Abstract
Aim:
Research suggests that Alzheimer’s disease (AD) is heterogeneous with numerous subtypes. Through a proprietary interactive ML system, several underlying biological mechanisms associated with AD pathology were uncovered. This paper is an introduction to emerging analytic efforts that can more precisely elucidate the heterogeneity of AD.
Methods:
A public AD data set (GSE84422) consisting of transcriptomic data of postmortem brain samples from healthy controls (n = 121) and AD (n = 380) subjects was analyzed. Data were processed by an artificial intelligence platform designed to discover potential drug repurposing candidates, followed by an interactive augmented intelligence program.
Results:
Using perspective analytics, six perspective classes were identified: Class I is defined by TUBB1, ASB4, and PDE5A; Class II by NRG2 and ZNF3; Class III by IGF1, ASB4, and GTSE1; Class IV is defined by cDNA FLJ39269, ITGA1, and CPM; Class V is defined by PDE5A, PSEN1, and NDUFS8; and Class VI is defined by DCAF17, cDNA FLJ75819, and SLC33A1. It is hypothesized that these classes represent biological mechanisms that may act alone or in any combination to manifest an Alzheimer’s pathology.
Conclusions:
Using a limited transcriptomic public database, six different classes that drive AD were uncovered, supporting the premise that AD is a heterogeneously complex disorder. The perspective classes highlighted genetic pathways associated with vasculogenesis, cellular signaling and differentiation, metabolic function, mitochondrial function, nitric oxide, and metal ion metabolism. The interplay among these genetic factors reveals a more profound underlying complexity of AD that may be responsible for the confluence of several biological factors. These results are not exhaustive; instead, they demonstrate that even within a relatively small study sample, next-generation machine intelligence can uncover multiple genetically driven subtypes. The models and the underlying hypotheses generated using novel analytic methods may translate into potential treatment pathways.
Keywords
Machine learning, genetic subtypes, disease heterogeneity, drug repurposing, augmented intelligence, machine intelligence, artificial intelligence, target discoveryIntroduction
Alzheimer’s disease (AD) is the most common form of dementia, contributing to 60–70% of dementia cases [1]. This neurodegenerative disease is characterized by neuronal cell damage and concomitant cognitive and functional decline, predominantly affecting older individuals, with two-thirds being women, and prevalence is expected to continue to rise as the population ages [2–4]. There is currently no definitive cure to prevent or attenuate the progression of this debilitating disease. Research efforts aimed at disease modification have focused on the amyloid and tau pathways as significant contributors of AD pathology due to excessive deposition of β-amyloid (Aβ) peptides and hyperphosphorylated tau proteins contributing to DNA and RNA damage [5–7]. However, none of the currently clinically approved AD drugs are disease-modifying therapies (DMTs) and instead broadly target AD symptoms [8]. Despite over 100 agents in the current AD treatment pipeline, the last AD drug approved by the U.S. Food and Drug Administration (FDA) was memantine, an N-methyl-D-aspartate (NMDA) receptor AD antagonist, in 2003 [9, 10]. While the Chinese FDA recently approved the clinical use of oligomannate (GV-971), international drug trials are underway to confirm results and validate use outside of China (NCT03715114, NCT02986529, NCT02293915) [11]. Due to gaps in our understanding of AD etiology and the complex interactions between genomic and environmental factors that lead to disease heterogeneity, a multimodal approach towards precision medicine is necessary.
There are currently very few consistently reported susceptible risk loci associated with AD. Early-onset Alzheimer’s disease (EOAD), which follows a Mendelian inheritance pattern, is primarily associated with mutations in one of three genes–amyloid precursor protein (APP), presenilin-1 (PSEN1), and presenilin-2 (PSEN2) [12]. However, late-onset Alzheimer’s disease (LOAD), which accounts for over 95% of AD cases, is associated with a more complex genomic makeup. To date, apolipoprotein E (APOE), a lipid carrier involved in cholesterol metabolism, is the strongest genetic risk factor for LOAD. Specifically, the APOE ε4 allele has been reported to have a lower affinity for lipoproteins and poorly binds Aβ [13]. Genome-wide association studies (GWAS) have identified several other susceptibility loci that confer AD risk to varying degrees that can be broadly categorized into those involved in immunity, lipid homeostasis, cytoskeletal interactions, endocytosis, and apoptosis [8, 14–16].
Machine learning (ML) efforts allow for a more systems-level approach that considers complex genetic interactions to reveal critical insights into disease etiology and identifying new drug targets [17]. While there has been extensive research using ML models to classify AD risk, discriminate between AD and mild cognitive impairment (MCI), and predict MCI-to-AD conversion based on structural and functional magnetic resonance imaging (MRI), positron emission tomography (PET) scans, and cerebrospinal fluid (CSF), there is less known about genetic subtypes within the AD patient population [18–21]. A recent study revealed sex- and age-based AD subpopulations. There was only a moderate genetic correlation between younger (60–79 years old) and older (> 80 years old) age-at-onset AD subjects, suggesting that the polygenic architecture of AD is heterogeneous across age. However, stratified GWAS and polygenic variation analyses highlighted BIN1, OR2S2, and PICALM as having significant effects at a younger age [22]. Relative expression ordering (REO)-based gene expression profiling analyses revealed two distinct subtypes within AD patients–one in which differentially expressed genes overlapped with age-related genes and one related to neuroinflammation [23]. Since AD primarily affects older individuals, it is not surprising that memory-spared individuals were often younger and APOE ε4 negative compared to memory-impaired individuals [24]. Furthermore, in-depth latent class analysis (LCA) of subjects with AD dementia revealed eight cognitive subtypes associated with distinct demographical and neurobiological characteristics. For example, the memory spared moderate-visuospatial cluster was associated with younger age, APOE ε4 negative genotype, and prominent atrophy of the posterior cortex [25].
