Abstract
Aim:
Rheumatoid arthritis (RA) is an autoimmune disorder marked by an overgrowth of joint tissue and inflammation of the synovium. The mammalian target of rapamycin (mTOR), functioning as a serine/threonine protein kinase, is recognized for its role in controlling cell proliferation, metabolism, and inflammatory responses. While there is some evidence hinting at a link between RA and proteins downstream of mTOR, the findings are not definitive. In light of this, we have undertaken a Mendelian randomization (MR) analysis to investigate the potential connection between mTOR and RA.
Methods:
A two-sample MR study was performed by utilizing significant single nucleotide polymorphisms (SNPs) derived from a comprehensive genome-wide association study (GWAS) dataset, which included 58,284 samples of RA and 3,301 samples of mTOR-related proteins, as instrumental variables (IVs). The primary MR analysis techniques employed were inverse variance weighted (IVW), weighted median (WM), and MR-Egger regression. Additionally, sensitivity analyses were carried out on the IVs to evaluate heterogeneity and pleiotropy using MR-Egger, leave-one-out, and MR pleiotropy residual sum and outlier (MR-PRESSO) methods. Furthermore, to validate the robustness of the findings, replication analyses were also conducted using another RA GWAS dataset focusing on European populations.
Results:
The main findings indicated that there is no causal link between mTOR-related proteins and RA. The genetically elevated levels of mTOR-related proteins do not appear to raise the risk of RA [AKT (p = 0.721), ATF6 (p = 0.369), ATG7 (p = 0.112), BECN1 (p = 0.599), EIF4A3 (p = 0.652), EIF4B (p = 0.989)]. Additionally, the Cochran’s Q test did not detect any heterogeneity across all proteins. The MR-PRESSO analysis also found no evidence of pleiotropy. The replication cohort confirmed these results, showing once more that there is no connection between mTOR and RA, which suggests that the initial findings are both robust and reliable.
Conclusions:
Although earlier research has hinted at a possible link between mTOR pathway proteins and RA, our study does not endorse a causal relationship between the two. Additional studies are required to clarify the intricate mechanisms that drive RA and to determine the role of mTOR signaling in the disease process.
Keywords
Mendelian randomization, rheumatoid arthritis, mTOR, casual association, GWASIntroduction
Rheumatoid arthritis (RA) is a persistent autoimmune disorder marked by the overgrowth of joint tissue and inflammation of the synovium [1]. It significantly diminishes physical capabilities and the quality of life, imposing a considerable strain on both individuals and society [2]. The heritability of RA is approximately 60%, which points to a substantial genetic contribution to its onset [3, 4]. Although genome-wide association study (GWAS) have pinpointed numerous genetic susceptibility sites for RA, they account for only a small part of its genetic heritability (~12%) [5]. The molecular mechanisms leading to RA are not yet fully understood.
Various studies have indicated that the development of RA is affected by the interaction of different immune cells, with the mammalian target of rapamycin (mTOR) signaling pathway playing a significant role in this process. In mammals, mTOR functions as a serine/threonine kinase and serves as a central regulator of cellular metabolism. Disruptions in the mTOR signaling pathway have been associated with a range of human diseases. The phosphatidylinositol 3-kinase/AKT/mTOR (PI3K/AKT/mTOR) signaling pathway influences cell proliferation, resistance to apoptosis, angiogenesis, autophagy, and metastasis. For instance, this pathway can stimulate the proliferation of fibroblast-like synovial cells (FLS), and the excessive proliferation of FLS cells results in synovial hyperplasia—a critical pathological feature of RA. Its role in RA implies its potential for reducing joint swelling in RA patients [6]. Additionally, the medication rapamycin (like Shikonin), which targets mTOR, has exhibited a wide range of anti-proliferative and immunosuppressive effects, holding promise for treating RA-associated pathological conditions. However, despite the abundance of studies that clarify the association between mTOR and RA, they predominantly focus on molecular, cellular, or pharmacological viewpoints. The genetic association between mTOR and RA has not been clearly established. Given the ubiquity of GWAS as a method for identifying genetic variants related to diseases [7], is vital to explore the potential causal link between mTOR and RA. Nevertheless, it is difficult to establish a causal connection between the mTOR pathways and RA through observational studies in humans, we have endeavored to investigate these relationships to the best of our ability.
We have chosen 14 mTOR-associated proteins for further investigation. Within this group, the EIF family, HIF1, ULK, ATF, and RPS6K are associated with mTORC1, while AKT and PKC are linked to mTORC2. The EIF family, HIF1, ULK, ATF, and RPS6K are downstream targets of mTORC1, and the activation of mTOR results in the phosphorylation of these downstream targets. The phosphorylation of EIF4 and 4EBP alters their protein conformation, which subsequently impacts the transcription and translation processes during the cell cycle and cell replication. EIF4 and S6K are key players in the translational regulation of the mTOR signaling pathway and are involved in the activation of the PI3K/AKT pathway and the preservation of autophagic transcription factors. AKT can directly influence TSC1 and TSC2, PKC can affect TSC1 via RSK, and they can further influence mTORC1 and mTORC2 through the PI3K/AKT pathway. mTORC1 activates HIF1 and impacts the vascular endothelial growth factor (VEGF) pathway by acting on VEGF.
