Abstract
The idea that proteins are the main determining factors in the functioning of cells and organisms, and their dysfunctions are the first cause of pathologies, has been predominant in biology and biomedicine until recently. This protein-centered view was too simplistic and failed to explain the physiological and pathological complexity of the cell. About 80% of the human genome is dynamically and pervasively transcribed, mostly as non-protein-coding RNAs (ncRNAs), which competitively interact with each other and with coding RNAs generating a complex RNA network regulating RNA processing, stability, and translation and, accordingly, fine-tuning the gene expression of the cells. Qualitative and quantitative dysregulations of RNA-RNA interaction networks are strongly involved in the onset and progression of many pathologies, including cancers and degenerative diseases. This review will summarize the RNA species involved in the competitive endogenous RNA network, their mechanisms of action, and involvement in pathological phenotypes. Moreover, it will give an overview of the most advanced experimental and computational methods to dissect and rebuild RNA networks.
Keywords
Competing endogenous RNAs, microRNAs, long non-coding RNAs, circular RNAs, pseudogenes, cancer, methods to study RNA-RNA interactions, RNA-RNA interaction databasesIntroduction
At the beginning of the Human Genome Project (HGP) in the late 1990s, scientists hypothesized that the human genome contained approximately 100,000 protein-coding genes [1]. Such (today considered) overabundant estimation was the result of several misunderstandings and misconceptions: i) the empirical observation of gene number in organisms less biologically complex than Homo sapiens (e.g., the nematode Caenorhabditis elegans), ii) the consideration of the average size of genes and their non-overlapping and uniform distribution inside the genome, iii) inferring the gene number from the assembly of expressed sequence tags (ESTs) [1–3]. Over the years, the initial estimate of 100,000 protein-coding genes for humans has been progressively reduced. In 2001, the International Human Genome Sequencing Consortium (IHGSC) published the first human genome sequence, estimating that there were approximately 30,000 protein-coding genes [4]. At once, Celera Genomics (the IHGSC competitor) estimated this figure to be 26,588 [5]. This number was reduced to about 24,500 [6] when the final draft of the human genome was published in 2004, but an additional analysis in 2007 determined that it was around 20,500 [7]. Although this estimate was reduced to nearly 19,000 in the following years [8], the number of human protein-coding genes has been now assessed to 19,969 according to a recent re-sequencing by the Telomere-to-Telomere (T2T) Consortium [9]. This relatively small number, very close to other mammals or invertebrates, came as a shock to many scientists because counting genes was considered as a way of quantifying genetic complexity. The raw number of protein-coding genes is not sufficient to describe the molecular and functional complexity of proteomics within human cells: a tremendously higher number of protein species originate from the protein-coding genes thanks to alternative splicing, while the activity of these molecules can be regulated by post-translational modifications, subcellular localization or modulation of half-life [10]. Nevertheless, there is a piece of evidence supporting the hypothesis that proteins alone are not sufficient to explain the complexity of multicellular organisms: although the number of protein-coding genes gradually decreased, the number of other gene types was going to explode. The T2T final estimate is particularly startling because it would imply that less than 2% of the entire human genome encodes proteins. Accordingly, the 98% of the human DNA (the so-called dark matter of the genome), which does not encode proteins but would be endowed with essential regulatory functions, may be the foundation of Homo sapiens’ complexity. The Encyclopedia of DNA Elements (ENCODE) and Roadmap Epigenomics projects, two significant scientific initiatives funded by the US National Institutes of Health over the past twenty years, released ground-breaking information on the hundreds of thousands of functional regions of the human genome that control gene expression [11, 12]. These findings suggested that structural functions take up much less room in the human genome than regulatory functions. Additionally, it was suggested by these studies that about 80% of the human genome is dynamically and widely transcribed, primarily as non-protein-coding RNAs (ncRNAs). Over the past years, the non-coding transcriptome’s biological importance has become indisputable. The relative amount of genome space occupied by the proteome-encoding-genome compared to the regulatory (non-protein encoding)-genome varies greatly among evolutionarily distant species. For example, the protein-coding genome makes up only 2% of mammalian genomes while it accounts for almost the entire genome of the unicellular yeast Saccharomyces cerevisiae [13]. Intriguingly, complex diseases like cancer frequently involve quantitative changes to the non-coding transcriptome [14, 15]. These findings strongly imply that the complexity of higher eukaryotes and ncRNAs are closely related, and that pathological phenotypes could result from ncRNA dysfunction. RNA is a structurally adaptable molecule that can carry out a variety of molecular tasks. RNA can bind both DNA and RNA molecules in a very specific way by simple base-pairing and can control their transcription, processing, editing, translation, or degradation. Moreover, the tridimensional folding of RNA molecules and their dynamic conformational changes due to ligand binding, which give them allosteric properties and expand the range of potential molecular interactors (also including proteins), represent a widening area for potential therapeutic applications [16]. For all of these reasons, molecular interactions of RNAs, above all ncRNAs, have recently received a lot of attention due to their important biological roles in regulating cellular mechanisms. NcRNA genes are classified into two groups based on their transcript length: i) long non-coding RNAs (lncRNAs), including circular RNAs (circRNAs), have a length greater than 200 nucleotides (nts); ii) small non-coding RNAs have a length equal to or inferior to 200 nts [i.e., microRNAs (miRNAs), small interfering RNAs (siRNAs), small nuclear RNAs (snRNAs or U-RNAs), small nucleolar RNAs (snoRNAs), PIWI-interacting RNAs (piRNAs), tRNA-derived small RNAs (tsRNA), and Y RNA-derived small RNAs (YsRNAs)] [14, 17, 18]. The majority of current research has concentrated on the roles that ncRNAs—particularly miRNAs—play in human pathophysiology and in modulating the expression of protein-coding genes. However, the unexpected interplay of ncRNAs, which affects cell physiology and diseases, has recently come to light, according to new evidence [19]. NcRNAs can interact with and regulate each other through a variety of molecular mechanisms in addition to the conventional multilayered control of the expression of protein-coding genes, which is succinctly described below. This interaction results in a complex network involving various RNA species. As competing endogenous RNAs (ceRNAs), ncRNAs in such a regulatory network compete with one another for binding messenger RNAs (mRNAs), thus influencing their translation and, consequently, cellular processes [20]. In this review, we will discuss the current understanding of the intricate crosstalk among ncRNAs (including miRNAs, lncRNAs, circRNAs, and pseudogenes) and how they might work in concert to control cell physiology and, accordingly, pathological phenotypes, including cancers.
Competing interactions among RNA molecules
With the recent identification of several classes of ncRNAs, the fundamental role of RNA molecules in regulating cell physiology has been widely recognized. Indeed, the sequence of an RNA molecule is not only important for the translation of a functional protein but also for the possibility to interact with other transcripts by sequence complementarity [21]. It is well known that mutations also occurring outside the sequence of a protein-coding gene may be detrimental to cell health, because these mutations may affect RNA-RNA interactions (RRIs) and, consequently, alter the fine regulation mediated by RNAs [22]. The ceRNA network hypothesis considers all the interactions between RNA molecules in one single scenario, where the simultaneous presence of different molecules creates a competition for the interaction with a single transcript, thus tuning cell phenotype according to the stoichiometric equilibrium among all the molecular species [23]. The most interesting aspect of this hypothesis is that it involves both coding and non-coding RNAs, namely mRNAs, miRNAs, lncRNAs, circRNAs, and transcribed pseudogenes. That means competitive interactions are able to i) regulate gene expression at the post-transcriptional level by acting on mRNA stability or translation and miRNA availability; ii) alter the subcellular localization of RNAs and proteins according to the involved molecules; iii) modulate splicing patterns towards the production of specific splicing variants; etc. These observations make the ceRNA network a very simple and basic, but also potent and precise mechanism of epigenetic regulation within cells [21, 24].
Classically, the ceRNA hypothesis is centered on miRNAs [25]: indeed, it is well known that each mRNA can bear several miRNA-response elements (MREs) and, consequently, it can be targeted by several miRNAs. Based on that, Seitz [26] proposed a first hypothesis about the competition among miRNA targets for the binding of the ncRNA. Similarly, MREs are also present in other RNA molecules, such as lncRNAs, circRNAs, and transcribed pseudogenes, creating a competition among all these RNAs for the binding of a single miRNA species [27]. However, the competition may not directly involve the interaction with the miRNA. As an example, it has been shown that some lncRNAs are natural antisense transcripts (NATs) for a protein-coding gene, being transcribed from the opposite strand of the same locus; the function of a NAT may be to directly bind the mRNA and prevent the recognition of MREs: sirtuin 1 (Sirt1) and Sirt1 antisense RNA (Sirt1-AS) in mouse [28], beta-secretase 1 (BACE1) and BACE1 antisense RNA (BACE1-AS) in human, see below [29] or splicing sequences zinc finger E-box binding homeobox 2 (ZEB2) and ZEB2 antisense RNA 1 (ZEB2-AS1) in human, see below [30]. These findings demonstrate that the ceRNA hypothesis must be widened to include all interactions among all classes of RNA molecules, including also those interactions not involving miRNAs.
Looking at this complex scenario where a variety of RNA species plays an important role, several different molecular mechanisms have been reported and suggested. In this paragraph, we will summarize some of the proposed mechanisms through which interactions involving a specific class of RNA molecules regulate cellular processes.
miRNAs
miRNAs are small endogenous non-coding transcripts sized 18–25 nts acting as post-transcriptional negative regulators of gene expression. Their mechanism of action has been widely studied in the last few decades, showing that miRNAs bind specific MREs within the 3’-untranslated region (UTR) of mRNAs, thus inducing their degradation or block of translation. Viruses also produce miRNAs to manipulate cell physiology and the host immune system to their advantage [31]. The portion of the miRNA involved in the binding of the target is named “seed region”: it is located at the 5’-end, includes bases 2 to 8, and is highly conserved among species. After miRNA binding, the target mRNA is loaded into the RNA-induced silencing complex (RISC), a ribonucleoprotein complex leading to mRNA cleavage of inhibition of protein synthesis. Classically, it was reported that perfect complementarity causes mRNA degradation, while repression of translation requires a shorter region of complementarity, including bases 2 to 7 of the miRNA [27, 32]. Also, imperfect complementarity mainly determines translation inhibition rather than mRNA degradation [33]. It is possible to classify miRNA target sites according to the complementarity with the seed region: i) 8mer sites are characterized by the binding with bases 2–8 of the miRNA (the entire seed region plus the following nucleotide) and include an adenine (A) in the opposite position to the first nucleotide of the miRNA; ii) 7mer sites show either base-pairing with bases 2–8 (7mer-m8 site) or complementarity with bases 2–7 of the miRNA and an A in the opposite position of the first nucleotide of the miRNA (7mer-A1 site); iii) 6mer sites present complementarity with bases 2–7 of the miRNA. The longer the region of complementarity between the miRNA and the target, the more effective the binding: indeed, 8mer sites show the highest binding affinity, followed by 7mer and 6mer sites [34]. The presence of an A in the opposite position to the first nucleotide of the miRNA is responsible for the recognition by the Argonaute (AGO) proteins, while the nucleotide present on the miRNA is irrelevant [35]. Importantly, it has been reported that miRNA levels may be also reduced after miRNA-target binding when the interaction involves the 3’-end of the miRNA [36].
It is now known that MREs are also present within the sequence of other transcripts besides mRNAs, including lncRNAs, circRNAs, transcribed pseudogenes, etc, and that the binding of the miRNA to these targets follows the same rules [20]. However, different from mRNAs, these transcripts generally do not undergo translation, even if the production of short peptides from ncRNAs has been recently reported [37]. Also, MREs within an mRNA are not limited to the 3’-UTR, where they induce the repressive mechanism previously described, but are also localized within the 5’-UTR and the coding sequence [38]. Which is the function of these MREs located in untranslated transcripts or outside the 3’-UTR of an mRNA? Nowadays, these MREs acquire a new function if the ceRNA network theory is considered. It has been suggested that these MREs may play an important role in the regulation of cytoplasmic availability of miRNAs: that means an mRNA/ncRNA (acting as ceRNA or sponge) may “sequester” a specific miRNA by binding it throughout its sequence, thus impairing the negative regulation induced by this miRNA on other mRNAs/ncRNAs [25]. This sponging function is influenced by several factors, such as the number of MREs, the type of miRNA target site, and, accordingly, the strength of the interaction, the abundance of both the ceRNA molecule and the miRNA; needless to say, the colocalization in the same subcellular compartment is required for the interaction, as discussed below [23, 24, 39].
