Abstract
Aim:
Physical activity of nursing home residents can be assessed with tools such as questionnaires and standardized fitness tests. For residents with dementia, however, those tools can be cognitively challenging and difficult to administer. Consumer wearables could potentially aid as an affordable tool for ubiquitous assessment.
Methods:
In this pilot study with 16 participants, we explored how measurements with an off-the-shelf wearable relate to structured observations of physical activity. We collected both processed and raw tri-axial accelerometer data from Samsung wrist-worn fitness trackers. To anchor those data in the free-living environment, we compared the measurements with the physical activity scale of the Medlo behavioral observation scheme.
Results:
We showed that consumer wearables are a valid tool for long-term data collection in this vulnerable patient population.
Conclusions:
Regarding the movement intensity, the data collected by fitness trackers is overall in accordance with the data collected with the observational tool. Regarding the type of movement, we concluded that the automatic activity classification on the wearables is not yet ready for use with a mostly sedentary patient population.
Keywords
Dementia, activity estimation, nursing homeIntroduction
Physical activity (PA) of nursing home residents is researched in the context of increasing health outcomes and well-being [1]. Examples are the association of PA with sleep [2], number of falls [3], or energy expenditure and intake [4]. Dementia and PA influence each other. Depression and apathy might manifest in passivity and low PA, while restless behaviors will manifest as higher PA [5]. Any measurement tool for PA will hence have to be able to account for a large range of activity levels. Additionally, residents with dementia have more non-psychiatric comorbidities than other residents [6]. If not taken into account, comorbidities affecting heart function might make PA estimation based on heart rate less reliable. As in other geriatric patient populations, any measurement tool will have to account for the use of walking aids, such as walkers or canes.
Here, we report on the feasibility and the limitations of measuring PA with a consumer wearable in a Dutch nursing home. The presented data were collected as part of a research project that assessed the impact of providing unobstructed access to green spaces to residents with dementia. The main research question was how more access to green spaces affects the residents’ quality of life, as well as the embedding of dementia care in the local community. We therefore assessed the PA of the residents with different measurement tools, collected data with installed sensors and conducted observations at the nursing home.
Commonly used assessment tools for PA of nursing home residents without cognitive impairment can be divided into three categories: observations of functional fitness tests, questionnaires, and technical measures [1]. The first two types of tools require the participant to understand and follow instructions. For residents with dementia, this might pose a problem if the test is performed at the wrong moment, e.g., after a troubling experience for the resident. Furthermore, a participant’s willingness to cooperate can fluctuate by day and time of the day. For our study, we opted for a consumer-grade fitness wearable, which was easy to put on and remove as well as water proof, which was an important requirement for the nursing staff. Furthermore, commercial wearables are often outfitted with more sensors, such as magnetometers, barometers, or GPS receivers. Those additional sensors collect useful data for activity recognition methods [7]. The option of tracking location outside by GPS was used to assess the use of green spaces by residents in another part of the study. To anchor the sensor data in real-life observations, we used data collected with the Medlo observational tool. The Medlo tool [8] was developed to capture the daily life activities of residents in nursing homes and daycare centers. Observations are logged per minute in 30-min sessions and observers use an observation sheet covering four dimensions of daily life activities in ten variables: activities (type, engagement, PA), physical environment (location, interaction with environment), social interaction (occurrence, type, interaction partner), and emotional well-being (mood, agitation). For the aim of this paper, we focused on the variable “physical activity”, which is scored on a 7-point Likert scale. We used off-the-shelf Samsung wrist-worn wearables to record accelerometer data. Additionally, we recorded manually the “24hrs log” of the watch, which are movement categories that are calculated and displayed on the device (low activity, medium activity, high activity, sleep, run, swim, cycle). We wanted to find out if those movement categories are useful in a mostly sedentary patient population. Furthermore, we wanted to compare PA estimates based on raw accelerometers with the modeled movement categories and observed activity levels.