APOE ε4 allele frequency is consistently associated with more extensive AD-associated neuropathology and cognitive deficits [26]. It is evident that specific genetic variants, such as APOE ε4, significantly contribute to disease heterogeneity compared to other genetic variants. The polygenic risk score (PRS) determines the cumulative genetic risk for an individual. Adopting a single nucleotide polymorphism (SNP) and transcriptomic approach when considering the PRS more accurately captures the contribution of individual SNPs and differential gene expression [12, 27]. Incorporating these strategies will contribute to the shift towards accurate patient stratification and classification, bringing precision medicine closer to reality. Rather than developing therapies for population averages of a biologically heterogeneous disease such as AD, artificial intelligence (AI)-based algorithms can be utilized for more individually-tailored therapies [28].
Here, we utilized a suite of ML tools designed to learn from subject datasets to analyze gene expression data derived from postmortem controls vs. AD subjects. Importantly, these next-generation methods can learn from smaller datasets than is typically assumed as necessary with many ML approaches and can explain the driving variables, as will be explained below. The novelty of this work lies in the machine’s ability to discover unknown subpopulations that are defined by several genes at a time. These genes may be related to each other and the dependent variable, e.g., AD status, in non-linear ways. The ability of some of these methods to extract non-linear relationships from small data is an exceptional trait, which in combination with explainability and the ability to learn from small datasets, uncovers a new avenue of exploring patient populations. Collectively, these properties will equip researchers to redefine our understanding of disease heterogeneity and significantly move the needle forward on the precision treatment of disease.
Materials and methods
Dataset assembly and analysis
A public AD dataset (GSE84422) consisting of transcriptomic data of postmortem brain samples from 121 healthy controls and 380 AD subjects was assembled. The analysis carried out in this paper was based on only AD subjects. This was done intentionally to extract a refined vantage into AD beyond APOE findings, which are well established in the field.
A unique suite of ML methods was assembled due to their ability to extract subpopulations from high-dimensional data and their ability to provide explanations for the driving mechanisms behind the subpopulations [29, 30]. These methods include statistical measures of feature importance, ensemble methods, neural networks, and a novel system designed to work with patient population data [31]. We also describe in detail methods that were used, which are freely available to researchers.
Perspective analytics
A significant feature of these machine intelligence methods is their ability to see a patient population in numerous ways. To be more precise, there are various ways to model a group of AD subjects vs. control subjects. Different collections of genes will reveal different relationships amongst the samples and different subtypes of subjects. These different models are called ‘perspectives,’ and this approach is referred to as perspective analytics. Each perspective is learned by the machine and consists of a unique set of variables, with each variable having a different contribution. Different collections of variables are arrived at through a feature selection methodology that consists of univariate statistics and Random Forest cross-validation verifications [32, 33]. If an independent dataset is available, the perspective analytics algorithm uses it; otherwise, it must rely on leave out and cross-validation protocols to establish reliability and avoid overfitting. The machine is rewarded for finding groups of samples within the same perspective class with several variables simultaneously in common, making it semi-supervised [29]. The results provided in this paper were derived from models that must have at least a 75% cross-validation score. This machine intelligence utilizes geometric representation methods coupled with a fast learner. These methods were created specifically for use with smaller datasets; therefore, they are inherently designed to find statistically significant pure subpopulations of a given label rather than trying to find perfect models.
Validation
Since the perspective analytics methods [29] used cannot be revealed due to intellectual property concerns, we utilized the following methodology using techniques that are available to the public to help validate our results [34]. Our analysis only utilized gene expression data, i.e., counts, and passed the data through the following process:
1) Each sample had gene expression levels associated with them derived by Affymetrix technology (GPL96: Affymetrix Human Genome U133A Array, GPL97: Affymetrix Human Genome U133B Array, GPL570: Affymetrix Human Genome U133 Plus 2.0 Array).
2) Different normalizations were performed so that each gene expression was ranked, broken into quantiles, or relative log expression was used.
3) Using Random Forest and univariate variable reduction methods [32, 33] left us with 9, 060 variables. The dependent variable for cross-validation was based on a binary variable we created, which distinguished low dementia vs. high dementia (See the supplemental materials; 0 = low and 1= high).
4) We then conducted a principal components analysis as a linear unsupervised clustering method to reveal different subclasses.
5) The loadings from the principal components were utilized to reduce the variables of focus to 16 variables.
6) Using the t-SNE and UMAP algorithms, we were able to extract subpopulations.
7) We then collected the sample IDs from the clusters formed from these two clustering models, systematically compared each group with the other, and looked for statistically significant genes. Several statistics were explored, but in order to deal with non-normality, the Wilcoxon signed-rank test was used [35].
8) The resulting statistically significant genes revealed by this process became associated with the sample clusters, and we called these the cluster-associated genes.
9) A study of the protein interaction networks formed from the cluster-associated genes helped us interpret their physiological role in AD. These are what is later referred to as the six progression mechanisms.
For transparency, this process was used to verify the more efficient method that we also used and previously outlined [29]. The perspective analytics platform known as NetraAI utilizes a similar process to extract subpopulations; however, it allows for human interaction via a user interface, and the subpopulation discovery is based on mathematics that allows for a very refined set of sub-populations to be discovered and explored.