An important recent discovery about miRNAs must be taken into consideration: indeed, different isoforms of miRNAs have been recently described [40]. These new miRNA isoforms (isomiRs) differ from the canonical miRNA, which is the isoform whose sequence is reported in the databases; in particular, isomiRs can show different lengths at the 5’-end (5’ isomiRs) or 3’-end (3’ isomiRs), or maintain the same length but include different bases in their sequence (polymorphic isomiRs); isomiRs characterized by differences in both sequence and length have also been described (mixed type isomiRs) [41]. These variations in length and sequence affect target recognition and play a crucial role in ceRNA networks [42], which must be investigated. It is interesting to note that the majority of canonical miRNAs listed in the miRBase database (www.mirbase.org) is not the most expressed isoform in cells, leading to misclassification of miRNAs dramatically affecting target prediction and ceRNA network reconstruction [27, 43].
The effectiveness of sponging miRNAs to modulate their function has been experimentally investigated back in 2007 by independent groups. The authors showed that the expression of artificial miRNA sponges was able to inhibit miRNA functions on target genes, giving the researcher the possibility to purposely modulate gene expression [44–46]. The expression of artificial sponges was induced by vectors (adenoviral or lentiviral) producing, under the control of a strong promoter, transcripts characterized by numerous MREs; both in vitro and in vivo, these sponges showed an efficiency similar to antisense oligonucleotides (ASOs) in repressing miRNA function [46–50]. Importantly, these molecular sponges can be constructed to bind and sequester single or multiple miRNA species, according to the MREs included [46, 48]. During these studies, it was surprisingly discovered that the presence of a central mismatch within the region of interaction increased the sponging efficiency compared to a perfectly complementary interaction [46–49, 51, 52]. This may sound atypical, considering that perfect complementarity should induce a stronger interaction; but it must be taken into consideration that, according to miRNA function, a perfect complementarity would induce the degradation of the sponge, causing a reduction of its levels which would impair its sponging function. On the contrary, the presence of a mismatch may delay or abolish the degradation of the sponge, resulting in a higher sponging efficiency [47, 51].
In the same period, the miRNA sponge mechanism was also demonstrated in nature, with the first evidence coming from plants [53]. Franco-Zorrilla et al. [53] investigated the relationship between the non-protein coding gene induced by phosphate starvation1 (IPS1) and miR-399, both induced by phosphate starvation, in Arabidopsis thaliana. The IPS1 transcript includes within its sequence an MRE for miR-399 with imperfect complementarity because of a mismatch in the middle of it. This imperfect interaction between the miRNA and the lncRNA results in the suppression of miRNA-mediated degradation and makes IPS1 a sponge for miR-399. Accordingly, the authors reported that upregulation of the sponge IPS1 caused reduced availability of miR-399 and induced the upregulation of its target phosphate 2 (PHO2). Also, the expression of a mutant variant of IPS1 showing perfect complementarity with miR-399 was reduced after miRNA overexpression, confirming that perfect complementarity at the MRE induced the degradation of IPS1, as expected according to miRNA function and congruently to evidence on artificial sponges previously discussed. The authors coined the expression “target mimicry” to describe the sponging function regulating miRNA activity [53]. This non-canonical binding of miRNAs and targets is more common than expected. Indeed, in 2013, Helwak et al. [54] analyzed the human miRNA interactome. Results showed that most of the miRNA-target interactions involved the seed region, even if interactions occurring at the 3’-end of the miRNA were also observed; importantly, about 60% of all analyzed interactions were not perfect, containing mismatches [54]. Unfortunately, this evidence represents bad news. Indeed, if non-canonical miRNA-target interactions are common, that suggests that finding the actual targets of miRNAs may be difficult. Several algorithms and tools are available online to predict which targets are regulated by a given miRNA, but the rules underlying the recognition of the target are not well understood yet. Predictions of miRNA targets are based on: i) complementarity with the seed region; ii) thermodynamic stability, evaluated by calculating the free energy of the predicted interaction (the lower the free energy, the stronger the interaction); iii) evolutionary conservation of the MRE, based on the assumption that a functional interaction is maintained across species; iv) accessibility on the target transcript of the MRE, which must not be involved in intramolecular interactions creating secondary structures; v) number and localization of MREs within the target sequence, since the presence of numerous MRE enhances miRNA functions and MREs very close to each other would compete for the interaction with the miRNA. Most of the tools consider more than one of the described parameters [35].
The miRNA sponge function has been observed not only in eukaryotes but also in viruses and prokaryotes, suggesting that it represents an important regulative mechanism in cell physiology. In 2010, it has been reported that Herpesvirus saimiri, a primate virus, expresses a non-coding transcript able to control gene expression of the host cell by the ceRNA mechanism [55]. Indeed, when the virus infected T cells, seven Herpesvirus saimiri U-rich noncoding RNAs (HSURs) were transcribed from the viral genome; by analyzing their sequences, the authors observed in HSUR1 and HSUR2 the presence of MREs specific for three miRNAs expressed in T cells, namely miR-142-3p, miR-27, and miR-16. By co-immunoprecipitation, it was proved that HSUR1 and HSUR2 effectively bound these miRNAs. Infection of T cells with mutant viruses lacking HSUR1 and HSUR2 showed that miR-27a and miR-27b levels were strongly reduced by the presence of HSUR1, bearing MREs for these miRNAs. The authors demonstrated that the reduction of miR-27a and miR-27b levels is due to the rapid degradation of the miRNAs induced by HSUR1 expression, while this effect was not observed for miR-16 and its predicted ceRNA HSUR2. Reduction of miR-27a and miR-27b levels increased the expression of their target forkhead box O1 (FOXO1), thus allowing the virus to control gene expression of the host cell. Importantly, mutations of the MRE within HSUR1 abolished miRNA degradation [55]. Among prokaryotes, the widespread existence of regulatory RNAs has been well-known for a long time. Indeed, RNA molecules are able to interact with proteins and other transcripts to modulate cell phenotype: antisense small RNAs (sRNAs) bind the target mRNA with base-pairing of 65 or more nucleotides to modulate mRNA stability or translation, while other prokaryotic sRNAs exert functions similar to miRNAs in eukaryotes [56].
Conclusively, the fundamental role of miRNAs as negative regulators of gene expression is not only confirmed but also strengthened and widened into a more complex scenario, where multiple interactors competing for miRNA binding contribute all together to the modulation of cell phenotype.
Protein-coding genes
The ceRNA hypothesis represents by itself a revolution in gene expression regulation, but one of the most intriguing aspects of this new perspective is the additional function conferred to mRNAs. Before the ceRNA hypothesis, mRNAs were considered exclusively as the manual of instruction for protein synthesis, carrying the information for the aminoacidic sequence of a functional protein. In light of this new complex scenario of competing RRIs, mRNAs became regulators able to modulate the gene expression of other mRNAs at the post-transcriptional level. That means an mRNA may exert its function even if it is not translated into a protein. Intriguingly, the non-coding function of the mRNA may be opposite to the function performed by the corresponding protein [25]. In addition, new effects must be taken into consideration when an mRNA is strongly up- or downregulated: dramatically increased levels of an mRNA would massively sequester miRNAs, thus freeing other transcripts from miRNA-mediated negative regulation; on the contrary, a strong reduction of mRNA levels would make a high number of miRNA molecules available for interaction with other mRNAs [20].
Once again, the researchers have to face a very intricate landscape. The human genome contains about 20,000 protein-coding genes [9], but, because of alternative splicing patterns, the total number of mRNAs potentially transcribed is higher. As already discussed, each one of these mRNAs, expressed in a given cell type in a specific moment, includes within its sequence numerous MREs for different miRNAs, which in turn may be expressed or not expressed in the same cell or moment or specific developmental stage. The potential co-expression (or lack of co-expression) of mRNAs and miRNAs adds a new layer of complexity and tunability to the fine mechanism of regulation represented by ceRNA networks, making the system flexible and able to quickly answer to new stimuli.
The non-coding function of mRNAs was described by Tay et al. [57] in 2011 by investigating the expression of the well-known tumor suppressor gene phosphatase and tensin homolog (PTEN). The authors applied the mutually targeted MRE enrichment (MuTaME) approach, a combination of computational analysis and experimental validation, to identify mRNAs sharing MREs with PTEN mRNA; in particular, the ceRNA transcripts included at least seven out of ten MREs present in the 3’-UTR of PTEN. This approach led to the identification of several ceRNAs, among which CCR4-NOT transcription complex subunit 6 like (CNOT6L) and VAMP associated protein A (VAPA) were validated as PTEN regulators. Indeed, siRNA-mediated downregulation of both ceRNAs induced a reduction of PTEN protein expression; a less significant reduction of PTEN mRNA levels was also observed. It was also demonstrated that this decreased expression of PTEN was mediated by miRNAs by using a dicer 1, ribonuclease III (DICER1)-mutant cell line, in which miRNA processing was impaired and PTEN downregulation was consequently reduced. The effective instauration of this ceRNA circuit in vivo is sustained by the correlation of expression among PTEN and its putative ceRNAs observed in vivo. Importantly, this ceRNA mechanism resulted in the regulation of cell proliferation, thus impacting cancer growth [57]. The same group reported other ceRNAs for PTEN identified by using a similar approach in melanoma [58]. ZEB2, involved in the epithelial to mesenchymal transition (EMT), was shown to regulate PTEN expression acting as ceRNA thanks to its 3’-UTR, able to sequester and suppress the function of miRNAs targeting PTEN. This represents an interesting example of an mRNA showing two opposite coding and non-coding functions: as a protein, ZEB2 activates EMT and thus fosters metastatization, while as an mRNA it sustains the expression of a tumor suppressor gene, namely PTEN, through ceRNA interactions [58]. In the same period, similar evidence was published by Sumazin et al. [59], who also focused on PTEN regulation of expression in glioblastoma. In this study, a different approach named Hermes, based on the modulator inference by network dynamics (MINDy) algorithm, was applied to identify potential ceRNAs of PTEN. Results again showed that the 3’-UTR of these ceRNAs was implicated in the regulative mechanism [59]. Another interesting example is represented by tyrosinase related protein 1 (TYRP1) mRNA-mediated sponging of miR-16 in melanoma, which occurs with non-canonical binding [60]. miR-16 sequestration fostered tumor progression by de-repressing RAB17, member RAS oncogene family (RAB17). A therapeutic approach using ASOs to impede the interaction between miR-16 and TYRP1 by masking its MRE led to increased RAB17 levels and apoptotic rate, also decreasing cell proliferation [60]. Collectively, this evidence suggests that competing interactions play a crucial role in carcinogenesis and may be considered targets for new therapeutic approaches.
As discussed, the most important region of the mRNAs driving miRNA-mediated regulation is the 3’-UTR, which acts in cis by regulating the stability of the transcript it belongs to. Given the non-coding function of mRNAs within ceRNA networks, the 3’-UTR becomes a trans-acting regulator by titrating miRNA availability and indirectly regulating the expression of other mRNAs sharing MREs for the same miRNAs [25]. This function in trans is confirmed by the reports discussed above in addition to many others where the 3’-UTR of a given ceRNA is ectopically expressed alone, independently from the mRNA to which it belongs. In particular, Lee et al. [61] investigated the role of the 3’-UTR of versican (VCAN) in regulating miRNA function. VCAN is a component of the extracellular matrix (ECM) involved in cancer progression through the regulation of cell adhesion and migration, angiogenesis, and other processes [62]. Ectopic expression of this 3’-UTR in a breast cancer cell line reduced cell proliferation in vitro and tumor growth in vivo by sponging miR-144, miR-199a-3p, and miR-136, miRNAs also targeting the tumor suppressor genes RB transcriptional corepressor 1 (RB1) and PTEN [61]. The same group focused on the role of VCAN 3’-UTR in the onset and progression of hepatocellular carcinoma (HCC) [63]. Enforced expression of the 3’-UTR of VCAN led to HCC onset in vivo; in vitro, VCAN 3’-UTR induced increased proliferation, migration, and invasion, while decreasing apoptosis rate. In vitro assays showed that this tumor-promoting effect was due to the miRNA sponging performed by VCAN 3’-UTR on miR-133a, miR-199a*, miR-144, and miR-431; this sponging function protected from miRNA-mediated silencing VCAN mRNA itself, but also the transcripts coding fibronectin 1 (FN1) and CD34 molecule (CD34) [63]. The same group also investigated the role of the 3’-UTR of [CD44 molecule (Indian blood group)] (CD44) in breast cancer [64, 65]. Overexpression of CD44 3’-UTR reduced cell proliferation and promoted apoptosis by sequestering miR-216a, miR-330, and miR-608, thus increasing protein levels of CD44 itself and cell division cycle 42 (CDC42), targeted by the same miRNAs [64]. Shortly after, expression of CD44 3’-UTR was reported to increase cell migration and invasion in vitro and promote metastasis in vivo by miRNA-mediated de-repression of collagen type I alpha 1 chain (COL1A1) and FN1, achieved by sequestering miR-512-3p, miR-328, miR-491 and miR-671 [65]. Considering this evidence all together, FN1 may represent a link between the ceRNA networks centered on CD44 and VCAN, regulating the expression of genes crucial for cell proliferation such as CDC42, PTEN, and RB1, all participating in tumorigenesis [24].