Materials and methods
Study design, setting, and participants
We report the results of a prospective and observational study. This study is part of the project “Dementia Back in the Heart of Society—a complex case study”. In this project, the impact of the renovation of a nursing home and reorganization of the care management on residents, staff, and family members were assessed. We are presenting here tools used within the study protocol to assess the PA of nursing home residents. The data collection for the overall project was in fall 2018, spring 2019, spring 2021, and fall 2021. In this paper, we present data collected in spring 2021. For the overall project, participants were recruited in two waves in 2019 and in 2021. All residents with a diagnosis of dementia were invited to join the study by a letter to their legal representatives. In spring 2021, 54 residents were invited, leading to 26 participants consenting to participate in the study. Unfortunately, due to informed consent letters reaching us too late, we only managed to collect the following data: for 12 participants, we collected both wearable and observational data; for 4 participants, we collected only wearable data and for one participant only observations. Participants were between 72 and 91 years old on the day of data collection [mean: 82, standard deviation (SD): 5.3 years]. Overall, 15 women and 1 man participated in the data collection with the wearables.
Ethics
The study protocol was approved by the Ethics Review Board at University Medical Center Utrecht (protocol 18-127). The legal representatives of all potential participants received a letter explaining the goal of the data collection and the types of data that were to be collected: observations, questionnaires, medical records, wearables, and interviews. They could consent to all data being collected or just parts of the data collection. While collecting data on-site, all research assistants and present nursing staff were instructed to remove wristbands as soon as residents requested this or were visibly bothered by it. To safeguard the participants’ privacy, all data was exclusively stored on the smartwatches themselves and on the researchers’ laptops. None of the collected data was shared with a third party or the watch manufacturer.
Preprocessing and variable selection
Wearables
We adjusted the settings of the wearables based on the expected participants’ characteristics and set the watches to: gender “female”, birthdate 1st January 1935, height 170 cm, and weight 65 kg. We refrained from setting the watches to the true participant characteristics and accepted a possible bias in the measurement results of the watch. Using the true characteristics would not only have prolonged the exchange moment of the watches but also prone to errors as we kept rotating the watches between participants. Height and weight of the residents are not reliably accessible in patient files and obtaining those just for this data collection would have been an extra burden on this vulnerable patient group. Further, it is unclear how different settings would impact the measurements. We therefore opted for quick and simple transfers, and ensuring that all data are comparable by always having the same settings. We followed the residents’ wishes regarding which wrist they wanted to wear the wristband on. Because we used a commercial wearable (Samsung Gear Fit 2 Pro), we had no documentation on the sensor calibration or the validation of the watch’s activity categories. We performed spinning tests to ensure that the extracted accelerometer data was of good quality [9]. Data from the wearables were collected with our own software and manually. Our software “WEARDA” [9] saved accelerometer data, barometer data, and GPS recordings. The data were saved in “.txt” files on the watches and downloaded from the watches onto the researchers’ computers. No cloud storage service or other third party was involved while storing and processing the data. Data from the app called “24hrs log” were copied manually directly from the watch onto a data collection sheet on a research computer. From the collected wearable data, we used the accelerometer data and the “24hrs log” data to estimate activity levels. The “24hrs log” records per minute the activity intensity as estimated by a Samsung-owned algorithm. The categories are: “not on wrist”, “sleeping”, “inactive”, “light”, “heavy”, “biking”, and “running”. Per resident, we created seven data points analogous to the seven categories by summing up the minutes spent per category. Because not all residents had the same wear time of the wristband, we divided the count by wear time in minutes and multiplied it by 30 min to standardize it.
Accelerometer data were collected at 100 Hz. Analogue to previous studies [10], we chose to calculate the mean amplitude deviation (MAD) metric over 5 second intervals and to derive several summary parameters from those data. The MAD is a measure of movement intensity, calculated based on the magnitude of acceleration within a predetermined interval [11]. We calculated the following measures from the MAD: mean (MADmean), SD (MADsd), median MAD (MADmedian), and 95th percentile of MAD (MADqant). Furthermore, we calculated per participant time spent above 100 milli gravitational units (MADrel), and the fragmentation rate of the MAD (MADfrag). The fragmentation rate is the SD of the first derivative of the MAD time series and is an indicator for intensity changes of PA, with a higher value indicating more changes.
Structured observations
To ground the PA estimates from the wristbands, we used the PA scale of the Medlo observational tool [8]. The PA scale is an ordinal 7-point Likert scale. Per observed minute, the research assistant notes the observed movement category. The movement categories are: ML01 = lying/no movement/sleeping/resting, ML02 = sitting quietly, ML03 = light to moderate sitting, ML04 = standing, ML05 = standing activity/walking around, ML06 = walking activity/cycling, ML07 = sports/whole body movement. In a related study, we checked for interrater reliability of observed activity classifications and found that the collected data matched 91% of the registered activities [12]. The data were transformed into seven data points per resident, by summing up the minutes a resident spent per category. Analogue to the “24hrs log” data, we divided the sums per category by the total observation time and standardized it to 30 min.
