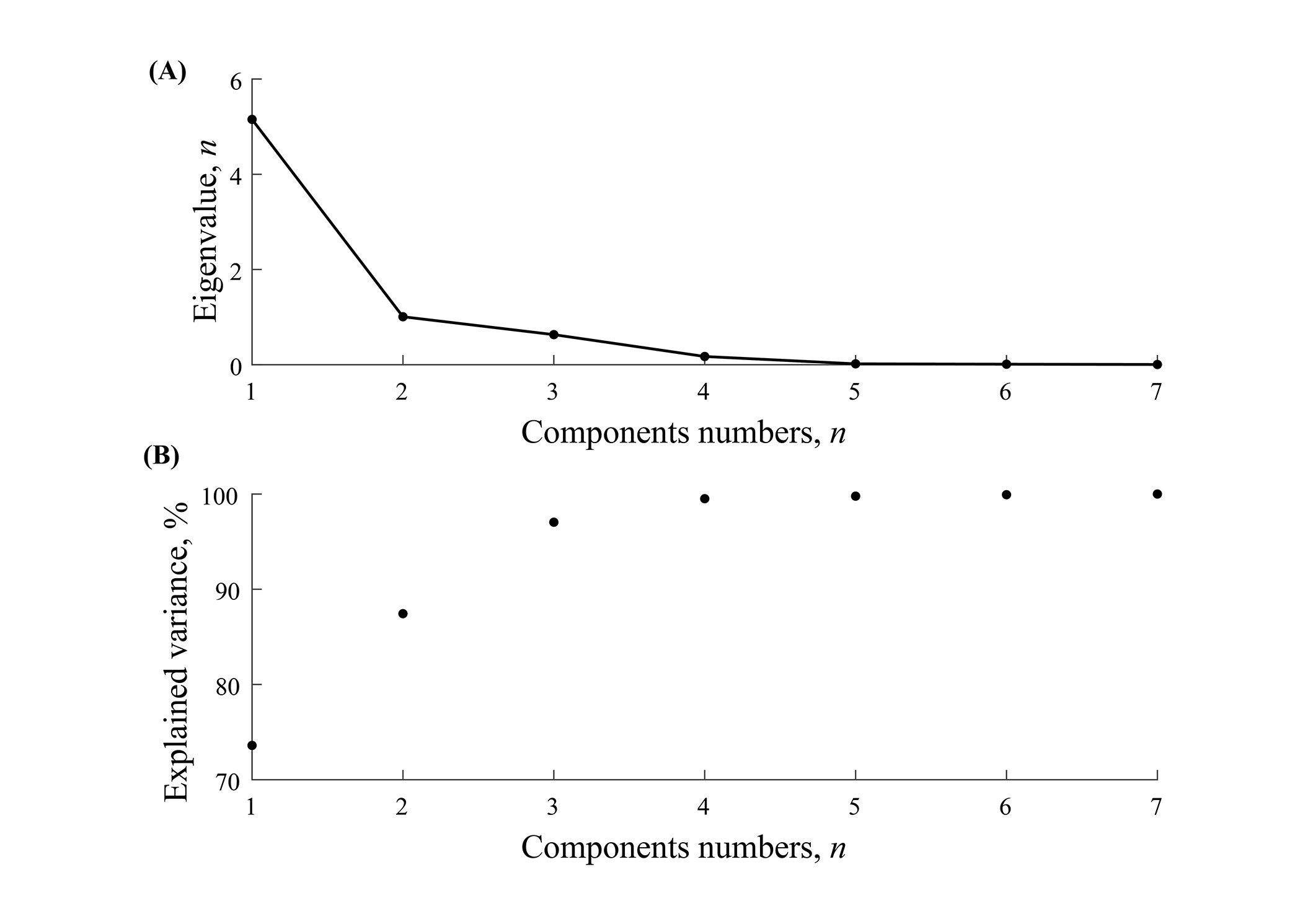
Scree plot. (A) Principal components applied in the eigenvalue; (B) principal components applied in an explained variance of the eigenvalue. The elbow in a scree plot, which in this case suggests the retention of two components, represents a point of diminishing returns in explained variance
Table 3 shows the weighting coefficients for the two components after the varimax rotation of the original variables. It is clear that LF, HF, and LF/HFNormlog contributed to the first PC1. While the MRR, SDNN, RMSSD, and pNN50 parameters contributed to the second PC2.
