Abstract
Aim:
The lipophilicity of amino acids plays a crucial role in delineating their physicochemical properties, offering insights into solubility, binding affinity, and bioavailability, properties that are a cornerstone for the use of peptides as therapeutic agents. In this study, we employ the integral equation formalism polarizable continuum model/Miertus-Scrocco-Tomasi (IEFPCM/MST) implicit solvation model to compute the n-octanol/water partition coefficient, serving as a lipophilic descriptor for non-standard amino acids. This approach allows us to expand upon our prior scale developed for canonical amino acids.
Methods:
Using the IEFPCM/MST implicit solvation model, we extended our previous work on the hydrophobicity scale of amino acids. To this end, we employed two structural models, Model 1 and 2, differentiated solely by their C-terminal capping groups using an N- or O-methyl substituent, respectively.
Results:
Our findings revealed substantial similarities between the models, validating the lipophilicity values for the non-standard side chains. Differences were observed in fewer cases, indicating an effect of the capping group on the side chain hydrophobicity. This effect is expected as one model contains a hydrogen bond donor (Model 1) while the other one uses a hydrogen bond acceptor (Model 2).
Conclusions:
Overall, both models exhibit good correlations with the experimental values, with Model 1 showing lower statistical errors. In addition, our predictions were able to correctly predict the experimental hydrophobicity change due to the number of acetylated lysines in peptide pairs determined by HPLC, suggesting that our scale can be employed for proteomics studies that include post-translational modifications beyond acetylation.
Keywords
Partition coefficient, lipophilicity, non-natural amino acids, solvation, post-translational modifications, proteomics, lysine acetylationIntroduction
Amino acids are organic molecules that constitute the basic building blocks of proteins. From a functional point of view, mainly directed by their sequence and 3D arrangement, they play a fundamental role in a multitude of biological processes and functions in living organisms, such as enzymatic catalysis, cell signaling, structural support, or immune response, among others [1].
Non-standard amino acids, also known as non-canonical or non-proteinogenic amino acids, deviate from the conventional ones typically present in proteins and synthesized by ribosomes in living organisms. Unlike the standard set of 20 amino acids which are enciphered by the genetic code and commonly incorporated into protein synthesis during translation [2], non-standard amino acids encompass a wide range of structurally diverse molecules, that may occur naturally or be synthesized artificially. Some non-standard amino acids occur naturally in certain organisms, although they are not part of the standard genetic code. For instance, selenocysteine and pyrrolysine, are examples of non-standard amino acids that are incorporated into proteins in certain bacteria and archaea, respectively [3].
Shifting the focus to the physicochemical aspect of amino acids and/or proteins, is important consider their lipophilicity, a fundamental feature with a clear impact on biology, pharmacology, medicinal chemistry, and drug discovery [4, 5]. In the context of proteins is important for understanding processes such as protein folding, where hydrophobic amino acids tend to cluster in the protein interior away from the aqueous environment. It also influences ligand binding, affecting the binding affinity and specificity of proteins and contributing to the formation of receptor-ligand interactions and also in protein-protein interactions, promoting the formation of protein complexes, among others. In addition, recent studies have created energy functions based on lipophilicity for membrane-protein studies of receptors, channels, and transporters [6]. Given these reasons, it is crucial to have tools that permit the quantification of the degree of hydrophobicity of proteins.
For proteins, lipophilicity is primarily influenced by the specific features of the amino acid side chains. Consequently, one of the main strategies, involves quantifying the individual hydrophobicity of each amino acid, leading to the development of lipophilicity scales. These scales consider various properties such as partitioning of small molecules in a bulk solvent, employing knowledge-based techniques based on structural data and/or using experimental information coming from biological assays [7–9].
By employing these scales, it is possible to generate lipophilicity profiles of peptides and/or proteins based on the individual hydrophobicity values of residues, assuming an additivity principle. However, depending on the employed scale, variations can occur not only in the absolute magnitude of residues but also in their relative values. These variations pose difficulties in correlating different scales, as well as reflect discrepancies between materials, methods, and experimental conditions that permit the definition of each scale.
In this line, in our previous study [10], we developed an extensive lipophilicity scale of the 20 standard amino acids based on theoretical computations that took into account the local context of each amino acid in the proteins deposited in the Dunbrak’s rotamer library [11]. Thus, this scale incorporated the structural features of the conformational landscape of amino acids, as well as the impact of pH, providing a reliable depiction of the pH-adapted lipophilicity profile in peptides and proteins.
However, when we move to non-standard amino acids, derivatives that differ in structure or composition from the 20 standard ones usually found in proteins, set a challenge to have new adaptations of the classical lipophilicity scales to be reliably standardized to be applied to those biomolecules with non-canonical modifications.
Recent efforts have focused on the impact of the presence of non-canonical amino acids on peptide and protein structure and function. In fact, this new class of amino acids has found an excellent opportunity for use in the design of peptidomimetics. This is mainly because they have been identified naturally and have been found to improve both the stability of the structures and their bioactivity [12], which clearly points out that all this knowledge promises to deliver new biologically active molecules and therefore that non-standard amino acids (NSAAs) are and will be fundamental in drug discovery. Concerning structure, it has been shown that the presence of such residues decreases the accuracy of structure prediction tools, so it has been recommended to simulate first using the proteinogenic amino acids and then perform the modification to carry out molecular dynamic studies [13]. Regarding function, non-canonical amino acids have emerged in the field of synthetic biology, focusing mainly on the research of biomaterials looking for adhesion capabilities, also in the design of antimicrobial peptides improving their protease resistance, solubility, and half-life [14]. Such efforts have led to novel structure/activity studies on modified peptides that present chemoinformatics tools to efficiently characterize the chemical space of these new peptides and thereby better understand their activities, e.g., their antimicrobial activity against multidrug-resistant bacteria [15].