All analyses were executed in R. The scripts for the preprocessing and plotting of the data are available on this Zenodo repository: https://zenodo.org/records/10931035.
Results
Summary statistics
For both the Medlo and the “24hrs log” categories, we see mainly sedentary behaviors. Residents spent the most time in the categories “inactive” for the wearable categories (Figure 1) and “sitting quietly” for the Medlo (Figure 2). The wearables detected approximately the same amount of “light” activity as the Medlo observations of “ML04 = standing” and “ML05 = standing activity/walking around” combined. The category “not on wrist” refers to moments when participants wore the watch, but the watch classified those moments wrongly as “not on wrist”. We collected almost no observations for the Medlo categories “ML03 = light to moderate sitting”, “ML06 = active walk/cycling”, and “ML07 = sports/full body movement” as well as for the “24hrs log” categories of “heavy”, “biking”, and “running”. We therefore removed those variables from further analysis. The MAD has an overall mean of 11.15 milligravity units (range: 4.5 to 20.4 milligravity units). This is in accordance with Bakrania et al. [11] who found a mean between 6 and 12 milligravity units (depending on the device) in their sample for sedentary behaviors.
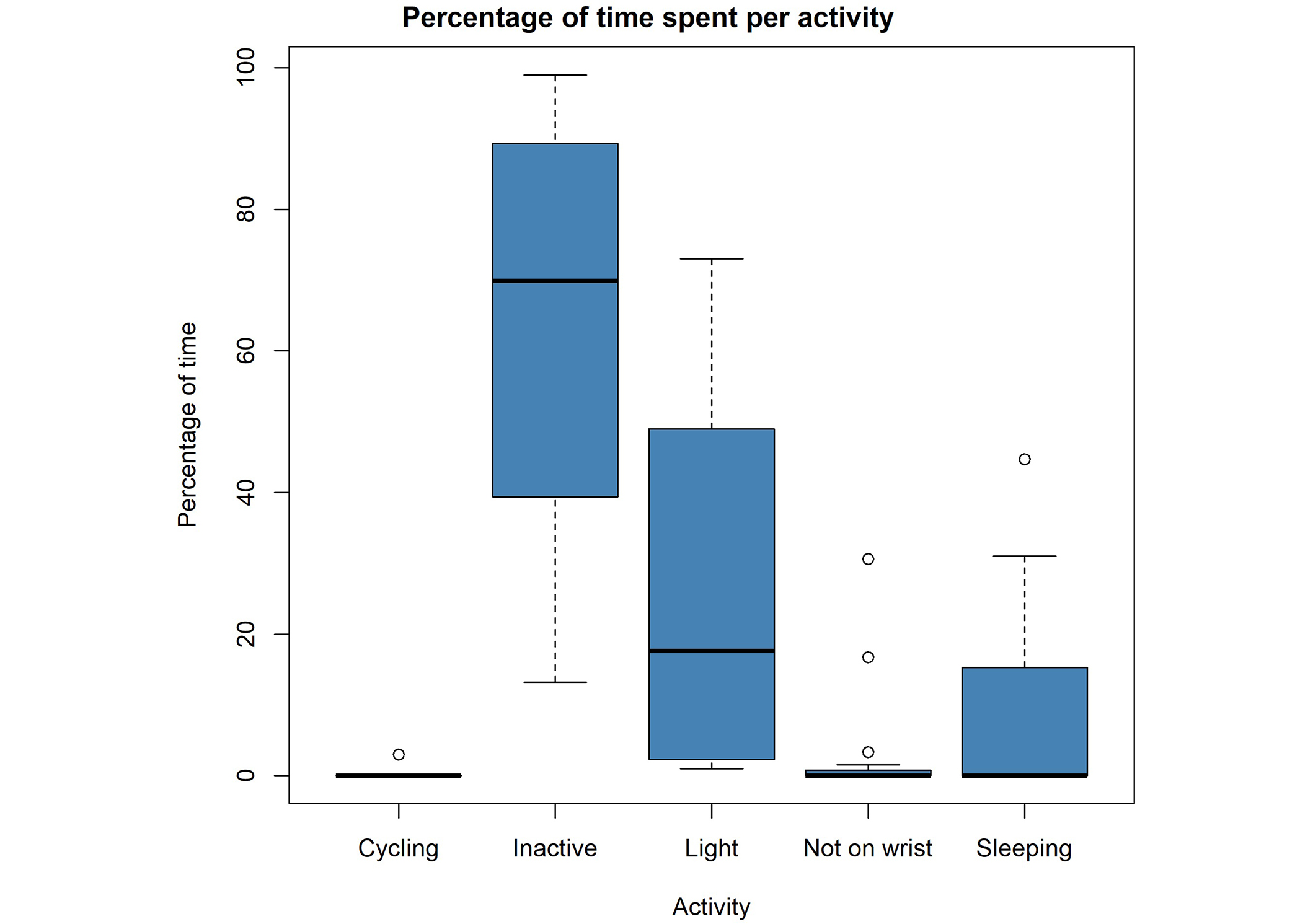
Boxplots of the collected “24hrs log” data of all participating nursing home residents (N = 16). Per activity, we plotted boxplots of the percentage of measurement time that residents were active in one category. The “not on wrist” category refers to moments that residents wore the watch but it was categorized as if the watch was not worn
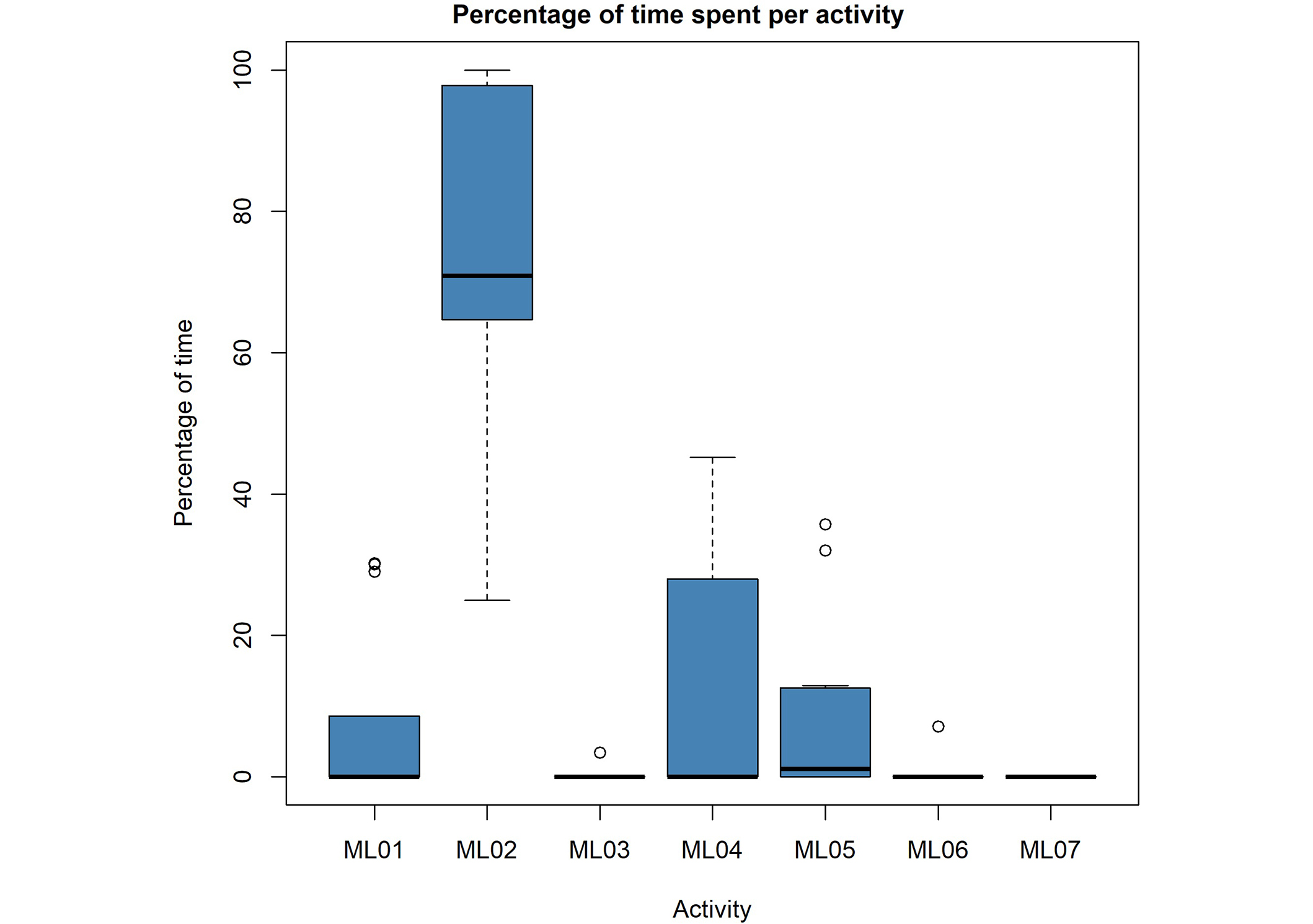
Boxplots of the collected Medlo data of all participating nursing home residents (N = 13). Per Medlo activity category, a boxplot shows the percentage of time that residents were active in one category. Categories are: ML01 = lying/no movement, ML02 = sitting quietly, ML03 = light to moderate sitting, ML04 = standing, ML05 = standing activity/walking around, ML06 = walking activity/cycling, ML07 = sports/whole body movement