Mendelian randomization (MR), a sophisticated genetic epidemiological technique, is extensively applied in numerous studies to deduce the causality between selected exposures and outcomes [8, 9]. MR employs single nucleotide polymorphisms (SNPs) as instrumental variables (IVs) [10], which can significantly reduce the impact of confounding variables and reverse causality, thereby providing solid and valuable scientific evidence for causal relationships. It is also known as an alternative to randomized trials for the segregation of alleles at the time of conception. In this study, we conducted MR analyses to explore the potential causality between the levels of 14 downstream proteins of the mTOR pathway and the risk of developing RA.
Materials and methods
Study design and participant flowchart
In this research, a two-sample MR study was undertaken to assess the causal link between RA and mTOR-related proteins. We identified relevant SNPs by performing quality control procedures on the GWAS summary data, and these selected SNPs served as IVs for the MR analysis. The effectiveness of MR analysis hinges on three key assumptions: (1) the genetic instruments are correlated with mTOR; (2) the genetic instruments are unrelated to possible confounding factors; (3) the genetic instruments are linked to RA through mTOR (Figure 1).
An overview of the study design with three fundamental assumptions about the MR study. Assumption 1: The genetic variants selected as IVs should be strongly associated with the risk factor of interest. Assumption 2: The genetic variants used as IVs should not be associated with confounders. Assumption 3: The IVs should affect the risk of the outcome merely through the risk factor, not via any alternative pathways.
Data extraction
The exposure data pertaining to 14 downstream proteins of mTOR, including plasma levels of RPS6K, EIF4EBP2, EIF4E2, EIF4A3, EIF4B, EIF4G3, AKT, ATG7, ATF6, BECN1, HIF1A, ULK3, LST1, and PRKCA, were sourced from the INTERVAL study Proteomics GWAS. This study encompassed 3,301 European participants and involved the assay of 3,600 plasma proteins [11].
The summary statistics for RA were derived from a large-scale GWAS meta-analysis that included 14,361 cases, 43,923 controls, and 87,479,963 SNPs [5]. All cases of RA met the 1987 diagnostic criteria for RA established by the American College of Rheumatology [12] or were diagnosed with RA by a certified rheumatologist. Moreover, to mitigate the impact of population stratification, we exclusively extracted SNPs and samples from studies that focused on populations of European descent.
Selection of instrumental variables
In our research, we utilized genetic variations linked to mTOR as instrumental SNPs. A series of quality control measures were implemented to select SNPs that aligned with three key assumptions for use as IVs. Firstly, we targeted independent SNPs with a strong association with mTOR and a p-value threshold of less than 5e-8 for selection, although this criterion yielded a limited number of SNPs. To ensure a sufficient count of eligible IVs for further analysis, we adjusted the p-value threshold to 1e-5. Secondly, we established a linkage disequilibrium (LD) threshold for clustering at r2 < 0.001, applying a window size of 10,000 kb for clumping. For SNP pairs that exceeded this LD r2 threshold, we retained the one with the lower p-value. Thirdly, we excluded SNPs with a minor allele frequency (MAF) below 0.01 from the scope of complex disease GWAS. Moreover, to uphold the assumption of independence, we scrutinized these SNPs in PhenoScanner to weed out any potential confounding influences. Specifically, SNPs connected to habits like smoking, alcohol consumption, and factors such as body mass index (BMI) were deemed likely to skew results and were therefore excluded. Finally, to ensure that the effect of an SNP on the exposure and the effect of that SNP on the outcome must each correspond to the same allele, we drop all palindromic SNPs. Lastly, to ensure that an SNP’s impact on both the exposure and outcome corresponded to the same allele, we discarded all palindromic SNPs. To confirm that our selected IVs were robust enough to detect the exposure’s causal impact on the outcome, we calculated the F statistic for each. An F value exceeding 10 indicated a strong instrument. The SNPs that passed these criteria were advanced as the final genetic IVs for subsequent MR analysis.
Statistical analysis
For the statistical analysis in our MR study, we employed three methods: inverse variance weighted (IVW), weighted median (WM), and MR-Egger regression. These analyses were conducted using the R programming language (version 4.2.1) and the “TwoSampleMR” package.
The IVW method served as our primary approach to estimating the causal relationship between the exposure and the outcome. This method calculates the effect size by dividing the strength of the association between the SNP and the outcome by the strength of the association between the SNP and the exposure [13]. The MR-Egger regression is a weighted linear regression technique that can provide a consistent estimate of the causal effect even if all genetic IVs are invalid [14]. However, this method has lower precision and is more sensitive to the influence of outlier genetic variants. The WM method is designed to be resilient against outliers, ensuring consistent causal estimates even when approximately half of the genetic variants are invalid IVs [15]. These methods were chosen to provide a comprehensive analysis and to ensure the robustness of our findings in the MR study.