In the context of the lipophilicity for non-canonical amino acids, prominent examples highlighting the significance and relevance of this topic include recent studies by Kubyshkin (2021) [16] and Oeller et al. (2023) [17]. The computational work of Oeller et al. introduced the Cambridge solvation post-translational modifications (CamSol-PTM) tool, which offers a rapid and accurate methodology for predicting the solubility of peptides containing non-standard amino acids. Regarding to the experimental work of Kubyshkin, aimed to develop an experimental lipophilicity scale incorporating both coded and non-coded amino acids, using the n-octanol/water partition coefficient. This work, based on N-acetyl and O-methyl amino acid analogs, determined the logP for these synthetic compounds using the nuclear magnetic resonance (NMR) technique, which provides a valuable opportunity to validate computational tools for lipophilicity determination. However, it has time constraints in case of generating new chemical modifications due to the experimental protocol to be implemented. Thus, a computational strategy with adequate accuracy to reproduce these experimental values can alleviate the laborious and time-consuming process of the experimental techniques and can offer the advantage of being able to apply a rapid and straightforward strategy to calculate the lipophilicity upon any modification to create a new non-standard amino acid.
Therefore, the present work aims to expand our previous work on pH-dependent lipophilicity scale of amino acids [10], specifically the scale that reproduces the behavior of residues in solvent-like environments (SolvL scale), by extending it to a set of non-standard amino acids presented and experimentally measured by Kubyshkin in 2021 [16]. The objective is to test, validate, and update our lipophilicity scale to properly account for this descriptor on non-coded amino acids.
Materials and methods
Dataset
In the present article, we selected different non-canonical amino acids (see Tables S1–6) that had been previously investigated and published in an experimental study [16]. The work presented by Kubyshkin [16] focused on examining the experimental lipophilicity of non-standard amino acid derivatives originating from methionine, phenylalanine, tyrosine, tryptophan, lysine, and proline using the n-octanol/water system. In our study, non-taking into account the standard versions of amino acids, a total of 57 non-canonical amino acids have been investigated. This includes 7 modifications of methionine, 4 of lysine, 9 of phenylalanine, 4 of tyrosine, 25 of proline, and 8 of tryptophan.
For each molecule, we considered two variants regarding the N- and C-terminal capping groups. These end fragments are responsible for mimicking the peptide bond which confers rigidity to these regions, as well as, aiming to mimic the physicochemical behavior of the amino acid when present inside a protein, rather than in an individual state. Our study included in parallel both variants for all amino acids, in order to preserve the original capping groups from our previous study [10], but also to compare with those used by Kubyshkin [16] in his experimental study (see Figure 1).

Chemical scaffolds of the capping group models used for the amino acids studied in this article: N-methyl (in blue), O-methyl (in green), and Acetyl group (in red). In the case of proline, scaffolds were slightly diverse, due to the natural features of this amino acid (see Figure S1)
Figure 1 shows the first variant, known as “Model 1”, which involves the introduction of an N-methyl (NME) group at the N-terminal end and an acetyl (ACE) group at the C-terminal end of the derivatives. “Model 2” uses the capping groups of the experimental data published in Kubyshkin’s [16] article, which presents slight modifications. While the C-terminal group remains the same, the N-terminal end features an O-methyl (OME) group instead of the NME group.
This a priori small change presumes to have a reasonable impact on the hydrophobicity of the studied compounds. Since the NH to O modification supposes the loss of a hydrogen bond donor interaction and translates into an increase of lipophilicity, like the experimental values of N-methylacetamide (logP = –1.05) and methyl acetate (logP = 0.18) reported by Hansch et al. in 1995 support [18].
Using the DataWarrior software [19], within the framework of Model 1 diverse simple descriptors were calculated to better capture the chemical diversity of the studied sets. Thanks to that it could be highlighted that, the molecular weights of the explored molecules lie in the range of 168 to 339 g/mol (see Figure S2). The total number of rotatable bonds varies from one to nine (see Figure S3). Additionally, the count of hydrogen bond acceptors spans from four to seven (see Figure S4), while hydrogen bond donors range from one to four (see Figure S5). In the context of Model 2, which involves substituting the NH group with an oxygen atom in one of the capping groups, there is an approximate one-unit increase in molecular weight. Simultaneously, there is a decrease of one unit in the count of hydrogen bond donor, while the number of acceptors remains unchanged. For more detailed information check Tables S7–12 in the Supplementary material.
Concerning their lipophilicity, if we consider the difference of each non-standard amino acid compared to the original, based on experimental values reported by Kubyshkin [16] for methionine derivatives, four of them are slightly more lipophilic, and three are more hydrophilic, with variations ranging from plus 0.80 units (most lipophilic) to minus 1.92 (most hydrophilic). In the case of lysine, all derivatives are more hydrophilic than the canonical, with the most marked difference being 2.43 units. Moving to tyrosine, among the four cases, except for a single case (Dopa) that is slightly more hydrophilic, all others are more lipophilic, despite moving in a narrow range from 0.50 (most hydrophilic) to 0.69 (most lipophilic).
For phenylalanine, five derivatives are more lipophilic, three are more hydrophilic, and one has the same experimental logP value. The variation range spans 2.29 units, from the most hydrophilic to the most lipophilic. A similar situation is observed for tryptophan derivatives, where out of the eight cases, only two derivatives with polar groups (5-amino and 5-hydroxy) are more hydrophilic than the standard residue (1.46 units less than the most hydrophilic and 1.17 units more than the most lipophilic, resulting in a range of 2.63 units). Among the 25 proline cases, except for six instances, all are more lipophilic, with the most lipophilic being 1.59 units greater than standard proline and the most hydrophilic being 0.93 units less lipophilic.
We decided not to include some tyrosine derivatives containing iodine atoms in our study. This decision was based on the limitations of the DFT-based integral equation formalism polarizable continuum model/Miertus-Scrocco-Tomasi (IEFPCM/MST) continuum solvation method used for estimating solvation energies, as it lacks parameterization for iodine atoms. However, this method does include parameterization for other halogen atoms like fluorine, chlorine, and bromine, which present minimal differences experimentally when compared to iodine derivatives. Hence, we included molecules containing these three halogen atoms in our study. A similar criterion was taken in the exclusion of selenomethionine from the analysis since the selenium atom is also not included in the IEFPCM/MST current parametrization [20].