Weighting coefficients of the original variables
Variables | PC1 | PC2 |
---|---|---|
MRR | 0.25 | 0.60 |
SDNN | 0.23 | 0.91 |
RMSSD | 0.37 | 0.89 |
pNN50 | 0.48 | 0.79 |
LF | –0.93 | –0.35 |
HF | 0.93 | 0.35 |
LF/HFNormlog | –0.92 | –0.36 |
Where MRR is the mean of the normal sinus (NN) intervals; SDNN is the standard deviation of all NN intervals; RMSSD is the root mean square of the successive differences between adjacent NN intervals; pNN50 is the percentage of times that the change in consecutive normal sinus intervals exceeded 50 ms; LF is low frequency; HF is high frequency, and LF/HFNormlog is normalized LF/HF by logarithmic transformation. The scores are latent factors or eigenvectors of factor analysis
Discussion
This study aimed to identify patterns and relationships between HRV parameters in soccer players using factor analysis. The results indicated that LF, HF, and LF/HFNormlog were associated with PC1, while MRR, SDNN, RMSSD, and pNN50 were associated with PC2. These findings are consistent with current knowledge of the autonomic regulation of heart rate in soccer players.
The ANS plays a crucial role in the regulation of heart rate, particularly in the context of physical activities such as soccer. Both branches of the ANS sympathetic and parasympathetic exert opposing effects on cardiac function and influence heart rate during different exercise intensities in soccer players [11, 12, 15]. The cardiovascular regulatory centers in the spinal cord and medulla integrate inputs from higher brain centers with afferent inputs from the cardiovascular system to adjust heart rate via sympathetic modulation involving intracellular cyclic adenosine monophosphate (cAMP) fluctuations, while parasympathetic effects are mediated by M2 muscarinic receptors, allowing for rapid adjustments [33]. The molecular interplay of the neurotransmitters norepinephrine and acetylcholine at the sinoatrial node determines the balance between sympathetic and parasympathetic activity [33], thereby influencing HRV patterns.
HRV is measured by analyzing the intervals between R-waves in an ECG, which are the peaks of the electrical signal representing a heartbeat [6]. The physiological mechanisms underlying HRV, particularly at the molecular level, are poorly understood, but understanding these mechanisms provides insights into how HRV parameters can serve as biomarkers of athlete performance in match situations, monitoring training load and fatigue [33–36], recovery [37, 38], mental well-being [39–41], and potentially optimizing recovery strategies in soccer players [42, 43].
Previous studies have shown that specific HRV parameters are significantly correlated with performance outcomes, providing insight into the physiological readiness of the athlete [4, 11, 12, 15, 44–47]. This association has been attributed to changes in the intrinsic mechanisms of the sinus node, the heart’s natural pacemaker, and changes in the ANS control of the heart with increased vagal activity, reflecting better adaptability and functional readiness for competition [48]. These studies support the results of the present study, as high-frequency variability (SDNN, RMSSD, pNN50, HF) is highly correlated, while sympathetic activity (LF and LF/HFNormlog) shows a negative correlation with high-frequency variability.
Recent research has shown that individuals with higher levels of MRR interval and pNN50 HRV parameters tend to have better aerobic fitness [4], suggesting that higher RMSSD values indicate better performance in professional footballers [44], parameters such as SDNN and RMSSD are critical in differentiating performance levels between athletes, with endurance athletes having distinct HRV profiles compared to strength and speed athletes [45], and the log-transformed root mean square of the successive differences between adjacent NN intervals (lnRMSSD) is strongly correlated with improvements in aerobic fitness [46]. In agreement, the study resulted in a high contribution of the weighting coefficient of MRR (0.60), SDNN (0.91), RMSSD (0.89), and pNN50 (0.79) in PC2, suggesting the time domain component.
The functions of the sympathetic and parasympathetic nervous systems have a significant impact on athletic performance and recovery strategies. The balance between these two systems is crucial for optimizing performance during high-intensity activity and facilitating recovery afterward [48, 49]. This interplay can be observed through various physiological responses that are important for athletes to understand and manage, as overactivation of the sympathetic nervous system can lead to symptoms of overtraining [50], and effective parasympathetic activity correlates with better recovery metrics [51]. These studies support the results of the present study as LF (–0.93), HF (0.93), and LF/HFNormlog (–0.92) significantly contributed to PC1, also known as the frequency domain component. On the other hand, a higher score on PC2 or parasympathetic dominance is crucial for recovery strategies [16, 39].
The LF/HF parameter of HRV is often analyzed through both linear and non-linear methods, revealing complex dynamics in autonomic regulation. During physical exercise, there may be non-linear interactions, such as context-dependent synergies or antagonisms, which affect cardiac activity [52], in agreement with the results of our study.