Sensitivity analysis
Additionally, sensitivity analyses were conducted to verify the reliability of the MR findings. Initially, Cochran’s Q statistic was utilized to evaluate the consistency among the IVs, with a p-value less than 0.05 indicating a statistically significant level of heterogeneity. Subsequently, MR-Egger regression was applied to investigate the presence of directional horizontal pleiotropy within our MR data set. The intercept from the MR-Egger regression was examined to assess if horizontal pleiotropy influenced the MR analysis, where a p-value less than 0.05 suggested its influence. The asymmetry observed in funnel plots also served as an indicator of potential horizontal pleiotropy. Furthermore, a leave-one-out analysis was implemented to discern if a specific SNP was driving the observed association. This step was crucial in establishing whether any one IV was predominantly influencing the causal relationship under examination.
Replication analysis
To rule out the chance findings that might arise from relying on a single data source, we further chose an additional set of summary statistics for RA from an extensive GWAS meta-analysis. This meta-analysis encompassed 97,173 RA subjects of European ancestry, consisting of 22,350 cases and 74,823 controls, for the replication analysis [16]. We excluded all non-autosomal SNPs, those with a call rate below 0.98, or a MAF less than 0.01, resulting in the inclusion of 8,218,396 SNPs for further analysis. The IV selection process and methods remained consistent with those detailed earlier, with MR analysis and sensitivity analysis carried out for 14 distinct protein exposures. The relevant clinical data from the RA GWAS datasets can be found in supplementary Table S1.
Results
Genetic variants selection
Genetic variation serves as a surrogate for risk factors in the MR approach to assess the causal effect of the risk factor in question. Our analysis focused on 14 proteins associated with mTOR, namely AKT, RPS6KA6, EIF4EBP2, EIF4B, EIF4G3, EIF4A3, EIF4E2, PKC-A, BECN1, ATG7, ATF6 (an activator of mTOR), ULK3, HIF-1a, and LST1. We applied a genome-wide significance threshold (p < 0.00001) and a clumping criterion (r2 < 0.001) to select the relevant SNPs for our MR analysis.
This stringent selection process allowed us to identify the following number of SNPs related to each protein: 5 for AKT, 14 for RPS6KA, 13 for EIF4EBP2, 12 for EIF4B, 11 for EIF4G3, 4 for EIF4A3, 11 for EIF4E2, 10 for PKC-A, 8 for BECN1, 6 for ATG7, 15 for ATF6, 7 for ULK3, 6 for HIF-1a, and 10 for LST1. After eliminating the interference of confounding factors (rs2072151 related to BMI) and SNPs (rs11708375, rs72806714) with palindromic structures, we got 10 SNPs associated with EIF4EBP2. For RPS6KA, 4 palindrome SNPs (rs1721376, rs17412698, rs2595942, rs6120946) were removing. For ATF6, we got rid of 3 SNPs (rs2276003, rs388081, rs61738953) with palindrome structure and rs8111 related to the confounding factor BMI. For EIF4G3, one SNP (rs891132) being palindromic with intermediate allele frequencies, 10 SNPs were selected as IVs in the final. Comprehensive details of the selected SNPs can be found in supplementary Table S2. All SNPs had F-statistic values exceeding 10, signifying that our chosen instruments were sufficiently robust.
Casual association of mTOR and rheumatoid arthritis
In summary, no causal link was established between genetically predicted mTOR-related proteins and RA. It appears that having a genetically elevated level of mTOR-related proteins does not heighten the risk of developing RA [AKT (p = 0.721), ATF6 (p = 0.369), ATG7 (p = 0.112), BECN1 (p = 0.599), EIF4A3 (p = 0.652), EIF4B (p = 0.989), EIF4E2 (p = 0.652), EIF4EBP2 (p = 0.182), EIF4G3 p = 0.903), HIF-1a (p = 0.363), ULK3 (p = 0.589), LST1 (p = 0.197), PKC-A (p = 0.496) and RPS6KA (p = 0.119)]. Furthermore, identical risk estimates were derived using both the MR-Egger and WM methods. Figure 2 and Figure 3 illustrate the forest and scatter plots, respectively, demonstrating the association between mTOR-related proteins and RA as analyzed by various methodologies.