Conformational studies and logP estimations
All molecules were designed using Avogadro software (version 1.1.1) [21]. Then, we employed OpenBabel 2.4.0 genetic algorithm to stochastically conduct a preliminary generation of the preferred conformations of the amino acids based on energy score [22]. Due to the structural complexity of some molecules (with several rotatable bonds ranging from 1 to 9), we limited the generation of conformers to a maximum of 100 structures, to make a balance between a complete conformational landscape of them, but at the same time deal with an acceptable number of conformers.
Then, generated geometries of the conformers in both water and n-octanol were optimized using the B3LYP/6-31G(d) level of theory [23–25]. The influence of solvent on the geometric parameters was considered by employing the IEFPCM/MST model [26–28], integrated into a local version of Gaussian16 [29]. The minimum energy state of optimized geometries in each solvent was confirmed by inspecting the vibrational frequencies, excluding those conformations presenting negative ones. Afterward, thermal corrections were introduced to estimate the relative free energy of the conformers in water and n-octanol. Also, single-point energy calculations were carried out in the gas phase to evaluate the solvation free energy of each conformation. Those redundant conformers that after visual inspection converged in the same geometry were eliminated to avoid weight imbalance between both solvents. Obtaining a final conformational distribution in both solvents (like the one highlighted in Figure S6). Finally, the logP value was estimated by considering the Boltzmann-weighted distribution of the conformational families obtained in water and n-octanol.
Capping group reference value based on glycine residue
To ensure that the logP values obtained from our computations were exclusively influenced by the inherent characteristics of their side chains rather than the capping groups, a reference framework was implemented. This involved considering the computationally predicted logP value for glycine, a molecule lacking a heavy atom side chain, but still marked by the influence of capping groups. In the context of the derivatives estimated in Model 1 (incorporating the ACE and NME capping groups), an adjustment was introduced by adding +0.17 logP units to the calculated value. This value originated from the disparity observed between the glycine amino acid value as reported by Zamora et al. [10] in their 2019 publication and the experimental value documented by Fauchère and Pliska [30] on their published scale. Conversely, within the framework of Model 2 (incorporating the ACE and OME capping groups), a correction was made by subtracting –0.78 logP units. This value reflected the difference existing between the logP value computed using the IEFPCM/MST approach and the experimental value detailed in Kubyshkin’s article [16]. Additionally, the structural models used in our previous work [10] did not contain the terminal methylated amide present in Model 1 of this work. However, the effect of methylation is well-known, leading to an increase of logP values by approximately 0.60 units (see Table S13) where we report the computed values for the 20 canonical amino acids using the capping group of the methylated amide). Furthermore, previous research conducted by our group has already explored models featuring methyl groups for hydration computations [31]. Consequently, for the present work, we decided to add a methyl group to better mimic the protein environment and facilitate comparison with the ester model (Model 2) used by Kubyshkin [16].
Results
This work focuses on the reproduction of the experimental values obtained for Kubyshkin [16] using our continuous solvation model. The discussion will be done by amino acid type as follows.
Methionine derivates
The canonical residue methionine is an essential amino acid for its antioxidant effect by reacting with oxidizing species [32], therefore, the tuning of its properties, e.g., lipophilicity, may be relevant to enhance its bioactivity. To this end, Figure 2 shows a consistent behavior between Models 1 and 2. Notably, most lipophilic moieties exhibit a congruent (response about the standard methionine residue values). In Model 1, the logP value is near zero, while in Model 2 there is a slightly augmented lipophilicity (0.27). More detailed values can be observed in Table S14.
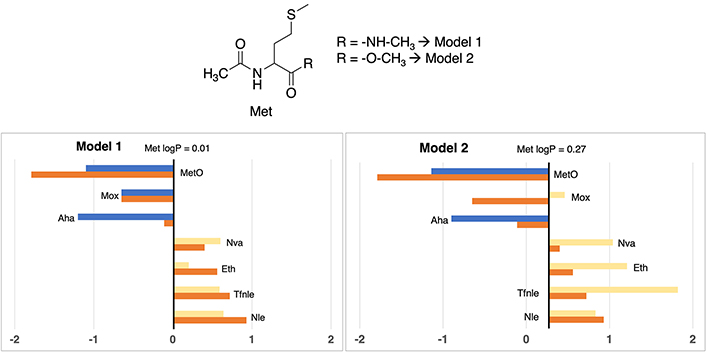
Partition coefficients for methionine (Met) derivatives using Models 1 (left) and Models 2 (right). Standard Met residue value is present in the central line of each representation. Nonstandard residue values more lipophilic and more hydrophilic than the original one, are represented in yellow and blue bars, respectively. Experimental values are represented in orange bars. Detailed experimental data can be found in Table S14
Nonstandard residues ethionine (Eth), norvaline (Nva), norleucine (Nle), and trifluoronorleucine (Tfnle) exhibited a more lipophilic profile than methionine, with logP values moving between 0.20 and 1.82, considering both models. This behavior is logical, attributable to the aliphatic nature of these derivatives (Nle, Nva, and Eth), or the addition of halogen moieties, exemplified by Tfnle.
In the case of hydrophilic derivatives, methionine sulfoxide (MetO) and azidohomoalanine (Aha) a consistent pattern is observed. The introduction of functional groups such as azide or sulfoxide provoked a discernible alteration in the lipophilic profile of methionine, culminating in marked negative values, moving between –0.90 and –1.20, considering both models. This is to be expected due to the high polarity of the oxygen and nitrogen atoms that confer hydrogen bond acceptor properties.
One of the most evident divergences between both approximations arises in the case of methoxinine (Mox), characterized by the replacement of the sulfur atom with an oxygen moiety relative to the standard methionine structure. Model 1 gives a sub-zero value of –0.65, whereas Model 2 manifests a migration towards an apolar value of 0.46. This small incongruity may be ascribed, at least in part, to the presence of an O-methyl capping group in Model 2, different from the NME capping group featured in Model 1. The computational method IEFPCM/MST elucidates a propensity to increase lipophilicity concerning Model 1, accentuated by the alteration of a nitrogen-hydrogen moiety to oxygen, resulting in the loss of a donor hydrogen bond interaction, a structural modification that IEFPCM/MST tends to penalize towards a more lipophilic value.