Results
We were able to derive six progression mechanisms (i.e., perspective classes) that represent the various ways that an individual may manifest an AD pathology (Figure 1). An individual can progress via a single class or any combination of the six classes, highlighting the complexity of the AD population and resulting in 63 possible combinations. However, there are likely even more mechanisms at play, including immune system function, which plays an important orchestration role, further contributing to the complex AD etiology.
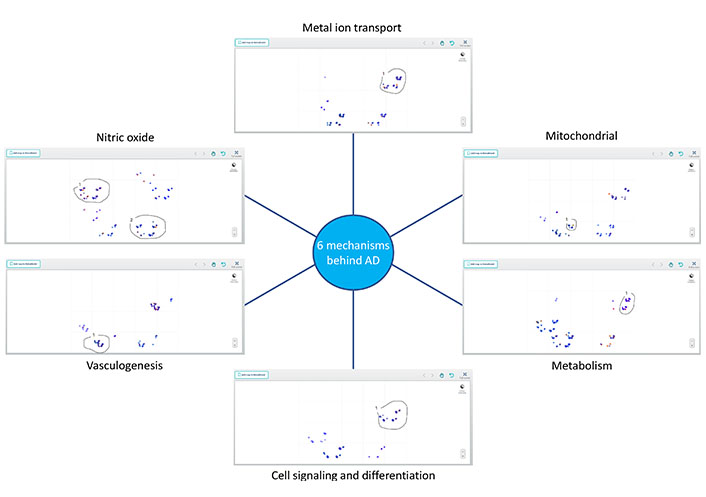
Perspective analytics for AD. Perspective analytics discovered a unique set of variables for each of the six different perspectives learned from an Alzheimer’s dataset. Within each set, there is a subgroup of subjects that are driven by the corresponding etiology
While it is evident that small datasets are not representative of the overall disease state, the significant occurrence of variables binding together subjects of the same Class can provide valuable insights with respect to precision medicine. The characteristics of each perspective class, each of which represents a novel avenue of the complex etiologies that drive neurodegeneration and cognitive aging, are highlighted in Table 1. Subjects that belong to more than one perspective class may be due to the overlapping components across some of the pathways implicated in each perspective class.
Perspective classes, characteristic genes, and defining traits of AD patients
Perspective class | Number of subjects | Gene name | Gene symbol | Defining trait |
---|---|---|---|---|
I | 156 | Tubulin beta 1 class VI | TUBB1 | Vasculogenesis |
Ankyrin repeat and SOCS box-containing protein 4 | ASB4 | |||
Phosphodiesterase 5A | PDE5A | |||
II | 164 | Neuregulin-2 | NRG2 | Cell signaling and differentiation |
Zinc-finger 3 | ZNF3 | |||
III | 84 | Insulin-like growth factor 1 | IGF1 | Metabolism |
Ankyrin repeat and SOCS box-containing protein 4 | ASB4 | |||
G2 and S-phase expressed protein 1 | GTSE1 | |||
IV | 134 | cDNA FLJ39269 | Nitric oxide | |
Integrin subunit alpha | ITGA1 | |||
Carboxypeptidase M | CPM | |||
V | 76 | Phosphodiesterase 5A | PDE5A | Mitochondrial |
Presenilin 1 | PSEN1 | |||
NADH-coenzyme Q reductase | NDUFS8 | |||
VI | 228 | DDB1 and CUL4 associated factor 17 | DCAF17 | Metal ion transport |
cDNA FLJ75819 | ||||
Solute carrier family 33 member 1 | SLC33AI |
SOCS: suppressor of cytokine signaling; NADH: nicotinamide adenine dinucleotide; DDB1: DNA damage-binding protein 1; CUL4: cullin-4
Due to reports of AD having a greater prevalence and severity in women, we investigated whether certain classes were more prevalent in females than males (Table 2). Interestingly the metal ion transport class was the most common, with 65% of the female AD subjects and 61% of the male AD subjects progressing via this Class. Of the 266 female AD subjects, 49.6% progressed via the cell signaling class, compared to only 35.5% of the male subjects. Of the 90 male AD subjects, 58.9% fell under the vasculogenesis perspective class, compared to only 38.7% of the female subjects.
Sex-based differences in perspective classes of AD progression
Sex | Perspective class | |||||
---|---|---|---|---|---|---|
Vasculogenesis | Cell signaling | Metabolism | Nitric oxide | Mitochondrial | Metal ion transport | |
Female | 103 | 132 | 60 | 104 | 44 | 173 |
Male | 53 | 32 | 24 | 30 | 32 | 55 |
Total | 156 | 164 | 84 | 134 | 76 | 228 |
In the remainder of this study, we utilized protein interaction/expression and gene pathway networks to assist in the interpretation of the physiological components behind our findings. We input the resulting statistically significant genes for each subclass we discovered into GeneMania [36], which allowed us to extract what we refer to as the perspective classes summarized in Table 2.
Class I is identified by TUBB1, ASB4, and PDE5A. As the primary identifier of Class I, TUBB1 mutations are associated with enlarged rounded platelets and result in thrombocytopenia [37]. The strong link of AD to vascular diseases such as stroke and atherosclerosis suggests a crucial role for vascularization in this subpopulation. Interestingly, there appears to be a network between TUBB1, ASB4, and PDE5A, suggesting Class I represents a subpopulation defined by the regulation of vasculogenesis [38–40] (Figure 2).