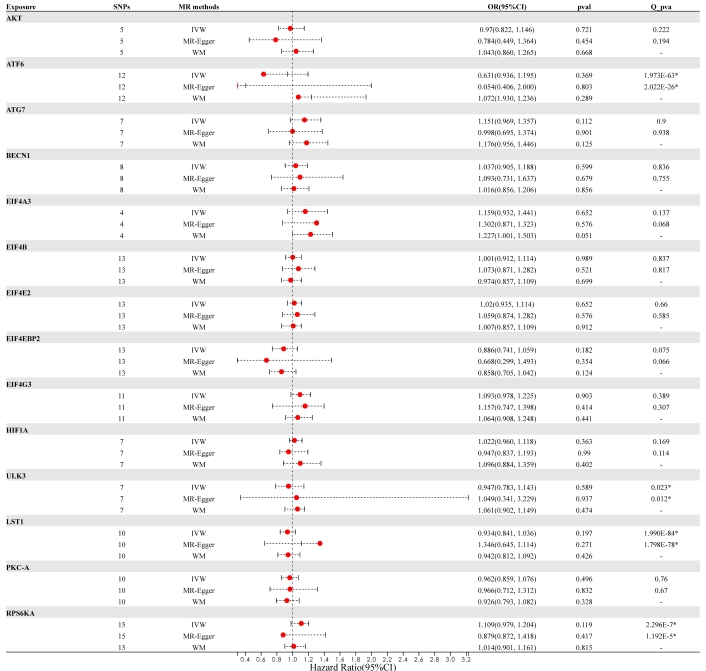
Comparison of Mendelian randomization (MR) results used by IVW, MR-Egger, and WM and visualization of forest plots. OR and 95% CI represent the change in the odds ratio of RA’s disease per 1-SD increase in the protein level. An asterisk (*) following the numbers indicates statistical significance at p < 0.05. CI: confidence interval; IVW: inverse variance weighted; OR: odds ratio; SNPs: single nucleotide polymorphisms; WM: weighted median
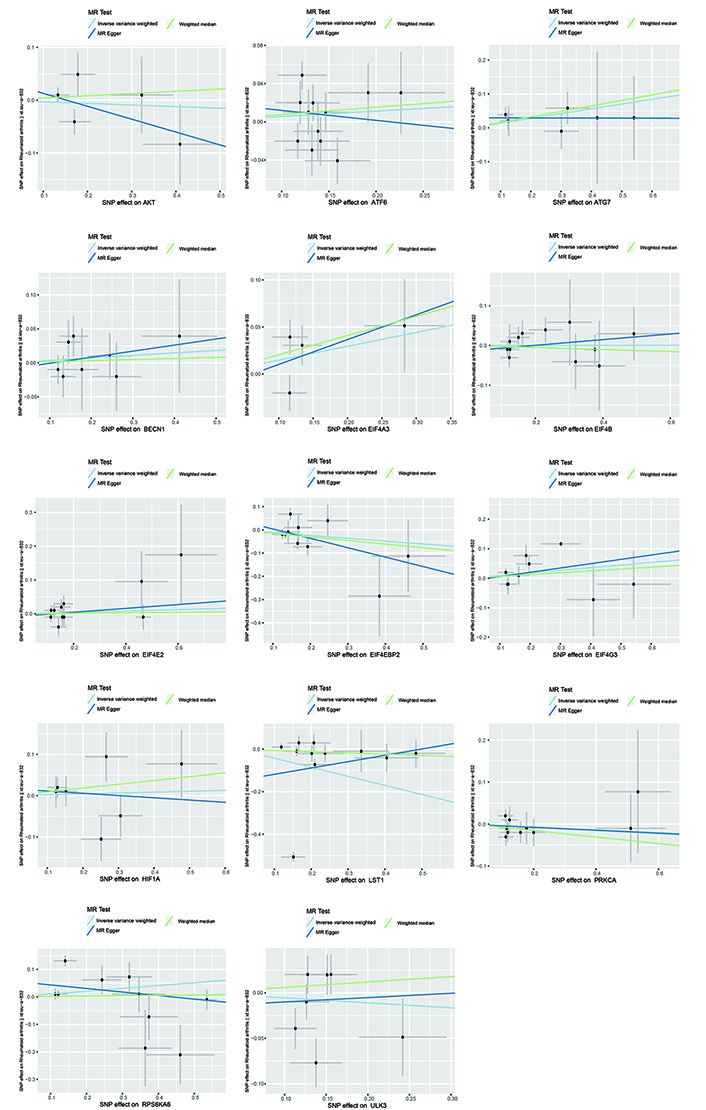
Scatter plots generated using the IVW, MR-Egger and WM methods in Mendelian randomization (MR) analysis. The scatter plot of MR analyses the causal effect between mTOR and RA using the conventional IVW, MR-Egger, and WM methods, and each line’s slope corresponds to the expected MR effect for each method. IVW: inverse variance weighted; SNPs: single nucleotide polymorphisms; WM: weighted median
Sensitivity analysis
The Cochran’s Q test indicated the presence of heterogeneity among ATF6, ULK3, LST1, and RPS6KA. Corresponding funnel plots corroborated these findings (Figure 4). Nevertheless, the intercept obtained from the MR-Egger regression analysis did not provide clear evidence of directional pleiotropy. The leave-one-out analysis demonstrated that the risk estimates remained notably consistent even when a single SNP was removed. No significant variations were observed in the causal estimates between mTOR and RA, suggesting that the identified associations were not influenced by any single IV (Figure 5).