Although the influence of capping groups is notably adjusted by the previously commented corrections in the methodological section, certain Model 2 values are corrected starting from an overestimated lipophilic value, and therefore exhibiting an inclination towards greater lipophilicity. This tendency is also observed in Eth and Tfnle cases, that present a difference of 1–1.2 logP units between Models 1 and 2.
According to correspondence with experimental data (Table S14), in Model 1, apart from Aha (+1.09 logP units), which contains a chemical group that tends to present difficulties in their estimation, all methionine cases maintain differences lower than 1 logP unit. Instead, in Model 2, two cases are above 1 unit of difference, more specifically Tfnle (1.10 units) and Mox (1.11 units), probably ascribed to the presence of groups that exaggerate their lipophilic profile.
Aromatic derivates
In our study, we analyzed modifications of the main aromatic residues, tyrosine, phenylalanine, and tryptophan. These residues have several functions that maintain the structure and function of proteins. Interactions with cations are essential for maintaining bioactive protein conformations [33]. Thus, any structural modification of these residues will have an impact on both their lipophilicity and aromaticity and thus on the structure/activity relationships.
Tyrosine derivates
Turning our attention to tyrosine derivatives (depicted in Figure 3), a similar pattern to methionine is observed, the logP value tends to augment lipophilicity in Model 2 (0.52), while registering a slightly hydrophilic quotient of –0.02 in Model 1. More detailed values can be consulted in Table S15.
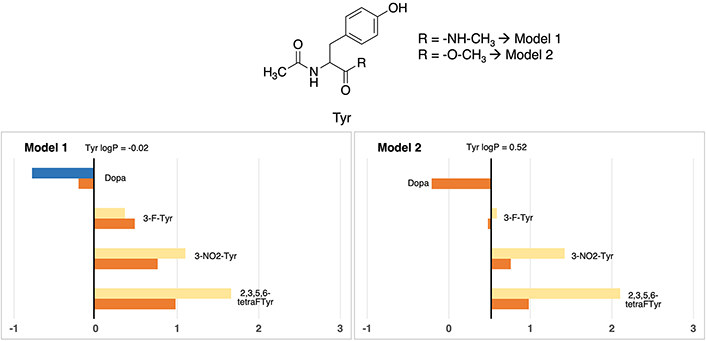
Partition coefficients for tyrosine (Tyr) derivatives using Models 1 (left) and Models 2 (right). Standard Tyr residue value is present in the central line of each representation. Nonstandard residue values more lipophilic and more hydrophilic than the original one, are represented in yellow and blue bars, respectively. Experimental values are represented in orange bars. Detailed experimental data can be found in Table S15
Delving into the assessment of the most lipophilic derivatives, a discernible hierarchy emerges, with 3-fluorotyrosine (3-F-Tyr), 3-nitrotyrosine (3-NO2-Tyr), and 2,3,5,6-tetrafluorotyrosine (2,3,5,6-tetraFTyr) exhibiting proportional lipophilic tendency. Within Model 1, their logP values span from 0.36 to 1.66, while Model 2 assigns values between 0.59 and 2.10—a correlation that is consistently maintained across both models, with the latter consistently indicating a slightly higher lipophilicity.
Conversely, a notable disagreement emerges in the characterization of the Dopa derivative. While Model 1 classifies it as a hydrophilic residue compared to the standard tyrosine (–0.78), Model 2 designates an equivalent lipophilicity to the reference amino acid (0.52). Once again, Model 2 tends to give more lipophilic values in certain cases with respect to Model 1. A trend was observed to a greater or lesser extent in the other tyrosine derivatives. From a chemical point of view, the main difference between tyrosine and Dopa is that the latter has an additional hydroxyl group, a hydrophilic group. Therefore, it is expected that Dopa should have a logP value more similar to that of Model 1 (–0.78), which is more hydrophilic and closer to the experimental value reported by Kubyshkin (–0.21).
In this case, all Model 1 estimations clearly maintain under a 1 logP unit difference with respect to the experimental value. The only case with greater deviation is 2,3,5,6-tetraFTyr, present in Model 2, which shows an overestimation of 1.12 units in logP, probably due to the simultaneous presence of 4 fluorine atoms in their structure.
Phenylalanine derivates
In a similar line with observations in other derivatives, the logP value associated with standard phenylalanine (see Figure 4) reveals a discernible contrast between Model 2 (1.60) and Model 1 (0.61), reflecting a notable increment of one unit in lipophilic propensity within the former. Detailed values can be checked in Table S16.
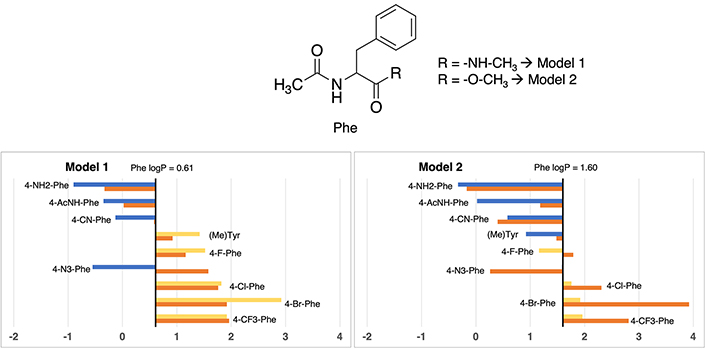
Partition coefficient for phenylalanine (Phe) derivatives using Models 1 (left) and Models 2 (right). Standard Phe residue value is present in the central line of each representation. Nonstandard residue values more lipophilic and more hydrophilic than the original one, are represented in yellow and blue bars, respectively. Experimental values are represented in orange bars. Detailed experimental data can be found in Table S16
The most lipophilic non-standard residues coincide between both models, encompassing 4-fluorophenylalanine (4-F-Phe), 4-chlorophenylalanine (4-Cl-Phe), 4-trifluoromethylphenylalanine (4-CF3-Phe), and 4-bromophenylalanine (4-Br-Phe). In Model 1, this subset gives logP values ranging from 1.52 to 2.92, while Model 2 attributes values span from 1.79 to 3.92. Despite not being an identical range, a certain proportionality is maintained between the 4 residues (4-F-Phe < 4-Cl-Phe < 4-CF3-Phe < 4-Br-Phe). All of them have in common that they are residues with halogen groups where those more lipophilic halogen residues (Br) correspond to those that are less lipophilic (F).