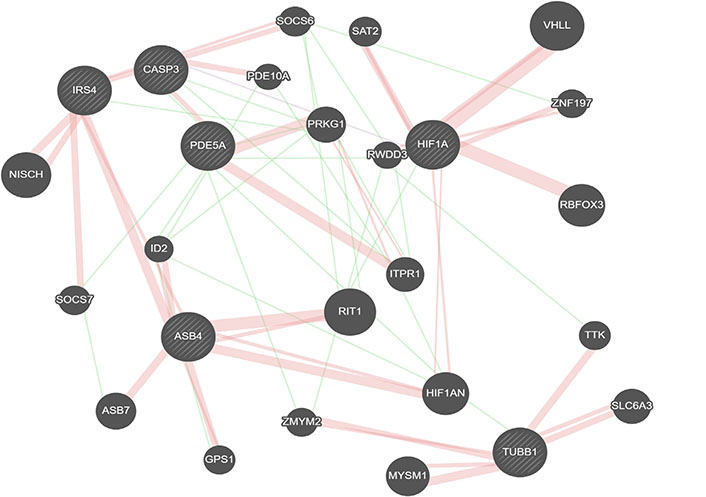
Gene interactions for Class I identifiers. Red represents physical interactions, purple represents co-expression, and green represents genetic interactions. Created using GeneMania [36].
NRG2 and ZNF3 define Class II. Neuregulins (NRGs) stimulate ErbB-receptor tyrosine phosphorylation that elicits different downstream signaling pathways such as MAPK, PI3, PKC, and the Janus kinase signal transducer and activator of transcription (JAK-STAT) pathways and are associated with synaptic plasticity [41, 42]. ZNF3 is a zinc-finger protein that is differentially expressed in AD and is involved in cell differentiation and proliferation [43]. Thus, we define Class II by cell signaling and differentiation.
Class III is defined by IGF1, ASB4, and GTSE1. Impairments in insulin/IGF1 signaling have been associated with AD [44, 45]. IGF1 is connected to ASB4 by IRS4 (insulin receptor substrate 4) and SOCS2 (Figure 3). In contrast, GTSE1 is a microtubule-associated protein that regulates G1/S cycle transition and microtubule stability [46]. Given the role of GTSE1 and TUBB1 on microtubule stability and formation and the shared presence of ASB4 as a descriptor in both Class I and Class III, these two classes may represent one larger overarching AD subpopulation that can be further stratified into microtubule formation and IGF1 pathway signaling. This is supported by the fact that the subjects the machine clustered together in the metabolism class have lower expression levels of IGF1 than those we classified as being members of the vasculogenesis class.
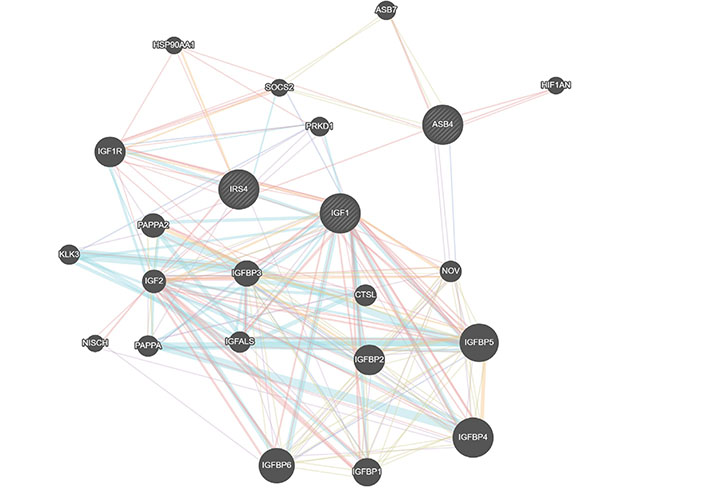
Gene interactions for Class III identifiers. Red represents physical interactions, purple represents co-expression, orange represents predicted interactions, blue represents co-localization, aqua represents a shared pathway, green represents genetic interactions, and yellow represents shared protein domains. Created using GeneMania
Class IV is defined by cDNA FLJ39269, ITGA1, and CPM. cDNA FLJ39269 is most closely associated with GUCY1A3, which is dysregulated in AD [47, 48]. CPM is known to enhance nitric oxide (NO) output, playing a role in NO signaling under inflammatory conditions [49]. Due to the recurrent role of NO, we define Class IV with NO, despite ITGA1 not being involved in this signaling.
Class V is defined by PDE5A, PSEN1, and NDUFS8. Despite PSEN1 mutations being one of the most common causes of familial Alzheimer’s disease (FAD), PSEN1 defined Class V to a lesser extent than PDE5A. However, within this population, SYK (spleen tyrosine kinase) was found to be driving a difference within this group (Figure 4). The system is seeing the disease at multiple scales. NDUFS8 [NADH dehydrogenase (ubiquinone) Fe-S protein 8; NADH-coenzyme Q reductase] along with other genes involved in oxidative phosphorylation are decreased in AD [50]. Given the role of these genes in mitochondrial function and redox, Class V was defined as mitochondrial.