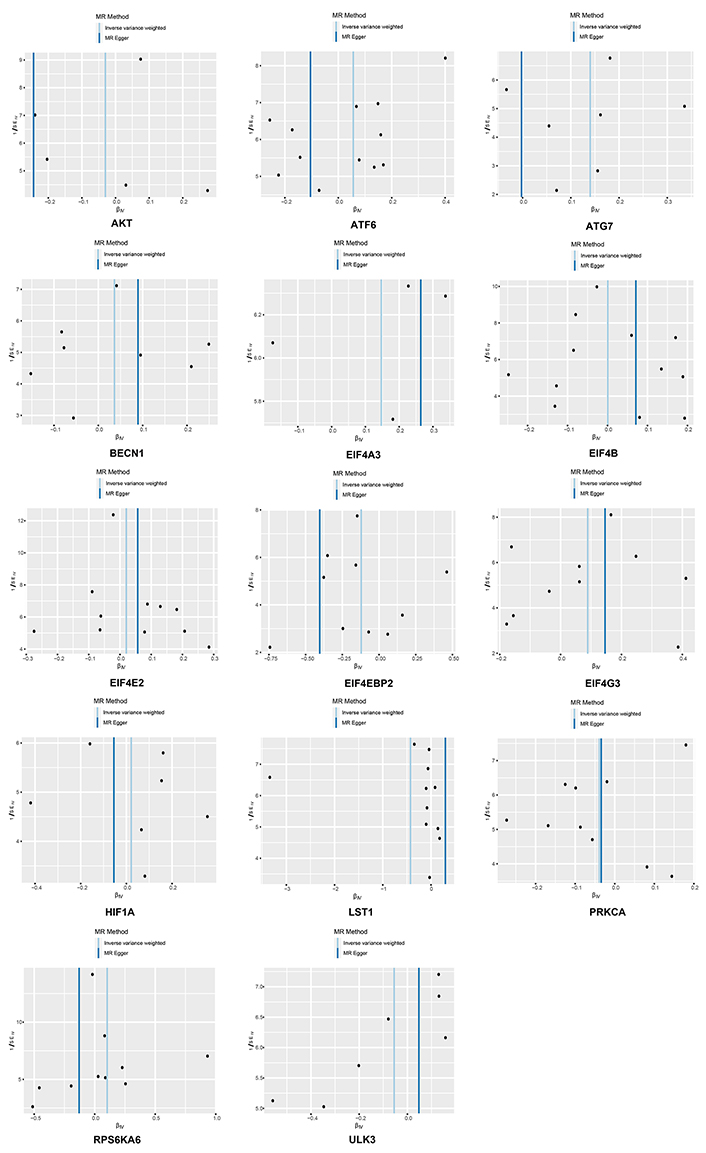
Funnel plots of inverse standard error estimations in Mendelian randomization (MR) analysis. The funnel plot shows the estimation using the inverse of the standard error of the causal estimate using each SNP as a tool. The vertical line shows the results of the IVW method using all SNPs. IVW: inverse variance weighted; SNPs: single nucleotide polymorphisms
The causal links between mTOR and RA are depicted using a leave-one-out plot. The leave-one-out figures depicted how removing a single variant altered the causal estimations (point with a horizontal line) for the effect of mTOR on RA.
Replication analysis
In the validation cohort, we effectively reproduced our initial observation that no causal link exists between mTOR-related proteins and RA (Table 1). The sensitivity analysis revealed heterogeneity among proteins ATF6, EIF4G3, ULK3, LST1, and RPS6KA, with a p-value less than 0.05, while no pleiotropy was detected (p-value greater than 0.05) across all 14 proteins based on the MR-Egger regression analysis (further details are presented in Supplementary Table S3). Furthermore, the leave-one-out analyses confirmed that the mTOR-RA association was not influenced by the exclusion of any specific SNP, indicating that no single SNP was exerting a decisive influence on the causal relationship, and thus confirming that our conclusion is both stable and dependable.