The discovery of this sponge function performed by 3’-UTRs becomes very important in carcinogenesis. Indeed, the phenomenon of 3’-UTR shortening has been observed in several cancer models [66]. Mayr and Bartel [66] reported that aberrant production of several oncogenic proteins may occur in cancer also in absence of genetic alterations. The reason for increased protein levels often lies in the presence of a shorter 3’-UTR, because of the phenomenon of alternative cleavage and polyadenylation (APA), which abolishes several MREs. These shorter 3’-UTRs promote carcinogenesis, as demonstrated for the proto-oncogene insulin like growth factor 2 mRNA binding protein 1 (IGF2BP1). Similarly, different splicing variants frequently show distinct 3’-UTRs; that means the regulation of alternative splicing patterns may affect competing interactions and modulate phenotype [67].
Transcribed pseudogenes
Pseudogenes are copies of a parental gene that, unlike the latter, do not undergo translation. The comparison between pseudogenes and their coding counterparts shows that the abolishment of the coding ability of these gene copies is due to the loss of control regions or the accumulation of several genetic alterations acquired over time, such as the formation of premature stop codons or frameshift insertion/deletion mutations. Indeed, pseudogenes were considered “genomic fossils” [20, 39]. According to their generation, pseudogenes can be classified into: i) unprocessed pseudogenes, originated from a duplication of protein-coding genes, usually maintain 5’-UTR, 3’-UTR, and introns within their sequence; ii) processed pseudogenes, generated by retrotransposition of an mRNA transcribed from a protein-coding gene, do not include introns but may maintain 5’-UTR and 3’-UTR; iii) unitary pseudogenes, deriving from unduplicated protein-coding genes which accumulated mutations and lost their protein-coding capability, usually do not show protein-coding counterparts in the same genome but have protein-coding orthologs in other species. It was estimated that the human genome overall includes more than 14,000 pseudogenes [68].
Albeit pseudogenes have been long considered part of the so-called “junk DNA”, lacking function, next-generation sequencing experiments showed that pseudogenes are often conserved and actively transcribed (and in some cases also translated into proteins [69]), suggesting that they do exert functions and are subject to selective pressure [39, 70]. Indeed, a function for a few pseudogenes has been recently reported in the context of ceRNA networks, where they act as miRNA sponges regulating the expression of the parental gene. This function is due to the high number of MREs shared with the parental gene thanks to the sharing of the same 3’-UTR and the high sequence homology [19].
The first pseudogene reported as ceRNA is phosphatase and tensin homolog pseudogene 1 (PTENP1), a processed pseudogene able to regulate the expression of its parental gene PTEN [71]. Given the involvement of PTEN in carcinogenesis as a tumor suppressor, PTENP1 plays a crucial role in several cancer models; indeed, PTEN is frequently lost or mutationally inactivated in cancer cells. PTENP1 and PTEN show very high sequence complementarity, with only 18 mismatches, but a mutation abolishing the AUG start codon prevents the translation of the pseudogene. PTENP1 shows a 3’-UTR shorter compared to PTEN, with a difference of about 1 kilobase (kb); nevertheless, MREs for miR-17, miR-21, miR-214, miR-19, and miR-26 families are shared between the two transcripts. It has been reported that PTENP1 sponged these miRNAs, thus protecting PTEN from miRNA-mediated repression at both mRNA and protein levels. In vitro assays showed that silencing of PTENP1 promoted cell proliferation, supporting the involvement of this pseudogene in carcinogenesis as a tumor suppressor. Accordingly, PTENP1 is also frequently lost in cancer and shows a correlation of expression with PTEN, suggesting that the pseudogene regulates the expression of the parental gene also in vivo [71]. PTENP1 represents a particular example in ceRNA networks because it also encodes a lncRNA participating in PTEN regulation (see below [72]). The function of regulator of parental gene expression was also reported for other pseudogenes, including the oncogenes KRAS proto-oncogene, GTPase pseudogene 1 (KRASP1) for KRAS proto-oncogene, GTPase (KRAS) [71] and catenin alpha 1 pseudogene 1 (CTNNAP1) for catenin alpha 1 (CTNNA) [73], or the tumor suppressors TUSC2 pseudogene 1 (TUSC2P1) for tumor suppressor 2, mitochondrial calcium regulator (TUSC2) [74] and integrator complex subunit 6 pseudogene 1 (INTS6P1) for integrator complex subunit 6 (INTS6) [75]. All these pseudogenes originated from parental genes involved in carcinogenesis, supporting the hypothesis that competing interactions are crucial for cancer onset and progression.
lncRNAs
lncRNAs are non-coding RNA molecules characterized by a length comprised between 200 nts and kbs; they are similar to mRNAs concerning biogenesis, being often transcribed by RNA polymerase II, capped, polyadenylated, and spliced [76]. The total number of genes encoding lncRNAs in the human genome has been estimated between 10,000 and 100,000 [77], representing a conspicuous portion of it. Similar to pseudogenes, it has been proposed that lncRNA genes derived from duplication events occurring at both DNA and RNA levels, insertion of transposable elements, or mutational inactivation of protein-coding genes. lncRNAs are poorly conserved during evolution; accordingly, more than 80% of lncRNA families originated in primates [78]. Typically, lncRNAs are mostly localized in the nucleus and poorly expressed compared to mRNAs [79]. For these reasons, lncRNAs have been long considered non-functional, but the observation of expression and localization patterns related to specific tissues or developmental stages, at least for a fraction of them, has suggested that they do exert functions within cells [77, 78]. To date, the exact function or mechanism of action of only a few lncRNAs has been demonstrated, showing that a high heterogeneity in functions is characteristic of this class of RNA molecules. Described functions include epigenetic regulation (such as chromatin remodeling), RNA or protein localization, regulation of transcriptional and post-transcriptional events (including mRNA stability, splicing, and editing), and structural functions (scaffolding) [77, 80]. In the last decade, one of the most investigated functions performed by lncRNAs has been represented by miRNA sponging, whose involvement both in neoplastic and non-neoplastic diseases has been demonstrated [81–83].
One of the first reports describing a ceRNA function for lncRNAs was published in 2010 [84]. Highly up-regulated in liver cancer (HULC) increased levels in HCC caused the decreased availability of miR-372, resulting in the upregulation of one of its targets, namely protein kinase cAMP-activated catalytic subunit beta (PRKACB). PRKACB in turn regulated the phosphorylation of cAMP responsive element binding protein 1 (CREB1), responsible for HULC transcription. Therefore, this lncRNA regulates its expression through miRNA sponging creating an auto-regulatory loop [84]. Shortly after, Cesana et al. [85] showed that the lncRNA long intergenic non-protein coding RNA, muscle differentiation 1 (LINCMD1) participates in muscle differentiation both in mouse and human. By sponging miR-133 and miR-135 in myoblasts, LINCMD1 regulated the levels of mastermind like transcriptional coactivator 1 (MAML1) and myocyte enhancer factor 2C (MEF2C), two transcription factors (TFs) promoting the expression of muscle-specific genes [85]. HOX transcript antisense RNA (HOTAIR) is a lncRNA acting as an oncogene in several cancer models [86]. In gastric cancer, this oncogenic function may be in part exerted by the sequestration of miR-331-3p, resulting in the modulation of its target erb-b2 receptor tyrosine kinase 2 (HER2/ERBB2) [87]. To date, the list of reports about lncRNAs acting as miRNA sponges is constantly growing [34], supporting the fundamental role of such a mechanism within cells.
Besides sponging miRNAs, lncRNAs are able to regulate gene expression through other mechanisms. In particular, a specific subclass of lncRNAs includes NATs, involved in the regulation of the sense protein-coding transcript. As an example, ZEB2-AS1 regulates the splicing of the sense transcript ZEB2 in epithelial cells [30]. ZEB2 acts as a transcriptional repressor of cadherin 1 (CDH1) and is upregulated when EMT is activated. One of the TFs triggering EMT is snail family transcriptional repressor 1 (SNAI1); intriguingly, Beltran et al. [30] showed that SNAI1 did not promote ZEB2 transcription, while it indirectly modulated ZEB2 splicing through its NAT ZEB2-AS1, expressed under the control of an alternative SNAI1 promoter. ZEB2-AS1 interacted by sequence complementarity with ZEB2 mRNA, thus preventing the binding of the spliceosome and the elimination of an intron including an internal ribosome entry site (IRES), responsible for ZEB2 translation. This example shows that RRIs not involving miRNAs are also crucial for gene expression regulation and modulation of biological processes associated with cell physiology and pathology. However, NATs may also indirectly interfere with miRNA function, supporting again the high complexity and heterogeneity of ceRNA interactions. BACE1-AS is the NAT of BACE1, the enzyme producing the amyloid-β peptide whose accumulation leads to Alzheimer’s disease [88]. BACE1-AS induced upregulation of BACE1 protein by interacting with its mRNA and masking the MRE for miR-485-5p [29]. Similar results were reported in mouse myoblasts for Sirt1-AS, sponging miR-34a and protecting Sirt1 mRNA from miRNA-mediated repression [28]. In these examples, the direct interaction between sense and antisense transcripts, showing intrinsic sequence complementarity, acts as a “protector” from miRNA-mediated repression for the sense mRNA when MREs are located within a region involved in the interaction with the antisense and are thus impeded from miRNA binding, despite the miRNA is not involved in competing interactions.
As previously mentioned, the locus of the pseudogene PTENP1 also encodes a lncRNA, the NAT PTENP1 antisense RNA (PTENP1-AS), acting as a regulator of PTEN expression [72]. In particular, two isoforms of the lncRNA are transcribed, exerting different functions. The α isoform directly regulated PTEN expression acting in trans: by interacting with the promoter of PTEN, this isoform recruited on it DNA methyltransferase 3 alpha (DNMT3A) and enhancer of zeste 2 polycomb repressive complex 2 subunit (EZH2), hence inducing chromatin remodeling. On the contrary, the β isoform directly bound the sense transcript PTENP1, promoting its stability. Indeed, the lack of the polyadenylated tail reported for the PTENP1 transcript determined its instability. That means the β isoform indirectly regulated PTEN expression by stabilizing the PTENP1 transcript, thus increasing its levels and, consequently, reducing the availability of miRNAs able to repress PTEN expression [72]. This regulatory mechanism is extremely complex and fascinating, and the ceRNA network surrounding PTEN is a good illustration of how multiple ceRNA interactions may co-occur.