Correlation analysis
We correlated all variables with each other after preprocessing the raw data. The correlation matrix in Figure 3 is sorted by hierarchical clustering using the complete linkage method. The clusters show clearly that the MAD-derived metrics correlate somewhat positively with the more active categories of the Medlo (ML04, ML05) and the “light” activity of the watches’ activity classification. There is also a positive correlation with the category “not on wrist”. They correlate negatively with the less active categories of the watches’ model (sleeping, inactive) and the Medlo (ML01, ML02). Unsurprisingly, all MAD-derived parameters correlate highly with each other, however, there are differences in association strength with the other variables. The SD and 95th percentile seem to differentiate best between the more active and more inactive Medlo and watch categories. There are larger positive and negative correlations with the watch categories than with the Medlo categories. The directions of the correlations are as expected; positive with the more active categories and negative with the less active categories. The watches’ and Medlo variables also correlate with each other in the expected way. This includes the watch category “sleeping” that correlates highly (0.66) with the ML01 category, which describes the sleeping and resting of participants. The “not on wrist” category has overall low correlations with other variables but a high correlation with ML05 (0.65), which describes light-standing activities.
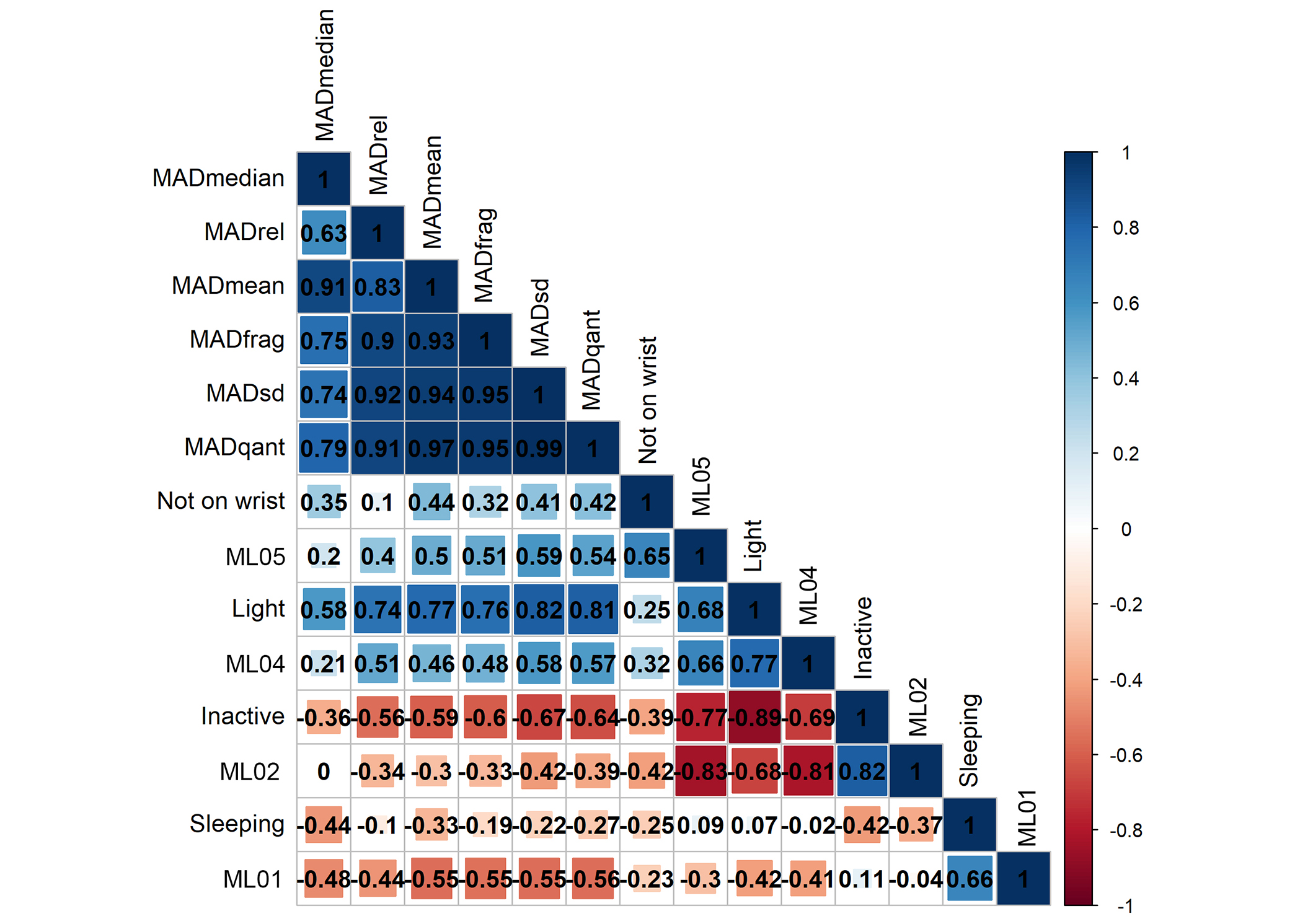