In addition, SDNN, RMSSD, pNN50, and HF are highly correlated, suggesting that these parameters represent similar aspects of HRV, particularly high-frequency variability. LF is highly negatively correlated, suggesting that sympathetic activity is associated with lower HRV, whereas parasympathetic activity, represented by HF, is associated with higher variability. While these HRV parameters are indicative of performance, it is important to consider that individual responses to exercise loads may vary and factors such as body composition [53], psychological [19], age [54], and respiratory frequency [55] may also influence HRV results.
Nowadays, machine learning has emerged as a promising tool for diagnosis, treatment, and management in the biomedical field [9, 55, 56]. None of these studies [4, 11, 12, 15, 44–47] used factor analysis to describe the HRV parameters associated with player soccer, showing a strong influence in the first two PCs after varimax rotation. HRV, combined with unsupervised machine learning, provides a powerful approach to understanding and optimizing the performance of soccer players. As technology continues to advance, the integration of HRV and machine learning is poised to revolutionize the way soccer is played and coached.
The use of a single team from the Macapá Sports Club in the Amazon region limits the generalizability of these findings to other soccer teams and populations. Future studies should use a control group, longitudinal study designs, larger sample sizes, and more diverse samples, including teams from different leagues, playing styles, and geographical locations. Besides, the factor analysis results would be strengthened by sensitivity analyses, using different correlation methods or exploring the impact of potential outliers. This would allow for a more robust assessment of the relationship between HRV parameters and performance or recovery across a wider range of soccer players.
Incorporate measures of training load, sleep quality, diet, and other relevant factors to account for their potential influence on HRV and to better isolate the effects of ANS activity. Investigating the underlying molecular mechanisms that regulate HRV in athletes is crucial for a deeper understanding of its physiological significance. This could include exploring the role of specific genes, hormones, and neurotransmitters in modulating ANS activity and its impact on athletic performance.
Finally, a more complete understanding of the role of HRV in athletic performance (e.g., sprint speed, endurance capacity, agility tests, and maximum oxygen uptake) and recovery indicators (e.g., heart rate recovery, perceived exertion, creatine kinase levels, and blood lactate) is needed to establish a stronger correlation between the identified HRV components (PC1 and PC2) and athletic outcomes. This could include the use of predictive modeling techniques to link the PCs to these performance and recovery measures, leading to more effective training strategies and injury prevention techniques.
In conclusion, this study provides valuable insights into the complex relationships between HRV parameters and cardiovascular health in soccer players. The identification of two distinct PCs related to sympathetic and parasympathetic activity highlights the importance of monitoring HRV to optimize performance and recovery. Machine learning is important to monitor these changes in the possible molecular mechanisms controlling HRV in soccer players.
Abbreviations
ANS: | autonomic nervous system |
ECG: | electrocardiogram |
HF: | high frequency |
HRV: | heart rate variability |
LF: | low frequency |
LF/HFNormlog: | normalized low frequency/high frequency by logarithmic transformation |
MRR: | mean of the normal sinus intervals |
PC: | principal component |
PCA: | principal component analysis |
pNN50: | percentage of times that the change in consecutive normal sinus intervals exceeded 50 ms |
RMSSD: | root mean square of the successive differences |
SDNN: | standard deviation of all normal sinus intervals |
Declarations
Author contributions
WM: Conceptualization, Writing—original draft, Validation, Supervision, Writing—review & editing. SAMM and THLB: Formal analysis, Investigation, Methodology. CAMdOF: Formal analysis, Investigation. All authors reviewed and approved the final version of the manuscript before submission.
Conflicts of interest
The authors declare that they have no conflicts of interest.
Ethical approval
The study protocol was ethically approved by the Human Research Ethics Committee of the Federal University of Amapá (CAAE: 50150121.1.0000.0003, n° 5.121.013) and complied with the Declaration of Helsinki.
Consent to participate
Informed consent was obtained from the athletes.
Consent to publication
Not applicable.
Availability of data and materials
Due to the privacy of the athletes’ data, we cannot provide the original dataset.
Funding
This research was funded by the Amapá Research Support Foundation (FAPEAP) through its public call 003/2018, specifically within the “Research Program for the Unified Health System (SUS): Management in Health-PPSUS”. The funder had no role in study design, data collection and analysis, decision to publish, or preparation of the manuscript.
Copyright
© The Author(s) 2025.
Publisher’s note
Open Exploration maintains a neutral stance on jurisdictional claims in published institutional affiliations and maps. All opinions expressed in this article are the personal views of the author(s) and do not represent the stance of the editorial team or the publisher.