The results of replication MR analysis
Outcome | Exposure | SNP | IVW | MR-Egger | WM | ||||||
---|---|---|---|---|---|---|---|---|---|---|---|
OR | 95%CI | p | OR | 95%CI | p | OR | 95%CI | p | |||
GCST90038685 | AKT | 21 | 1.006 | (0.943, 1.072) | 0.866 | 1.051 | (0.906, 1.221) | 0.519 | 1.027 | (0.947, 1.114) | 0.524 |
ATF6 | 34 | 0.831 | (0.682, 1.014) | 0.068 | 0.54 | (0.294, 0.992) | 0.057 | 1.048 | (0.973, 1.129) | 0.213 | |
ATG7 | 18 | 1.044 | (0.953, 1.144) | 0.353 | 0.923 | (0.762, 1.118) | 0.425 | 1.037 | (0.925, 1.164) | 0.533 | |
BECN1 | 26 | 1.033 | (0.966, 1.105) | 0.346 | 0.972 | (0.825, 1.145) | 0.737 | 1.051 | (0.958, 1.152) | 0.29 | |
EIF4A3 | 13 | 1.059 | (0.996, 1.126) | 0.068 | 1.024 | (0.911, 1.150) | 0.705 | 1.031 | (0.955, 1.112) | 0.439 | |
EIF4B | 26 | 1.013 | (0.958, 1.071) | 0.65 | 1.082 | (0.956, 1.225) | 0.223 | 1.000 | (0.919, 1.089) | 0.994 | |
EIF4E2 | 24 | 0.994 | (0.943, 1.047) | 0.809 | 1.031 | (0.929, 1.145) | 0.570 | 1.012 | (0.937, 1.094) | 0.762 | |
EIF4EBP2 | 28 | 0.993 | (0.918, 1.074) | 0.861 | 1.064 | (0.863, 1.312) | 0.566 | 0.959 | (0.866, 1.064) | 0.433 | |
EIF4G3 | 21 | 1.009 | (0.916, 1.112) | 0.852 | 0.953 | (0.755, 1.204) | 0.694 | 0.982 | (0.873, 1.105) | 0.768 | |
HIF1A | 24 | 0.999 | (0.933, 1.070) | 0.969 | 0.963 | (0.833, 1.112) | 0.612 | 1.028 | (0.933, 1.132) | 0.579 | |
ULK3 | 19 | 1.006 | (0.917, 1.103) | 0.899 | 1.052 | (0.820, 1.350) | 0.697 | 1.001 | (0.905, 1.1.7) | 0.986 | |
LST1 | 24 | 0.829 | (0.614, 1.120) | 0.222 | 0.870 | (0.405, 1.868) | 0.724 | 0.976 | (0.895, 1.064) | 0.585 | |
PKC-A | 20 | 0.989 | (0.926, 1.057) | 0.750 | 0.988 | (0.853, 1.143) | 0.870 | 0.984 | (0.898, 1.079) | 0.735 | |
RPS6KA | 28 | 1.048 | (0.956, 1.150) | 0.315 | 0.930 | (0.797, 1.085) | 0.369 | 1.011 | (0.941, 1.087) | 0.763 |
CI: confidence interval; IVW: inverse variance weighted; MR: Mendelian randomization; OR: odds ratio; SNP: single nucleotide polymorphism; WM: weighted median
In conclusion, our study showed no indication supporting that the 14 downstream proteins of mTOR were causally associated with RA risk.
Discussion
This research pioneers the assessment of the causal link between mTOR-related proteins and RA, employing the MR methodology. Our findings provide no indication of a causal connection between the 14 mTOR proteins and RA.
The mTOR pathway comprises the mTORC1 and mTORC2 complexes. AKT, a serine/threonine kinase, is recognized as a key target of mTORC2. According to the research by Suchismita Raha and colleagues [17], the activation of the PI3K/AKT pathway acts upon mTOR. This pathway is significant in advancing chondrocyte apoptosis and autophagy [18], thereby establishing a close association between mTOR and the advancement of RA through autophagy. Moreover, AKT is also a notable feature in RA, particularly in the context of aberrant angiogenesis.
Earlier investigations have determined that angiogenesis is a key component in the onset of arthritis in RA. A substantial body of research has substantiated the relationship between the PI3K-AKT pathway and angiogenesis. This pathway is recognized as a primary influencer of various metabolic alterations, notably the heightened release of VEGF. The pathway also plays a regulatory part through the signaling of AKT1 and mTOR, which in turn prompts the activation of the hypoxia-inducible factor 1 (HIF-1) transcription factor downstream [19]. Concurrently, the AKT/mTOR pathway has the capacity to stimulate the proliferation and survival of aggressive immune cells and synoviocytes, which significantly contributes to the advancement of RA [20]. So that some metabolic alterations and enhanced inflammatory signals, such as tumor necrosis factor (TNF), might be responsible for increased mTOR activity in RA [21]. Bishop et al. [22] discovered that upon differentiation, human naive CD4 T cells emit TNF, which intensifies metabolic changes by triggering the metabolic reprogramming and activation of AKT and mTOR. Within the RA patient population, an upsurge in poorly differentiated CD4 T cells has been observed, potentially linking to the misregulation of their ongoing inflammatory reactions. Moreover, various studies have indicated that mTORC1 primarily enhances protein synthesis via the phosphorylation of its two principal downstream targets, S6 Kinase 1 (S6K1) and eukaryotic initiation factor 4E-binding protein (EIF4EBP). Furthermore, S6K1 phosphorylates the insulin receptor substrate (IRS), which then leads to a decrease in the activation of the PI3K pathway [23]. The pathways and phosphorylation events of these proteins are intertwined with the development of RA. Consequently, this substantiates the significant part played by EIF4EBP and S6K1 in the onset and progression of RA.