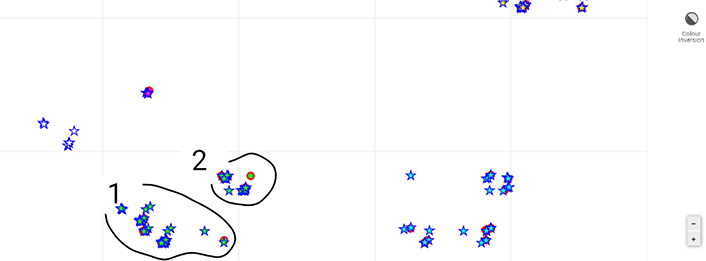
Sub-map of the AD population of Class V. This sub-map (zoomed in) provides another facet of the Alzheimer’s population within this dataset. From one perspective, PDE5A and PSEN1 are driving the relationships between the subjects illustrated. However, SYK is driving the slight separation between loop 1 and 2
A map of samples in terms of how they compare to each other according to some non-linear metric via a set of genes is shown in Figure 4. Each point in this figure is a subject, and if they are near each other, it means that they are similar according to a set of genes. In this way, one can see non-linear relationships via a simple 2-dimensional representation, like in principle components analysis, except principle components only reveal linear relationships. Further, by using a looping feature with a mouse, one can query the machine in terms of what is driving the separation between groups of subjects. Looping triggers a statistical process to provide confidence in whatever variables are implicated. Thus, there are no axes, but instead, relative distances according to what is driving the heterogeneity of the subjects. This process is explained in detail elsewhere [29].
Class VI is defined by DCAF17, cDNA FLJ75819, and SLC33A1. DCAF17 (DDB1 and CUL4 associated factor 17) is a nuclear transmembrane protein associated with damaged DNA binding protein 1 ubiquitin ligase complex and is involved in iron accumulation [51]. Given the mitochondrial role of the primary identifier of Class VI, there may be some overlap in subjects in Class V and VI. Interestingly, 44 subjects fell under both Class V and Class VI (Figure 5). cDNA FLJ75819 is most similar to ZNF652, which is associated with metal protein. With these two genes in mind, Class VI appears to be defined by metal ion metabolism.
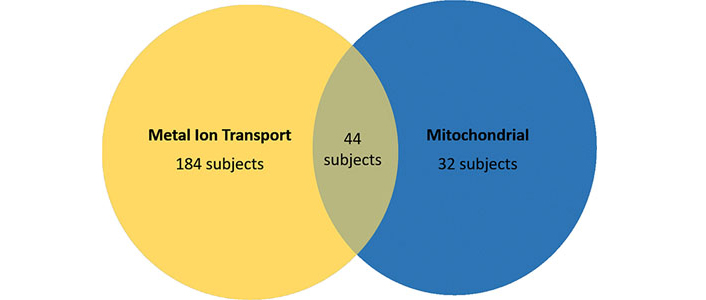
Overlap of subjects in the metal ion transport and mitochondrial perspective classes. A complete list of the AD samples from the data we used, in addition to which classes they fall in, is available in the supplementary materials
Discussion
ML efforts in the field of Alzheimer’s genomic research have been primarily focused on discovering subjects at-risk for AD or with high MCI-to-AD conversion. This work has been increasingly focused on identifying genetic subtypes within the presumption of a heterogeneous AD population. The need to expand biomarker-based stratification within the AD population has been highlighted with as many as 30 altered transcriptional signatures found to distinguish AD samples from non-demented brain samples [52]. However, there are currently few predictions for AD-associated genes based on brain gene expression data alone. This study sought to develop a brain-specific gene interaction network to predict the potential AD association for every gene in the genome by integrating the relationship between each pair of AD-associated genes and the correlation coefficient of known AD-associated and -unassociated genes [53]. This genome-wide complement of AD candidate genes provides a precision medicine approach that can be used to explore AD mechanisms further and pave the path towards individualized novel treatments similar to what is already being done in cancer genomics.
Within the Class I identifiers, TUBB1 encodes part of one of the core protein families that heterodimerize and assemble to form microtubules [54]. The tubulin β-1 chain is the major β-tubulin isotype expressed in megakaryocytes and platelets. Mutations or absence of TUBB1 is associated with enlarged rounded platelets and result in thrombocytopenia [37]. TUBB1 has been reported to be downregulated in AD; thus, it is not surprising that taxanes and other microtubule-targeting drugs restore lost nerve signals in AD and other neurodegenerative diseases [55]. The second Class I identifier, ASB4, encodes a protein that degrades filamin B proteins and plays a role in vascular differentiation and insulin signaling [56]. Asb-4 is co-localized and interacts with IRS4 in hypothalamic neurons [57]. The Asb-4 and IRS4 interaction mediates the degradation of IRS4, which in turn decreases insulin signaling, implicating ASB4 in energy homeostasis [58]. Asb-4 has also been associated with the regulation of inflammation, angiogenesis, and apoptosis via interactions with factor inhibiting HIF-1α (FIH) and TNF-α [59, 60]. Phosphodiesterases (PDEs) are responsible for the hydrolysis of cyclic adenosine monophosphate (cAMP) and cyclic guanosine monophosphate (cGMP). PDE inhibition is involved in neurodegenerative processes due to the regulation of cAMP and cGMP [61]. PDE5 is a cGMP-specific PDE and is upregulated in AD subjects compared to age-matched healthy controls [62]. PDE5 inhibitors, such as Sildenafil, have been suggested as Alzheimer’s drugs, leading to vascular smooth muscle relaxation, vasodilation, improved cognition, and restoring memory function [63–65]. Collectively, the Class I identifiers support the proposition of AD as a vascular disorder [38–40].