Correlation matrix of all variables. The correlation matrix is sorted hierarchically, which yields two clusters, one with the derivates of the mean amplitude deviation (MAD) and three active categories plus “not on wrist” and another cluster of the least active movement categories. MADrel: time spent above 100 milli gravitational units of the mean amplitude deviation; MADfrag: fragmentation rate of the mean amplitude deviation; MADsd: standard deviation of the mean amplitude deviation; MADqant: 95th percentile of mean amplitude deviation
Within the Medlo categories, there are high negative correlations between ML02 (sitting quietly) and ML04 (–0.81) as well as ML05 (standing and walking around) (–0.83). The more a resident is sitting the less they are standing or walking around. Similarly, within the Samsung categories, we can see a high negative correlation (–0.89) between inactivity and light activity. Again, the more a resident is inactive, the less light activity is recorded. The sleep categories of both the Medlo and the watch are somewhat puzzling as they do not correlate with other variables of the same category, apart from a negative correlation (–0.41) between ML01 (sleep) and ML04 (standing) as well as sleep and inactive (–0.42). The inactive category also correlates negatively with “not on wrist” (–0.39). Unfortunately, we cannot explain those correlations without knowing more about the proprietary Samsung activity classification.
Discussion
Our main question was if we can use consumer wearables to estimate activity levels in nursing home residents with dementia, and how those estimations would relate to observational data. The summary statistics confirmed our expectation of a rather sedentary sample. For the Medlo scores, this is also an artifact of the data collection process, where Medlo observations took place in shared living rooms, which invite residents to sit down and generally do not encourage physical activities. Activities outside the observer’s field of view were registered as “non-observable”, which might cause an underestimation of PA [12]. The wearable data confirm low activity levels over the entire measurement period, which includes time spent outside shared living