ATF6 plays a protective function by triggering the expression of genes that encode proteins for the endoplasmic reticulum (ER), which in turn boosts protein folding within this cellular compartment. Studies in various cell line models have demonstrated that the modulation of ATF6 is generally an adaptive response, thereby establishing its conventional role in cellular physiology [24–28]. Beth Levine and colleagues [29] discovered that ATG proteins participate in a broad spectrum of biological processes, extending well beyond the realm of autophagy. BECN1, functioning as an allosteric modulator of class III PI3K (PI3KC3) complexes, which include PI3KC3-C1 and PI3KC3-C2, is crucial for the process of autophagy. The PI3KC3-C1 complex, consisting of BECN1, VPS34, VPS15, ATG14, and Ambra, is involved in the nucleation of autophagic vesicles during the early stages of autophagosome formation. In contrast, the PI3KC3-C2 complex, which includes BECN1, VPS34, VPS15, and UVRAG, plays a role in the maturation of autophagolysosomes [30]. EIF4A3, a protein belonging to the eukaryotic translation initiation factor 4A family, serves as an RNA helicase and a key element of the exon junction complex. Mechanically, EIF4A3 is capable of preserving spliceosome integrity, which can help in maintaining basal autophagy levels by sequestering the critical autophagic transcription factor TFEB within the cytoplasm [31]. Findings from FISH assays revealed that circEIF4G3 is predominantly located in the cytoplasm. Here, circular RNAs (circRNAs) can potentially act as miRNA decoys, engage with RNA-binding proteins (RBPs), or even have a role in protein-coding [32–34]. The initiation of autophagy was associated with the ongoing suppression of MAP1LC3 gene expression by molecular events occurring further upstream, and it was discovered that ULK3-dependent activation of GLI1 played a role in the increased transcription of the DNMT3A gene following the induction of autophagy. Concurrently, certain studies have brought to light a novel function for ULK3 in autophagy initiation and its contribution to GLI1-driven DNMT3A gene methylation [35]. The protein LST1, encoded by the LST1 gene (alternatively known as B144), is situated within the MHCIII locus. This particular genomic region is also home to a multitude of other genes with significant immunological roles, including those that encode for Lymphotoxin-β, TNF-α, various complement proteins, and more. Consequently, LST1 plays a part in modulating leukocyte homeostasis and inflammatory responses [36]. Regarding the protein PRKCA, studies have indicated that it can modulate a variety of physiological processes, including those related to lung injury. Liu et al. [37] proposed that the PKC signaling pathway might be triggered by mechanical ventilation, which is associated with ventilator-induced lung injury. Nevertheless, there exists a debate surrounding its precise function, as some investigators have discovered that PRKCA could also serve a protective role in lung injury.
In RA, a combination of genetic and environmental factors initiates an autoimmune response that predominantly targets the joints [4]. The condition evolves from an initial phase characterized by a loss of immune tolerance and the emergence of autoantibodies to a chronic state marked by persistent inflammation and tissue damage. This chronicity is fueled by ongoing interactions between stromal cells and immune cells that have infiltrated the joint [38]. Within the joint’s inner lining, there is a proliferation of FLS and macrophage-like synovial cells (MLS), leading to the invasion and destruction of neighboring cartilage and bone by pannus. Activated FLSs are instrumental in the etiology and progression of RA, mediating damage to cartilage and bone. Additionally, macrophages play a significant role in RA pathogenesis; mir-100-5p is notably downregulated in bone marrow derived macrophages-small extracellular vesicles (BMDM-SEV), impairing mTOR’s capacity to regulate the invasion and growth of RA-FLSs. TNF released by macrophages can activate FLSs, and conversely, activated FLSs can stimulate macrophages, creating a positive feedback loop that intensifies the disease’s inflammatory cycle [39]. FLSs in RA, once activated, can migrate to and invade adjacent joints, causing joint degradation, and can also move to the lower layers of the synovial lining to promote angiogenesis and increase synovial hyperplasia and bone destruction [40, 41]. Meanwhile, the mTOR signaling pathway is known to regulate the invasiveness of RA-FLSs [42]. Numerous studies have shown mTOR to be activated in the rheumatoid synovium [21]. A recent investigation revealed that rapamycin can effectively suppress the expression of proteins in the AKT/mTORC1 pathway within RA-FLSs [43]. Inhibition of mTOR in FLSs reduces the activity of the transcription factor signal transducer and activator of transcription 1 (STAT1), which is responsible for the expression of many pro-inflammatory genes in RA [21]. Furthermore, it has been demonstrated that mTOR inhibition can prevent osteoclast formation, potentially halting bone erosion and cartilage loss in RA [44]. While some small trials hint that mTOR inhibition could be beneficial for rheumatic diseases, especially in systemic lupus erythematosus (SLE), its applicability to RA requires further investigation, as it is a distinct rheumatic condition.