We described Class II by cellular signaling and differentiation due to being identified by NRG2 and ZNF3. NRGs are a member of epidermal growth factor (EGF)-related proteins, which stimulate ErbB-receptor tyrosine phosphorylation that elicits different downstream signaling pathways such as MAPK, PI3K, PKC, and JAK-STAT pathways and are associated with synaptic plasticity [41, 42]. Neuregulin-2 (Nrg2) dysregulation has been associated with cancer, schizophrenia, and AD [41]. Neuregulin-1 (Nrg1) is the primary substrate for Beta-secretase 1 (BACE-1), which is the only β-secretase that generates Aβ peptides. Although Nrg1 and Nrg2 are highly homologous, it remains unclear whether Nrg2 is also a BACE-1 substrate [66]. However, ADAM10 and BACE-2 cleave Nrg2 to generate a C-terminal fragment that serves as a substrate for γ-secretase [67]. Little remains known about NRG2; however, other members of the NRG family, including the more widely reported NRG1 and less known NRG3, have both been speculated to be involved in AD and cognitive impairment [68, 69]. In line with the overlying cell differentiation theme of Class II, ZNF3 is a transcription factor involved in cell differentiation and proliferation. In a recent GWAS, ZNF3 has been associated with AD along with NDUFS3 and MTCH2 [70]. ZNF3 interacts with BAG3, which is involved in ubiquitin/proteasomal functions in protein degradation and is regulated by the upstream binding of BACH1, whose target genes have roles in the oxidative stress response and control of the cell cycle [71]. AD-associated tau has been identified as a BACH1 target, making it a potential AD target [72]. However, a clear link explaining this subpopulation remains to be identified and warrants further investigations.
We propose that Class III, which is defined by IGF1, ASB4, and GTSE1 to be classified by metabolism. Several studies have reported impaired insulin receptor/IGF1 receptor signaling in AD subjects with decreased receptor expression, suggesting that AD is brain-type diabetes [73]. However, the association between IGF1 and AD remains controversial [74, 75]. Low IGF1 serum levels are associated with aging, one of the significant risk factors for AD. This suggests that high IGF1 may protect against neurodegeneration [60]. Some studies report that IGF1 enhances the transport of Aβ-carrier proteins into the brain and promotes transport across the blood-brain barrier [76].
In contrast, other studies have shown that long-term suppression of IGF1R signaling alleviates AD progression, providing protection from neuroinflammation and memory impairments induced by Aβ oligomers [77]. A recent study identified that within APOE ε4 carriers, there is a threshold at which IGF1R stimulating activity becomes associated with dementia [78]. Thus, IGF1 expression and response to IGF1 signaling may present as a way to stratify AD subjects into different subtypes. One study suggests that IRS4 may be a negative regulator of IGF1 signaling by suppressing other IRS proteins [79]. Given this link, the IGF1 signaling pathway presents an interesting way to classify AD subpopulations. IRS4 is reported to be the most downregulated gene in the insulin signaling pathway, with IRS genes implicated in tau phosphorylation [80]. Asb-4 co-localization with IRS4 mediates IRS4 degradation, which in turn decreases insulin signaling [57]. Given this link, the IGF1 signaling pathway represents a unique classification of AD subpopulations, as increased ASB4 would promote decreased insulin signaling, as would IRS4 downregulation. Considering GTSE1, which encodes a microtubule-associated protein, as the third prevalent identifier for Class III, there is overlap between Class I and Class III. Both TUBB1 and GTSE1 are involved in microtubule stability and formation, and ASB4 defines both classes. Thus, these two classes may actually represent one larger subpopulation that can further be defined or stratified on the basis of insulin/insulin-like growth factor signaling.
Class IV was defined by cDNA FLJ39269, ITGA1, and CPM. As mentioned, GUCY1A3 is the most closely associated gene to cDNA FLJ39269. GUCY1A3 encodes for a subunit of the guanylyl cyclase, a key enzyme in the NO signaling pathway, which catalyzes the conversion of GTP to cGMP, which in turn regulates the activity of protein kinases, PDEs, and ion channels [81]. Furthermore, GUCY1A3 has been associated with vascular dementia [82]. GUCY1A3 mutations are associated with NO signaling disruption that leads to hypertension [83]. ITGA1 encodes the α1 subunit of integrin receptors, which heterodimerizes with the β1 subunit to form a cell-surface receptor for collagen and laminin [84]. More specifically, the α1β1 complex has been associated with mediating the Aβ neurotoxic effect, playing an essential role in initiating events that lead to neurite degeneration in the presence of Aβ [85]. ITGA1 is downregulated in neuroplastin 65 (NP65) knockout mice, which exhibit abnormal cognition and emotional disorders that resemble AD characteristics [86]. CPM is a carboxypeptidase for peptides and proteins involved in inflammation and neuropeptide processing and has been found to be downregulated in the lymphocytes of AD subjects [87]. CPM is known to enhance NO output, playing a role in NO signaling under inflammatory conditions [49]. The AD patient population is characterized by chronic inflammation in the brain and are increasingly susceptible to infections, suggesting a possible link between CPM and AD [88]. NO has been implicated in AD neurotoxicity as NO-dependent pathways have been reported to contribute to cognitive decline and neurodegeneration [89]. As an inflammatory disease, NO synthesis is increased in the AD brain, which is thought to contribute to oxidative stress-associated neurodegeneration. However, there are reports of an early neuroprotective role of NO in AD that may be harnessed as a therapeutic strategy [90]. NO has been reported to impair autophagy by several mechanisms, with nitric oxide synthase (NOS) inhibition enhancing clearance of autophagic substrates and reducing neurodegeneration [91]. However, autophagy impairment has been reported in individuals with neurodegenerative diseases, and the causal mechanistic links between NO, autophagy and AD remain to be elucidated [92, 93]. Furthermore, there have been links with other carboxypeptidases to AD. Specifically, a new human mutation in the carboxypeptidase E (CPE)/neurotrophic factor-α1 (NF-α1) gene from an AD patient was found to cause memory deficit and depressive-like behavior in transgenic mice [94]. Thus, this AD subpopulation appears to be linked to NO, which has been implicated in AD neurodegeneration.