spaces and outside registered observations. We did not collect data during morning and evening routines, which we would expect to come with a big share of PA, such as walking to and from the living room, moving in and out of bed, and bathroom routines. In future research, one could use wearables during those moments to collect data without breaching residents’ privacy. This would give a more complete picture of the residents’ PA throughout the day. Overall, the found correlations are according to our expectations, with higher scores on MAD metrics being associated with more time spent in higher activity categories. We found positive correlations between the MAD metrics and the active Medlo categories (ML04 and ML05). It is noteworthy that the correlations between the watches’ “light” activity category and the Medlo categories are more pronounced than the correlations between the MAD metrics and the Medlo categories. In our sample, conclusions based on any of the collection tools will be very similar. Apart from PA levels, researchers might be interested in how engaged participants are when performing certain activities or in which context the activity takes place. Observational tools can generate such additional information. We argue that PA scores can be estimated similarly well with observations and wearables. A combination of both however would allow researchers to set the results of the observation period into the context of an entire day of PA. Data from wearables can provide this context without having to rely on carers’ or residents’ memory of that day. For our analysis, we derived several MAD metrics. From the correlation matrix, we see that there are differences in how those metrics correlate with the activity categories. Especially, the SD and the fragmentation rate seem to differentiate better between categories. We suggest including derivatives for the spread of MAD when using raw accelerometer data in predictive models to increase predictive performance.