Clear disparities appear in two cases. The characterization of methyltyrosine within Model 1 denotes an apolar amino acid, diverging from Model 2’s classification as slightly polar relative to the reference phenylalanine value. Although the absolute values in both models (1.42 vs. 1.48) closely approximate the experimental value of 0.92—which unmistakably denotes an apolar nature—the discrepant classifications stem from Model 2’s overestimation of the lipophilic propensity of standard phenylalanine (valued at 1.60), influencing the classification. Also, a slight discrepancy between models of the amino acid 4-(Acetylamino)phenylalanine is observed, being more hydrophilic in Model 1 than in Model 2 with a difference of 1.53 units between them.
Delving into experimental values consonance, just some specific cases present deviations greater than 1 unit of logP: 4-azidophenylalanine (2.13 units in Model 1 and 1.32 in Model 2), 4-bromophenylalanine (2.00 units Model 2) and 4-acetamidophenylalanine (1.16 units in Model 2). All cases are in line with other situations observed.
Tryptophan derivates
In this specific scenario, differences between Model 1 and Model 2 about the logP value assessment for standard tryptophan exhibit a nearly negligible difference. As evidenced in Figure 5, Model 1 assigns a value of 1.37, while its Model 2 counterpart presents a value of 1.71, illustrating a marginal deviation of merely 0.34 units. It is worth noting that, both of these values closely approximate the experimental measurement (1.20), differing between 0.17 to 0.51 logP units, denoting a modest shift towards heightened lipophilicity. More exact values are available in Table S17.
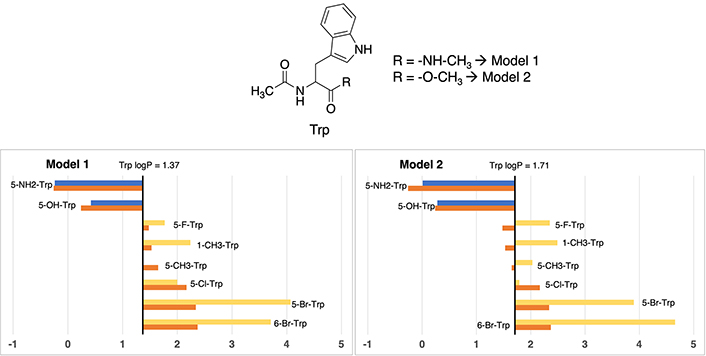
Partition coefficient for tryptophan (Trp) derivatives using Models 1 (left) and Models 2 (right). Standard Trp residue value is present in the central line of each representation. Nonstandard residue values more lipophilic and more hydrophilic than the original one, are represented in yellow and blue, respectively. Experimental values are represented in orange bars. Detailed experimental data can be found in Table S17
A clear trend emerges, demonstrating the congruence in residue classification across both models. On one hand, residues exhibiting enhanced polarity relative to standard phenylalanine are characterized by the introduction of aliphatic or halogen substituents. Examples encompass 5-methyltryptophan (5-CH3-Trp), 5-fluorotryptophan (5-F-Trp), 5-chlorotryptophan (5-Cl-Trp), 1-methyltryptophan (1-CH3-Trp), 6-bromotryptophan (6-Br-Trp), and 5-bromotryptophan (5-Br-Trp), characterized by absolute values ranging from 1.37 to 4.07 in Model 1, and 1.79 to 4.66 in Model 2. On the other hand, polar residues are exemplified by 5-aminotryptophan (5-NH2-Trp) and 5-hydroxytryptophan (5-OH-Trp), exhibiting values of –0.24 and 0.42 in Model 1, and 0.01 and 0.28 in Model 2, respectively. While the trend holds true for hydrophilic residues, certain alterations in the order become evident in the case of hydrophobic derivatives, particularly noticeable in the order of bromine and methyl derivatives.
In this case, divergent values compared to experimental ones are, in both models, the two compounds with bromine atoms: 5-Br-Trp and 6-Br-Trp, being always too lipophilic. For 5-bromotryptophan, the difference is between 1.56 and 1.73 logP units, and for 6-bromotryptohan is between 1.34 and 2.29. The rest of cases maintain values lower than 1 logP unit.
Lysine derivates
This amino acid represents the most frequently post-translationally modified so it is no coincidence its impact on protein regulation and function [34]. The reference logP value for standard lysine is taken from Zamora et al. [10], which gives an approximate logP of –0.40 for the amide model (the derived experimental logP from the Fauchère’s experimental [30] logD7.4 = –3.07 and using a pKa = 10.0 yields an experimental logP of –0.47) and 0.17 for the N-acetyl-L-amino-acid-N-methyl amide (Model 1) residue (see Table S13). Based on that, as can be seen in Figure 6, most lipophilic nonstandard residue is S-allylcysteine (Sac), which have almost identical values in one each model, around ~1.30, respectively. Absolute values can be checked in Table S18.