PDE5A, PSEN1, and NDUFS8 identify Class V, which we described as a mitochondrial subpopulation, that may suggest a familial role. PDE5 is upregulated in AD subjects compared to age-matched healthy controls, underscoring the use of PDE5 inhibitors to restore memory function and cognition [62, 63]. Even further, PDE5 inhibition has been shown to decrease Aβ load in models of AD [95]. Although PDE5A was the third most prominent identifier for Class I, it was the primary identifier for Class V. PDE inhibition is involved in neurodegenerative processes by regulating cAMP and cGMP concentrations [61]. cGMP-specific PDE5 is reported to be upregulated in AD subjects compared to age-matched healthy controls [62]. What stood out the most for this Class was that although PSEN1 mutations are the most common cause of autosomal dominant FAD [96], PSEN1 was not the primary identifier. Two hypotheses describe the role of PSEN1 on AD pathogenesis–the amyloid hypothesis and the presenilin hypothesis. The amyloid hypothesis proposes that PSEN1 mutations initiate AD pathogenesis by increasing the production of Aβ42, which contributes to amyloid plaque deposition. In contrast, the presenilin hypothesis proposes that PSEN1 mutations cause loss of function of presenilin in the brain, which triggers neurodegeneration and dementia [97]. Looking even further into this subpopulation, we noticed that SYK drives an additional difference within this group, also highlighting the complexity of the disease. SYK regulates Aβ production and tau hyperphosphorylation [98, 99]. The Aβ and the NO/cGMP pathway can stimulate synaptic plasticity and memory at low doses and inhibit them at high doses. With aging, the body’s ability to regulate the balance between oxidant and antioxidant systems decreases, resulting in an increased production of reactive oxygen and nitrogen species that result in tissue damage. This oxidative stress also promotes the accumulation of Aβ [95]. Furthermore, NDUFS8 being one of the identifiers for Class V, highlights the mitochondrial role of AD. Complex I has essential bioenergetic and metabolic functions and is a known source of reactive oxygen species, linking it to many hereditary and degenerative diseases [100].
Class VI was defined by DCAF17, cDNA FLJ75819, and SLC33A1. DCAF17 encodes a nuclear transmembrane protein associated with damaged DNA binding protein 1 ubiquitin ligase complex and is involved in iron accumulation in Globus pallidus and in white matter [51]. Similar to Class V, this highlights the role of mitochondrial dysfunction in AD pathogenesis. Within Class VI specifically, this highlights the pathological role of iron overload in the mitochondria to cause mitochondrial dysfunction [101]. It appears that iron overload-induced mitochondrial dysfunction is the driving difference between Class V and Class VI. This idea is reinforced with ZNF652, which, although not the identifier for Class VI, is the most closely associated gene to cDNA FLJ75819. ZNF652 is associated with metal protein and has been reported to be upregulated in severe AD [102, 103]. The third identifier of this subpopulation, SLC33A1, and its associated protein AT-1, are associated with the import of acetyl-CoA by regulating Nε-lysine acetylation of ER-resident and -transiting proteins, which causes a progeria-like phenotype that mimics an accelerated form of aging [104]. Mutations and increased expression of AT-1/SLC33A1 have been associated with several diseases, including neurologic, intellectual, and dysmorphic conditions, and have also been reported in LOAD subjects [105]. Interestingly, SLC33A1 mutations have also been associated with low serum copper [106]. Homeostasis of metal ions, including iron, copper, zinc, and calcium, in the brain is crucial for maintaining normal physiological functions–and an imbalance is closely related to the onset and progression of AD. This is due to metal ion dysregulation contributing to oxidative stress and the induction of tau and Aβ pathologies [107]. Although there appears to be an underlying role of metals or metal metabolism, this represents a subpopulation that warrants additional investigation to understand how they collectively contribute to AD pathology.
Interestingly, PSEN1 was the only one of the genes primarily associated with AD and increased AD risk (APOE ε4, APP, PSEN1, and PSEN2) to be a primary identifier for a perspective class. Although we only utilized AD subjects in the study, this highlights the heterogeneous nature of AD pathology. This explains why APOE does not show up in the analysis as a driving variable, and it was our intention to extract a refined vantage into AD outside of APOE findings. The heterogeneity of the AD population used, in terms of beta-amyloid status, also contributed to APOE mRNA counts to be insignificant compared to other gene expression products. Furthermore, there have been reports on the molecular differences in AD between males and females [108]. Thus, identifying whether certain classes or combinations of classes are more prevalent in males or females will continue to shed light on disease etiology. Females are at a greater risk of developing AD dementia, while males are at a greater risk of developing vascular dementia [109]. Analysis of our dataset revealed that 58.9% of males fell under the vasculogenesis perspective class, compared to only 38.7% of females. Surprisingly, only 16.5% of females fell under the mitochondrial perspective class. Gender has been reported to not only influence AD evolution directly but also through other comorbidity factors [110]. Note that the perspective classes discovered by the machine intelligence we are using offers a view into how AD progresses for different people and how different people evolve towards this phenotype through potentially different combinations of factors. The six progression mechanisms discussed here appear to be an essential part of this story. By providing an increased granularity into the mechanisms at play, the advent of AI and ML algorithms provide a means of expediting the drug repurposing and development process, particularly with respect to heterogeneous neurodegenerative diseases. This is because ML approaches permit the mining of different kinds of data that shed light on disease etiology through precise subpopulations, which can, in turn, assist in the discovery and development of effective anti-AD drugs. Future work will explore statistical evaluations of several subpopulations.