As previously discussed, mTOR is a regulator of numerous cellular activities and plays a role in the onset of RA. Consequently, a variety of mTOR-targeting medications have been explored for the treatment of RA. Rapamycin, a prominent inhibitor of mTOR, is capable of managing T-cell proliferation triggered by antigens and curbing arthritic symptoms, thus enhancing the immune system’s function in individuals with RA [45]. However, despite rapamycin and its related compounds being extensively tested in clinical trials, the outcomes have often been disappointing, possibly due to rapamycin’s role in halting the cell cycle [46]. Additionally, second-generation mTOR inhibitors, such as the dual PI3K and mTOR inhibitor NVP-BEZ23527, have been shown to expedite the apoptosis of osteoclasts. NAC, an antioxidant and anti-inflammatory substance, has been demonstrated to prevent chondrocyte death and cartilage degradation in a rat model of RA [47]. However, prolonged oral use of NAC has been linked to an increased risk of RA [48]. These studies propose that mTOR pathway targeting is a potential therapeutic strategy for RA, albeit one that cannot escape the issue of treatment variability. Our findings, which indicate no direct causality between mTOR and RA, offer some etiological explanation for the variability in treatment responses. Nevertheless, further research is needed to uncover the precise mechanisms involved.
Our study possesses numerous advantages. Firstly, to our knowledge, it stands as the inaugural MR study to probe the causal nexus between mTOR and the risk factors associated with RA. Subsequently, we conducted these analyses multiple times utilizing various methodologies, yielding congruent findings. Furthermore, sensitivity analysis was deployed to affirm that our results were not prone to bias. Thirdly, we ruled out the impact of BMI, alcohol consumption, smoking, TM2D, and educational level as potential confounding elements. Additionally, MR employs genetic variants as surrogates for specific biomarkers, enabling the exploration of putative causal connections between biomarkers and clinical endpoints. MR offers a methodology for gauging causal impacts from genetic data, thereby furnishing supplementary insights akin to those from randomized controlled trials. Randomized controlled trials often appraise treatment efficacy but seldom elucidate the intrinsic mechanistic pathways. Conversely, MR studies are capable of evaluating the causal impacts on these underlying mechanistic pathways. However, our study is not without its limitations. For instance, the prevalence and mortality rates of RA differ across ethnicities. Given that all participants in this MR analysis were of European descent, it becomes challenging to extrapolate the potential causal relationship between mTOR and RA to other demographic groups.
In conclusion, while we are unable to definitively determine from a genetic standpoint that higher levels of mTOR protein could elevate the risk of RA, this study’s findings have certainly enhanced our comprehension of genetic correlations. They may also offer fresh perspectives on the functional mechanisms that underlie the connections among SNPs, mTOR, and RA. The involvement of mTOR in RA is clear, yet there is a recognized need for additional innovative approaches to further delve into this complex relationship in future research.
Abbreviations
BMI: | body mass index |
FLS: | fibroblast-like synovial cells |
GWAS: | genome-wide association study |
IVs: | instrumental variables |
IVW: | inverse variance weighted |
MR: | Mendelian randomization |
MR-PRESSO: | MR pleiotropy residual sum and outlier |
mTOR: | mammalian target of rapamycin |
PI3K: | phosphatidylinositol 3-kinase |
RA: | rheumatoid arthritis |
SNPs: | single nucleotide polymorphisms |
S6K1: | S6 Kinase 1 |
TNF: | tumor necrosis factor |
VEGF: | vascular endothelial growth factor |
WM: | weighted median |
Supplementary materials
The supplementary tables for this article are available at: https://www.explorationpub.com/uploads/Article/file/1001264_sup_1.pdf.
Declarations
Author contributions
ZY: Conceptualization, Validation, Writing—original draft. JL: Data curation, Formal analysis, Visualization, Writing—original draft. RZ: Conceptualization, Writing—original draft. HZ: Data curation, Formal analysis, Visualization. SZ: Validation, Supervision, Writing—review & editing.
Conflicts of interest
The authors declare that they have no conflicts of interest.
Ethical approval
The study utilized summary statistics from publicly available datasets, the IEU database. As such, this research did not involve direct contact with human participants or the collection of new personal data, and therefore, the ethical approval was not required.
Consent to participate
This study analyzed secondary data from the IEU database, therefore, the consent to participate is not required.
Consent to publication
Not applicable.
Availability of data and materials
Publicly available datasets were analyzed in this study. All of our data comes from the IEU database. This data can be found here: IEU OpenGWAS project (mrcieu.ac.uk). The raw data supporting the conclusions of this manuscript will be made available by the authors, without undue reservation, to any qualified researcher.
Funding
This work was supported by the National Natural Science Foundation of China [No. 82001740]. The funders had no role in study design, data collection and analysis, decision to publish, or preparation of the manuscript.
Copyright
© The Author(s) 2024.