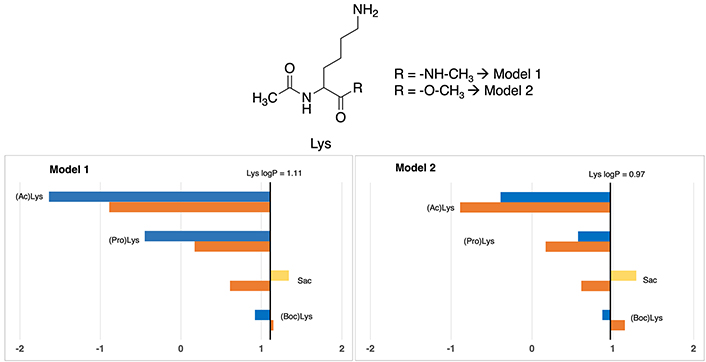
Partition coefficients for lysine (Lys) derivatives using Models 1 (left) and Models 2 (right). Standard Lys residue value is present in the central line of each representation. Nonstandard residue values more lipophilic and more hydrophilic than the original one, are represented in yellow and blue, respectively. Experimental values are represented in orange bars. Detailed experimental data can be found in Table S18. For more detailed information from the Lys logP reference value (for Models 1 and 2), check Supplementary material
However, discrepancies manifest in the two other cases. Concerning N-propargyloxycarbonyl-lysine [(Pro)Lys], Model 1 considers it slightly polar (–0.45), whereas Model 2 positions it distinctly as more apolar (0.57), but in both cases more apolar compared to coded lysine (1.11). Let us mention that, these differences, although not negligible, are within the range found even in experimental measurements.
According to N-acetyl-lysine [(Ac)Lys], Model 1 denotes an extremely hydrophilic characterization of –1.64, while Model 2 persists in –0.39. Chemically insight underscores the incorporation of an ACE group, analogous to (Boc)Lys and [(Pro)Lys], that should provoke a slight increase in the lipophilicity. Consequently, Model 1 tends to excessively accentuate the hydrophilic trait of this residue, while Model 2’s depiction is more aligned with a hydrophobic profile. However, the tendency is to be more hydrophilic than the standard version of the amino acid.
In this case, the comparison with experimental data shows that all cases in both Models almost have a perfect fitting, presenting a difference of lower than 1 unit. In Model 1, the most deviated case has a 0.75 units divergence while in Model 2 is 0.68 units.
Proline derivates
In the context of proline residues, their examination was previously undertaken by Matamoros et al. in 2022 [35], employing the SMD solvation approach [36], In the current study, we subject these residues to analysis via our IEFPCM/MST methodology. As illustrated in Figure 7 and detailed in Table S19, the outcomes affirm the comparable efficacy of our approach, thus rendering it well-suited and eminently applicable for extending our scale of lipophilicity.
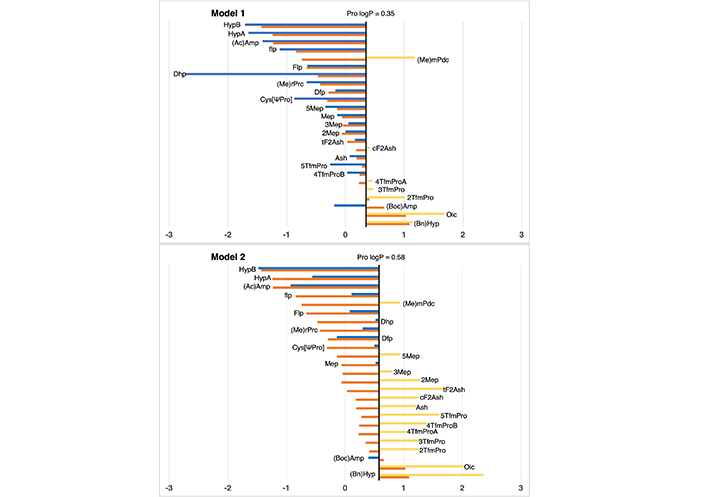
Partition coefficient for proline (Pro) derivatives using Models 1 (up) and 2 (down). Standard Pro residue value is present in the central line of each representation. Nonstandard residue values more lipophilic and more hydrophilic than the original one, are represented in yellow and blue, respectively. Detailed experimental values can be found in Table S19. For more detailed information from the Pro logP reference value (for Models 1 and 2), check Supplementary material
Regarding proline, in general, all cases maintain a difference with respect to the experimental value, lower than 2 units of logP in both models. Only dehydroproline (Dhp) in Model 1, presents a deviation of 2.26 logP units, presenting an excessive hydrophilic profile due to the capacity of performing hydrogen bond interaction of the NH group.
General correlation between experimental and computational data
Regarding the correlation between experimental and computational estimations, Figures 8 and 9 reveal a noteworthy similarity between the two models. Both exhibit a correlation coefficient (R2) that is moderately acceptable—0.73 for Model 1 and 0.71 for Model 2. Also, the root mean square error (RMSE) for Model 1 is 0.7, while Model 2 stands at 0.9. Furthermore, additional statistical parameters such as MSE and mean unsigned error (MUE) also indicate a consistent pattern across both models. Despite the subtle differences, Model 1 demonstrates a stronger correlation and exhibits lower error when compared to the experimental values. This observation aligns coherently with our previously published findings that utilized similar capping groups as those in Model 1.
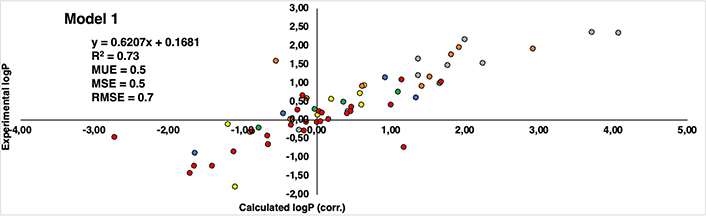
Correlation between calculated logP (corrected by eliminating the influence of capping groups of Model 1) (axis X) and experimental logP values reported by Kubyshkin (axis Y). Groups of residues are represented in different patterns of colors: methionine (yellow), tyrosine (green), phenylalanine (orange), tryptophan (grey), lysine (blue), and proline (red)
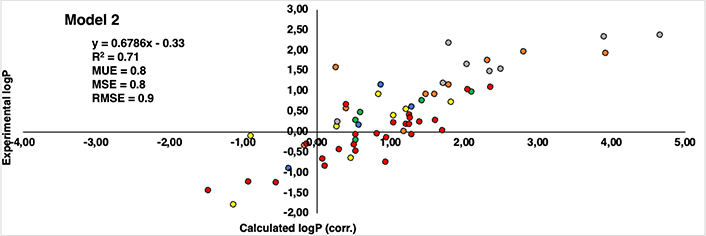
Correlation between calculated logP (corrected by eliminating the influence of capping groups of Model 2) (axis X) and experimental logP values reported by Kubyshkin (axis Y). Groups of residues are represented in different patterns of colors: methionine (yellow), tyrosine (green), phenylalanine (orange), tryptophan (grey), lysine (blue), and proline (red)
Delving into specifics, it becomes evident that indistinctly of the model considered, all methionine and tyrosine residues (yellow and green colored dots in Figures 8 and 9, respectively) consistently exhibit present values with a discrepancy not higher than 1.2 logP units. Similarly, in the case of lysine is even better, remaining below than 1 logP unit in all cases (blue colored dots in Figures 8 and 9). This can be translated into the fact that there is hardly any difference between the experimental values and those reproduced computationally. It’s worth noting that a deviation near 1 logP unit can often be attributed to inherent errors within the method itself.