The presented data are limited by a rather small sample. However, we collected per person a long interval of movement data with the wearables. This makes the associations between the watch-derived data points more robust. Our sample includes almost exclusively women. Future research should ensure to have a gender-diverse sample and register the use of mobility aids throughout the data collection. In an experiment with more participants, it could also be investigated whether setting the watches to the participants’ true characteristics would positively affect the data. A bigger and more diverse sample would allow for subgroup-specific conclusions. At the level of recorded sensor data, future work might consider extending the recordings to other in-built sensors such as the gyroscope or barometer as well as recording physiological measurements such as heart rate. It would be interesting to compare those measurements to observations or as input for machine learning.
Regarding limitations of the used wearable, we would like to point out that this watch was not designed with nursing home residents in mind. The wrists of most residents were very slim, which might add movements of the watch around the wrist to the data. The watches were however comfortable enough to be worn for long stretches of time by most participants, which makes them a good tool for ubiquitous measurement.
We would like to point out that Medlo is not the only observational tool to assess activity levels amongst dementia patients. Any future research should include other tools (surveys, diaries, medical records) to compare the wearable data with and to ground the conclusions drawn based on wearable data.
Conclusions
In this study, we showed that collecting movement data with commercial and consumer-grade wearables in a population of nursing home residents with dementia is possible. The watch allowed us to collect movement data outside shared living spaces and for several hours at a time. The collected wearable data are in general in accordance with the data collected with an observational tool. The data was collected with a commercial wearable but in a GDPR-proof manner, as none of the collected data, nor the settings of the watch was shared with a third party (for example by sending it via a cloud service for access). This opens opportunities for similar research with other vulnerable patient groups. Practical applications include the use of commercial wearables to assess interventions to increase the PA of nursing home residents. In medical care, physiotherapists might be interested in using watches to see if PA changed after a resident’s medication got adjusted or new walking aids were introduced.
Using wearables for ubiquitous long-term assessment of activity levels in a population of dementia patients living in a nursing home is possible and holds many opportunities for assessing PA in real-life situations.
Abbreviations
MAD: | mean amplitude deviation |
PA: | physical activity |
Declarations
Acknowledgments
We thank all participants and staff members for their time and help during the data collection.
Author contributions
DG: Conceptualization, Data curation, Writing—original draft. SP: Conceptualization, Data curation, Writing—review & editing. APAvB and MvL: Conceptualization, Supervision, Funding acquisition, Writing—review & editing.
Conflicts of interest
The authors declare that they have no conflicts of interest.
Ethical approval
The study protocol was approved by the Ethics Review Board at University Medical Center Utrecht (protocol 18-127).
Consent to participate
Informed consent to participate in the study was obtained from all participants.
Consent to publication
Not applicable.
Availability of data and materials
A release of the code to clean the data and generate the plots is avail-able on Zenodo. There is a metadata file available in the same release folder: https://zenodo.org/records/10931035. Data is available for reviewers and to check the computational reproducibility of this work. Data requests from other researchers might be honored but will need to be checked on an individual basis and will require data sharing agreements, contact the first author for this purpose (gawehnsd@liacs.leidenuniv.nl).
Funding
Research funding for this project was received from ZorgOnderzoek Nederland (ZON) and Medische Wetenschappen (MW) (Project number: 733050846), the Dutch funding agency for health and medical research. The funder had no role in study design, data collection and analysis, decision to publish, or preparation of the manuscript.
Copyright
© The Author(s) 2025.
Publisher’s note
Open Exploration maintains a neutral stance on jurisdictional claims in published institutional affiliations and maps. All opinions expressed in this article are the personal views of the author(s) and do not represent the stance of the editorial team or the publisher.