Another peculiar example concerning lncRNA-mRNA direct interactions reported in 2011 induced mRNA degradation [89]. The RNA-binding protein (RBP) Staufen double-stranded RNA binding protein 1 (STAU1) was reported to recognize double-stranded RNA, leading them to degradation, through a STAU1-binding site included within the 3’-UTR of the target mRNAs, in particular in a 19-base-pair stem [90]. Gong and Maquat [89] reported that not all STAU1-targeted mRNAs presented this stem structure within their 3’-UTR; on the contrary, the double-stranded RNA structure inducing STAU1 binding may be created by the imperfect interaction between an Alu element included within the mRNA 3’-UTR and a cytoplasmic polyadenylated lncRNA also including an Alu element, guiding the interaction with the mRNA.
circRNAs
circRNAs represent an unusual class of lncRNAs, characterized by a circular structure resulting from the covalent binding of the two extremities. circRNAs arise from transcripts coded by protein-coding genes which undergo a particular form of alternative splicing, named backsplicing, where the 5’-site of an upstream exon is bound with the 3’-end of the same or a downstream exon; accordingly, circRNAs are transcribed by RNA polymerase II, but they do not show 5’-cap or 3’-polyadenilation [91, 92]. Because of their structure, circRNAs are resistant to exonucleases and show higher stability compared to linear transcript [92]. circRNAs are abundantly expressed in brain, where they participate in the physiology of the central nervous system [93], albeit being ubiquitously expressed in human tissues [94]. Generally, circRNAs are mostly cytoplasmic and evolutionarily conserved [92, 93] and are transcribed also by viruses, such as SARS-CoV-2 [95]. According to the portions of protein-coding genes included within their sequences, circRNAs can be classified into: exonic circular RNAs (ecircRNAs), circular intronic RNAs (ciRNAs), and exon–intron circRNA (EIciRNA). Recent evidence suggested that ecircRNAs may prevalently act as miRNA sponges, while ciRNAs and EIciRNAs (that is, the species including introns) are localized in the nucleus, where they regulate gene expression [96]. In particular, circRNAs can regulate the expression of the parental gene, acting as cis-regulators, or of independent genes, acting in trans. Several functions have been reported: i) miRNA sponging; ii) protein binding for activation or stabilization of enzymatic activity, promotion of subcellular localization, regulation of translation, epigenetic modifications, ubiquitination; iii) translation of small peptides or proteins [97, 98]. Recently, circRNA-protein interactions are gaining attention, suggesting a role in regulating also competing interactions between RNA molecules and RBPs [99, 100]. To date, more than 140,000 annotated human circRNAs have been included in the database circBank (http://www.circbank.cn/). Several isoforms of various circRNAs deriving from the same protein-coding gene have been reported, making nomenclature very important to identify the specific transcript object of study [101].
One of the most known examples of miRNA-sponging circRNAs is represented by CDR1 antisense RNA (CDR1-AS), also known as circular RNA sponge for miR-7 (ciRS-7), which includes within its sequence 63 MREs for miR-7 [102, 103]. CDR1-AS is transcribed from the same locus of cerebellar degeneration related 1 (CDR1) (of which it represents a circular NAT) and is involved in brain physiology. Recently, the involvement of this circRNA in several diseases, including cancer, has been reported [104]. Another circRNA whose miRNA-sponging function has been widely investigated is circular RNA itchy E3 ubiquitin protein ligase (circ-ITCH), transcribed from the ITCH locus and acting as a tumor suppressor in esophageal squamous cell carcinoma (ESCC), colorectal cancer (CRC) and bladder cancer [105–107]. In ESCC, circ-ITCH sponged miR-7, miR-17, and miR-214, leading to inhibition of the Wnt/β-catenin pathway [105]; the same effect was reported in CRC through the sequestration of miR-7, miR-20a, and miR-214 [106]. In bladder cancer, circ-ITHC downregulation resulted in increased availability of miR-17 and miR-224, in turn inducing repression of the targets PTEN and p21/cyclin dependent kinase inhibitor 1A (CDKN1A) [107]. Multiple evidence about circ-ITCH acting as a sponge in several cancer models supports the hypothesis of a fundamental and conserved role of ceRNA mechanisms in both physiological and pathological conditions.
Interestingly, a study published in 2022 reported that circRNAs may also interact with mRNAs [108], similarly to lncRNAs. The study focused on circular RNA zinc finger protein 609 (circZNF609), encoded from the ZNF609 locus, an oncogenic circRNA upregulated in several cancer models and reported to act as a miRNA sponge. Rossi et al. [108] showed that circZNF609 also interacted in vivo with mRNAs, namely cytoskeleton associated protein 5 (CKAP5), UPF2 regulator of nonsense mediated mRNA decay (UPF2), and serine and arginine repetitive matrix 1 (SRRM1), recruiting on their sequences ELAV like RNA binding protein 1 (ELAVL1), an RBP, in turn, regulating stability and translation of these mRNAs [108]. This new recent finding demonstrates that little is known about the potentiality of ceRNA networks, and further investigations will be needed to increase the knowledge about their mechanisms.
Conditions for ceRNA networks
Looking at ceRNA interactions as a whole, their complexity is undeniable (Figure 1). To understand how a particular mechanism operates within cells, researchers must ignore all other phenomena and concentrate only on a single molecular event. Nonetheless, it’s important to keep in mind that the mechanisms explained here occur in the complex framework presented above. To integrate the small puzzle pieces in the comprehensive context of a living cell, several studies investigated the conditions required for the existence of a ceRNA network. First of all, each component of the ceRNA network is characterized by specific expression levels, which may differ between the single actors involved in the interactions. The abundance of a given miRNA is crucial to understand if it will be able to bind all its potential targets or only a portion of them (most likely the ones with the higher binding affinity); on the contrary, if a ceRNA molecule (such as an mRNA, a pseudogene, a lncRNA or a circRNA) is more abundant than the miRNA, it could be speculated that only a few miRNA molecules (the majority of them being sponged) will be available for target-binding. Accordingly, Denzler et al. [109] demonstrated that the miRNA: target ratio is crucial for competing interaction by investigating miR-122 function in the liver. There are numerous examples of potential scenarios occurring within a cell, but it is easy to understand that the stoichiometric equilibrium among interacting RNAs is one of the most important parameters regulating ceRNA interactions. What seems to be elucidated is that a near equimolar expression among RNA interactors is necessary for ceRNA network establishment. This would allow a small alteration in the expression levels of a given ceRNA to affect the expression of the other interactors. As an example, consider a ceRNA that is highly more abundant than a miRNA, being able to sponge virtually all miRNA molecules: a small variation in ceRNA levels would not produce a significant effect on miRNA availability in the cytoplasm. The nearly equimolar equilibrium was estimated by a mathematical model using PTEN and VAPA interplay as a model of ceRNA interactions [110]. It should be noted that a debate arose regarding the true impact of ceRNA networks on cell biology. Denzler et al. [109] concluded in their study on miR-122 in the liver that variation in expression of a single miRNA family was unlikely to impact both physiology and pathology: indeed, modulation of miR-122 expression was able to regulate the expression of miRNA targets, while ceRNA effects were induced when the concentration of the miRNA reached levels exceeding physiology. Despite this negative result, the authors did not exclude the existence of other unidentified ceRNAs highly expressed within cells nor the possibility that their method was not sensitive enough to detect perturbations of the ceRNA network [109].
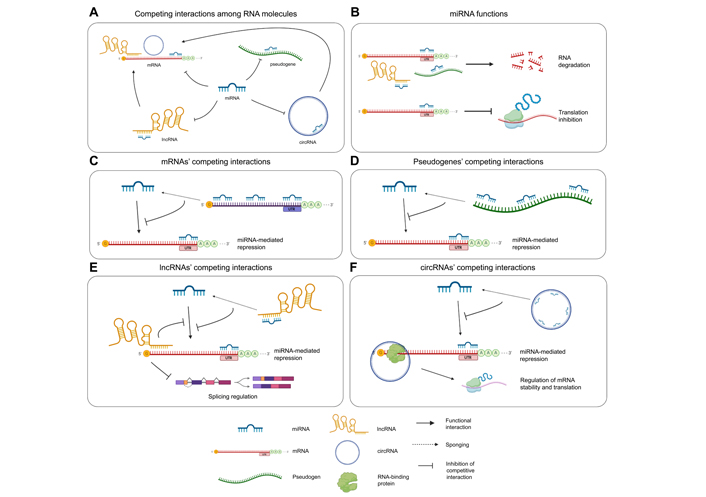
Representation of ceRNA interactions and molecular effects. (A) ceRNA interactions occurring among the involved RNA species: miRNAs bind and negatively regulate mRNAs, pseudogenes, lncRNAs, and circRNAs; lncRNAs and circRNAs bind mRNAs and modulate their splicing or stability; (B) function of miRNAs: miRNAs bind mRNAs, lncRNAs, and pseudogenes with imperfect complementarity and induce target degradation; when a miRNA interacts with its mRNA target with perfect complementarity, translation is inhibited; (C) mRNAs act as miRNA sponges by binding miRNAs through multiple MREs included throughout their sequence, thus protecting other mRNAs from miRNA-mediated repression; (D) pseudogenes can regulate the expression of parental protein-coding genes by sponging miRNAs; high sequence homology between the pseudogene and the parental gene determines the high number of shared MREs; (E) lncRNAs can protect other transcripts from miRNA-mediated repression by sponging miRNAs; direct interactions between lncRNAs and mRNAs modulate the splicing of the latter; (F) circRNAs act as miRNA sponges, impairing their function on other transcripts, or directly interact with mRNAs recruiting on the RBPs involved in splicing or mRNA stabilization
Another undeniable parameter is, as mentioned, the binding affinity between the miRNA and the MRE. The existence of MREs differing in the length of sequence complementarity with miRNA seed region (classified as 8mers, 7mers, and 6mers, see above) suggests that miRNAs may have primary targets, bound with higher affinity and effectively regulated, while other transcripts showing MREs with lower affinity may be less affected by modulation of miRNA availability, or they may act as sponges [25, 26]. Indeed, it has been reported that 7mers and 6mers were respectively 50% and 20% as effective as 8mers in regulating target abundance [111]. Therefore, it can be assumed that low levels of a given miRNA would induce its preferential binding with high-affinity MREs, while binding to low-affinity target sites would require a higher abundance of the miRNA. It could be hypothesized that this binding affinity would also mirror the efficacy of miRNA-mediated repression, suggesting that targets bearing low-affinity MREs would be negatively regulated by the miRNA only whether it is abundantly expressed. Actually, effective binding between miRNAs and low-affinity MREs (and consequential negative regulation of the target) does occur within cells, in some cases with unexpected repression efficiency. This contradiction may be explained by other MRE-related parameters influencing ceRNA networks. First of all, it is important the number of MREs included within the sequence of a given ceRNA: the higher the number of MREs, the more miRNA molecules sponged. But localization and distribution of MREs are also crucial since neighbor MREs showed to increase the binding affinity by acting in cooperation. Intriguingly, cooperation among low affinity or non-canonical MREs may contribute to target site abundance more than high affinity or canonical binding sites; experimental evidence showed that two closely spaced (58 nts apart) MREs induced a more efficient negative regulation than expected by their independent action [111].
Even in the presence of multiple MREs with comparable affinity, ceRNA interactors have to share the same subcellular localization for the physical interaction to occur. As discussed, lncRNAs (including circRNAs) showed to exert functions both in the nucleus and in the cytoplasm, while mRNAs and miRNAs typically localize in the cytoplasm where they are translated into proteins and act as negative regulators of gene expression, respectively. However, a nuclear function for miRNAs was reported, showing their involvement in regulation of transcription and alternative splicing [112]. The asymmetric distribution would impair a direct interaction. Nevertheless, this potential obstacle may be overcome by the shuttling of miRNAs and lncRNAs between nucleus and cytoplasm that has been demonstrated for some of them [113, 114], thus allowing subcellular colocalization (even if temporary) and direct interaction.
Supposing that ceRNA interactors colocalize within the same subcellular compartment, the regions of each molecule being involved in ceRNA interactions must be free to bind to each other. That means no other interactions should occur, neither intramolecular (formation of secondary structures) nor intermolecular (interactions with either RNAs or proteins). In this context, interactions with RBPs involved in physiological processes (such as splicing, miRNA-induced silencing, etc.) may represent an additional competitor for RNA binding affecting ceRNA interactions [34].