As regards the other derivatives, in general trend is maintained, however, certain cases with notably substantial discrepancies have come to light. In the case of the phenylalanine derivatives (orange-colored dots in Figures 8 and 9), the Aha derivative (4-N3-Phe) in Model 1, presents a deviation of 2.13 units from the experimental value, though this deviation is somewhat mitigated in Model 2 (1.32 units). An underlying explanation could be associated with the azide group present within the side chain. Despite the group’s net charge being neutral, there exists a subtle polarization distributed across its nitrogen atoms. This charge distribution potentially contributes to deviations and fluctuations in accurately estimating the compound’s lipophilicity [37–42]. The difference of 0.81 units in the assessment of the identical residue across Model 1 and Model 2 might arise from the distinct origins of their unadjusted initial values. In Model 1, the original value comes from a measurement involving the NME capping group, introducing a hydrogen bond interaction that doesn’t occur with the OME capping in Model 2. Consequently, this residue could potentially be overestimated as hydrophilic, driven by the presence of the NME capping group. This last aspect is also observed in the most deviated case from the proline dataset (red dots in Figures 8 and 9), Dhp in the case of Model 1 deviates 2.26 units more hydrophilic than the experimental one.
Another pattern observed in deviated cases is the presence of bromine atoms, more specifically, in the case of phenylalanine and tryptophan residues (orange and grey colored dots in figures 8 and 9, respectively), 4-Br-Phe, 5-Br-Trp and 6-Br-Trp present a more marked difference. While the rest of the residues present a deviation around 1 logP unit, these cases move around 1.34 and 2.29, between both models. The bromine atom is a relatively heavy atom compared to other lighter halogens like fluor and chlorine (deviations around 0–0.8 logP units) and it has unique electronic properties due to its larger size and higher atomic number. These factors can influence the interactions of bromine with its surrounding environment, including solvent molecules and other atoms in a molecule.
Another potential source of mismatch between experimental and computational results can be attributed to the fact that IEFPCM/MST method is an implicit solvation model, that treats the solvent as a continuous dielectric medium, simplifying the simulation and reducing the computational cost. This approach permits to be more efficient but sometimes can oversimplify the solvent behavior and fail to capture specific solvent-solute interactions accurately. Contrary to that explicit solvation models represent individual solvent molecules, which can sometimes permit a detailed description of these interactions. For instance, the polarized structure-specific backbone charge (PSBC) explicit model has been successful in predicting both the experimental folding of helical peptides [41] and β-sheet structures [42] thanks to considering the polarizability of backbone hydrogen bonds by implementing the partial charges of backbone hydrogen-bond donor and acceptor atoms during peptide folding simulations. However, despite their differences some bibliography holds that for predicting solvation energies and partition coefficients, both methods can perform quite reasonable results [43, 44].
Application of non-standard amino acids in proteomics
Acetylation is a relevant post-translational modification that is mainly carried out in both the ε-amine on the side chain of lysine and in the α-amine of the N-terminus in peptides and proteins [45]. In health and pathological states, the reversible acetylation of lysine residues plays a crucial role in regulating cellular and developmental processes. These facts make the correct identification of acetylated peptides and proteins a constantly developing field in modern proteomics [46]. In this context, experimental studies have analyzed the impact of peptide acetylation on chromatographic retention time in reversed phase-high performance liquid chromatography (RP HPLC) in order to create predictive models that suggest an efficient way for the separation of these peptides that will allow their identification [46].
Table 1 reports the experimental hydrophobicity index (ΔHI) obtained by Mizero and collaborators [47], calculated as the difference between the hydrophobicity index for modified (acetylated) and non-modified peptide pairs expressed in % acetonitrile (% ACN). As can be seen, it is evident that the greater the change in the degree of acetylation in the lysines of the peptide pairs, the greater the amount of organic solvent (% ACN) will be necessary.
Variation of the experimental hydrophobicity index (HI) using as separation mode acetonitrile (% ACN) in RP HPLC 0.1 % of formic acid for modified (acetylated) and non-modified peptide pairs
Acetylated lysine residues in peptide (number of peptide pairs) | Experimental hydrophobicity index (ΔHI) in RP HPLC 0.1% formic acid (% ACN) | Δ(Ac)Lys | ΔΔHI/Δ(Ac)Lys |
---|---|---|---|
0 (10,632) | 5.02 | - | - |
1 (13,791) | 9.14 | 1 | 4.12 |
2 (2,390) | 11.20 | 2 | 6.18 |
3 (316) | 12.54 | 3 | 7.52 |
Figure 10 depicts the derivative of the change in the hydrophobic index with respect to the change in (Ac)Lys residues in the modified/non-modified pairs [ΔΔHI/Δ(Ac)Lys] from which the slope permit to obtain the increase in hydrophobicity due to the acetylation of a lysine residue (~1.70 units).