It is widely known that AD is a heterogeneous disease, yet AD drug trials often have broad inclusion criteria, not accounting for disease heterogeneity in trial design [111–113]. Stratifying treatment trial designs to account for disease heterogeneity using algorithms and omics data will lead to personalized medicine in AD drug development. Hypothesis-generating AI technologies like the one described in [28] are able to help usher disease definitions that precisely relate to the molecular machinery at play. The improvement to clinical trial outcomes can be substantial as we will be better able to select patients and match them with drug candidates.
Perspective analytics allowed us to understand an AD patient population in various ways with the goal of being able to precisely define the various mechanisms at play behind this complex disease and how these perspectives can improve clinical trial efforts in this space. It is possible that certain drugs that have been designed for AD are actually effective at improving the health of specific subpopulations, and even more possible that several drug candidates can be repositioned for specific subtypes. Here, we have identified six perspective classes corresponding to disease progression mechanisms that contribute to AD heterogeneity. The six perspective classes highlight the critical roles of vasculogenesis, cellular signaling and differentiation, metabolic function, mitochondrial function, NO, and metal ion metabolism. Although these specific AD patient subpopulations have not explicitly been identified previously, the genetic identifiers for each perspective have been implicated in AD. The ability to utilize a small dataset to extract such precise insights opens up the possibility to boil away much of the noise that exists within the AD field, redefining the way we think about AD as a set of diseases that emerge through various molecular pathways.
Many remarkable advances using machine intelligence have been made over the last several years. Computer vision applications have been given particular attention as the advent of convolutional neural networks are beautifully suited for these tasks. Similarly, other types of deep neural networks are currently being used for drug discovery. There is great potential that comes with creating a new taxonomy of disease for complex disorders. These efforts will allow researchers and pharmaceutical companies to derive precise and novel ways to attack these disorders through the drug paradigm or genetic engineering. It should be noted that oligomannate has been approved for the treatment of Alzheimer’s in China, and that aducanumab is currently seeking approval. It will be interesting to see how our efforts to understand patient subpopulations will influence the utilization of existing and future therapies.
Although results from this study are not exhaustive, they demonstrate that even within a relatively small study sample, next-generation machine intelligence is capable of uncovering multiple genetically driven subtypes. We hope to continue this work with a larger Alzheimer’s transcriptomic dataset so that we can continue to unravel the etiology behind dementia. In future analyses, we are considering the combinatorial aspects of the patient population within this dataset and from others. Are there certain combinations of the six perspective classes that are statistically more likely to occur? Machine intelligence has opened up a door that is allowing us to pursue therapies for neurodegeneration with a much finer granularity of understanding.
Abbreviations
AD: | Alzheimer’s disease |
AI: | artificial intelligence |
Aβ: | β-amyloid |
BACE-1: | Beta-secretase 1 |
cAMP: | cyclic adenosine monophosphate |
cGMP: | cyclic guanosine monophosphate |
CUL4: | cullin-4 |
DDB1: | DNA damage-binding protein 1 |
FAD: | familial Alzheimer’s disease |
GWAS: | genome-wide association study |
IRS4: | insulin receptor substrate 4 |
JAK-STAT: | Janus kinase-signal transducer and activator of transcription |
LOAD: | late onset Alzheimer’s disease |
MCI: | mild cognitive impairment |
ML: | machine learning |
NADH: | nicotinamide adenine dinucleotide |
NO: | nitric oxide |
NRG: | neuregulin |
PDE: | phosphodiesterase |
PRS: | polygenic risk score |
REO: | relative expression ordering |
SNP: | single nucleotide polymorphism |
SOCS: | suppressor of cytokine signaling |
SYK: | spleen tyrosine kinase |
Supplementary materials
The supplementary materials for this article are available at:
.Declarations
Author contributions
JG created the mathematics from which the machine intelligence techniques utilized were derived, curated the data, led the vision, and carried out a good portion of the research along with MT, who contributed essential bioinformatics. BQ wrote the majority of the manuscript and carried out critical protein interaction work that allowed us to interpret the results provided by the machine intelligence. RA and AA were both primary readers of the manuscript, provided direction, criticism, and helped shape the overall flow of the research during the course of this work. All authors contributed to manuscript revision, read, and approved the submitted version.
Conflicts of interest
The author Joseph Geraci declares that he owns substantial shares in NetraMark Corp, which funded a major portion of this study.
Ethical approval
Not applicable.
Consent to participate
Not applicable.
Consent to publication
Not applicable.
Availability of data and materials
The data for this work was extracted from data available at https://www.ebi.ac.uk/arrayexpress/experiments/E-GEOD-84422/?query=GSE84422.
Funding
This project was supported by NetraMark Corp., an AI company focused on advanced machine intelligence methods, and by graduate student support from Queen’s University for Bessi Qorri. The funders had no role in the study design, data collection and analysis, decision to publish, or preparation of the manuscript.
Copyright
© The Author(s) 2020.