As previously discussed, alternative splicing patterns producing mRNAs differing at the level of the 3’-UTR may contribute to MRE abundance within the cell [67], as well as the phenomenon of 3’-UTR shortening observed in cancer [66]. There is another molecular process, occurring physiologically in cells, that affects RRIs, namely RNA editing. Being part of post-transcriptional processes, RNA editing consists in modifying the sequence of a transcript [including mRNAs, transfer RNAs (tRNAs), ribosomal RNAs (rRNAs), etc] by insertion, deletion, or substitution of specific nucleotides [115]. Interestingly, RNA editing is also responsible for isomiR biogenesis [41]. Adenosine-to-inosine modifications represent the most frequent sequence alterations induced by editing, modulating the function of the modified transcript [116]. Obviously, changes in a transcript sequence would affect ceRNA interactions, potentially disrupting or creating MREs. Similarly, the different patterns of expression of isomiRs, characterized by variation in length or sequence, would affect the affinity to existent MREs or induce a given isomiR to recognize new targets [41]. These observations suggest that ceRNA interactions may be finely regulated even in physiological contexts by the intricate modifications underlying the dynamics and complexity of cell processes. Hence, the dramatic twisting of molecular mechanisms inducing the onset of a disease, such as cancer, which includes accumulation of mutations, alternative splicing, and 3’-UTR shortening, would be able to extremely alter the ceRNA interactions, being simultaneously the cause and the effect of the pathological transformation of diseased cells.
Experimental methods for identification of RRIs
RNA molecules play crucial roles in a variety of biological processes by interacting with other RNAs. The significant achievement in resolving transcriptome-wide RRI networks has been the identification of a massive quantity of RRI data that considerably increased the understanding of the regulatory functions of various RNA classes.
For decades, RRIs have been studied using various biochemical approaches. Low-throughput techniques, before, and high-throughput next-generation sequencing of RNA permitted large-scale experimental detection of ceRNAs by identifying the entire network of endogenous RRIs [34, 117].
For example, using the miRNA pull-down assay, Chan et al. [118] identified ferritin heavy chain 1 (FTH1) and its numerous pseudogenes as targets of oncogenic miR-638 in prostate cancer. The authors observed that the FTH1-pseudogene-miRNA ceRNA network plays a significant role in the onset and progression of prostate cancer via regulating iron homeostasis. Chi et al. [119] performed AGO cross-linking and immunoprecipitation (AGO-CLIP) to detect miRNA-mRNA interactions in mouse brain, and their results indicated that miRNA binding sites may be located in both 3’-UTRs and coding regions [119]. In a study by Nguyen et al. [120], interactions of the lncRNA metastasis associated lung adenocarcinoma transcript 1 (MALAT1) with eukaryotic translation initiation factor 4A2 (EIF4A2), transferrin receptor (TFRC), and solute carrier family 2 member 3 (SLC2A3) mRNAs were successfully validated by antisense RNA pulldown; also, MALAT1 and SLC2A3 association was proved via single-molecule Förster resonance energy transfer (smFRET), confirming the validity of MALAT1 targets discovered by Mapping RNA interactome in vivo (MARIO) technology.
The most common approaches for RRI identifications, including conventional methods and recently developed large-scale sequencing-based techniques, are summarized below.
Low-throughput biophysical, biochemical, and cellular methods for detecting RRIs
Electrophoretic mobility shift assay
In electrophoretic mobility shift assay (EMSA), RNA fragments are extracted from cells or synthesized according to the potentially interacting regions. This method is based on the principle of change in RNA mobility as a result of its association with other biomolecules, including other RNAs [121, 122]. Radiolabeled RNA probes are commonly used in RNA EMSA. To evaluate the shift in mobility of the labeled RNA-target complex, the labeled RNA probe is incubated with many target RNA molecules before separation on a non-denaturing polyacrylamide gel. Direct interaction of RNA with another ligand leads to retarded RNA mobility in the agarose or polyacrylamide gel due to the increase in mass/size of the complex. Numerous researchers have used this method to investigate RRIs, and a modified EMSA using biotin-labeled RNA probes has been created for the colorimetric detection of gel-shift bands [123].
Surface plasmon resonance
Surface plasmon resonance (SPR) is a common optical approach for detecting biomolecule interactions in which the target is mobile, while a probe (RNA-ligand) is immobilized on a sensor chip and the interaction with possible targets is analyzed in real-time [124, 125]. RNA ligands can be fixed to the chip surface for RRI evaluation using one of three following methods: bio-affinity, physical adsorption, or covalent cross-linking. The target RNA molecule is then allowed to flow over the chip and interact with the RNA-ligand; the interaction is optically detected by measuring the signal resulting from a shift in refractive index at the chip’s surface. Given SPR’s strength, it might be used to find interactions between RNAs and other RNAs, peptides, proteins, or other biomolecules [125].
smFRET
Another biophysical method for determining energy transfer between two or more fluorophores is Förster resonance energy transfer (FRET) [126]. When used at the molecular level, this technique is known as smFRET and involves the excitation of donor and acceptor fluorophores. This non-radioactive technique needs the excitation of a donor fluorophore, which transfers its excitation energy to its acceptor fluorophore partner, resulting in specific fluorescence emission [127]. RNA molecules are anchored to the surface of quartz or enclosed in lipid vesicles during RRI investigations. Moreover, real-time monitoring can be performed using two fluorescent dyes labeled on precise positions of the two RNA interactors. Correct fluorescence signals are produced and recorded when two fluorescent dyes interact in a close area [128].
Co-sedimentation assay
In RNA co-sedimentation assays, RNA molecules are separated on sucrose/glycerol gradients, and the positions of distinct RNA molecules within the gradient are analyzed by northern blotting [129]. According to the desired resolution and study, a variable proportion of sucrose/glycerol gradient is used as a supporting and stabilizing medium, permitting centrifugal migration of the RNAs and the associated proteins. The existence of two RNA molecules at a specific fraction in the sucrose gradient fractions implies their potential interaction [129].
Single-molecule fluorescent in situ hybridization
Single-molecule fluorescent in situ hybridization (smFISH) uses multiple fluorescent-labeled probes that hybridize with the target RNA in a fixed cell, allowing for the quantification and localization of individual RNA molecules using fluorescence microscopy [130]. Because of its high sensitivity, it has great potential for studying mRNAs and ncRNAs at the single-molecule level. smFISH has been successfully used to investigate RRIs and co-localization of several RNAs, including the lncRNAs nuclear paraspeckle assembly transcript 1 (NEAT1) and MALAT1, making it an interesting tool for exploring RRIs in the cellular context [129, 131].
Luciferase reporter assay
The luciferase reporter assay is a functional assay used to explore intermolecular interactions. Traditionally, it involves two constructs transfected into a cell: one construct contains the gene of interest combined with the gene encoding the luciferase enzyme, while the other includes the protein of interest [132]. The transfected cells are lysed, then luciferin is added as a substrate to evaluate luciferase activity. Although this assay was initially created to examine protein-mediated transcriptional regulation of genes, more recent studies have modified it to examine post-transcriptional regulation via interactions between miRNAs and mRNAs [133]. A particular dual-fluorescence assay system using constructs expressing mCherry with the miRNA target sequence was developed in 2012: this assay shows several advantages, including the possibility to use a larger sample size, and time-course investigations, and can be used for high-throughput approaches, making it a reliable method for studying RRIs [129].
High-throughput targeted methods for detecting RRIs
Next-generation sequencing technologies have been developed to study RNA molecules at the transcriptome level [136].
Cross-linking, ligation, and sequencing of hybrids
Cross-linking, ligation, and sequencing of hybrids (CLASH) is the first high-throughput RNA-sequencing (RNA-seq) technique adapted from cross-linking and immunoprecipitation (CLIP-seq) to decode transcriptome-wide analysis of RRIs [137]. CLASH involves ultraviolet (UV) cross-linking of RNAs associated with proteins, followed by protein purification and partial RNA digestion, and the extremities of the RNA-RNA hybrid are ligated together. Following linker addition, complementary DNA (cDNA) library preparation and high throughput sequencing of the ligated hybrid provide information about chimeric sequences, which can be analyzed to identify RNA-RNA interacting sites [129]. CLASH has been used to identify many types of RRIs in various species, including sRNA-centered interactions in bacteria, snoRNA-rRNA interactions in yeast [137], and miRNA-mRNA interactions in humans [138, 139].
RNA antisense purification
RNA antisense purification (RAP) is a biochemical method used to investigate the interaction of a given lncRNA with its target. This method uses biotinylated antisense probes that are complementary to the target lncRNA and hybridize with it to capture the interacting RNA targets [140]. The biotinylated antisense probe and its interacting RNAs are subsequently isolated and detected by RNA-seq and quantitative polymerase chain reaction (PCR). Engreitz et al. [141] used the RAP method to prove that MALAT1 interacted with various pre-mRNAs and chromatin after 4’-aminomethyltrioxsalen (AMT) cross-linking, purification with an antisense probe, and sequencing [141]. Another example is the study by Panda et al. [142], in which RAP assay was applied to validate the circRNA interactions with miRNAs using ASOs targeting the back-splice junction of circRNAs.
AGO-CLIP
As previously discussed, the miRNAs incorporated within the RISC containing AGO proteins target the 3’-UTR of mRNAs and suppress mRNA translation [38]. Because miRNA-mRNA complex interacts directly with AGO proteins, AGO-CLIP followed by sequencing is aimed at finding transcriptome-wide miRNA targets [143]. UV cross-linking is used in this procedure to establish protein-RNA interaction. The cellular lysate is therefore treated with ribonuclease (RNase) before immunoprecipitation of AGO proteins (by using a specific antibody) to produce RNA fragments of 20–100 nts. The fragmented RNA is then subjected to alkaline phosphatase treatment and radio-labeled by γ-32P-ATP using T4 polynucleotide kinase (PNK), and the protein–RNA complex is then separated in sodium dodecyl sulfate-polyacrylamide gel electrophoresis (SDS-PAGE) Following that, the gel is observed by autoradiography to excise out the complex-corresponding portion, which is then treated with proteinase K to release the immunoprecipitated RNA fragments [143]. AGO-CLIP was used in some studies to detect miRNA interactions in pathological conditions such as ischemic stroke and cancer [144].
RNA hybrid and individual-nucleotide resolution ultraviolet cross-linking and immunoprecipitation
RNA hybrid and individual-nucleotide resolution ultraviolet cross-linking and immunoprecipitation (HiCLIP) is another advanced technique for detecting intra- and intermolecular RRIs by using the RBP STAU1 [145]. HiCLIP, like CLASH, starts with UV crosslinking of RNAs to proteins, followed by lysis and RNA digestion by RNase I. STAU1 is subsequently co-immunoprecipitated with its associated RNAs, but unlike CLASH, HiCLIP achieves better specificity by adding an adaptor in ligation: cloning adapter and linker adapters are ligated to both strands of the RNA duplex. The linker adaptor is removed to ligate the RNA strands flanking to the linker, which can result in either a duplex formed by the same mRNA or two distinct mRNAs; the RNA-RNA hybrid is subsequently converted to a cDNA library and sequenced [145]. HiCLIP has been used to decode RNA secondary structure bound by STAU1, but, in principle, it can also identify inter-molecular RRIs [145, 146].
RNA walk
The RNA walk approach, like CLASH, uses AMT-induced UV cross-linking and affinity purification, but rather than ligation, RNA walk performs reverse transcriptase-PCR (RT-PCR) immediately after crosslinking, with primers designed to target distinct portions of the investigated RNA [147].
Identification of transcriptome-wide RRIs
The development of modern biochemical methods and high-throughput sequencing technology resulted in the discovery of ncRNAs, a novel family of RNAs, and the various functions exerted through interactions with other RNAs. Several technologies using biochemical RNA cross-linking followed by high-throughput sequencing have enabled us to discover the global map of RRIs crucial for physiological activities in recent years. The transcriptome-wide RNA interactome, which has the potential to include all of the RNAs in a cell, leads to the development of several novel techniques.
MARIO
MARIO is an in vivo enzyme-based method for detecting protein-assisted RNA interactions and structures allowed by proteins-RNA cross-linking [120]. Using this approach, RNA interactions were mapped in mouse embryonic stem cells, fibroblast cells, and brain cells. To capture RNA linked via protein, this technique performs RNA cross-linking using formaldehyde and ethylglycol bis (succinimidyl succinate). The biotinylated RNA linkers are subsequently coupled to the protein-bound proximal ends of RNAs, resulting in the formation of an RNA1-linker-RNA2 chimeric form of RNA. The linker-mediated chimeric RNA is subsequently isolated using streptavidin-coated magnetic beads and successively used for the construction of a sequencing library. As a result, the hybrid’s paired-end reads reflect the interaction between RNAs [129]. Furthermore, the MARIO technique can detect in vivo RNA structure, including the proximal spatial organization of secondary and tertiary structures. For the first time, thousands of endogenous RNA connections in mouse embryonic stem cells and brain cells were discovered using this technology. Moreover, MALAT1 interactions with ergosterol biosynthesis 28 (ERG28), EIF4A2, TFRC, and SLC2A3 mRNAs were successfully validated by antisense RNA pulldown; MALAT1 and SLC2A3 association was confirmed via smFISH, thus confirming the validity of MALAT1 targets discovered by MARIO [120].