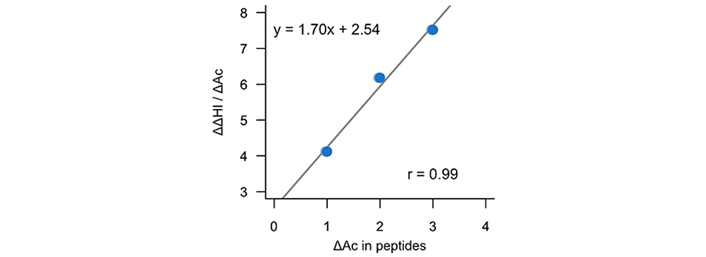
Representation of the variation of the experimental hydrophobic index (% ACN) by the change in the number of acetylated lysines in peptide pairs as a function of the change of acetylated lysines in peptide pairs
In order to further evaluate the reliability of the predictions of the calculations performed in this work, Table 2 reports the increase of hydrophobicity under the acetylation of lysine residues (ΔlogPAc) using our computations but also those obtained of ChemAxon [48] and milogP [49]. Let us mention that HPLC separation systems are carried out under acidic conditions (pH around 2). Therefore, our reported value of –3.07 refers mainly to the partitioning of the ionic species (logD2) and not to the neutral species (logP). For acetylated lysine, the reported value corresponds to the logP. Thus, since our prediction (~1.43 logP units) is close to that obtained experimentally (see Figure 10), it can be used to efficiently predict experimental HPLC conditions to separate acetylated peptides and thus, be used for proteomic studies.
Partition coefficients (logP) values for lysine (Lys) and acetyllysine [(Ac)Lys] using the capping groups of the Model 1 and change in lipophilicity due to acetylation (ΔlogPAc) of lysine residues
Amino acid code | Lipophilicity | ΔlogPAc | |
---|---|---|---|
Lys (logD2) | (Ac)Lys (logP) | ||
This work | –3.07 | –1.64 | 1.43 |
ChemAxon | –4.51 | –1.66 | 2.85 |
milogP | –3.73 | –1.25 | 2.48 |
Discussion
The lipophilicity of amino acids is one of the main physicochemical properties of these biomolecules as it gives an estimate of solubility, binding propensity, and bioavailability. In this work we show how several structural modifications in residues such as methionine, aromatic, lysine, and proline can tune the hydrophobic properties of these residues, opening a window of possibilities to be used as a guide for the design of peptides and proteins with tailor-made characteristics. The structural models used, based on differences in capping groups showed mostly important similarities, validating the lipophilicity values obtained for the non-standard side chains.
Delving into the specifics of the deviations in both number and magnitude, the results presented herein demonstrate considerable promise. Evaluating the proposed models and considering that deviations of up to 2 logP units fall within the expected variation for the estimated parameter [50], we can observe that out of the 126 estimated cases (considering Models 1 and 2) only 3 surpass the threshold of two units. This translates to a deviation rate of less than 2.5% across the dataset. Notably, amino acids such as methionine, tyrosine, and lysine exhibit no instances surpassing this limit. Even employing a stricter criterion for all the sets (deviation exceeding 1 logP unit), still 80% of the cases remain within an acceptable range of accuracy. As previously pointed out in this study, the calculated values and associated errors demonstrate promise, aligning well with those reported in previous research on widely studied canonical amino acids with established functional groups [10]. Once again, the IEFPCM/MST model has demonstrated good accuracy with minimal uncertainties, a performance consistently reflected in the SAMPL blind challenges [51, 52]
Differences were found in fewer cases, which can be expected as one model uses a hydrogen bond donor (Model 1) while the other uses a hydrogen bond acceptor (Model 2), this lies in the effect of the polarizability on the atoms in the backbone which includes the capping groups [41, 42]. In general, both models correlate well with the experimental values, obtaining lower statistical errors in the case of Model 1.
In addition, our predictions were able to efficiently predict the experimental hydrophobicity change due to the number of acetylated lysines in peptide pairs determined by HPLC, opening up the possibility that our scale can be employed for proteomics studies that include post-translational modifications beyond acetylation.
Overall, this work represents the first computational work to systematically reproduce the lipophilicity values of non-canonical amino acids, paving the way for these can be easily implemented in computational tools related to the calculation of peptide solubility, aggregation, scoring functions of molecular docking programs and also to efficiently predict the presence of post-translational modifications in the area of proteomics.
Abbreviations
ACE: | acetyl |
(Ac)Lys: | N-acetyl-lysine |
Aha: | azidohomoalanine |
Eth: | ethionine |
IEFPCM/MST: | integral equation formalism polarizable continuum model/miertus-scrocco-tomasi |
Tfnle: | trifluoronorleucine |
NME: | N-methyl |
OME: | O-methyl |
RP HPLC: | reversed phase-high performance liquid chromatography |
4-Br-Phe: | 4-bromophenylalanine |
5-Br-Trp: | 5-bromotryptophan |
6-Br-Trp: | 6-bromotryptophan |
Supplementary materials
The supplementary materials for this article are available at: https://www.explorationpub.com/uploads/Article/file/100853_sup_1.pdf.
Declarations
Author contributions
AV and WJZ: Conceptualization, Data curation, Formal analysis, Funding acquisition, Investigation, Methodology, Project administration, Resources, Supervision, Writing—original draft, Writing—review & editing. PM and DBR: Investigation, Data curation, Visualization, Writing—review & editing.
Conflicts of interest
The authors declare that they have no conflicts of interest.
Ethical approval
Not applicable.
Consent to participate
Not applicable.
Consent to publication
Not applicable.
Availability of data and materials
The datasets generated and/or analyzed for this study can be found in the NSAAs-Lipophilicity Github repository: https://github.com/tviayna/NSAAs-Lipophilicity.
Funding
The authors thank the Spanish Ministerio de Ciencia e Innovación [PID2020-117646RB-I00, MCIN/AEI/10.13039/501100011033], Generalitat de Catalunya [2021SGR00671], and Consorci de Serveis Universitaris de Catalunya [CSUC; Molecular Recognition project] for financial support. The authors thank the Vice Chancellor for Research of the University of Costa Rica for its support work via the research project [115-C1-450]. The funders had no role in study design, data collection and analysis, decision to publish, or preparation of the manuscript.
Copyright
© The Author(s) 2024.