Sequencing of psoralen cross-linked, ligated, and selected hybrids
Sequencing of psoralen cross-linked, ligated, and selected hybrids (SPLASH) is another high-throughput approach used to study transcriptome-wide intra- and inter-molecular RRIs [148]. This method relies on RNA-RNA cross-linking using biotin-labeled psoralen, RNA isolation, fragmentation followed by pulldown of biotin-labeled psoralen, and the ligation of proximal ends of psoralen cross-linked RNA hybrids. After that, the RNA-hybrids are reversely cross-linked. The biotin group on bio-psoralen also allows the selective enrichment of crosslinked interaction sites during the library preparation process, increasing the signal of pairwise interactions over the background of non-interacting sites. In this method, psoralen swiftly permeates cells and selectively cross-links at pyrimidines, making this approach highly specific for RRIs. This technique was used for in vivo mapping of RRIs in eukaryotes, leading to the identification of intermolecular interactions, including mRNA-rRNA, mRNA-mRNA, snoRNA-rRNA, and mRNA-lncRNA interactions [129, 148].
Psoralen analysis of RNA interactions and structures
The psoralen analysis of RNA interactions and structures (PARIS) method enables genome-wide determination of RRIs and RNA structures [149]. This method involves cross-linking RNA with AMT (a psoralen derivative) after UV irradiation at 365 nm. Total RNA is isolated and partially digested using RNase, followed by two-dimensional electrophoresis to purify interacting RNAs, and finally proximity ligation [129]. The RNA hybrid is then reversely cross-linked by UV irradiation at 254 nm and used for library preparation, followed by high-throughput sequencing and bioinformatics analysis [149]. This method was used to discover long-range structures originating from intramolecular base-pairing at near base-pair resolution, in addition to intermolecular RRIs across the transcriptome. For example, lncRNA X inactive specific transcript (XIST) was found to fold into conserved RNA structures by PARIS analysis [129]. Recently, an improved version of PARIS technology, namely PARIS2, has been developed to detect in vivo RNA interactome with more than 4,000-fold higher efficiency [150].
Ligation of interacting RNA followed by high-throughput sequencing
Ligation of interacting RNA followed by high-throughput sequencing (LIGR-seq) was developed to show the complex RNA-RNA interactome in human cells, facilitating the detection of multiple RRIs, particularly those involving mRNAs. LIGR-seq performs cross-linking by AMT and UV irradiation, resulting in pyrimidine-mediated interlinked RNA hybrids [129]. Cells are then lysed to isolate RNA and highly abundant rRNAs are removed via rRNA depletion. Moreover, S1 nuclease is used to remove single-stranded RNA, and the free overhangs next to the duplexes are ligated with circRNA ligase. RNase R, an exoribonuclease that digests linear or uncross-linked RNAs, is used to digest the AMT cross-linked RNA. After reverse crosslinking at UV irradiation of the enriched duplexes at 254 nm, library preparation, and high throughput sequencing are performed [129, 151]. Unexpected and previously unknown interactions were observed between snoRNAs and mRNAs, including NOP14 nucleolar protein (NOP14), ribosomal protein S5 (RPS5), and serine and arginine rich splicing factor 3 (SRSF3), indicating that small nucleolar RNA, C/D box 83B (SNORD83B) contributes to the steady-state expression of its mRNA targets. Furthermore, parallel libraries from uncross-linked samples can aid to reduce artifacts caused by the library preparation to boost the sensitivity of this method [129].
Parallel analysis of RNA structure
Parallel analysis of RNA structure (PARS) analyses global RNA structures by combining the traditional biochemical method of RNA cleavage with parallel DNA sequencing [152]. This approach was first used to investigate the structure of mRNAs in yeast and their role in gene regulation. Total RNA isolation from cells is performed to enrich mRNAs, which are then treated with S1 or V1 nuclease separately. After that, the obtained small structural fragments are retro-transcribed into double-stranded cDNA libraries. Different libraries are generated from the materials digested by each nuclease. However, only the sites cleaved by RNase V1 or S1 nuclease contain 5’-phosphate that are ligation-competent. The RNA is then size-selected, followed by 5’-adapter ligation, PAGE purification, and PCR amplification [152]. The amplified products are sequenced in the Sequencing by Oligo Ligation Detection (SOLiD) system or Illumina platform resulting in tens of millions of reads. Data analysis allows to identify the site digested by a specific nuclease or by both of them, comparing the cleaving frequencies of both RNases at each nucleotide [129]. This comparison is known as the PARS score, calculated through the formula log2 (V1/S1), which provides the single or double-stranded characteristic of each nucleotide in RNA sequence and provides the basis for the secondary structure of RNA [152]. A positive PARS score is indicative of a double-stranded base, while a negative value indicates a single-stranded base. Mapping the PARS scores to already known motifs of DNA-binding transcription repressor ASH1 (ASH1) and glutathione peroxidase (URE2) mRNAs also confirmed that this technique may decode the structural information of these elements [153].
Parallel analysis of RNA structures with temperature elevation
Parallel analysis of RNA structures with temperature elevation (PARTE) is a modified version of the PARS technique based on a difference in temperature values for the analysis of RRIs. The relative melting temperature (Tm) of RNA structure of 4,000 transcripts was determined by testing the RNA secondary structures (called RNA thermometers) in yeast throughout a temperature range of 23°–75°C. Tm distinguishes mRNA polarity as well as coding, open reading frame (ORFs), and noncoding sections [154]. The PARTE profile reveals that the weakest pairs occur at the start and stop site of mRNAs, making them easily accessible for ribosome binding. Highly stable base-pairing is detected in regions 20 nts upstream of the start codon and 10 nts downstream of the stop codon, showing that the highly ordered structure plays an important role in the translation process [155]. The enriched mRNA samples are allowed to refold before being treated with V1 nuclease. Following Tm estimation and genomic analysis, the RNA fragments are retro-transcribed in cDNA libraries for sequencing [154].
Fragmentation sequencing
Fragmentation sequencing (FragSeq) is an enzyme-based high-throughput sequencing methodology that was developed to investigate the RNA structure by probing the whole mouse nuclear transcriptome [156]. Endonuclease P1, which destroys single-stranded RNA molecules and generates 3’-OH and 5’-phosphate ends, is used in this procedure. RNA is first denatured, then allowed to refold before being treated with PNK; then, size selection of 20–100 nts by using the flash PAGE technique is performed. The RNA fragments are ligated with specific 3’- and 5’-SOLiD adapters, then reverse-transcribed, barcoded, and sequenced on the SOLiD3 platform. The sequenced reads are mapped to the specific genome, and the FragSeq algorithm is used to estimate the “cutting score” for each site in each transcript, which represents the enzyme’s catalytic preference at a specific position in control or treated RNA. A high cutting score indicated base pairing or tertiary interactions and was consistently high at stem-loop and hinge sites [156].
Selective 2’-hydroxyl acylation analyzed by primer extension-sequencing
Selective 2’-hydroxyl acylation analyzed by primer extension-sequencing (SHAPE-seq) provides information for every nucleotide of an RNA molecule. In SHAPE-seq, chemical probes covalently modify the RNA in a structure-dependent fashion [157, 158]. The positions of these adducts are detected using primer extension, which stops one nucleotide before the modification, to create a pool of cDNAs whose lengths reflect the location of SHAPE modification [159]. The cDNAs are then mapped by linking Illumina adaptors with a single-stranded DNA ligase. The cDNA library is PCR amplified, then sequenced and bioinformatically analyzed to identify RNA-RNA duplexes and map RNA secondary structures [160]. In conclusion, SHAPE-seq is a valid and reproducible method for obtaining RRI information at a single-nucleotide resolution, which may then be used in RNA folding algorithms to reconstruct RNA structures [129].
In silico methods for identifying RRIs
Discovering ceRNA networks provides a comprehensive view of the biological functions and regulatory processes of RNAs. Coupling computational prediction with experimental validation improves the efficiency and accuracy of RNA-RNA association detection at the systemic level.
In recent years, some in silico approaches for detecting ceRNA interactions have been developed to find RNA interactions, and according to the computational approach, the process of reconstructing ceRNA networks can be substantially divided into some fundamental steps: 1) collecting sample data, 2) identifying differentially expressed miRNAs and ceRNAs, 3) recognizing ceRNAs with MREs, 4) evaluating the statistical significance of ceRNA pairs sharing common miRNAs, 5) inferring candidate ceRNA interactions and 6) reconstructing global or condition-specific ceRNA networks [161, 162].
For ceRNA network reconstruction, correlated gene expression data from tumors and nearby normal controls are typically prioritized and used to investigate the role of ceRNAs, for example in the development and progression of cancer.
Expression data can be initially generated by high-throughput sequencing or downloaded from publicly available data repositories, such as The Cancer Genome Atlas (TCGA) [163] and Gene Expression Omnibus (GEO) [164]. Due to individual differences, it should be noted that the collected datasets need to include a sufficiently large number of human samples to be statistically meaningful. Additionally, the GENCODE project (part of the ENCODE project) [165] together with the databases miRBase [166] and circBase (http://www.circbase.org/) [167] can be used for functional annotation during data processing. As RNA molecules with altered expression patterns were more likely associated with specific biological processes, differentially expressed miRNAs, lncRNAs, circRNAs, and mRNAs are expected to be identified and analyzed using appropriate statistical methods.
Predicting ceRNAs is initially based on the principle of complementary base-pairing between miRNA and MREs included within the potential ceRNAs. Currently, multiple miRNA-target prediction algorithms, including miRanda (https://cbio.mskcc.org/miRNA2003/miranda.html) [168], TargetScan (https://www.targetscan.org/vert_80/) [169], RNAhybrid (http://bibiserv.techfak.uni-bielefeld.de/rnahybrid) [170] and RNA22 (https://cm.jefferson.edu/rna22/) [171], have been widely used to identify ceRNAs considering a variety of factors, such as complementarity to the miRNA, evolutionary conservation of MREs, the free energy of the miRNA-ceRNA heteroduplex, and flanking secondary structures. Although developments in computational algorithms have been made to increase prediction accuracy, the most commonly used miRNA-target prediction algorithms still have more than 40% false positive and false negative rates [34]. Predicted miRNA-ceRNA interactions should be further filtered and validated experimentally, as suggested by The Encyclopedia of RNA Interactomes (ENCORI) (previously known as Starbase v2.0) (https://rnasysu.com/encori/) [172], DIANA-TarBase v8 (https://dianalab.e-ce.uth.gr/html/diana/web/index.php?r=tarbasev8) [173] and mirTarBase (https://mirtarbase.cuhk.edu.cn/~miRTarBase/miRTarBase_2022/php/index.php) [174]. Furthermore, while the combination of prediction algorithms and experiment-supported databases could facilitate the prediction of ceRNA interactions with relatively high accuracy, there is no gold standard for the effective selection of appropriate combination methods in practice.
The ‘ceRNA hypothesis’ [25] states that information about the expression correlation between interacting ceRNA pairs and between ceRNA-miRNA pairs strengthens the computer-aided knowledge of ceRNA interaction.
Three types of computational approaches, based on the network concept, are mainly used: 1) pair-wise correlation approach, 2) partial correlation approach, and 3) mathematical modeling approach [175].
Pair-wise correlation approach
The pair-wise correlation technique is based on the assumption that the expression levels of each pair of interacting ceRNAs in a network show a significant positive correlation, which can be evaluated by Pearson correlation coefficients. The positive correlation principle is based on the mechanism which evidences that a high concentration of a miRNA sponge will reduce the available miRNAs for interacting with its target RNAs [175], which, in turn, will not undergo miRNA-based repression and, accordingly, will increase their levels.
Using target prediction databases, this approach first searches a set of miRNAs for all pairs of RNAs that contain MREs. Given an RNA pair including RNA1 and RNA2, a statistical test, such as a hypergeometric test, is performed to determine the significance of the shared MREs; pairs with significant positive correlations or significant differences in correlations in two separate situations are predicted to have miRNA sponge interactions [175]. Zhou et al. [176] combined matched breast cancer miRNA and mRNA expression data with miRNA-mRNA relationships to identify breast cancer-specific miRNA sponge interactions. Using predicted miRNA target information and a hypergeometric test, the authors first identified common miRNAs for all mRNA-mRNA pairs. They then used Pearson correlation coefficients to evaluate the significance of the correlations between putative miRNA sponge interaction pairings sharing the same MREs. The miRNA sponge network was developed using the significant miRNA sponge interaction pairs that have been found. The targets in the miRNA sponge network revealed discriminative capability and were considered good biomarker candidates in breast cancer, according to the results of the survival study [176]. Xu et al. [177] applied a similar strategy to predict the landscape of mRNA-related miRNA sponge interactions across 20 major cancer types. Even though the majority of miRNA sponge connections are cancer-specific, malignancies involving the same cell type share a considerable number of common miRNA sponge interactions. In each cancer, functional analysis revealed preserved and rewired network miRNA sponge hubs, which established tense miRNA sponge connections to form conserved and cancer-specific modules [177]. Using expression profiles and miRNA-target interactions, Shao et al. [178] suggested a pair-wise correlation-based technique to uncover dysregulated miRNA sponge interactions in lung adenocarcinoma. The authors classified the dysregulated miRNA sponge interactions as “gain” or “loss”. They discovered that correlations of gain miRNA sponge interactions are significantly positive in cancer, and correlations of loss miRNA sponge interactions are significantly positive in normal conditions. It was also observed that gain miRNA sponge pairs exhibit consistent expression in cancer, whereas loss miRNA sponge pairs do not. Additionally, gain and loss miRNA sponges that participate in gain and loss miRNA sponge interaction networks as topologically important nodes are linked to cancer development. Additionally, several dysregulated miRNA sponge modules may be considered diagnostic biomarkers even in other three independent lung datasets. In the field of biomarkers for diagnosis, this study suggests the possibility of a new biological mechanism in cancer [178]. Chiu et al. [179] analyzed optimal conditions for miRNA sponge regulation by integrating expression profiles and putative miRNA-target interactions in TCGA datasets of glioblastoma multiforme (GBM), ovarian serous cystadenocarcinoma (OV), lung squamous cell carcinoma (LUSC), and large acute myeloid leukemia (LAML). They reported that miRNA sponge regulation is dynamic, differed between cancer types, and was related to the number of common miRNAs and target binding sites [179]. Their findings help us comprehend the dynamic nature and important roles of miRNA sponge regulation in cancer [175].
Partial correlation approach
Differently from pair-wise correlation methods, partial association methods consider the miRNA expression levels when examining ceRNA-ceRNA interactions. When the relationships between miRNA sponges are assumed linear, the partial correlation methods measure the correlation between two ceRNAs sharing a common MRE [175, 180]. Also, when no assumptions are made about the nature of relationships (linear or non-linear) between miRNA sponges, conditional mutual information (CMI) methods can be used rather than partial correlation [59, 180].
In their study, Sumazin et al. [59] examined the ability of coding and non-coding RNAs to interact as miRNA sponges in glioblastoma by evaluating a large set of related miRNA and gene expression profiles. They identified an extensive miRNA-mediated network of RRIs and uncovered miRNA-mediated crosstalk between different oncogenic pathways. They analyzed two types of miRNA-mediated modulators using distinctive molecular mechanisms: sponge modulators and non-sponge modulators. Owing to the prohibitive computational cost of examining a large number of ceRNA-miRNA-ceRNA triangles, they reduced the number of triangles to be evaluated by using experimentally validated or predicted miRNA-target information. Only RNA-RNA pairs with a significant overlap of common miRNAs were examined for sponge modulators, and expression data were combined with predicted miRNA target regulatory interactions to form ceRNA-miRNA-ceRNA triangles. Concerning non-sponge modulators, all RNA-RNA pairs have been analyzed to predict triangles, including the empty and non-statistically significant overlap of common miRNAs, and their expression data were merged with experimentally validated miRNA-target information. Using mutual information (MI) and CMI, Sumazin et al. [59] identified over 7,000 sponge modulators as miRNA sponges with over 248,000 pairs of miRNA-mediated RRIs, and 148 non-sponge modulators with 169 miRNA-mediated RRIs with more than 100 mRNAs involved. This wide miRNA-mediated network offers a new view on the deregulation of essential pathogenic and physiological processes. Paci et al. [181] suggested a partial correlation-based approach to investigate the role of lncRNAs as miRNA sponges. They studied the matched miRNA and gene expression profiles of 72 patients with breast invasive carcinoma (BRCA) obtained from TCGA. The tumor and normal tissue samples came from the same patients. They designed the miRNA-mediated interaction (MMI) networks in tumor and normal conditions by evaluating the difference between the Pearson correlation and partial correlation (sensitivity correlation) for each lncRNA-miRNA-RNA triangle. The tumor-MMI network appears to be clearly distinct from the normal-MMI network, demonstrating that the miRNA decoy mechanism may switch on or off its mediators depending on physiological or pathological conditions [181]. Chiu et al. [182] developed Cupid, an integrative framework for context-specific miRNA target and miRNA sponge interaction networks by combining sequence-based evidence and expression data. Cupid first applies a support vector machine (SVM) classifier to predict candidate miRNA-target interactions and then uses expression-based evidence to further determine ceRNA activities [182]. Cupid is a 3-step prediction method: firstly, it applies Fisher’s exact test [175] to estimate candidate miRNA sponge interaction pairs; secondly, it also uses MI and CMI to identify miRNA sponge interactions and finally performs Brown’s method to further infer significant miRNA sponge interactions [175]. The authors reported over 500 validated miRNA-target interactions involved in breast cancer by employing high-throughput data in breast cancer cell lines for validation. To evaluate the miRNA targets which compete for miRNA regulation, they focused on five breast cancer-associated RNA regulators, cyclin D1 (CCND1), estrogen receptor 1 (ESR1), hypoxia inducible factor 1 subunit alpha (HIF1A), platelet derived growth factor receptor alpha (PDGFRA), and nuclear receptor coactivator 3 (NCOA3). As a result, 8 out of 11 of the predicted miRNA sponge interactions showed significant up-regulation [59].
Mathematical modeling approach
As the term suggests, mathematical modeling approaches focus on predicting ceRNA interactions using different mathematical models. In addition, among the various mathematical model, the minimal model and stochastic model reported results indicating that binding free energies and repression mechanisms are actively involved in ceRNA interactions [183, 184]. Bosia et al. [183] designed a stochastic model to define the equilibrium and disequilibrium characteristic of a miRNA-ceRNA interaction network, revealing how ceRNAs and miRNAs could oscillate correlatively under complex conditions like feedback or feedforward loops. In their study, Swain et al. [185] introduced a competitive endogenous RNA score model to identify candidate ceRNA pairs and estimate their ability to sequester miRNAs. Considering that the high number of components in ceRNA networks can enhance the computational complexity of mathematical models, it could be more efficient to use this method for the construction of smaller ceRNA networks. Following ceRNA interaction prediction, Cytoscape software [186] can be used to generate and analyze the network based on the identified ceRNA interactions [185].
Ala et al. [110] and Figliuzzi et al. [184], used a similar in silico model to find the best settings for miRNA sponge activity. The model illustrates the molecular transition process for miRNA sponge interactions which are mediated by a shared pool of miRNAs. They improved the resulting network by deleting all edges that were not included in the conserved co-expression networks developed in a previous study by Piro et al. [187]. The evaluation of the complexity of the miRNA sponge interaction networks revealed that TFs and ceRNA interactions were cross-regulated, and the latter could cause upregulation or aberrant expression of the TFs in cancer. Furthermore, the results suggested that alterations of a single miRNA sponge have significant effects on the integrated transcriptional and miRNA sponge networks [110]. Yuan et al. [188] developed a coarse-grained model for a minimum miRNA sponge interaction network to quantitatively analyze the behavior of miRNA sponge regulation. They validated the computational results using a synthetic gene in cultured HEK293 cells and found that the number and binding strength of miRNAs and miRNA sponges, as well as the degree of complementarity between miRNAs and miRNA sponges, influenced the extent and strength of the miRNA sponge impact. The combined computational model and experimental validation results provided a clearer understanding of miRNA-mediated cross-regulation on miRNA sponges.
It should be mentioned that more research, optimization, and comparative study of bioinformatic approaches for creating ceRNA networks is required to gain a better understanding of ceRNA regulation.
To uncover miRNA sponge regulation in human cancers, most of the existing computational methods are proposed at the network level, and only a small number of methods are presented at the module level. Modules in this context refer to groups of miRNA sponges having mutual competition and acting as functional units in the regulation of biological processes [189]. Therefore, module identification is an important way to provide biological insights into ceRNA networks. As an example, modules could facilitate functional genome annotation by using the guilt-by-association principle [190] and help understand the origin and progression of human diseases [191]. Currently, most of the existing module discovery methods infer miRNA sponge modules by using ceRNA network data. Although these methods also use heterogeneous data (gene expression data, miRNA-target binding, and MRE information) in the process of inferring ceRNA networks, the identified miRNA sponge modules are network-level modules, each containing a set of miRNA sponges that frequently interact with each other in a ceRNA network. These module-based methods are categorized into three types: network-based clustering, matrix factorization, and step-wise evaluation, as summarized by Zhang et al. [189].
Since non-coding and protein-coding RNAs can act as miRNA sponges, RRI databases are valuable resources applied in computational approaches to develop potential networks of miRNA sponge interactions. There are two types of miRNA-target binding databases: those with experimentally validated target bindings and those with predicted miRNA-ceRNA interactions [175]. To identify potential miRNA sponge interactions, the users can query distinct databases or merge different datasets as the initial framework of computational approaches. Some of the most widely used datasets of miRNA sponge interactions are presented below (Table 1).
Summary of some of the most widely used databases for the investigation of miRNA sponge interactions
Database | Type of data | Description | Ref | URL |
---|---|---|---|---|
CeRDB | Predicted | One of the earliest miRNA sponge interaction databases, finds miRNA sponges for a specific target mRNA based on the homology of miRNA binding sites | [192] | https://www.oncomir.umn.edu/cefinder/ |
DIANA-TarBase v.8 | Validated | Aims to provide hundreds of thousands of high-quality manually curated experimentally validated miRNA: gene interactions, enhanced with detailed meta-data | [193] | https://dianalab.e-ce.uth.gr/html/diana/web/index.php?r=tarbasev8 |
DIANA-microT | Predicted | Web server dedicated to miRNA target prediction/functional analysis | [194] | https://dianalab.e-ce.uth.gr/microt_webserver/#/ |
ENCORI | Validated | Previously known as starBase v2.0, identifies millions of RBP-RNA and RRIs by analyzing thousands of CLIP-seq and various high-throughput sequencing data, studying their functions and mechanisms in human diseases | [172] | https://rnasysu.com/encori/ |
LncACTdb v3.0 | Predicted | A comprehensive database of experimentally supported interactions among ceRNAs and the corresponding personalized networks contributing to precision medicine | [195] | http://bio-bigdata.hrbmu.edu.cn/LncACTdb/ |
LnCeCell | Predicted and validated | Provides functional lncRNA-related miRNA sponge interaction networks in single cells and sub-cellular locations curated from the published literature and high-throughput datasets | [196] | http://bio-bigdata.hrbmu.edu.cn/LnCeCell/ |
LnCeVar | Predicted and validated | Database collecting genomic variations that disturb lncRNA-related miRNA sponge regulation curated from the published literature and high-throughput datasets across 33 human cancers | [197] | http://www.bio-bigdata.net/LnCeVar/ |
miRanda | Predicted | Algorithm for finding genomic targets for miRNAs, written in C and available as an open-source method | [168] | https://cbio.mskcc.org/miRNA2003/miranda.html |
miRcode | Predicted | Provides “whole transcriptome” human microRNA target predictions based on the comprehensive GENCODE gene annotation | [198] | http://www.mircode.org/ |
miRTarBase | Validated | Database providing information about experimentally validated miRNA-target interactions | [199] | https://mirtarbase.cuhk.edu.cn/~miRTarBase/miRTarBase_2022/php/index.php |
miRTissuece | Predicted | Web service for the analysis and characterization of miRNA sponge interactions in several cancer tissue types | [200] | http://tblab.pa.icar.cnr.it/mirtissue.html |
miRWalk | Validated | A comprehensive archive supplying the largest available collection of predicted and experimentally verified miRNA-target interactions | [201] | http://mirwalk.umm.uni-heidelberg.de/ |
Pan-ceRNADB | Predicted | Investigates mRNA-related miRNA sponge interactions across 20 major human cancers | [177] | http://bio-bigdata.hrbmu.edu.cn/pan-cernadb/ |
PicTar | Predicted | An algorithm for the identification of miRNA targets | [202] | https://pictar.mdc-berlin.de/ |
PITA | Predicted | An algorithm using standard settings to identify initial seeds for each miRNA in 3’ UTRs | [203] | https://genie.weizmann.ac.il/pubs/mir07/mir07_prediction.html |
RNA22 | Predicted | A method for identifying miRNA binding sites and their corresponding heteroduplexes | [204] | https://cm.jefferson.edu/rna22/ |
SomamiR | Predicted | Database of cancer somatic mutations that potentially alter the interactions between miRNAs and miRNA sponges | [205] | http://compbio.uthsc.edu/SomamiR/ |
TargetScan | Predicted | Prediction of miRNA target sites conserved among orthologous 3’-UTRs of vertebrates | [206] | https://www.targetscan.org/vert_80/ |
VECTOR | Predicted and validated | An interactive tool that identifies and visualizes the relationships among RNA molecules associated with ChiP-seq data and expression data of miRNAs, lncRNAs, and mRNAs both in UM and physiological tissues | [207] | https://vectordb.it/ |
CeRDB: Competitive Endogenous mRNA DataBase; Pan-ceRNADB: Pan-cancer associated ceRNA database; ChiP-seq: chromatin immunoprecipitation sequencing; VECTOR: uVeal mElanoma Correlation NeTwORk; UM: uveal melanoma
CeRDB (https://www.oncomir.umn.edu/cefinder/) [192] was one of the first-developed miRNA sponge interaction databases; the authors used TargetScan (https://www.targetscan.org/vert_80/) [206] to check for the co-occurrence of MREs in mRNAs across the genome. CeRDB estimates the miRNA sponges that compete with the other RNAs for the binding of a specific miRNA. CEFINDER, a web-based tool provided by CeRDB, allows users to find miRNA sponges for a specific mRNA target based on the homology of MREs located within the 3’-UTR sequence [192].
The ENCORI database (https://rnasysu.com/encori/) [172] stores 108 datasets obtained from 37 studies, including 47 high-throughput sequencing of RNA isolated by CLIP (HITS-CLIP) datasets, 51 photoactivatable-ribonucleoside-enhanced CLIP (PAR-CLIP) datasets, 9 individual-nucleotide resolution UV CLIP (ICLIP) datasets and 1 CLASH dataset. Recently, a miRNA sponge interaction database was added to ENCORI. The experiment results stored in ENCORI provide information about the physical binding between miRNA-mRNA, miRNA-lncRNA, miRNA-circRNA, miRNA-pseudogene, and miRNA-small ncRNAs. The interactions can be used to verify computational predictions [172].
LncACTdb (http://bio-bigdata.hrbmu.edu.cn/LncACTdb/) is another database created by Wang et al. [161] defining lncRNA-miRNA-gene interactions as lncRNA-associated competing triplets (lncACTs). This is a database of lncRNA-associated competing triplets designed to aid in the study of lncRNA function as miRNA sponges. The database contains 5,119 functionally active and over 530,000 computationally predicted lncACTs derived from merging heterogeneous data from multiple in silico target prediction studies, AGO-CLIP assays, and transcriptome sequencing expression profiles. The database has been recently improved in LncACTdb 3.0, an updated and significantly expanded version that provides comprehensive information on ceRNAs in different species and diseases [195].
Another database is LnCeCell (http://bio-bigdata.hrbmu.edu.cn/LnCeCell/) which provides functional lncRNA-related miRNA sponge interaction networks in single cells and sub-cellular locations curated from the published literature and high-throughput datasets across 25 human cancers [196].
LnCeVar (http://www.bio-bigdata.net/LnCeVar/) is a comprehensive database that collects genomic variations that disturb lncRNA-related miRNA sponge regulation curated from the published literature and high-throughput datasets across 33 human cancers [197].
Other useful resources are: miRTissuece (http://tblab.pa.icar.cnr.it/mirtissue.html), a web service for the analysis and characterization of miRNA sponge interactions in several cancer tissue types [200]; SomamiR 2.0 (http://compbio.uthsc.edu/SomamiR/), a database of cancer somatic mutations that potentially alter the interactions between miRNAs and miRNA sponges (including mRNAs, circRNAs, and lncRNAs) [205]; Pan-ceRNADB (http://bio-bigdata.hrbmu.edu.cn/pan-cernadb/), a web resource which investigates mRNA-related miRNA sponge interactions across 20 major human cancers [177].
The VECTOR (https://vectordb.it/) database is also worth mentioning; it is an interactive tool that identifies and visualizes the relationships among RNA molecules. VECTOR database contains: i) the TCGA-derived expression correlation values of miRNA-mRNA, miRNA-lncRNA, and lncRNA-mRNA pairs combined with predicted or validated RRIs; ii) data of sense-antisense sequence overlapping; iii) correlation values of TF-miRNA, TF-lncRNA, and TF-mRNA pairs associated with ChiP-seq data; iv) expression data of miRNAs, lncRNAs and mRNAs both in UM and physiological tissues [207].
Experimentally validated miRNA-mRNA binding databases mainly include miRTarBase (https://mirtarbase.cuhk.edu.cn/~miRTarBase/miRTarBase_2022/php/index.php) [199], DIANA-TarBase v.8 (https://dianalab.e-ce.uth.gr/html/diana/web/index.php?r=tarbasev8) [193], and miRWalk (http://mirwalk.umm.uni-heidelberg.de/) [201]. The commonly used predicted miRNA-mRNA binding databases are TargetScan (https://www.targetscan.org/vert_80/) [206], miRanda (https://cbio.mskcc.org/miRNA2003/miranda.html) [168], PicTar (https://pictar.mdc-berlin.de/) [202], DIANA-microT (https://dianalab.e-ce.uth.gr/microt_webserver/#/) [194], and PITA (https://genie.weizmann.ac.il/pubs/mir07/mir07_prediction.html) [203].
Conclusions
Understanding the intricate interactions between ncRNAs and protein-coding RNAs will help us better comprehend how RNA signaling controls the complex processes of genome expression in both healthy and unhealthy conditions. The development of new experimental and computational methods for identifying and characterizing RRIs has allowed for the collection of an ever-increasing amount of data, which will have positive effects on the treatment of diseases, particularly cancer. Indeed, researchers have been developing effective RNA-based anticancer strategies realistically applicable to patients [14, 208]. These RNA-based drugs are conceived to target (i.e., repress) or replace RNA molecules whose dysregulation alters the stoichiometric equilibrium of ceRNA networks. Importantly, these new RNA-based therapeutic approaches may be associated with the already developed strategies targeting proteins or replace them aiming to improve the delivery thanks to the different chemical nature of nucleic acids [209]. To fully realize the clinical potential of this extremely promising approach, it will be essential to organize significant collaborative efforts between research institutes and industry before RNA therapeutics is widely used in clinical settings.
Abbreviations
A: |
adenine |
AGO: |
Argonaute |
AGO-CLIP: |
Argonaute cross-linking and immunoprecipitation |
AMT: |
4’-aminomethyltrioxsalen |
ASOs: |
antisense oligonucleotides |
BACE1: |
beta-secretase 1 |
BACE1-AS: |
beta-secretase 1 antisense RNA |
CD44 : |
CD44 molecule (Indian blood group) |
cDNA: |
complementary DNA |
CDR1: |
cerebellar degeneration related 1 |
CDR1-AS: |
CDR1 antisense RNA |
ceRDB: |
competitive endogenous messenger RNA database |
ceRNAs: |
competing endogenous RNAs |
circ-ITCH: |
circular RNA itchy E3 ubiquitin protein ligase |
circRNAs: |
circular RNAs |
ciRNAs: |
circular intronic RNAs |
CLASH: |
cross-linking, ligation, and sequencing of hybrids |
CMI: |
conditional mutual information |
EMSA: |
electrophoretic mobility shift assay |
EMT: |
epithelial to mesenchymal transition |
ENCORI: |
Encyclopedia of RNA Interactomes |
FN1 : |
fibronectin 1 |
FragSeq: |
fragmentation sequencing |
FRET: |
Förster resonance energy transfer |
HiCLIP: |
RNA hybrid and individual-nucleotide resolution ultraviolet cross-linking and immunoprecipitation |
HSUR: |
Herpesvirus saimiri U-rich noncoding RNAs |
IPS1 : |
induced by phosphate starvation1 |
isomiRs: |
microRNA isoforms |
LIGR-seq: |
ligation of interacting RNA followed by high-throughput sequencing |
lncRNAs: |
long non-coding RNAs |
MALAT1 : |
metastasis associated lung adenocarcinoma transcript 1 |
MARIO: |
mapping RNA interactome in vivo |
MI: |
mutual information |
miRNAs: |
microRNAs |
MMI: |
microRNA-mediated interaction |
MREs: |
microRNA-response elements |
mRNAs: |
messenger RNAs |
NATs: |
natural antisense transcripts |
ncRNAs: |
non-protein-coding RNAs |
nts: |
nucleotides |
OV: |
ovarian serous cystadenocarcinoma |
PAGE: |
polyacrylamide gel electrophoresis |
PARIS: |
psoralen analysis of RNA interactions and structures |
PARS: |
parallel analysis of RNA structure |
PARTE: |
parallel analysis of RNA structures with temperature elevation |
PCR: |
polymerase chain reaction |
PTEN : |
phosphatase and tensin homolog |
PTENP1 : |
phosphatase and tensin homolog pseudogene 1 |
RAP: |
RNA antisense purification |
RBP: |
RNA-binding protein |
RNase: |
ribonuclease |
RRIs: |
RNA-RNA interactions |
rRNAs: |
ribosomal RNAs |
SHAPE-seq: |
selective 2’-hydroxyl acylation analyzed by primer extension-sequencing |
Sirt1 : |
sirtuin 1 |
SLC2A3 : |
solute carrier family 2 member 3 |
smFISH: |
single-molecule fluorescent in situ hybridization |
smFRET: |
single-molecule Förster resonance energy transfer |
SNAI1: |
snail family transcriptional repressor 1 |
snoRNAs: |
small nucleolar RNAs |
SOLiD: |
Sequencing by Oligo Ligation Detection |
SPR: |
surface plasmon resonance |
sRNAs: |
small RNAs |
TCGA: |
The Cancer Genome Atlas |
TFs: |
transcription factors |
Tm: |
melting temperature |
tRNAs: |
transfer RNAs |
TUSC2: |
tumor suppressor 2, mitochondrial calcium regulator |
UTR: |
untranslated region |
UV: |
ultraviolet |
VCAN: |
versican |
VECTOR: |
uVeal mElanoma Correlation NeTwORk |
ZEB2 : |
zinc finger E-box binding homeobox 2 |
ZEB2-AS1 : |
ZEB2 antisense RNA 1 |
ZNF609: |
zinc finger protein 609 |
Declarations
Acknowledgments
The authors thank the Scientific Bureau of the University of Catania for language support.
Author contributions
CB: Conceptualization, Writing—original draft, Writing—review & editing. MS: Writing—original draft, Writing—review & editing. CF, AC, RB, DB, and CDP: Writing—review & editing. MR: Conceptualization, Supervision, Writing—review & editing.
Conflicts of interest
The authors declare that they have no conflicts of interest.
Ethical approval
Not applicable.
Consent to participate
Not applicable.
Consent to publication
Not applicable.
Availability of data and materials
Not applicable.
Funding
This study was supported by “linea intervento 3 Open Access Piano PIACERI 2020‐2022” from the University of Catania. The funders had no role in study design, data collection and analysis, decision to publish, or preparation of the manuscript.
Copyright
© The Author(s) 2023.